
An official website of the United States government
The .gov means it’s official. Federal government websites often end in .gov or .mil. Before sharing sensitive information, make sure you’re on a federal government site.
The site is secure. The https:// ensures that you are connecting to the official website and that any information you provide is encrypted and transmitted securely.
- Publications
- Account settings
- My Bibliography
- Collections
- Citation manager

Save citation to file
Email citation, add to collections.
- Create a new collection
- Add to an existing collection
Add to My Bibliography
Your saved search, create a file for external citation management software, your rss feed.
- Search in PubMed
- Search in NLM Catalog
- Add to Search
About sleep's role in memory
Affiliation.
- 1 Division of Biopsychology, Neuroscience Center Zurich, University of Zurich, Zurich, Switzerland. [email protected]
- PMID: 23589831
- PMCID: PMC3768102
- DOI: 10.1152/physrev.00032.2012
Over more than a century of research has established the fact that sleep benefits the retention of memory. In this review we aim to comprehensively cover the field of "sleep and memory" research by providing a historical perspective on concepts and a discussion of more recent key findings. Whereas initial theories posed a passive role for sleep enhancing memories by protecting them from interfering stimuli, current theories highlight an active role for sleep in which memories undergo a process of system consolidation during sleep. Whereas older research concentrated on the role of rapid-eye-movement (REM) sleep, recent work has revealed the importance of slow-wave sleep (SWS) for memory consolidation and also enlightened some of the underlying electrophysiological, neurochemical, and genetic mechanisms, as well as developmental aspects in these processes. Specifically, newer findings characterize sleep as a brain state optimizing memory consolidation, in opposition to the waking brain being optimized for encoding of memories. Consolidation originates from reactivation of recently encoded neuronal memory representations, which occur during SWS and transform respective representations for integration into long-term memory. Ensuing REM sleep may stabilize transformed memories. While elaborated with respect to hippocampus-dependent memories, the concept of an active redistribution of memory representations from networks serving as temporary store into long-term stores might hold also for non-hippocampus-dependent memory, and even for nonneuronal, i.e., immunological memories, giving rise to the idea that the offline consolidation of memory during sleep represents a principle of long-term memory formation established in quite different physiological systems.
PubMed Disclaimer
Typical human sleep profile and…
Typical human sleep profile and sleep-related signals. A : sleep is characterized by…
Effects of sleep and wake…
Effects of sleep and wake intervals of different length after learning on memory…
A model of active system…
A model of active system consolidation during sleep. A : during SWS, memories…
Odor-induced reactivations during SWS benefit…
Odor-induced reactivations during SWS benefit memory consolidation. A : procedures: participants learned a…
Probing the functional relevance of…
Probing the functional relevance of slow oscillatory activity for memory processes by transcranial…
Influence of cholinergic activity on…
Influence of cholinergic activity on memory consolidation during wakefulness and sleep. A :…
Different time courses of plasticity…
Different time courses of plasticity in the hippocampus and neocortex. A : concept:…
Sleep-dependent formation and reactivation of…
Sleep-dependent formation and reactivation of song memory in birds. A : the measure…
Sleep supports the initiation of…
Sleep supports the initiation of an adaptive immune response. A : concept: the…
Similar articles
- Neural reactivation during human sleep. Denis D, Cairney SA. Denis D, et al. Emerg Top Life Sci. 2023 Dec 22;7(5):487-498. doi: 10.1042/ETLS20230109. Emerg Top Life Sci. 2023. PMID: 38054531 Free PMC article. Review.
- System consolidation of memory during sleep. Born J, Wilhelm I. Born J, et al. Psychol Res. 2012 Mar;76(2):192-203. doi: 10.1007/s00426-011-0335-6. Epub 2011 May 4. Psychol Res. 2012. PMID: 21541757 Free PMC article. Review.
- The memory function of sleep. Diekelmann S, Born J. Diekelmann S, et al. Nat Rev Neurosci. 2010 Feb;11(2):114-26. doi: 10.1038/nrn2762. Epub 2010 Jan 4. Nat Rev Neurosci. 2010. PMID: 20046194 Review.
- The contribution of sleep to hippocampus-dependent memory consolidation. Marshall L, Born J. Marshall L, et al. Trends Cogn Sci. 2007 Oct;11(10):442-50. doi: 10.1016/j.tics.2007.09.001. Epub 2007 Oct 1. Trends Cogn Sci. 2007. PMID: 17905642 Review.
- Sleep to remember. Born J, Rasch B, Gais S. Born J, et al. Neuroscientist. 2006 Oct;12(5):410-24. doi: 10.1177/1073858406292647. Neuroscientist. 2006. PMID: 16957003 Review.
- Does sleep benefit source memory? Investigating 12-h retention intervals with a multinomial modeling approach. Berres S, Erdfelder E, Kuhlmann BG. Berres S, et al. Mem Cognit. 2024 Jun 3. doi: 10.3758/s13421-024-01579-8. Online ahead of print. Mem Cognit. 2024. PMID: 38831160
- Neurobiology of cognitive abilities in early childhood autism. Denisova K. Denisova K. JCPP Adv. 2024 Jan 20;4(2):e12214. doi: 10.1002/jcv2.12214. eCollection 2024 Jun. JCPP Adv. 2024. PMID: 38827984 Free PMC article.
- Serum levels of neurotensin, pannexin-1, and sestrin-2 and the correlations with sleep quality or/and cognitive function in the patients with chronic insomnia disorder. Su AX, Ma ZJ, Li ZY, Li XY, Xia L, Ge YJ, Chen GH. Su AX, et al. Front Psychiatry. 2024 May 13;15:1360305. doi: 10.3389/fpsyt.2024.1360305. eCollection 2024. Front Psychiatry. 2024. PMID: 38803679 Free PMC article.
- Deciphering the Link: Correlating REM Sleep Patterns with Depressive Symptoms via Consumer Wearable Technology. Crișan CA, Stretea R, Bonea M, Fîntînari V, Țața IM, Stan A, Micluția IV, Cherecheș RM, Milhem Z. Crișan CA, et al. J Pers Med. 2024 May 14;14(5):519. doi: 10.3390/jpm14050519. J Pers Med. 2024. PMID: 38793101 Free PMC article.
- Delineating memory reactivation in sleep with verbal and non-verbal retrieval cues. Guttesen AÁV, Denis D, Gaskell MG, Cairney SA. Guttesen AÁV, et al. Cereb Cortex. 2024 May 2;34(5):bhae183. doi: 10.1093/cercor/bhae183. Cereb Cortex. 2024. PMID: 38745557 Free PMC article.
- Abel M, Bäuml KT. Retrieval-induced forgetting, delay, and sleep. Memory 20: 420–428, 2012 - PubMed
- Abel M, Bäuml KT. Sleep can eliminate list-method directed forgetting. J Exp Psychol Learn Mem Cogn 2012 - PubMed
- Abraham WC, Robins A. Memory retention: the synaptic stability versus plasticity dilemma. Trends Neurosci 28: 73–78, 2005 - PubMed
- Abraham WC, Williams JM. LTP maintenance and its protein synthesis-dependence. Neurobiol Learn Mem 89: 260–268, 2008 - PubMed
- Achermann P. The two-process model of sleep regulation revisited. Aviat Space Environ Med 75: A37–43, 2004 - PubMed
Publication types
- Search in MeSH
Related information
Linkout - more resources, full text sources.
- Europe PubMed Central
- PubMed Central
Other Literature Sources
- The Lens - Patent Citations
- scite Smart Citations
- MedlinePlus Health Information
- Citation Manager
NCBI Literature Resources
MeSH PMC Bookshelf Disclaimer
The PubMed wordmark and PubMed logo are registered trademarks of the U.S. Department of Health and Human Services (HHS). Unauthorized use of these marks is strictly prohibited.
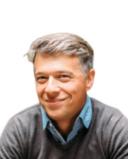
Sleep and Memory: How They Work Together
New research indicates a poor night’s sleep negatively impacts brain function..
Posted August 19, 2019 | Reviewed by Jessica Schrader
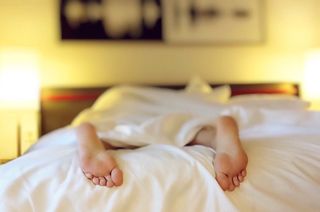
Medieval philosopher Thomas Aquinas once wrote that “sorrow can be alleviated by good sleep.” Now, scientists are learning he was not far from the truth.
A study published in July 2019 in the journal Current Biology indicates a poor night’s sleep—specifically, restless rapid-eye-movement ( REM ) sleep—negatively impacts brain function, including the work of amygdalae. These are almond-sized clusters of nuclei located deep within the brain’s temporal lobes and responsible for the consolidation of memories for long-term learning, as well as the processing and storage of memories associated with events that elicit strong emotions like sorrow, embarrassment , fear , and anxiety . Upon awakening, study volunteers who experienced disrupted REM sleep remained reactive to emotional events from the previous day while well-rested individuals labeled prior-day events as being of lesser emotional significance than they originally thought, according to the researchers.
We have long been aware that a good night's sleep benefits mood, alertness, concentration , and judgment. Science also has established that sleep plays a vital role in memory retention. What we have not known clearly, at least until now, how these sleep and memory processes, are potentially linked and how a negative impact on how one affects the other.
Study authors writing in Proceedings of the National Academy of Sciences in 2018 suggest even a single night of sleep deprivation can cause beta-amyloid, a metabolic waste product, to accumulate in brain structures, including the amygdalae, which regulate mood, emotion , memory, and ability to learn and are implicated in development of Alzheimer’s disease. The amygdalae maintain neural pathways to the hypothalamus, which regulates important biological systems, such as sleep, the menstrual cycle, and circadian rhythm , and they interact with the hippocampus, a key component in memory processing. In fact, neural circuits connecting the hippocampus to other regions of the brain are considered repositories for the storage of episodic memories, specifically events, their location, and the emotions associated with them.
For memory to function properly, three vital processes must occur:
- Acquisition: learning or experiencing something new
- Consolidation: integrating the new information in the brain, making it stick
- Recall: accessing the information after it is stored
Acquisition and recall occur when one is awake; consolidation, while asleep. When awake, the brain reacts to external stimuli and encodes new memories that are, at that point, unstable and subject to forgetting. The sleeping brain, with greatly reduced exposure to external stimuli, provides optimal conditions for memory consolidation, which strengthens and integrates new memory into existing knowledge networks.
At one time, experts thought sleep simply protected memory from interference by external stimuli. Now we know that both REM and slow-wave sleep (SWS) take more active roles in memory consolidation, with different kinds of memories being processed during different stages of sleep. A study in a 2018 issue of the Journal of Sleep Research, for example, indicates that one night of sleep loss can impair working memory, which is important for reasoning and planning.
However, of more concern, study participants most affected by sleep deprivation, women, were unaware of the decline in their performance, increasing their risk for accidents and mistakes. The relationship between car crashes and sleep deprivation is a prime example of such a risk. Other studies suggest that declarative memory, which is fact-based, benefits primarily from sleep periods dominated by SWS, and procedural memory, remembering how to do something, is related to REM sleep.
Although, as physicians and scientists, we still have much to learn about sleep and memory, we can say with certainty that a good night’s sleep improves concentration for learning and remembering what we learned. Here are a few tips for improving the quantity and quality of sleep:
- Exercise earlier in the day—not several hours before bedtime.
- Reduce or avoid stimulants such as caffeine later in the day and alcohol in the evening.
- Limit naps to 30 minutes; don't nap after midday.
- Stick to a sleep schedule; go to bed and wake up about the same time each day, including weekends and holidays.
- Relax and clear the mind before bedtime; read a book, listen to quiet music.
- Keep the bedroom cooler at night. Use “white noise” like that from a fan motor to mask distracting sounds. Install room-darkening shades.
- Make sure your mattress is comfortable and try sleeping on one pillow—not two or three.
- Don't eat a heavy meal or drink an excessive amount of liquid just prior to bedtime.
- Avoid using a computer, tablet, or smartphone right before going to bed. The light from the screen stimulates the brain, making it difficult to fall asleep.
Sleep architecture or quality is as important as quantity. Proper bed and wake times allow us to go through the phases of slow-wave and REM sleep. Alcohol, sedatives, and many drugs can also diminish slow wave as well as REM sleep. And a cool bedroom, or hot bath before bed, enhances deep, slow-wave sleep.
Current Biology/July 2019; https://www.cell.com/current-biology/fulltext/S0960-9822(19)30761-4?_re…
Consumer-Related Overview of Study of Current Biology Study; https://www.the-scientist.com/news-opinion/karaoke-sleep-study-links-di…
Proceedings of the National Academy of Sciences (2018); https://www.pnas.org/content/pnas/115/17/4483.full.pdf
Journal of Sleep Research (2018); https://onlinelibrary.wiley.com/doi/full/10.1111/jsr.12651
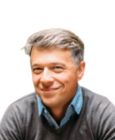
Alex Dimitriu M.D . applies expertise in sleep medicine and in psychiatry to help people obtain balance and improve performance.
- Find a Therapist
- Find a Treatment Center
- Find a Psychiatrist
- Find a Support Group
- Find Online Therapy
- United States
- Brooklyn, NY
- Chicago, IL
- Houston, TX
- Los Angeles, CA
- New York, NY
- Portland, OR
- San Diego, CA
- San Francisco, CA
- Seattle, WA
- Washington, DC
- Asperger's
- Bipolar Disorder
- Chronic Pain
- Eating Disorders
- Passive Aggression
- Personality
- Goal Setting
- Positive Psychology
- Stopping Smoking
- Low Sexual Desire
- Relationships
- Child Development
- Self Tests NEW
- Therapy Center
- Diagnosis Dictionary
- Types of Therapy
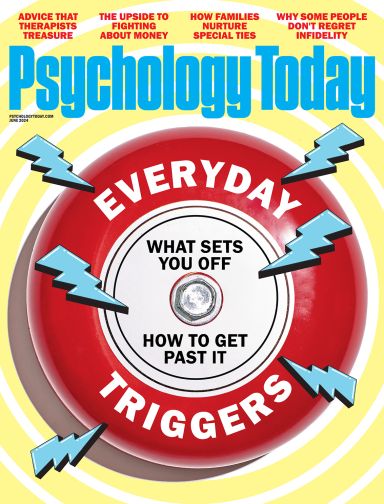
At any moment, someone’s aggravating behavior or our own bad luck can set us off on an emotional spiral that could derail our entire day. Here’s how we can face triggers with less reactivity and get on with our lives.
- Emotional Intelligence
- Gaslighting
- Affective Forecasting
- Neuroscience
Click through the PLOS taxonomy to find articles in your field.
For more information about PLOS Subject Areas, click here .
Loading metrics
Open Access
Peer-reviewed
Research Article
Sleep Improves Memory: The Effect of Sleep on Long Term Memory in Early Adolescence
* E-mail: [email protected] (WEB); [email protected] (KTP)
Affiliation Department of Human Biology, Brown University, Providence, Rhode Island, United States of America
Affiliation Department of Psychiatry and Human Behavior, University of California Irvine, Irvine, California, United States of America
- Katya Trudeau Potkin,
- William E. Bunney Jr
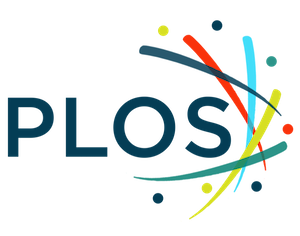
- Published: August 7, 2012
- https://doi.org/10.1371/journal.pone.0042191
- Reader Comments
Sleep plays an important role in the consolidation of memory. This has been most clearly shown in adults for procedural memory (i.e. skills and procedures) and declarative memory (e.g. recall of facts). The effects of sleep and memory are relatively unstudied in adolescents. Declarative memory is important in school performance and consequent social functioning in adolescents. This is the first study to specifically examine the effects of normal sleep on auditory declarative memory in an early adolescent sample. Given that the majority of adolescents do not obtain the recommended amount of sleep, it is critical to study the cognitive effects of normal sleep. Forty male and female normal, healthy adolescents between the ages of ten and fourteen years old were randomly assigned to sleep and no sleep conditions. Subjects were trained on a paired-associate declarative memory task and a control working memory task at 9am, and tested at night (12 hours later) without sleep. The same number of subjects was trained at 9pm and tested 9am following sleep. An increase of 20.6% in declarative memory, as measured by the number correct in a paired-associate test, following sleep was observed compared to the group which was tested at the same time interval without sleep (p<0.03). The performance on the control working memory task that involved encoding and memoranda manipulation was not affected by time of day or relationship to sleep. Declarative memory is significantly improved by sleep in a sample of normal adolescents.
Citation: Potkin KT, Bunney WE Jr (2012) Sleep Improves Memory: The Effect of Sleep on Long Term Memory in Early Adolescence. PLoS ONE 7(8): e42191. https://doi.org/10.1371/journal.pone.0042191
Editor: Antonio Verdejo García, University of Granada, Spain
Received: February 10, 2012; Accepted: July 4, 2012; Published: August 7, 2012
Copyright: © Potkin, Bunney Jr. This is an open-access article distributed under the terms of the Creative Commons Attribution License, which permits unrestricted use, distribution, and reproduction in any medium, provided the original author and source are credited.
Funding: The authors have no support or funding to report.
Competing interests: The authors have declared that no competing interests exist.
Introduction
Several studies primarily in adults have shown that sleep improves procedural memory, i.e. skills and procedures [1] , [2] as well as declarative memory [3] . REM and slow-wave sleep (SWS) have been implicated in memory consolidation [3] – [5] . Lack of REM sleep is associated with poor recall of visual location [6] . Decline in declarative memory consolidation is correlated with a decline in slow-wave sleep [7] . Spencer et al. observed similar initial procedural learning in older and younger adults; however, the older adults’ performance did not improve following sleep, suggesting that sleep dependent memory consolidation decreases with age [8] . This may reflect the disturbed sleep and disrupted SWS in the elderly [3] , [8] , [9] . Slow wave sleep increases until shortly before puberty and then shows a prominent drop across adolescence, decreasing by more than 60% between ages 10 and 20 years [10] . It is critical to understand the cognitive effects of normal sleep in order to understand the consequences of disrupted sleep. This is important since the majority of adolescents do not obtain the recommended amount of sleep and that disrupted sleep is a key symptom in most adolescent psychiatric and developmental disorders [11] .
Backhaus et al. studied twenty-seven children with an average age of 10.1 years (range of nine to twelve), on a learned word pairs list, employing a within subject design and two post-learning assessments. They found that declarative memory was significantly increased immediately after an interval of sleep, as well as with delayed post-learning sleep [12] . As the authors had noted, no control task was administered to determine if circadian confounds were responsible for this increase in recall post sleep. Our study addressed this limitation by administering a control task and evaluating the effect of sleep on auditory declarative memory consolidation in early adolescence. Visual declarative memory has been reported to be enhanced following sleep in children; however, auditory declarative memory has not been previously studied [13] .
Participants
Twenty female and twenty male adolescents, between the ages of 10 and 14, were recruited in a public middle school. The study was considered exempt by the institutional review board because it involved the use of educational tests without personal subject identifiers. In accord with the principals of the Declaration of Helsinki, subjects were asked to participate in a school class project and only told that they would be tested two times for about 15 minutes each time. Subjects with academic failure or accelerated academic performance or sleep problems were not included. The subjects agreeing to participate were grouped by sex and assigned to sleep or no sleep conditions with a separate randomization table for each group, to ensure a balanced design.
Subjects were tested in their homes in a quiet room without distractions for the duration of the learning and testing. The testing sessions were conducted during weekends or during school break. All subjects were given the paired-associate test, one of the standard tests of declarative memory [14] , which consisted of repeating semantically related and unrelated pairs of words (e.g. tree/leaf; lamp/shoe), in a standardized manner. After each word pair was presented out loud, the subject repeated the pair out loud to ensure registration of the paired associate. The list of the same 10 pairs was administered three times in immediate succession. Subjects assigned to the sleep condition learned the paired associates at 9∶00pm (±30 minutes), and were tested for cued recall twelve hours later, after a night of sleep. The no-sleep group received the same paired-associate presentation at 9∶00am (±30 minutes) and was tested for recall twelve hours later, with no intervening sleep or naps. The control working memory task, letter-number, was given just prior to learning the paired-associate words and again just prior to being tested on the paired-associate words. The letter-number test was administered to control for possible circadian confounds and to control for attention and encoding. The letter-number control task (LN, immediate recall and reordering of letters and numbers) is a subtest of the WAIS-III (Wechsler Adult Intelligence Scale) and WMS-III (Wechsler memory Scale), the most widely used intelligence and memory scales. An increasing long series of mixed letters and numbers is read to the subject and the subject then orders the numbers and letters in ascending order, e.g. b3a1 is read and subject correctly responds with 13ab. The letters and numbers must be encoded and then manipulated to get the correct answer. Two versions of the letter-number task were used in random order. The number correct was scored for the paired-associate and the letter-number tests. The memory scores were transformed into Z scores to determine if outliers were present; an exclusionary Z score of ±2.57 was applied (1% of the normal distribution). Between group comparisons were calculated by students t-test (2 tailed) after testing for equal variances by Levene’s test, and ANCOVA as necessary. Within subject comparisons were calculated by paired t-test.
Subjects were instructed to eat their usual meals approximately one hour before learning the paired-associates and one hour before being tested on the paired-associates. Subjects were instructed to get a good night’s sleep. All the subjects included reported having had typical night of sleep and rated the quality of the sleep as good to very good prior to the testing.
The sleep group’s mean age was 12.9 compared to 12.4 for the non-sleep group (t = (1.52), df (1,38), p = 0.14). (See Table 1 for demographic characteristics and performance scores). There was no statistically significant sex difference in performance for either task.
- PPT PowerPoint slide
- PNG larger image
- TIFF original image
https://doi.org/10.1371/journal.pone.0042191.t001
Three outliers were identified and removed; one high scoring subject assigned to the sleep and two lower scoring subjects assigned to no sleep. After removing outliers, 19 sleep subjects and 18 no- sleep subjects remained. The Levene’s Test showed equality of variances for all comparisons. The number correct on the letter-number control task at initial testing was 6.58 for the sleep group and 6.06 for the no-sleep group, (t = (1.54), df (1,35), p = 0.13). The letter-number correct score on the second administration was 6.26 and 6.33, respectively, (t = (-.16), df (1,35), p = 0.88), ( Figure 1 ). There was also no statistically significant difference in performance for either group on letter-number task between the first and second administration (paired t test, p = 0.32 for sleep group and 0.45 for no-sleep group).
A histogram of mean number correct (± SD) for the Paired-Associate Test (PA) and Letter Number Test (Letter #), with (n = 19) (outliers removed) and without sleep (n = 18).
https://doi.org/10.1371/journal.pone.0042191.g001
An increase of 20.6% in long-term memory ( Figure 1 ) was found as measured by the number correct in the paired-associate test following sleep, compared to the group which was tested at the same time interval, but without sleep (p<0.029). When the three outliers are included, the number correct for recall of the paired-associates was statistically greater for the sleep group (7.5) compared to the no sleep group (5.9, t = (2.76), df (1,37), p<0.009), a 32.7% increase.
The paired-associate test is one of the standard tests of declarative memory and has been previously used to study declarative memory and the effects of sleep on declarative memory in adults and children [3] . All subjects were evaluated at the same two times of day, approximately 9 AM and 9 PM, using standardized conditions. Performance on the paired-associate test was significantly affected by sleep in our adolescent sample. In contrast, working memory performance as measured by the letter-number test, a standard subtest of the WAIS-III and WMS-III was not affected by time of day or in relation to sleep. Correct performance on the letter-number working memory task (LN) requires that the letters and numbers presented to the subject must be encoded and then correctly manipulated. We had 80% power to detect a standardized difference of.76 correct (∼11% change) or greater, following sleep. A small difference in working memory performance (<11%), however, may exist that could not be detected with our sample size.
The equal performance at both sessions and between groups on the LN supports the view that equal registration and encoding of the memoranda was comparable at both time points and between groups. Performance on the working memory control task did not change with the second session for either group, suggesting that the time of day had no effect on performance on the working memory control task. Consequently, the observed difference in paired-associate performance, i.e. consolidation of working memory, is most likely related to sleep itself and not any differences in encoding. Memory consolidation has been reported to be affected by sleep [1] , [2] , [8] , [9] . Both REM and slow-wave sleep have been associated with improved memory [3] – [5] . Slow wave sleep particularly enhances declarative memory. 7 .
Our results are consistent with Gais et al.’s study of young males (mean age 17.4) showing that enhanced declarative memory was related to periods of sleep, and not to time of day effects [15] . Naps improve declarative memory regardless of time of nap [16] and closely resembled memory improvement after an eight-hour night of sleep [17] . In reviewing the timing of sleep and circadian rhythms, Diekelmann et al. conclude that sleep promotes memory consolidation independently of the time of day in which it occurs [3] . Voderholzer et al. studying 14–16 year old adolescents showed that several nights of sleep restriction did not impact memory consolidation nor performance in a working memory task, when two recovery night of sleep were provided, an effect they ascribed to a compensatory enhancement of SWS [18] . The paired-associate test begins as a working memory task and after a period of time with consolidation becomes a declarative memory task. Correct performance on the letter-number test and the paired-associate tests are dependent upon encoding the memoranda.
A limitation of this study is that we did not test for encoding strength by immediate recall after the administration of the paired-associate test. The letter-number test requires attention and encoding. An element of immediate recall is to prove that the subject was attending. This was assured by having the subjects read the words (similar to other learning tests like the CERAD and ADAS-COG) and supported by the finding of the performance on the letter-number test. It is likely that if immediate recall following each presentation was obtained, higher accuracy rates would have been observed. Recent studies have demonstrated that salience increases declarative memory performance [13] , [19] . Nevertheless, our data demonstrate that sleep improves memory consolidation even in conditions where encoding has not been reinforced.
Neither time of day or sleep affected the performance on the letter-number test suggesting that the material was being learned and encoded. There is no evidence that memory consolidation depends on time of day independent of sleep. The lack of interference during sleep has been considered as a possible cause of the beneficial effects of sleep on declarative memory, i.e. there are no daytime demands to interfere with memory consolidation. Our design tested subjects on non-school days, thus mitigating the effects of interference of memory consolidation during the day by learning competition and other demands of a normal school day. Gais et al. controlled for waking associated interference and found no effect of interference on memory [15] . In a review of controversy regarding whether absence of interference accounts for memory improvement during sleep, Ellenbogen at al. point out “although sleep might passively protect declarative memories from interference, consolidation must also occur during sleep for the memories to become resistant to interference the following day”. Based on their review of related animal and human studies, they point out that “hippocampus-dependent memories are reactivated during sleep, and that this reactivation leads to strengthened memory traces”, finally concluding “that specific, sleep-dependent, neurobiological processes directly lead to the consolidation of declarative memories” [1] . Diekelmann et al. hypothesized that both encoding and sleep-dependent consolidation during sleep involve prefrontal-hippocampal circuitry [3] .
Children have high amounts of slow wave sleep and sleep in general. Sleep has been shown to improve declarative and procedural memory in children and older age groups. Subjects were asked about their sleep and confirmed that they had a typical night sleep, consisting of 8–10 hours of sleep, average for adolescents [20] . We did not, however, specifically measure sleep. Lack of sleep can result in poor cognitive performance, which was not observed in our sample, and is consistent with the subjects’ report of a good night sleep and that poor sleepers were excluded from the sample.
A cross-over design would have provided additional confirmation at the individual subject level in contrast to our parallel group design. Our study was limited as the sample was opportune, from a California middle school, and was not epidemiologically based. No subjects approached declined to participate. No accelerated or failing students were included, although this was not a strict exclusion criterion. There were 3% African-American, 5% Asian, and 92% Caucasian. The sample population reflected the general school population in this geographic area, although Asians were underrepresented (12.8%).
Our sample size was relatively small and limited to early adolescence, ages 10–14, although twice the sample of Prehn-Kristensen et al. who found 10 to 13 year olds improved visual memory following sleep, especially to emotional pictures [13] . The 10–14 age group was deliberately chosen because of the importance of declarative memory on adolescent school performance and related social functioning [21] . Marked changes in sleep and sleep architecture are a defining feature of adolescence [22] . Disorders of adolescence frequently disrupt sleep. Twenty-five to forty percent of adolescents have sleep disorders that can have an important effect on daytime school and consequent social functioning [23] . Sleep disorders are even more prevalent in adolescents with psychiatric disorders and developmental disabilities [24] . It is important to have data on the effects of normal sleep on declarative memory in normal adolescents to better understand the consequences of lack of sleep and abnormal sleep patterns.
Given the importance of adolescent memory on school performance and consequent social functioning, a fuller understanding of the effects of sleep on memory consolidation is needed. Other studies are needed to investigate the specific effects of sleep on other types of memory, such as visual, procedural, and emotional. Understanding the role of normal sleep on memory consolidation in adolescence is critical in identifying the consequences of disrupted sleep in adolescent disorders and their treatment.
Author Contributions
Conceived and designed the experiments: KTP WEB. Performed the experiments: KTP. Analyzed the data: KTP WEB. Contributed reagents/materials/analysis tools: KTP WEB. Wrote the paper: KTP WEB.
- View Article
- Google Scholar

The Impact of Sleep on Learning and Memory
By Kelly Cappello, B.A.
For many students, staying awake all night to study is common practice. According to Medical News Today , around 20 percent of students pull all-nighters at least once a month, and about 35 percent stay up past three in the morning once or more weekly.
That being said, staying up all night to study is one of the worst things students can do for their grades. In October of 2019, two MIT professors found a correlation between sleep and test scores : The less students slept during the semester, the worse their scores.
So, why is it that sleep is so important for test scores? While the answer seems simple, that students simply perform better when they’re not mentally or physically tired, the truth may be far more complicated and interesting.
In the last 20 years, scientists have found that sleep impacts more than just students’ ability to perform well; it improves their ability to learn, memorize, retain, recall, and use their new knowledge to solve problems creatively. All of which contribute to better test scores.
Let’s take a look at some of the most interesting research regarding the impact of sleep on learning and memory.
How does sleep improve the ability to learn?
When learning facts and information, most of what we learn is temporarily stored in a region of the brain called the hippocampus. Some scientists hypothesize that , like most storage centers, the hippocampus has limited storage capacity. This means, if the hippocampus is full, and we try to learn more information, we won’t be able to.
Fortunately, many scientists also hypothesize that sleep, particularly Stages 2 and 3 sleep, plays a role in replenishing our ability to learn. In one study, a group of 44 participants underwent two rigorous sessions of learning, once at noon and again at 6:00 PM. Half of the group was allowed to nap between sessions, while the other half took part in standard activities. The researchers found that the group that napped between learning sessions learned just as easily at 6:00 PM as they did at noon. The group that didn’t nap, however, experienced a significant decrease in learning ability [1].
How does sleep improve the ability to recall information?
Humans have known about the benefits of sleep for memory recall for thousands of years. In fact, the first record of this revelation is from the first century AD. Rhetorician Quintilian stated, “It is a curious fact, of which the reason is not obvious, that the interval of a single night will greatly increase the strength of the memory.”
In the last century, scientists have tested this theory many times, often finding that sleep improves memory retention and recall by between 20 and 40 percent. Recent research has led scientists to hypothesize that Stage 3 (deep non-Rapid Eye Movement sleep, or Slow Wave Sleep) may be especially important for the improvement of memory retention and recall [2].
How does sleep improve long-term memory?
Scientists hypothesize that sleep also plays a major role in forming long-term memories. According to Matthew Walker, professor of neuroscience and psychology at UC Berkeley, MRI scans indicate that the slow brain waves of stage 3 sleep (deep NREM sleep) “serve as a courier service,” transporting memories from the hippocampus to other more permanent storage sites [3].
How does sleep improve the ability to solve problems creatively?
Many tests are designed to assess critical thinking and creative problem-solving skills. Recent research has led scientists to hypothesize that sleep, particularly REM sleep, plays a role in strengthening these skills. In one study, scientists tested the effect of REM sleep on the ability to solve anagram puzzles (word scrambles like “EOUSM” for “MOUSE”), an ability that requires strong creative thinking and problem-solving skills.
In the study, participants solved a couple of anagram puzzles before going to sleep in a sleep laboratory with electrodes placed on their heads. The subjects were woken up four times during the night to solve anagram puzzles, twice during NREM sleep and twice during REM sleep.
The researchers found that when participants were woken up during REM sleep, they could solve 15 to 35 percent more puzzles than they could when woken up from NREM sleep. They also performed 15 to 35 percent better than they did in the middle of the day [4]. It seems that REM sleep may play a major role in improving the ability to solve complex problems.
So, what’s the point?
Sleep research from the last 20 years indicates that sleep does more than simply give students the energy they need to study and perform well on tests. Sleep actually helps students learn, memorize, retain, recall, and use their new knowledge to come up with creative and innovative solutions.
It’s no surprise that the MIT study previously mentioned revealed no improvement in scores for those who only prioritized their sleep the night before a big test. In fact, the MIT researchers concluded that if students want to see an improvement in their test scores, they have to prioritize their sleep during the entire learning process. Staying up late to study just doesn’t pay off.
Interested in learning more about the impact of sleep on learning and memory? Check out this Student Sleep Guide .
Author Biography
Kelly Cappello graduated from East Stroudsburg University of Pennsylvania with a B.A. in Interdisciplinary Studies in 2015. She is now a writer, specialized in researching complex topics and writing about them in simple English. She currently writes for Recharge.Energy , a company dedicated to helping the public improve their sleep and improve their lives.
- Mander, Bryce A., et al. “Wake Deterioration and Sleep Restoration of Human Learning.” Current Biology, vol. 21, no. 5, 2011, doi:10.1016/j.cub.2011.01.019.
- Walker M. P. (2009). The role of slow wave sleep in memory processing. Journal of clinical sleep medicine : JCSM : official publication of the American Academy of Sleep Medicine, 5(2 Suppl), S20–S26.
- Walker, Matthew. Why We Sleep. Scribner, 2017.
- Walker, Matthew P, et al. “Cognitive Flexibility across the Sleep–Wake Cycle: REM-Sleep Enhancement of Anagram Problem Solving.” Cognitive Brain Research, vol. 14, no. 3, 2002, pp. 317–324., doi:10.1016/s0926-6410(02)00134-9.
Posted on Dec 21, 2020 | Tagged: learning and memory
© The Trustees of the University of Pennsylvania | Site best viewed in a supported browser . | Report Accessibility Issues and Get Help | Privacy Policy | Site Design: PMACS Web Team. | Sitemap
Save 45% on a Home Sleep Test – Shop Now
How Lack of Sleep Impacts Cognitive Performance and Focus
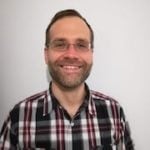
Staff Writer
Eric Suni has over a decade of experience as a science writer and was previously an information specialist for the National Cancer Institute.
Want to read more about all our experts in the field?
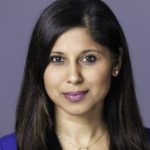
Dr. Nilong Vyas
Pediatrician
Dr. Vyas is a pediatrician and founder of Sleepless in NOLA. She specializes in helping parents establish healthy sleep habits for children.
Sleep Foundation
Fact-Checking: Our Process
The Sleep Foundation editorial team is dedicated to providing content that meets the highest standards for accuracy and objectivity. Our editors and medical experts rigorously evaluate every article and guide to ensure the information is factual, up-to-date, and free of bias.
The Sleep Foundation fact-checking guidelines are as follows:
- We only cite reputable sources when researching our guides and articles. These include peer-reviewed journals, government reports, academic and medical associations, and interviews with credentialed medical experts and practitioners.
- All scientific data and information must be backed up by at least one reputable source. Each guide and article includes a comprehensive bibliography with full citations and links to the original sources.
- Some guides and articles feature links to other relevant Sleep Foundation pages. These internal links are intended to improve ease of navigation across the site, and are never used as original sources for scientific data or information.
- A member of our medical expert team provides a final review of the content and sources cited for every guide, article, and product review concerning medical- and health-related topics. Inaccurate or unverifiable information will be removed prior to publication.
- Plagiarism is never tolerated. Writers and editors caught stealing content or improperly citing sources are immediately terminated, and we will work to rectify the situation with the original publisher(s)
- Although Sleep Foundation maintains affiliate partnerships with brands and e-commerce portals, these relationships never have any bearing on our product reviews or recommendations. Read our full Advertising Disclosure for more information.
Table of Contents
How Poor Sleep Affects the Brain
How does poor sleep affect creativity and other cognitive processes, are the cognitive impacts of poor sleep the same for everyone, can sleep disorders affect cognition, does too much sleep affect cognition, will improving sleep quality benefit cognition.
Getting enough hours of high-quality sleep fosters attention and concentration, which are prerequisites for most learning. Sleep also supports numerous other aspects of cognition, including memory, problem-solving, creativity, emotional processing, and judgment. Levels of brain activity fluctuate during each stage of sleep — including both rapid eye movement (REM) and non-REM (NREM) sleep — and evidence increasingly suggests that sleep enhances most types of cognitive function.
For people with sleep deprivation, insomnia, sleep apnea, or other conditions that prevent them from getting adequate rest, short-term daytime cognitive impairment is common. Improving sleep quality can boost cognitive performance, promote sharper thinking, and may reduce the likelihood of age-related cognitive decline.
During a typical night of sleep, an individual cycles through the three stages of NREM sleep, followed by a period of REM sleep every 90 to 120 minutes, several times per night. Both the brain and body experience distinct changes during these cycles that correspond to individual stages of sleep . During each part of this process, different chemicals in the brain become activated or deactivated to coordinate rest and recovery.
Poor sleep can take many forms, including short sleep duration or fragmented sleep. Without adequate sleep, the brain struggles to function properly. Because they do not have time to recuperate, neurons in the brain become overworked and less capable of optimal performance in various types of thinking.
The short-term detriments of poor sleep on the brain and cognition can be the result of pulling an occasional all-nighter, while those with chronic sleep problems may see a continuous negative effect on day-to-day tasks. Over the long-term, poor sleep may put someone at a higher risk of cognitive decline and dementia.
What Are the Short-Term Cognitive Impacts of Poor Sleep?
Poor sleep can harm intellectual performance, academic achievement, creative pursuits, and productivity at work. The cognitive impacts of poor sleep can also create safety risks, including drowsy driving . Motor skills, keeping rhythm, and even some types of speech can decline without proper sleep. The potential short-term impacts of poor sleep are wide-ranging:
- Excessive Sleepiness: Drowsiness and fatigue are common daytime effects of a night of poor sleep. In response to excessive fatigue, a person may inadvertently nod off for a few seconds, which is known as a microsleep .
- Poor Attention Span: Poor sleep reduces a person’s attention, as well as their learning and processing. A lack of sleep has also been found to induce effects that are similar to being drunk Trusted Source National Library of Medicine, Biotech Information The National Center for Biotechnology Information advances science and health by providing access to biomedical and genomic information. View Source , which slows down thinking and reaction time . Poor sleep also diminishes placekeeping Trusted Source National Library of Medicine, Biotech Information The National Center for Biotechnology Information advances science and health by providing access to biomedical and genomic information. View Source , which includes the ability to carry out instructions.
- Reduced Adaptability: Some studies have found lack of sleep to hinder cognitive flexibility, reducing the ability to adapt and thrive in uncertain or changing circumstances. A major reason this occurs is rigid thinking and “feedback blunting” Trusted Source Oxford Academic Journals (OUP) OUP publishes the highest quality journals and delivers this research to the widest possible audience. View Source , in which the capacity to learn and improve on-the-fly is diminished.
- Reduced Emotional Capacity: Poor sleep can also alter how emotional information is understood Trusted Source National Library of Medicine, Biotech Information The National Center for Biotechnology Information advances science and health by providing access to biomedical and genomic information. View Source . When learning something new, analyzing a problem, or making a decision, recognizing the emotional context is often important. However, insufficient sleep impedes the ability to properly process the emotional component of information.
- Impaired Judgment: In some cases, this dysregulated emotional response impairs judgment. People who do not get sufficient sleep are more likely to make risky choices Trusted Source National Library of Medicine, Biotech Information The National Center for Biotechnology Information advances science and health by providing access to biomedical and genomic information. View Source and may focus on a potential reward rather than downsides. It can be difficult to learn from these mistakes, since the normal method of processing and consolidating emotional memory is compromised due to lack of sleep.
What Are the Long-Term Cognitive Impacts of Poor Sleep?
Insufficient sleep and sleep fragmentation are frequently associated with cognitive decline and dementia. Furthermore, in people already diagnosed with dementia, poor sleep has been linked to a worse disease prognosis. Some cognitive effects of poor sleep can be felt immediately, but mounting evidence shows that sleep influences your long-term risk of cognition issues:
- Impaired Memory: Both NREM and REM sleep appear to be important for broader memory consolidation Trusted Source National Library of Medicine, Biotech Information The National Center for Biotechnology Information advances science and health by providing access to biomedical and genomic information. View Source , which helps reinforce information in the brain so that it can be recalled when needed. NREM sleep has been linked with declarative memory, which includes things like basic facts or statistics, and REM sleep is believed to boost procedural memory such as remembering a sequence of steps. Poor sleep impairs memory consolidation by disrupting the normal process that draws on both NREM and REM sleep for building and retaining memories. Studies have even found that people who are sleep deprived are at risk of forming false memories Trusted Source National Library of Medicine, Biotech Information The National Center for Biotechnology Information advances science and health by providing access to biomedical and genomic information. View Source .
- Alzheimer’s Disease: Research shows that sleep helps the brain conduct important housekeeping, such as clearing out potentially dangerous beta amyloid proteins. In Alzheimer’s disease, beta amyloid forms in clusters, called plaques, that worsen cognitive function. Studies have found that even one night of sleep deprivation can increase the amount of beta amyloid in the brain Trusted Source National Library of Medicine, Biotech Information The National Center for Biotechnology Information advances science and health by providing access to biomedical and genomic information. View Source . One analysis found a considerably higher risk of Alzheimer’s disease Trusted Source National Library of Medicine, Biotech Information The National Center for Biotechnology Information advances science and health by providing access to biomedical and genomic information. View Source in people with sleep problems, estimating as many as 15% of cases of Alzheimer’s disease were attributable to poor sleep.
Creativity is another aspect of cognition that is hindered by sleeping problems. Connecting loosely associated ideas is a hallmark of creativity, and this ability is strengthened by good sleep. NREM sleep provides an opportunity for information to be restructured and reorganized Trusted Source National Library of Medicine, Biotech Information The National Center for Biotechnology Information advances science and health by providing access to biomedical and genomic information. View Source in the brain, while new ideas and links between thoughts often emerge during REM sleep. These processes enable insight, a core element of innovation and creative problem-solving.
Limited or restless sleep can also indirectly affect cognition. For example, migraine sufferers are more likely to have morning headache attacks Trusted Source National Library of Medicine, Biotech Information The National Center for Biotechnology Information advances science and health by providing access to biomedical and genomic information. View Source when they do not get enough sleep, and lack of sleep can increase the risk of infections Trusted Source National Library of Medicine, Biotech Information The National Center for Biotechnology Information advances science and health by providing access to biomedical and genomic information. View Source like the common cold. Sleep deprivation may worsen symptoms of mental health conditions like anxiety and depression. These and numerous other physical and mental health issues are shaped by sleep quality, and may affect a person’s attention and concentration.
Not everyone is affected by poor sleep in the same way. Studies have found that some individuals may be more susceptible to cognitive impairment from sleep deprivation, and this may be influenced by genetics.
Research has discovered that adults are better at overcoming the effects of sleep deprivation than younger people. Teens are considered to be at a heightened risk for detrimental effects of poor sleep on thinking, decision-making, and academic performance because of the ongoing brain development that occurs during teen years .
Some studies have also found that women are more adept at coping with the effects of sleep deprivation than men, although it is not yet clear if this is related to biological factors, social and cultural influences, or a combination of both.
Sleep disorders, like insomnia, frequently involve insufficient or fragmented sleep, so it comes as little surprise that they can be linked to cognitive impairment.
Obstructive sleep apnea (OSA) is among the most common sleep disorders. It occurs when the airway gets blocked, which then leads to lapses in breathing during sleep and reduced oxygen in the blood. OSA has been associated with daytime sleepiness as well as notable cognitive problems related to attention, thinking, memory, and communication. Studies have also found that people with sleep apnea have a higher risk of developing dementia .
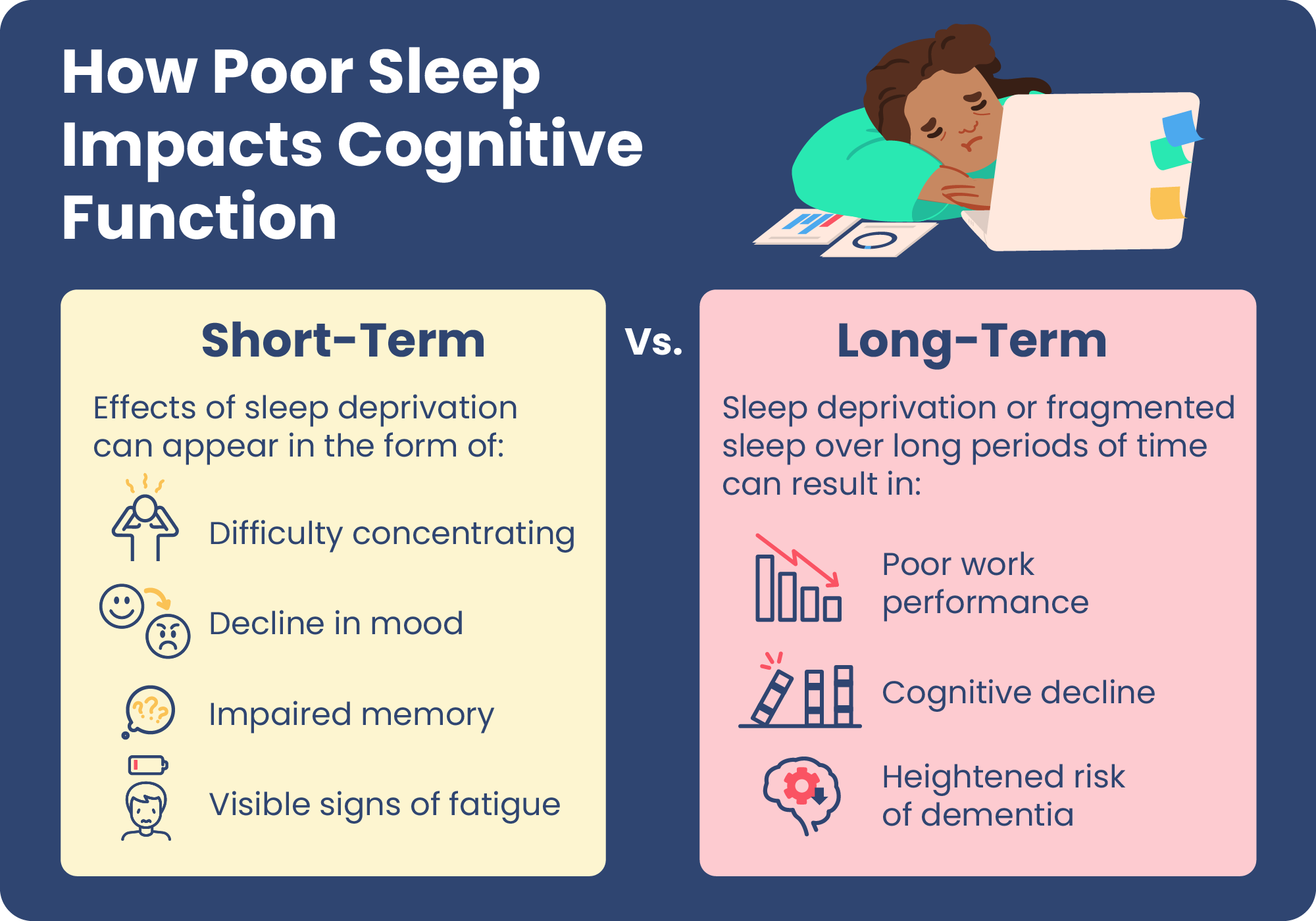
Many studies examining the effects of sleep on thinking have found that an excess of sleep can also be problematic for brain health. In many cases, research has discovered that both too little and too much sleep Trusted Source National Library of Medicine, Biotech Information The National Center for Biotechnology Information advances science and health by providing access to biomedical and genomic information. View Source are associated with cognitive decline.
The explanation for this association remains unclear. It is not known if excess sleep is caused by a coexisting health condition that may also predispose someone to cognitive problems. Overall, these research findings are an important reminder to get the right amount of sleep each night.
For people with sleeping problems, improving sleep quality offers a practical way to enhance cognitive performance. Getting the recommended amount of uninterrupted sleep can help the brain recuperate and avoid many of the negative consequences of poor sleep on diverse aspects of thinking.
Researchers and public health experts increasingly view good sleep as a potential form of prevention against dementia and Alzheimer’s disease Trusted Source National Library of Medicine, Biotech Information The National Center for Biotechnology Information advances science and health by providing access to biomedical and genomic information. View Source . Although more studies are needed to conclusively determine sleep’s role in preventing cognitive decline, early research suggests that taking steps to improve sleep may reduce the longer-term likelihood of developing Alzheimer’s disease.
Tips To Improve Sleep and Cognitive Performance
Anyone who feels that they are experiencing cognitive impairment or excessive daytime sleepiness should first speak with their doctor. A physician can help identify or rule out any other conditions, including sleep disorders, that may be causing these symptoms. They can also discuss strategies to get better sleep.
Many approaches to improving sleep start with healthy sleep hygiene . By optimizing your bedroom environment and everyday habits and routines, you can eliminate many common barriers to sleep. Setting a regular bedtime and sleep schedule, avoiding alcohol and caffeine in the evening, and minimizing electronics in the bedroom are a few examples of sleep hygiene tips that can make it easier to rest well each night.
About Our Editorial Team
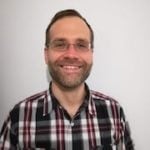
Eric Suni, Staff Writer
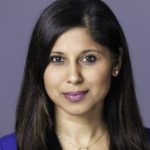
Medically Reviewed by
Dr. Nilong Vyas, Pediatrician MD
References 14 sources.
Dawson, D., & Reid, K. (1997). Fatigue, alcohol and performance impairment. Nature, 388(6639), 235.
Stepan, M. E., Altmann, E. M., & Fenn, K. M. (2020). Effects of total sleep deprivation on procedural placekeeping: More than just lapses of attention. Journal of experimental psychology. General, 149(4), 800–806.
Whitney, P., Hinson, J. M., Jackson, M. L., & Van Dongen, H. P. (2015). Feedback Blunting: Total Sleep Deprivation Impairs Decision Making that Requires Updating Based on Feedback. Sleep, 38(5), 745–754.
Killgore, W. D. (2010). Effects of sleep deprivation on cognition. Progress in Brain Research, 185, 105–129.
Van Someren, E. J., Cirelli, C., Dijk, D. J., Van Cauter, E., Schwartz, S., & Chee, M. W. (2015). Disrupted sleep: From molecules to cognition. The Journal of Neuroscience, 35(41), 13889–13895.
Maquet P. (2000). Sleep on it!. Nature neuroscience, 3(12), 1235–1236.
Lo, J. C., Chong, P. L., Ganesan, S., Leong, R. L., & Chee, M. W. (2016). Sleep deprivation increases formation of false memory. Journal of sleep research, 25(6), 673–682.
Shokri-Kojori, E., Wang, G. J., Wiers, C. E., Demiral, S. B., Guo, M., Kim, S. W., Lindgren, E., Ramirez, V., Zehra, A., Freeman, C., Miller, G., Manza, P., Srivastava, T., De Santi, S., Tomasi, D., Benveniste, H., & Volkow, N. D. (2018). β-Amyloid accumulation in the human brain after one night of sleep deprivation. Proceedings of the National Academy of Sciences of the United States of America, 115(17), 4483–4488.
Bubu, O. M., Brannick, M., Mortimer, J., Umasabor-Bubu, O., Sebastião, Y. V., Wen, Y., Schwartz, S., Borenstein, A. R., Wu, Y., Morgan, D., & Anderson, W. M. (2017). Sleep, Cognitive impairment, and Alzheimer’s disease: A Systematic Review and Meta-Analysis. Sleep, 40(1), 10.1093/sleep/zsw032.
Yordanova, J., Kolev, V., Wagner, U., & Verleger, R. (2010). Differential associations of early- and late-night sleep with functional brain states promoting insight to abstract task regularity. PloS one, 5(2), e9442.
Lin, Y. K., Lin, G. Y., Lee, J. T., Lee, M. S., Tsai, C. K., Hsu, Y. W., Lin, Y. Z., Tsai, Y. C., & Yang, F. C. (2016). Associations Between Sleep Quality and Migraine Frequency: A Cross-Sectional Case-Control Study. Medicine, 95(17), e3554.
Prather, A. A., Janicki-Deverts, D., Hall, M. H., & Cohen, S. (2015). Behaviorally Assessed Sleep and Susceptibility to the Common Cold. Sleep, 38(9), 1353–1359.
Ma, Y., Liang, L., Zheng, F., Shi, L., Zhong, B., & Xie, W. (2020). Association Between Sleep Duration and Cognitive Decline. JAMA network open, 3(9), e2013573.
Spira, A. P., Chen-Edinboro, L. P., Wu, M. N., & Yaffe, K. (2014). Impact of sleep on the risk of cognitive decline and dementia. Current Opinion in Psychiatry, 27(6):478-83.
Learn More About Sleep Deprivation
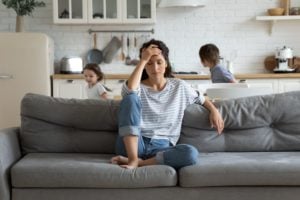
Can a Lack of Sleep Cause Headaches?
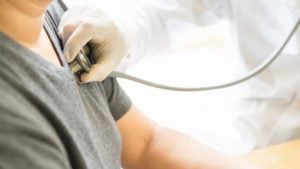
How Sleep Deprivation Affects Your Heart
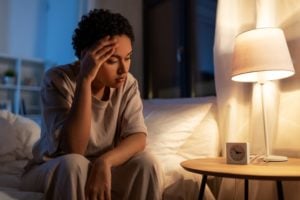
Interrupted Sleep: Causes & Helpful Tips
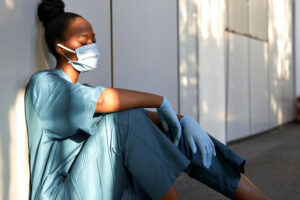
Sleep Deprivation: Symptoms, Treatment, & Effects
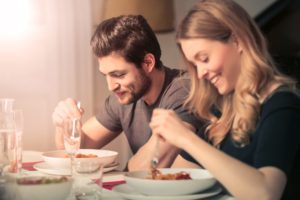
Lack of Sleep May Increase Calorie Consumption
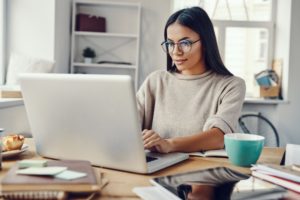
Sleepless Nights: How to Function on No Sleep
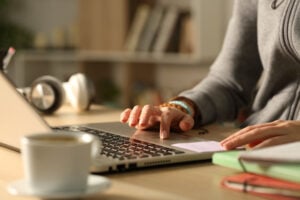
What All-Nighters Do To Your Cognition
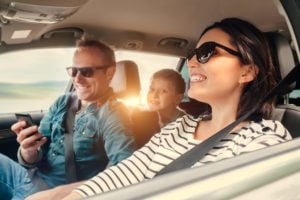
Sleep Deprivation and Reaction Time
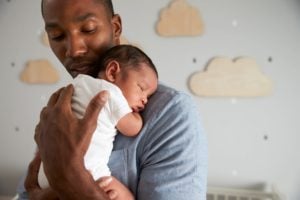
Understanding Sleep Deprivation and New Parenthood
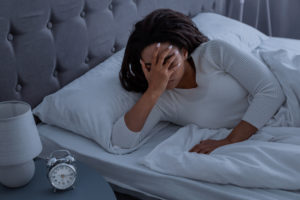
Effects of Sleep Deprivation
Other articles of interest, sleep solutions, sleep hygiene, sleep apnea.
Information
- Author Services
Initiatives
You are accessing a machine-readable page. In order to be human-readable, please install an RSS reader.
All articles published by MDPI are made immediately available worldwide under an open access license. No special permission is required to reuse all or part of the article published by MDPI, including figures and tables. For articles published under an open access Creative Common CC BY license, any part of the article may be reused without permission provided that the original article is clearly cited. For more information, please refer to https://www.mdpi.com/openaccess .
Feature papers represent the most advanced research with significant potential for high impact in the field. A Feature Paper should be a substantial original Article that involves several techniques or approaches, provides an outlook for future research directions and describes possible research applications.
Feature papers are submitted upon individual invitation or recommendation by the scientific editors and must receive positive feedback from the reviewers.
Editor’s Choice articles are based on recommendations by the scientific editors of MDPI journals from around the world. Editors select a small number of articles recently published in the journal that they believe will be particularly interesting to readers, or important in the respective research area. The aim is to provide a snapshot of some of the most exciting work published in the various research areas of the journal.
Original Submission Date Received: .
- Active Journals
- Find a Journal
- Proceedings Series
- For Authors
- For Reviewers
- For Editors
- For Librarians
- For Publishers
- For Societies
- For Conference Organizers
- Open Access Policy
- Institutional Open Access Program
- Special Issues Guidelines
- Editorial Process
- Research and Publication Ethics
- Article Processing Charges
- Testimonials
- Preprints.org
- SciProfiles
- Encyclopedia
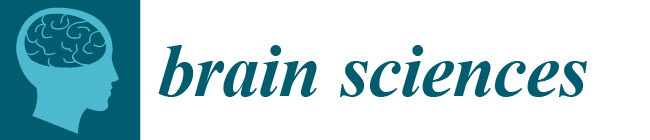
Article Menu
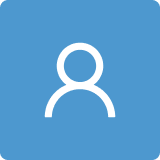
- Subscribe SciFeed
- Recommended Articles
- Author Biographies
- Google Scholar
- on Google Scholar
- Table of Contents
Find support for a specific problem in the support section of our website.
Please let us know what you think of our products and services.
Visit our dedicated information section to learn more about MDPI.
JSmol Viewer
The complex relationship between sleep and cognitive reserve: a narrative review based on human studies.
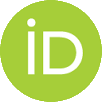
1. Introduction
2. literature search strategy.
- The first section discusses findings related to sleep as a factor that enhances CR;
- The second section discusses findings regarding CR as a moderator between sleep and cognitive functions.
3.1. Sleep as Factor Involved in Cognitive Reserve
3.1.1. healthy population, 3.1.2. population with alzheimer’s disease.
References | Sleep Component/Disorder | Sleep Measure | Cognitive Function | Cognitive Function Measure | Reserve Measure | Results |
---|---|---|---|---|---|---|
Zijlmans et al. (2023) [ ] | 24 h activity rhythms (sleep onset latency and sleep efficiency) | 7-day actigraphy; Sleep diary; PSQI. | - | - | Cognitive battery assessment: Verbal memory (15-word verbal learning test) Attention and interference of automatic processes (Stroop test) Long-term memory (word fluency test) Processing speed (letter–digit substitution task) Fine motor skills (Purdue pegboard test) Education level Brain-MRI Education | ↑ |
Zavecz et al. (2023) [ ] | NREM SWS AD-related | PSG | Memory | Hippocampal-dependent face–name learning task | Brain-MRI Education Physical activity | ↑ |
3.2. Cognitive Reserve as a Moderator in the Relationship between Sleep and Cognitive Functions
3.2.1. healthy population, 3.2.2. population with sleep disorders, 3.2.3. population with parkinson’s disease and sleep disorders.
Reference | Sleep Component/Disorder | Sleep Measure | Cognitive Function | Cognitive Function Measure | Reserve Measure | Results |
---|---|---|---|---|---|---|
Zimmerman et al. (2012) [ ] | SO/MD difficulties | MOS-SS. | Verbal memory | Free and Cued Selective Reminding Test | Education | ↑ language fluency |
Executive functions | Trail Making Test-Part B, Category Fluency; Letter Fluency Tests | |||||
Attention | Digit Span Subtest from WAIS-III; Trail Making Test-Part A | |||||
Parker et al. (2020) [ ] | Sleep quality (frequency and duration of awakenings) | Actigraphy; Consensus; Sleep Diary. | Inhibition | Anti-Saccades and Flanker tests; | Education | ↑ Executive functions |
Shifting | NIH Examiner Set-Shifting Subtest; Trail Making Test-Part B; Verbal Fluency Category Switching Subtest from the Delis–Kaplan Executive Function System; | |||||
Working memory | Digit Span Backwards from WAIS-III; | |||||
Dual Task Subtest of the Test of Everyday Attention | ||||||
Generativity | Controlled Oral Word Association Test; the Action Fluency Task | |||||
Yeh et al. (2021) [ ] | Sleep–wake disturbances | 7-day actigraphy; PSQI; ESS questionnaire. | Episodic memory | Hopkins Verbal Learning Test-Revised | Wide Range Achievement Test 4-Reading subtest | |
Ourry et al. (2023) [ ] | SWS | PSG | Executive functions | Digit Span Backward; Trail Making Test-Part B; Stroop Interference Test; Letter Fluency; | CAQ Questionnaire; LEQ Questionnaire. | |
Memory | The California Verbal Learning Test; Wechsler Memory Scale IV Logical Memory, Story B (WMS IV) | ↑ Memory | ||||
Alchanatis et al. (2005) [ ] | OSA | PSG; ESS questionnaire. | Attention/ alertness | Vienna Test System | RPM IQ | ↑ Attention/Alertness |
Olaithe et al. (2020) [ ] | OSA | PSG; ApneaLink; ESS questionnaire. | Attention Short-term memory Episodic long-term memory | Cognitive Drug Research System | National Adult Reading Test | ↑ Attention (only in the clinical sample) ↑ Memory (only in the clinic sample) |
Hlaing et al. (2021) [ ] | OSA | PSG; ESS questionnaire; PSQI; Morningness–Eveningness Questionnaire. | Language | Semantic and Phonemic Fluency Tests; | Education | ↑ Language |
Attention | Psychomotor Vigilance Task; | ↑ Attention (regardless of OSA) | ||||
Visuospatial abilities | WAIS-III Block Design; | ↑ Visuospatial abilities | ||||
Executive functions | Wisconsin Card Sorting Test, WAIS-III digit span | ↑ Executive functions (regardless of OSA) | ||||
D’Este et al. (2023) [ ] | iRBD | PSG. | General cognitive function | Mini Mental State Examination | CRIq | |
Language | Token Test; Semantic and Phonemic Verbal Fluency Tests; CAGI Oral Denomination Test; | |||||
Memory | Digit-Span Test; Corsi Block-Tapping Test; Rey Auditory Verbal Learning Test; Recall of the Rey Osterrieth Complex Figure; | ↑ Verbal memory functions | ||||
Executive functions | RPM Task; Attentive Matrices Test | |||||
Visuospatial abilities | Copy Rey Osterrieth Complex Figure | ↑ Visuo-constructive functions | ||||
Prete et al. (2023) [ ] | Sleep difficulties PD-related | RBD Screening Questionnaire; PSQI; ESS questionnaire. | General cognitive functions | Telephone-Global Examination of Mental State | CRIq | ↑ Cognitive functions |
4. Discussion
Click here to enlarge figure
5. Conclusions
Author contributions, institutional review board statement, informed consent statement, data availability statement, conflicts of interest.
- Mandolesi, L.; Gelfo, F.; Serra, L.; Montuori, S.; Polverino, A.; Curcio, G.; Sorrentino, G. Environmental Factors Promoting Neural Plasticity: Insights from Animal and Human Studies. Neural Plast. 2017 , 2017 , 7219461. [ Google Scholar ] [ CrossRef ] [ PubMed ]
- Sale, A.; Berardi, N.; Maffei, L. Environment and Brain Plasticity: Towards an Endogenous Pharmacotherapy. Physiol. Rev. 2014 , 94 , 189–234. [ Google Scholar ] [ CrossRef ] [ PubMed ]
- Berlucchi, G.; Buchtel, H.A. Neuronal Plasticity: Historical Roots and Evolution of Meaning. Exp. Brain Res. 2009 , 192 , 307–319. [ Google Scholar ] [ CrossRef ]
- Stern, Y. What Is Cognitive Reserve? Theory and Research Application of the Reserve Concept. J. Int. Neuropsychol. Soc. 2002 , 8 , 448–460. [ Google Scholar ] [ CrossRef ] [ PubMed ]
- Stern, Y.; Albert, S.; Tang, M.-X.; Tsai, W.-Y. Rate of Memory Decline in AD Is Related to Education and Occupation. Neurology 1999 , 53 , 1942. [ Google Scholar ] [ CrossRef ]
- Gelfo, F.; Mandolesi, L.; Serra, L.; Sorrentino, G.; Caltagirone, C. The Neuroprotective Effects of Experience on Cognitive Functions: Evidence from Animal Studies on the Neurobiological Bases of Brain Reserve. Neuroscience 2018 , 370 , 218–235. [ Google Scholar ] [ CrossRef ]
- Stern, Y.; Barnes, C.A.; Grady, C.; Jones, R.N.; Raz, N. Brain Reserve, Cognitive Reserve, Compensation, and Maintenance: Operationalization, Validity, and Mechanisms of Cognitive Resilience. Neurobiol. Aging 2019 , 83 , 124–129. [ Google Scholar ] [ CrossRef ]
- Stern, Y. Cognitive Reserve in Ageing and Alzheimer’s Disease. Lancet Neurol. 2012 , 11 , 1006–1012. [ Google Scholar ] [ CrossRef ] [ PubMed ]
- Cutuli, D.; Landolfo, E.; Petrosini, L.; Gelfo, F. Environmental Enrichment Effects on the Brain-Derived Neurotrophic Factor Expression in Healthy Condition, Alzheimer’s Disease, and Other Neurodegenerative Disorders. J. Alzheimers Dis. 2022 , 85 , 975–992. [ Google Scholar ] [ CrossRef ]
- Landolfo, E.; Cutuli, D.; Decandia, D.; Balsamo, F.; Petrosini, L.; Gelfo, F. Environmental Enrichment Protects against Neurotoxic Effects of Lipopolysaccharide: A Comprehensive Overview. IJMS 2023 , 24 , 5404. [ Google Scholar ] [ CrossRef ]
- Steffener, J.; Stern, Y. Exploring the Neural Basis of Cognitive Reserve in Aging. Biochim. Biophys. Acta 2012 , 1822 , 467–473. [ Google Scholar ] [ CrossRef ] [ PubMed ]
- Stern, Y.; Arenaza-Urquijo, E.M.; Bartrés-Faz, D.; Belleville, S.; Cantilon, M.; Chetelat, G.; Ewers, M.; Franzmeier, N.; Kempermann, G.; Kremen, W.S.; et al. Whitepaper: Defining and Investigating Cognitive Reserve, Brain Reserve, and Brain Maintenance. Alzheimers Dement. 2020 , 16 , 1305–1311. [ Google Scholar ] [ CrossRef ] [ PubMed ]
- Serra, L.; Gelfo, F. What Good Is the Reserve? A Translational Perspective for the Managing of Cognitive Decline. Neural Regen. Res. 2019 , 14 , 1219–1220. [ Google Scholar ] [ CrossRef ] [ PubMed ]
- Serra, L.; Gelfo, F.; Petrosini, L.; Di Domenico, C.; Bozzali, M.; Caltagirone, C. Rethinking the Reserve with a Translational Approach: Novel Ideas on the Construct and the Interventions. J. Alzheimers Dis. 2018 , 65 , 1065–1078. [ Google Scholar ] [ CrossRef ]
- Song, S.; Stern, Y.; Gu, Y. Modifiable Lifestyle Factors and Cognitive Reserve: A Systematic Review of Current Evidence. Ageing Res. Rev. 2022 , 74 , 101551. [ Google Scholar ] [ CrossRef ]
- Decandia, D.; Gelfo, F.; Landolfo, E.; Balsamo, F.; Petrosini, L.; Cutuli, D. Dietary Protection against Cognitive Impairment, Neuroinflammation and Oxidative Stress in Alzheimer’s Disease Animal Models of Lipopolysaccharide-Induced Inflammation. Int. J. Mol. Sci. 2023 , 24 , 5921. [ Google Scholar ] [ CrossRef ]
- Gelfo, F.; Petrosini, L.; Mandolesi, L.; Landolfo, E.; Caruso, G.; Balsamo, F.; Bonarota, S.; Bozzali, M.; Caltagirone, C.; Serra, L. Land/Water Aerobic Activities: Two Sides of the Same Coin. A Comparative Analysis on the Effects in Cognition of Alzheimer’s Disease. J. Alzheimers Dis. 2024 , 98 , 1181–1197. [ Google Scholar ] [ CrossRef ]
- Petrosini, L.; De Bartolo, P.; Foti, F.; Gelfo, F.; Cutuli, D.; Leggio, M.G.; Mandolesi, L. On Whether the Environmental Enrichment May Provide Cognitive and Brain Reserves. Brain Res. Rev. 2009 , 61 , 221–239. [ Google Scholar ] [ CrossRef ]
- Walker, M.P. The Role of Slow Wave Sleep in Memory Processing. J. Clin. Sleep Med. 2009 , 5 , S20–S26. [ Google Scholar ] [ CrossRef ]
- Raven, F.; Van der Zee, E.A.; Meerlo, P.; Havekes, R. The Role of Sleep in Regulating Structural Plasticity and Synaptic Strength: Implications for Memory and Cognitive Function. Sleep Med. Rev. 2018 , 39 , 3–11. [ Google Scholar ] [ CrossRef ]
- Killgore, W.D.S. Effects of Sleep Deprivation on Cognition. Prog. Brain Res. 2010 , 185 , 105–129. [ Google Scholar ] [ CrossRef ] [ PubMed ]
- Zijlmans, J.L.; Riemens, M.S.; Vernooij, M.W.; Ikram, M.A.; Luik, A.I. Sleep, 24-Hour Activity Rhythms, and Cognitive Reserve: A Population-Based Study. J. Alzheimers Dis. 2023 , 91 , 663–672. [ Google Scholar ] [ CrossRef ] [ PubMed ]
- Keene, A.C.; Duboue, E.R. The Origins and Evolution of Sleep. J. Exp. Biol. 2018 , 221 , jeb159533. [ Google Scholar ] [ CrossRef ] [ PubMed ]
- Acerbi, A.; McNamara, P.; Nunn, C.L. To Sleep or Not to Sleep: The Ecology of Sleep in Artificial Organisms. BMC Ecol. 2008 , 8 , 10. [ Google Scholar ] [ CrossRef ]
- Frank, M.G. The Ontogenesis of Mammalian Sleep: Form and Function. Curr. Sleep Med. Rep. 2020 , 6 , 267–279. [ Google Scholar ] [ CrossRef ] [ PubMed ]
- Zielinski, M.R.; McKenna, J.T.; McCarley, R.W. Functions and Mechanisms of Sleep. AIMS Neurosci. 2016 , 3 , 67–104. [ Google Scholar ] [ CrossRef ]
- Borbély, A.A. A Two Process Model of Sleep Regulation. Hum. Neurobiol. 1982 , 1 , 195–204. [ Google Scholar ]
- Borbély, A.A.; Achermann, P. Sleep Homeostasis and Models of Sleep Regulation. J. Biol. Rhythms. 1999 , 14 , 557–568. [ Google Scholar ] [ CrossRef ]
- Moore, R.Y.; Eichler, V.B. Loss of a Circadian Adrenal Corticosterone Rhythm Following Suprachiasmatic Lesions in the Rat. Brain Res. 1972 , 42 , 201–206. [ Google Scholar ] [ CrossRef ]
- Borbély, A.A.; Daan, S.; Wirz-Justice, A.; Deboer, T. The Two-Process Model of Sleep Regulation: A Reappraisal. J. Sleep Res. 2016 , 25 , 131–143. [ Google Scholar ] [ CrossRef ]
- Eydipour, Z.; Nasehi, M.; Vaseghi, S.; Jamaldini, S.H.; Zarrindast, M.-R. The Role of 5-HT4 Serotonin Receptors in the CA1 Hippocampal Region on Memory Acquisition Impairment Induced by Total (TSD) and REM Sleep Deprivation (RSD). Physiol. Behav. 2020 , 215 , 112788. [ Google Scholar ] [ CrossRef ]
- Mukai, Y.; Yamanaka, A. Functional Roles of REM Sleep. Neurosci. Res. 2023 , 189 , 44–53. [ Google Scholar ] [ CrossRef ] [ PubMed ]
- Xia, Z.; Storm, D. Role of Circadian Rhythm and REM Sleep for Memory Consolidation. Neurosci. Res. 2017 , 118 , 13–20. [ Google Scholar ] [ CrossRef ] [ PubMed ]
- Villafuerte, G.; Miguel-Puga, A.; Rodríguez, E.M.; Machado, S.; Manjarrez, E.; Arias-Carrión, O. Sleep Deprivation and Oxidative Stress in Animal Models: A Systematic Review. Oxid. Med. Cell. Longev. 2015 , 2015 , 234952. [ Google Scholar ] [ CrossRef ]
- Antonioni, A.; Raho, E.M.; Sensi, M.; Di Lorenzo, F.; Fadiga, L.; Koch, G. A New Perspective on Positive Symptoms: Expression of Damage or Self-Defence Mechanism of the Brain? Neurol. Sci. 2024 , 45 , 2347–2351. [ Google Scholar ] [ CrossRef ]
- Fox, S. Accessing Active Inference Theory through Its Implicit and Deliberative Practice in Human Organizations. Entropy 2021 , 23 , 1521. [ Google Scholar ] [ CrossRef ]
- Priorelli, M.; Stoianov, I.P. Flexible Intentions: An Active Inference Theory. Front. Comput. Neurosci. 2023 , 17 , 1128694. [ Google Scholar ] [ CrossRef ]
- Diagnostic and Statistical Manual of Mental Disorders. Available online: https://dsm.psychiatryonline.org/doi/book/10.1176/appi.books.9780890425596 (accessed on 31 May 2024).
- Sateia, M.J. International Classification of Sleep Disorders-Third Edition. Chest 2014 , 146 , 1387–1394. [ Google Scholar ] [ CrossRef ] [ PubMed ]
- Baglioni, C.; Battagliese, G.; Feige, B.; Spiegelhalder, K.; Nissen, C.; Voderholzer, U.; Lombardo, C.; Riemann, D. Insomnia as a Predictor of Depression: A Meta-Analytic Evaluation of Longitudinal Epidemiological Studies. J. Affect. Disord. 2011 , 135 , 10–19. [ Google Scholar ] [ CrossRef ]
- Benz, F.; Meneo, D.; Baglioni, C.; Hertenstein, E. Insomnia Symptoms as Risk Factor for Somatic Disorders: An Umbrella Review of Systematic Reviews and Meta-Analyses. J. Sleep Res. 2023 , 32 , e13984. [ Google Scholar ] [ CrossRef ]
- Hertenstein, E.; Benz, F.; Schneider, C.L.; Baglioni, C. Insomnia-A Risk Factor for Mental Disorders. J. Sleep Res. 2023 , 32 , e13930. [ Google Scholar ] [ CrossRef ] [ PubMed ]
- Daley, M.; Morin, C.M.; LeBlanc, M.; Grégoire, J.-P.; Savard, J. The Economic Burden of Insomnia: Direct and Indirect Costs for Individuals with Insomnia Syndrome, Insomnia Symptoms, and Good Sleepers. Sleep 2009 , 32 , 55–64. [ Google Scholar ] [ PubMed ]
- Leger, M.; Quiedeville, A.; Paizanis, E.; Natkunarajah, S.; Freret, T.; Boulouard, M.; Schumann-Bard, P. Environmental Enrichment Enhances Episodic-Like Memory in Association with a Modified Neuronal Activation Profile in Adult Mice. PLoS ONE 2012 , 7 , e48043. [ Google Scholar ] [ CrossRef ] [ PubMed ]
- White, D.P. Pathogenesis of Obstructive and Central Sleep Apnea. Am. J. Respir. Crit. Care Med. 2005 , 172 , 1363–1370. [ Google Scholar ] [ CrossRef ]
- Howell, M.J. Rapid Eye Movement Sleep Behavior Disorder and Other Rapid Eye Movement Parasomnias. Continuum 2020 , 26 , 929–945. [ Google Scholar ] [ CrossRef ]
- Miglis, M.G.; Adler, C.H.; Antelmi, E.; Amaldi, D.; Baldelli, L.; Boeve, B.F.; Cesari, M.; Antonia, I.D.; Diederich, N.J.; Doppler, K.; et al. Biomarkers of Conversion to α-Synucleinopathy in Isolated Rapid-Eye-Movement Sleep Behaviour Disorder. Lancet Neurol. 2021 , 20 , 671–684. [ Google Scholar ] [ CrossRef ] [ PubMed ]
- Iranzo, A.; Ramos, L.A.; Novo, S. The Isolated Form of Rapid Eye Movement Sleep Behavior Disorder: The Upcoming Challenges. Sleep Med. Clin. 2021 , 16 , 335–348. [ Google Scholar ] [ CrossRef ]
- Gnarra, O.; Wulf, M.-A.; Schäfer, C.; Nef, T.; Bassetti, C.L.A. Rapid Eye Movement Sleep Behavior Disorder: A Narrative Review from a Technological Perspective. Sleep 2023 , 46 , zsad030. [ Google Scholar ] [ CrossRef ] [ PubMed ]
- Buysse, D.J. Sleep Health: Can We Define It? Does It Matter? Sleep 2014 , 37 , 9–17. [ Google Scholar ] [ CrossRef ]
- Meltzer, L.J.; Williamson, A.A.; Mindell, J.A. Pediatric Sleep Health: It Matters, and so Does How We Define It. Sleep Med. Rev. 2021 , 57 , 101425. [ Google Scholar ] [ CrossRef ]
- Cappuccio, F.P.; Taggart, F.M.; Kandala, N.-B.; Currie, A.; Peile, E.; Stranges, S.; Miller, M.A. Meta-Analysis of Short Sleep Duration and Obesity in Children and Adults. Sleep 2008 , 31 , 619–626. [ Google Scholar ] [ CrossRef ]
- Gallicchio, L.; Kalesan, B. Sleep Duration and Mortality: A Systematic Review and Meta-Analysis. J. Sleep Res. 2009 , 18 , 148–158. [ Google Scholar ] [ CrossRef ]
- Bock, J.; Covassin, N.; Somers, V. Excessive Daytime Sleepiness: An Emerging Marker of Cardiovascular Risk. Heart 2022 , 108 , 1761–1766. [ Google Scholar ] [ CrossRef ]
- Yan, B.; Yang, J.; Zhao, B.; Fan, Y.; Wang, W.; Ma, X. Objective Sleep Efficiency Predicts Cardiovascular Disease in a Community Population: The Sleep Heart Health Study. J. Am. Heart Assoc. 2021 , 10 , e016201. [ Google Scholar ] [ CrossRef ]
- Xi, B.; He, D.; Zhang, M.; Xue, J.; Zhou, D. Short Sleep Duration Predicts Risk of Metabolic Syndrome: A Systematic Review and Meta-Analysis. Sleep Med. Rev. 2014 , 18 , 293–297. [ Google Scholar ] [ CrossRef ]
- Becker, S.P.; Sidol, C.A.; Van Dyk, T.R.; Epstein, J.N.; Beebe, D.W. Intraindividual Variability of Sleep/Wake Patterns in Relation to Child and Adolescent Functioning: A Systematic Review. Sleep Med. Rev. 2017 , 34 , 94–121. [ Google Scholar ] [ CrossRef ]
- Yan, B.; Zhao, B.; Jin, X.; Xi, W.; Yang, J.; Yang, L.; Ma, X. Sleep Efficiency May Predict Depression in a Large Population-Based Study. Front. Psychiatry 2022 , 13 , 838907. [ Google Scholar ] [ CrossRef ]
- Sharma, S.; Kavuru, M. Sleep and Metabolism: An Overview. Int. J. Endocrinol. 2010 , 2010 , 270832. [ Google Scholar ] [ CrossRef ]
- Tai, X.Y.; Chen, C.; Manohar, S.; Husain, M. Impact of Sleep Duration on Executive Function and Brain Structure. Commun. Biol. 2022 , 5 , 201. [ Google Scholar ] [ CrossRef ] [ PubMed ]
- Yoo, S.-S.; Gujar, N.; Hu, P.; Jolesz, F.A.; Walker, M.P. The Human Emotional Brain without Sleep--a Prefrontal Amygdala Disconnect. Curr. Biol. 2007 , 17 , R877–R878. [ Google Scholar ] [ CrossRef ] [ PubMed ]
- Meneo, D.; Samea, F.; Tahmasian, M.; Baglioni, C. The Emotional Component of Insomnia Disorder: A Focus on Emotion Regulation and Affect Dynamics in Relation to Sleep Quality and Insomnia. J. Sleep Res. 2023 , 32 , e13983. [ Google Scholar ] [ CrossRef ]
- Kerner, N.; Goldberg, T.E.; Cohen, H.R.; Phillips, J.G.; Cohen, D.E.; Andrews, H.; Pelton, G.; Devanand, D.P. Sleep-Wake Behavior, Perceived Fatigability, and Cognitive Reserve in Older Adults. Alzheimers Dement. 2024 , 20 , 4020–4031. [ Google Scholar ] [ CrossRef ]
- Buzsáki, G. Hippocampal Sharp Wave-ripple: A Cognitive Biomarker for Episodic Memory and Planning. Hippocampus 2015 , 25 , 1073–1188. [ Google Scholar ] [ CrossRef ]
- Khan, M.A.; Al-Jahdali, H. The Consequences of Sleep Deprivation on Cognitive Performance. Neurosciences 2023 , 28 , 91–99. [ Google Scholar ] [ CrossRef ]
- Diekelmann, S.; Born, J. The Memory Function of Sleep. Nat. Rev. Neurosci. 2010 , 11 , 114–126. [ Google Scholar ] [ CrossRef ]
- Papalambros, N.A.; Santostasi, G.; Malkani, R.G.; Braun, R.; Weintraub, S.; Paller, K.A.; Zee, P.C. Acoustic Enhancement of Sleep Slow Oscillations and Concomitant Memory Improvement in Older Adults. Front. Hum. Neurosci. 2017 , 11 , 109. [ Google Scholar ] [ CrossRef ]
- Page, M.J.; McKenzie, J.E.; Bossuyt, P.M.; Boutron, I.; Hoffmann, T.C.; Mulrow, C.D.; Shamseer, L.; Tetzlaff, J.M.; Akl, E.A.; Brennan, S.E.; et al. The PRISMA 2020 Statement: An Updated Guideline for Reporting Systematic Reviews. BMJ 2021 , 372 , n71. [ Google Scholar ] [ CrossRef ]
- Zimmerman, M.E.; Bigal, M.E.; Katz, M.J.; Brickman, A.M.; Lipton, R.B. Sleep Onset/Maintenance Difficulties and Cognitive Function in Nondemented Older Adults: The Role of Cognitive Reserve. J. Int. Neuropsychol. Soc. 2012 , 18 , 461–470. [ Google Scholar ] [ CrossRef ]
- Parker, D.; Bucks, R.S.; Rainey-Smith, S.R.; Hodgson, E.; Fine, L.; Sohrabi, H.R.; Martins, R.N.; Weinborn, M. Sleep Mediates Age-Related Executive Function for Older Adults with Limited Cognitive Reserve. J. Int. Neuropsychol. Soc. 2021 , 27 , 711–721. [ Google Scholar ] [ CrossRef ] [ PubMed ]
- Yeh, A.-Y.; Pressler, S.J.; Algase, D.; Struble, L.M.; Pozehl, B.J.; Berger, A.M.; Giordani, B.J. Sleep-Wake Disturbances and Episodic Memory in Older Adults. Biol. Res. Nurs. 2021 , 23 , 141–150. [ Google Scholar ] [ CrossRef ] [ PubMed ]
- Ourry, V.; Rehel, S.; André, C.; Mary, A.; Paly, L.; Delarue, M.; Requier, F.; Hendy, A.; Collette, F.; Marchant, N.L.; et al. Effect of Cognitive Reserve on the Association between Slow Wave Sleep and Cognition in Community-Dwelling Older Adults. Aging 2023 , 15 , 9275–9292. [ Google Scholar ] [ CrossRef ] [ PubMed ]
- Alchanatis, M.; Zias, N.; Deligiorgis, N.; Amfilochiou, A.; Dionellis, G.; Orphanidou, D. Sleep Apnea-Related Cognitive Deficits and Intelligence: An Implication of Cognitive Reserve Theory. J. Sleep Res. 2005 , 14 , 69–75. [ Google Scholar ] [ CrossRef ] [ PubMed ]
- Olaithe, M.; Pushpanathan, M.; Hillman, D.; Eastwood, P.R.; Hunter, M.; Skinner, T.; James, A.; Wesnes, K.A.; Bucks, R.S. Cognitive Profiles in Obstructive Sleep Apnea: A Cluster Analysis in Sleep Clinic and Community Samples. J. Clin. Sleep Med. 2020 , 16 , 1493–1505. [ Google Scholar ] [ CrossRef ] [ PubMed ]
- Hlaing, E.E.; Dollinger, S.M.C.; Brown, T.M. The Role of Education in Cognitive Functions among Middle-Age and Older Patients with Untreated Obstructive Sleep Apnea. Sleep Sci. 2021 , 14 , 319–329. [ Google Scholar ] [ CrossRef ] [ PubMed ]
- D’Este, G.; Berra, F.; Carli, G.; Leitner, C.; Marelli, S.; Zucconi, M.; Casoni, F.; Ferini-Strambi, L.; Galbiati, A. Cognitive Reserve in Isolated Rapid Eye-Movement Sleep Behavior Disorder. Brain Sci. 2023 , 13 , 176. [ Google Scholar ] [ CrossRef ] [ PubMed ]
- Prete, M.; Cellini, N.; Ronconi, L.; Di Rosa, E. Cognitive Reserve Moderates the Relationship between Sleep Difficulties and Executive Functions in Patients with Parkinson’s Disease. Sleep Med. 2023 , 111 , 82–85. [ Google Scholar ] [ CrossRef ]
- Zavecz, Z.; Shah, V.D.; Murillo, O.G.; Vallat, R.; Mander, B.A.; Winer, J.R.; Jagust, W.J.; Walker, M.P. NREM Sleep as a Novel Protective Cognitive Reserve Factor in the Face of Alzheimer’s Disease Pathology. BMC Med. 2023 , 21 , 156. [ Google Scholar ] [ CrossRef ]
- Diekelmann, S. Sleep for Cognitive Enhancement. Front. Syst. Neurosci. 2014 , 8 , 46. [ Google Scholar ] [ CrossRef ]
- Born, J.; Rasch, B.; Gais, S. Sleep to Remember. Neuroscientist 2006 , 12 , 410–424. [ Google Scholar ] [ CrossRef ]
- Wick, A.; Rasch, B. Targeted Memory Reactivation during Slow-Wave Sleep vs. Sleep Stage N2: No Significant Differences in a Vocabulary Task. Learn. Mem. 2023 , 30 , 192–200. [ Google Scholar ] [ CrossRef ]
- Manly, J.J.; Touradji, P.; Tang, M.-X.; Stern, Y. Literacy and Memory Decline among Ethnically Diverse Elders. J. Clin. Exp. Neuropsychol. 2003 , 25 , 680–690. [ Google Scholar ] [ CrossRef ] [ PubMed ]
- Wilckens, K.A.; Ferrarelli, F.; Walker, M.P.; Buysse, D.J. Slow-Wave Activity Enhancement to Improve Cognition. Trends Neurosci. 2018 , 41 , 470–482. [ Google Scholar ] [ CrossRef ]
- Himali, J.J.; Baril, A.-A.; Cavuoto, M.G.; Yiallourou, S.; Wiedner, C.D.; Himali, D.; DeCarli, C.; Redline, S.; Beiser, A.S.; Seshadri, S.; et al. Association Between Slow-Wave Sleep Loss and Incident Dementia. JAMA Neurol. 2023 , 80 , 1326–1333. [ Google Scholar ] [ CrossRef ] [ PubMed ]
- Stern, Y. Cognitive Reserve. Neuropsychologia 2009 , 47 , 2015–2028. [ Google Scholar ] [ CrossRef ]
- Pettigrew, C.; Soldan, A. Defining Cognitive Reserve and Implications for Cognitive Aging. Curr. Neurol. Neurosci. Rep. 2019 , 19 , 1. [ Google Scholar ] [ CrossRef ]
- Ramos, H.; Alacreu, M.; Guerrero, M.D.; Sánchez, R.; Moreno, L. Lifestyle Variables Such as Daily Internet Use, as Promising Protective Factors against Cognitive Impairment in Patients with Subjective Memory Complaints. Preliminary Results. J. Pers. Med. 2021 , 11 , 1366. [ Google Scholar ] [ CrossRef ]
- Kaur, A.; Sonal, A.; Ghosh, T.; Ahamed, F. Cognitive Reserve and Other Determinants of Cognitive Function in Older Adults: Insights from a Community-Based Cross-Sectional Study. J. Fam. Med. Prim. Care 2023 , 12 , 1957–1964. [ Google Scholar ] [ CrossRef ]
- Savarimuthu, A.; Ponniah, R.J. Cognition and Cognitive Reserve. Integr. Psychol. Behav. Sci. 2024 , 58 , 483–501. [ Google Scholar ] [ CrossRef ] [ PubMed ]
- Bubu, O.M.; Brannick, M.; Mortimer, J.; Umasabor-Bubu, O.; Sebastião, Y.V.; Wen, Y.; Schwartz, S.; Borenstein, A.R.; Wu, Y.; Morgan, D.; et al. Sleep, Cognitive Impairment, and Alzheimer’s Disease: A Systematic Review and Meta-Analysis. Sleep 2017 , 40 . [ Google Scholar ] [ CrossRef ]
- Vance, D.E.; Roberson, A.J.; McGuinness, T.M.; Fazeli, P.L. How Neuroplasticity and Cognitive Reserve Protect Cognitive Functioning. J. Psychosoc. Nurs. Ment. Health Serv. 2010 , 48 , 23–30. [ Google Scholar ] [ CrossRef ]
- Fortier-Brochu, E.; Beaulieu-Bonneau, S.; Ivers, H.; Morin, C.M. Insomnia and Daytime Cognitive Performance: A Meta-Analysis. Sleep Med. Rev. 2012 , 16 , 83–94. [ Google Scholar ] [ CrossRef ] [ PubMed ]
- Uddin, M.S.; Tewari, D.; Mamun, A.A.; Kabir, M.T.; Niaz, K.; Wahed, M.I.I.; Barreto, G.E.; Ashraf, G.M. Circadian and Sleep Dysfunction in Alzheimer’s Disease. Ageing Res. Rev. 2020 , 60 , 101046. [ Google Scholar ] [ CrossRef ] [ PubMed ]
- Stern, Y.; Barulli, D. Cognitive Reserve. Handb. Clin. Neurol. 2019 , 167 , 181–190. [ Google Scholar ] [ CrossRef ] [ PubMed ]
- Cadar, D.; Robitaille, A.; Clouston, S.; Hofer, S.M.; Piccinin, A.M.; Muniz-Terrera, G. An International Evaluation of Cognitive Reserve and Memory Changes in Early Old Age in 10 European Countries. Neuroepidemiology 2017 , 48 , 9–20. [ Google Scholar ] [ CrossRef ]
Reference | Population | Age Range (Years) | Mean Age (Years) | Sex | Sample Size (N) |
---|---|---|---|---|---|
Zimmerman et al. (2012) [ ] | SO/MD L.E. F 67.2% | 549 | |||
Healthy | 71–97 | 79.7 | SO/MD H.E. F 60% | ||
No SO/MD L.E. F 59% | |||||
No SO/MD H.E F 62.4% | |||||
Parker et al. (2020) [ ] | Healthy | 55–93 | 71.7 | N/A | 184 |
Yeh et al. (2021) [ ] | Healthy | 60–88 | 69.9 | F 75.8% | 62 |
Ourry et al. (2023) [ ] | Healthy | 65–84 | 69.4 | F 61.5% | 135 |
Alchanatis e al. (2005) [ ] | OSA | N/A | N/A | N/A | 83 |
Olaithe et al. (2020) [ ] | OSA | 40–65 | SCS 53.94 | SCS M N = 64; 52.9% | 519 |
CS 60 | CS M N = 245; 61% | ||||
Hlaing et al. (2021) [ ] | OSA | OSA 40–92 | OSA 54.82 | OSA F 56.5% | 109 |
CTRL 40–81 | CTRL 56.60 | CTRL F 80.9% | |||
D’Este et al. (2023) [ ] | iRBD | 50–78 | 66.38 | F 80% | 55 |
Prete et al. (2023) [ ] | PD | N/A | 63.81 | F N = 60.4% | 43 |
Zijlmans et al. (2023) [ ] | Healthy | 58–72 | 65.0 | F 51.3% | 1002 |
Zavecz et al. (2023) [ ] | AD | 68–72 | Aβ+ 75.97 | F Aβ+ 68% | 62 |
Aβ− 75.26 | F Aβ− 55% |
The statements, opinions and data contained in all publications are solely those of the individual author(s) and contributor(s) and not of MDPI and/or the editor(s). MDPI and/or the editor(s) disclaim responsibility for any injury to people or property resulting from any ideas, methods, instructions or products referred to in the content. |
Share and Cite
Balsamo, F.; Berretta, E.; Meneo, D.; Baglioni, C.; Gelfo, F. The Complex Relationship between Sleep and Cognitive Reserve: A Narrative Review Based on Human Studies. Brain Sci. 2024 , 14 , 654. https://doi.org/10.3390/brainsci14070654
Balsamo F, Berretta E, Meneo D, Baglioni C, Gelfo F. The Complex Relationship between Sleep and Cognitive Reserve: A Narrative Review Based on Human Studies. Brain Sciences . 2024; 14(7):654. https://doi.org/10.3390/brainsci14070654
Balsamo, Francesca, Erica Berretta, Debora Meneo, Chiara Baglioni, and Francesca Gelfo. 2024. "The Complex Relationship between Sleep and Cognitive Reserve: A Narrative Review Based on Human Studies" Brain Sciences 14, no. 7: 654. https://doi.org/10.3390/brainsci14070654
Article Metrics
Article access statistics, further information, mdpi initiatives, follow mdpi.
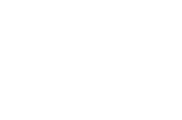
Subscribe to receive issue release notifications and newsletters from MDPI journals
Research explores reversing memory loss with existing drugs
- Download PDF Copy
The loss of social memories caused by sleep deprivation could potentially be reversed using currently available drugs, according to a study in mice presented today (Friday) at the Federation of European Neuroscience Societies (FENS) Forum 2024. Lack of sleep is known to affect the brain, including memory, in mice and in humans, but research is beginning to show that these memories are not lost, they are just 'hidden' in the brain and difficult to retrieve. The new research shows that access to these otherwise hidden social memories can be restored in mice with a drug currently used to treat asthma and chronic obstructive pulmonary disease. The team of researchers have also shown that another drug currently used to treat erectile dysfunction can restore access to spatial memories. Researchers say these spatial memories in mice are akin to humans remembering where they put their keys the night before, whereas the social memories could be compared with remembering a new person you met. The research was presented by Dr Robbert Havekes from the University of Groningen in the Netherlands. He said: "Ever since starting as a PhD student, many years ago, I have been intrigued by the observation that even a single period of sleep deprivation can have a major impact on memory processes and the brain as a whole. The early work published years ago helped us identify some of the molecular mechanisms that mediate amnesia.
By manipulating these pathways specifically in the hippocampus, we have been able to make memory processes resilient to the negative impact of sleep deprivation. In our new studies, we have examined whether we could reverse amnesia even days after the initial learning event and period of sleep deprivation." Dr Robbert Havekes, University of Groningen
The new studies, presented at the FENS Forum and funded by the Air Force Office of Scientific Research (AFOSR), were conducted by Dr Havekes' PhD students Adithya Sarma and Camilla Paraciani, who will also be presenting their work as poster presentations. To study social memories in the lab, the researchers gave mice the opportunity to choose between interacting with a mouse they have never encountered before or a sibling from their own cage. Under normal circumstances, the mice prefer interacting with the new mouse over their litter-mate that they already know. Given the same choice the next day, mice will interact to a similar extent with both their litter-mate and the mouse they met the day before as both mice are now considered familiar. However, if the mice are sleep-deprived after their first encounter then the next day they still prefer to interact with the new mouse as if they never met it before. These findings suggest that they simply cannot recall their previous encounter. The team found they were able to permanently restore these hidden social memories, first using a technique called optogenetic engram technology. This technique allows them to identify neurons in the brain that together form a memory (known as a memory engram) for a specific experience and alter those neurons so they can be reactivated by light. Researchers can then use light to reactivate this specific group of neurons resulting in the recall of the specific experience (in this case a social memory). They were also able to restore the mice's social memories by treating them with roflumilast, a type of anti-inflammatory drug, approved by the US Food and Drug Administration, that is used to treat chronic obstructive pulmonary disease. Dr Havekes says this finding is particularly interesting as it provides a stepping stone towards studies of sleep deprivation and memory in humans, and he is now collaborating with another research group that is embarking on human studies. In parallel, the same researchers have investigated the loss of spatial memory caused by sleep deprivation by studying mice's abilities to learn and remember the location of individual objects. A brief period of sleep deprivation following training meant the mice could not recall the original locations of the object and so they did not notice when an object was moved to a new location during a test. As with the social memories, access to these spatial memories could be restored by treating the mice with another drug, vardenafil, that is currently used to treat erectile dysfunction. This is a second drug that is approved by the US Food and Drug Administration that the researchers have successfully used to reverse amnesia in mice. Dr Havekes said: "We have been able to show that sleep deprivation leads to amnesia in the case of specific spatial and social recognition memories. This amnesia can be reversed days later after the initial learning experience and sleep deprivation episode using drugs already approved for human consumption. We now want to focus on understanding what processes are at the core of these accessible and inaccessible memories. In the long term, we hope that these fundamental studies will help pave the way for studies in humans aimed at reversing forgetfulness by restoring access to otherwise inaccessible information in the brain." Professor Richard Roche is chair of the FENS Forum communication committee and Deputy Head of the Department of Psychology at Maynooth University, Maynooth, County Kildare, Ireland, and was not involved in the research. He said: "This research shows that social and spatial memories seemingly lost through sleep-deprivation can be recovered. Although these studies were carried out in mice, they suggest that it may be possible to recover people's lost social and spatial memories using certain drug treatments that are already approved for human use. There are many situations where people cannot get the amount of sleep they need, so this area of research has obvious potential. However, it will take time and a lot more work to move this research from mice into humans."
Federation of European Neuroscience Societies
Posted in: Medical Science News | Medical Research News | Healthcare News
Tags: Amnesia , Anti-Inflammatory , Asthma , Brain , Chronic , Chronic Obstructive Pulmonary Disease , Drugs , Erectile Dysfunction , Food , Hippocampus , Neurons , Neuroscience , Psychology , Research , Sleep , students , Technology
Suggested Reading

Cancel reply to comment
- Trending Stories
- Latest Interviews
- Top Health Articles

Revolutionizing Life Science: An Interview with SCIEX on ASMS, the SCIEX 7500+ System, and AI-Driven Quantitation
Jose Castro-Perez and Chris Lock, SCIEX
In our latest interview, News Medical speaks with SCIEX, a global leader in life science analytical technologies, about their exciting announcements at ASMS, the SCIEX 7500+ System, and how they utilize AI quantitation software to streamline solutions.

From Discovery Biology to ELRIG Chair
Melanie Leveridge
In this interview, we speak with Melanie Leveridge, Vice President of Discovery Biology at AstraZeneca and Chair of the Board for ELRIG UK, to discuss her extensive career in the pharmaceutical industry, her role in fostering scientific innovation, and her vision for ELRIG's future.

Breathing New Life into Diagnostics: Plasmion's SICRIT Technology
Revolutionizing Non-Invasive Diagnostics with Plasmion’s SICRIT Breath Analysis.

Latest News

Newsletters you may be interested in

Your AI Powered Scientific Assistant
Hi, I'm Azthena, you can trust me to find commercial scientific answers from News-Medical.net.
A few things you need to know before we start. Please read and accept to continue.
- Use of “Azthena” is subject to the terms and conditions of use as set out by OpenAI .
- Content provided on any AZoNetwork sites are subject to the site Terms & Conditions and Privacy Policy .
- Large Language Models can make mistakes. Consider checking important information.
Great. Ask your question.
Azthena may occasionally provide inaccurate responses. Read the full terms .
While we only use edited and approved content for Azthena answers, it may on occasions provide incorrect responses. Please confirm any data provided with the related suppliers or authors. We do not provide medical advice, if you search for medical information you must always consult a medical professional before acting on any information provided.
Your questions, but not your email details will be shared with OpenAI and retained for 30 days in accordance with their privacy principles.
Please do not ask questions that use sensitive or confidential information.
Read the full Terms & Conditions .
Provide Feedback


- Advanced Search
LAUREATE: A Dataset for Supporting Research in Affective Computing and Human Memory Augmentation

New Citation Alert added!
This alert has been successfully added and will be sent to:
You will be notified whenever a record that you have chosen has been cited.
To manage your alert preferences, click on the button below.
New Citation Alert!
Please log in to your account
Information & Contributors
Bibliometrics & citations, index terms.
Applied computing
Human-centered computing
Human computer interaction (HCI)
Empirical studies in HCI
Ubiquitous and mobile computing
Empirical studies in ubiquitous and mobile computing
Recommendations
Affect and learning in the laureate dataset.
Affective states and cognition share a close relationship, influencing each other in several ways. In particular, our affect, i.e. our feelings and mood, impacts our perception of the world, our memory, and our decisions. This relationship also ...
Exploring LAUREATE - the Longitudinal multimodAl stUdent expeRience datasEt for AffecT and mEmory research
Studies in the lab have shown that affect recognition using physiological data is feasible with machine learning methods. Datasets collected in-the-wild can further improve such methods’ robustness and applicability. This study presents LAUREATE, a ...
Affective guidance of intelligent agents: How emotion controls cognition
How do emotions and moods color cognition? In this article, we examine how such reactions influence both judgments and cognitive performance. We argue that many affective influences are due, not to affective reactions themselves, but to the information ...
Information
Published in.

Association for Computing Machinery
New York, NY, United States
Publication History
Permissions, check for updates, author tags.
- affective computing
- human memory augmentation
- physiological signals
- student performance
- Research-article
Contributors
Other metrics, bibliometrics, article metrics.
- 0 Total Citations
- 373 Total Downloads
- Downloads (Last 12 months) 373
- Downloads (Last 6 weeks) 27
View Options
Login options.
Check if you have access through your login credentials or your institution to get full access on this article.
Full Access
View options.
View or Download as a PDF file.
View online with eReader .
Share this Publication link
Copying failed.

Share on social media
Affiliations, export citations.
- Please download or close your previous search result export first before starting a new bulk export. Preview is not available. By clicking download, a status dialog will open to start the export process. The process may take a few minutes but once it finishes a file will be downloadable from your browser. You may continue to browse the DL while the export process is in progress. Download
- Download citation
- Copy citation
We are preparing your search results for download ...
We will inform you here when the file is ready.
Your file of search results citations is now ready.
Your search export query has expired. Please try again.
Thank you for visiting nature.com. You are using a browser version with limited support for CSS. To obtain the best experience, we recommend you use a more up to date browser (or turn off compatibility mode in Internet Explorer). In the meantime, to ensure continued support, we are displaying the site without styles and JavaScript.
- View all journals
- Explore content
- About the journal
- Publish with us
- Sign up for alerts
- Open access
- Published: 19 June 2024
Spindle-locked ripples mediate memory reactivation during human NREM sleep
- Thomas Schreiner ORCID: orcid.org/0000-0003-2030-5998 1 ,
- Benjamin J. Griffiths ORCID: orcid.org/0000-0001-8600-4480 1 , 2 ,
- Merve Kutlu 1 ,
- Christian Vollmar 3 ,
- Elisabeth Kaufmann ORCID: orcid.org/0000-0002-7582-2215 3 ,
- Stefanie Quach ORCID: orcid.org/0000-0003-2914-3649 4 ,
- Jan Remi 3 ,
- Soheyl Noachtar 3 &
- Tobias Staudigl ORCID: orcid.org/0000-0003-2885-1280 1
Nature Communications volume 15 , Article number: 5249 ( 2024 ) Cite this article
1000 Accesses
24 Altmetric
Metrics details
- Cognitive neuroscience
- Long-term memory
Memory consolidation relies in part on the reactivation of previous experiences during sleep. The precise interplay of sleep-related oscillations (slow oscillations, spindles and ripples) is thought to coordinate the information flow between relevant brain areas, with ripples mediating memory reactivation. However, in humans empirical evidence for a role of ripples in memory reactivation is lacking. Here, we investigated the relevance of sleep oscillations and specifically ripples for memory reactivation during human sleep using targeted memory reactivation. Intracranial electrophysiology in epilepsy patients and scalp EEG in healthy participants revealed that elevated levels of slow oscillation - spindle activity coincided with the read-out of experimentally induced memory reactivation. Importantly, spindle-locked ripples recorded intracranially from the medial temporal lobe were found to be correlated with the identification of memory reactivation during non-rapid eye movement sleep. Our findings establish ripples as key-oscillation for sleep-related memory reactivation in humans and emphasize the importance of the coordinated interplay of the cardinal sleep oscillations.
Similar content being viewed by others
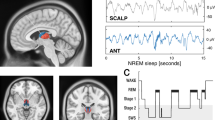
The human thalamus orchestrates neocortical oscillations during NREM sleep
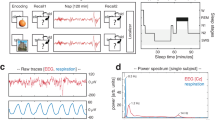
Respiration modulates sleep oscillations and memory reactivation in humans
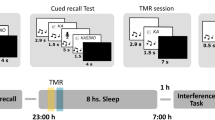
Comparing targeted memory reactivation during slow wave sleep and sleep stage 2
Introduction.
Contemporary models propose that memory consolidation, i.e., the strengthening of memories during sleep, is achieved in part by reactivating experiences that were encoded during prior wakefulness 1 , 2 . Through reactivation, memories are relayed between the hippocampus and cortical long-term stores, transforming initially labile memories into long-lasting ones 3 . The essential communication between the hippocampus, thalamus, and cortex, as well as the strengthening of memories in cortical networks, is thought to be facilitated by precise temporal coordination between the cardinal non-rapid eye movement (NREM) sleep-related oscillations, namely cortical slow oscillations (SOs), thalamocortical sleep spindles and hippocampal ripples 4 , 5 , 6 .
SOs (< 1 Hz) initiate time windows of excitability and inhibition not only in cortical but also in subcortical areas 7 , 8 , 9 . They ignite the generation of sleep spindles in the thalamus, which nest in the excitable upstates of cortical SOs 10 , 11 . Spindles (12–16 Hz), in turn, have been shown to gate Ca2+ influx into dendrites, putatively facilitating synaptic plasticity in cortical areas 12 , 13 , 14 . Lastly, hippocampal sharp-wave ripples (80–120 Hz in humans) are assumed to coordinate neural population dynamics in the hippocampus to reactivate recently formed memories 15 , 16 . Ripples tend to occur during the excitable troughs of spindles 17 , 18 . The formation of such spindle-ripple events is thought to facilitate the transfer of reactivated memories to the cortex 19 , 20 . Hence, while SO-spindle coupling is assumed to ensure that cortical target areas are optimally tuned for synaptic plasticity when memories are reactivated, memory consolidation ultimately relies on ripples to trigger and coordinate memory reactivation processes both in the hippocampus and cortical long-term stores 16 .
Studies using intracranial recordings in epileptic patients have established the hierarchical synchronization of SOs, spindles, and ripples during human NREM sleep 17 , 21 , 22 , 23 , 24 , 25 , 26 . However, whether spindle-locked ripples contribute to memory consolidation by mediating memory reactivation in humans is currently unknown. Here, we set out to assess the relevance of sleep oscillations and specifically sharp wave ripples for memory reactivation during human NREM sleep. We recorded scalp EEG in healthy participants and intracranial EEG in epilepsy patients while they retrieved real-world spatial memories (i.e., prior learned head orientation – image associations). Importantly, head orientations were linked to specific sound cues, which were presented again during subsequent NREM sleep to trigger the reactivation of head orientation-related memories (i.e., targeted memory reactivation, TMR 27 ). Using multivariate classification, we find that head orientation-related electrophysiological signatures are reactivated during successful awake memory retrieval as well as during TMR while participants were asleep. During sleep, elevated levels of SO-spindle activity promote the read-out of memory reactivation in both scalp and intracranial EEG recordings. Leveraging direct access to medial temporal lobe (MTL) electrophysiology in epilepsy patient, we show that memory reactivation is tightly locked to spindle-locked ripples during human sleep, establishing a role of sharp wave-ripples for memory reactivation in human NREM sleep.
Results [EEG – healthy participants]
Twenty-five participants (age: 25.2 ± 0.6; 16 female) took part in the scalp EEG study. Experimental sessions started in the evening around 7 p.m. After an initial training phase (see Methods), participants performed a real-world spatial memory task, where they learned to associate 168 items (images of objects) with specific head orientations (see Fig. 1a ). Importantly, a specific sound cue was assigned to each of the four non-central head orientations. Memory performance was tested in a stepwise manner. First, participants made object-recognition judgments for all old items, randomly intermixed with new items. Then, for recognized items only, participants indicated which of the four head orientations was associated with the item during the learning phase (associative retrieval, Fig. 1a ). Participants received no feedback during the retrieval test. After finishing the memory task, participants went to sleep. During one hour of NREM sleep, two out of the four sounds (one sound associated with the right-sided and one with the left-sided head orientations, respectively) were repeatedly presented as TMR cues, while an additional sound, unrelated to any learning, served as a control sound. We reasoned that presenting TMR cues during sleep would ignite reactivation of the related head orientations and the associated items.
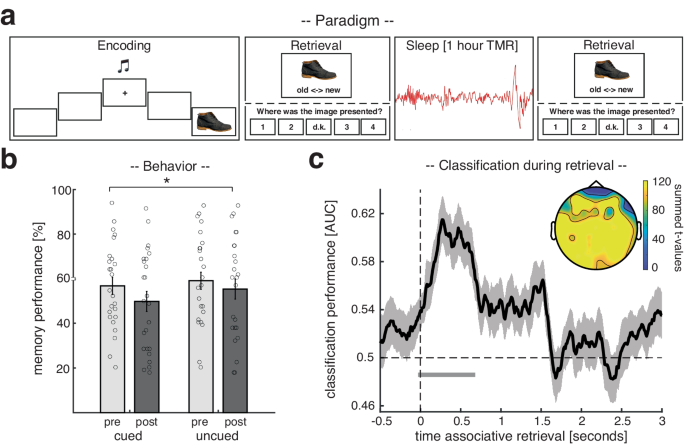
a During encoding, participants were consecutively presented with 168 images (EEG study) / 144 images (intracranial EEG study) of objects on four flanking screens (positioned at − 60°, − 30°, + 30° and +60° relative to the center screen). Participants turned their heads towards the relevant screen, cued by one of four orientation-specific sounds. Memory performance was tested via a recognition test followed by an associative retrieval (this procedure was used before and after sleep): First, participants made object-recognition judgments (old or new). Then, for recognized images only, participants indicated which of the four head orientations was associated with the item during the learning phase. During NREM sleep, two of the learning-related sounds (one related to left-sided and one related to right-sided head orientation) and one control sound, which was not part of the learning material, were presented for 60 min. b Behavioral results for both experimental sessions pre- (light gray) and post-sleep (dark gray), separated into cued and uncued trials. Bar graphs show the mean ( ± SEM across participants) percentage of recalled head orientations. Dots indicate individual memory performance of participants ( N = 25). The star denotes the significant interaction (pre vs. post x cued vs. uncued) as derived from a repeated measures ANOVA (F 1,24 = 5.48; p = 0.028). c Later cued head orientations (left vs. right) could be reliably decoded (above chance) from the retrieval data, starting around the onset of the associative memory prompt (the black solid line indicates decoding performance ( ± SEM across participants)). The horizontal dashed line indicates chance level performance (i.e., 0.5). The vertical solid line indicates the onset of associative retrieval trials (time = 0). The lower horizontal gray line shows the temporal extent of significant decoding results as derived from a two-sided cluster-based permutation test ( p = 0.0009, corrected for multiple comparisons across time, N = 25). The topographical insert illustrates the results of a “searchlight decoding procedure”, indicating that bilateral centro-parietal and occipital areas exhibited stimulus-category related effects (please note that statistical tests with regards to the searchlight procedure were done for illustrative purposes only, N = 25). Source data are provided as a Source Data file.
Behavioral results
To test for potential differences in memory performance between test times and TMR conditions, we conducted an ANOVA for the cued recall, including the factors cueing (cued vs. uncued) and test-time (pre- vs. post- sleep). Results indicated that memory performance declined throughout sleep (main factor test-time: F 1,24 = 19.24; p < 0.001). Importantly though, the interaction between test-time and cueing (F 1,24 = 5.48; p = 0.028) was also significant, indicating that TMR did modulate memory performance. However, TMR did not benefit memory performance as expected 28 , but had a detrimental effect on retrieval abilities (cued pre-sleep: 57.23 ± 3.92% vs. cued post-sleep: 50.42 ± 4.56%; uncued pre-sleep: 58.76 ± 4.13% vs. uncued post-sleep: 54.90 ± 4.61%; see Fig. 1b ). A follow up post-hoc t-test (relative memory performance pre- to post-sleep) also indicated that uncued items were better remembered as compared to cued items (t 1,24 = 2.747; p = 0.011). For recognition memory (hit rate from old/new judgments), we neither found a significant main effect of test time (F 1,24 = 0.29; p = 0.59); nor a significant interaction between test-time and cueing (F 1,24 = 0.08; p = 0.77; see Supplementary Fig. 1 for details).
Head orientation-related activity is reactivated during successful retrieval
Next, we set out to test whether we could decode head orientation-related activity from EEG signals during retrieval, which would allow us to track corresponding reactivation processes during TMR (see below). To extract head orientation-related patterns of neuronal activity during retrieval, we pooled the data from the associative retrieval (i.e., when participants had to remember image related head orientations) across pre- and post-sleep sessions. Furthermore, we restricted the analysis to those items whose head orientations were remembered correctly and that were selected for TMR (i.e., one left sided and one right sided head orientation per participant). We performed multivariate classification (linear discriminant analysis; LDA) on these data (Fig. 1c ). Using fivefold cross-validation (see Methods), above-chance classification accuracy emerged around the onset of the associative memory prompt (time window: -30 ms to 680 ms; peak at 270 ms; p < 0.001, corrected for multiple comparisons across time). The fact that decoding accuracies ramped up slightly before the onset of the memory prompt indicates that associative retrieval processes putatively started already towards the end of old/new judgments (i.e., recognition testing; see Supplementary Fig. 2 for classification locked to recognition onset). Taken together, the retrieval data allowed us to isolate brain patterns associated with the reactivation of head orientation-related activity, which we then used to guide the analysis of memory reactivation during TMR (for results concerning the classification of later uncued head orientations during retrieval see Supplementary Fig. 3 ).
TMR elicits reactivation of head orientation-related activity during NREM sleep
First, we tested whether TMR-induced electrophysiological activity would discriminate between learning-related and control sounds. Consistent with previous findings 29 , 30 , 31 , learned TMR cues, as compared to control cues, triggered a significant power increase in the SO-spindle range (i.e., an initial low frequency burst followed by a fast spindle burst; p = 0.002, corrected for multiple comparisons across time, frequency, and space; see Fig. 2a ), foreshadowing that learning-related TMR cues might have triggered relevant neuronal processing in the sleeping brain (for spindle characteristics associated with experimental and control sounds see Supplementary Fig. 4 ).
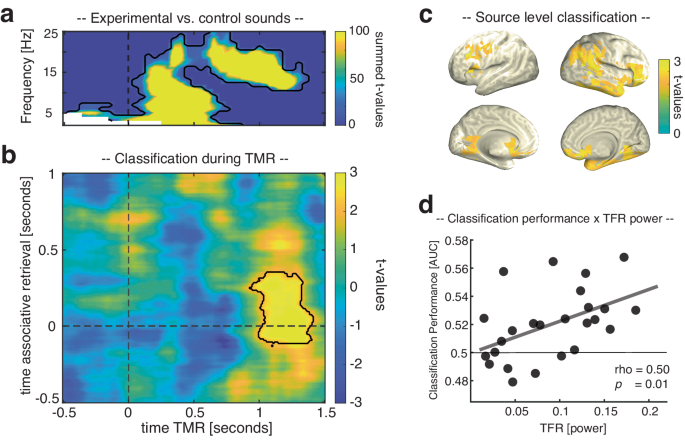
a Power difference between learning-related TMR cues versus new control cues after statistical thresholding ( p = 0.002, two-sided cluster-based permutation test corrected for multiple comparisons; N = 25) (b) Retrieval-related brain patterns (left vs. right head orientations) were decodable during TMR ( p = 0.023, two-sided cluster-based permutation test corrected for multiple comparisons across time, contour lines indicate the extent of the cluster, N = 25); color range (blue to yellow) represents t values against chance level performance. c The source plots illustrate the results of a “searchlight decoding procedure”, indicating that frontoparietal networks and the right medial temporal lobe exhibited head orientation related effects (please note that statistical tests for the searchlight procedure were done for illustrative purposes only, N = 25). d Classification performance correlated positively with TMR-triggered power (Spearman correlation; r = 0.50, p = 0.01; N = 25). Source data are provided as a Source Data file.
To specifically test this, we next determined whether neuronal activity related to remembered head orientations would be reactivated during TMR. We first trained a classifier on the pooled associative retrieval data from both pre- and post-sleep sessions [− 0.5 to 1 s]. The resulting training weights were then applied to the TMR data [− 0.5 to 1.5 s]. Classifier testing labels reflected the stimulus categories used in the retrieval sessions (left- or right-sided head orientation), such that above-chance classification hallmarks TMR-related activation patterns more strongly resembling the related stimulus category than the alternative stimulus category. As shown in Fig. 2b , results revealed significant above-chance classification from 930 to 1410 ms relative to TMR onset ( p = 0.023, corrected for multiple comparisons across time), emerging during the presence of sleep spindles (associative retrieval time-window: − 10 to 330 ms; the fact that decodability preceded the onset of the associative memory prompt again indicates that associative retrieval processes were probably ignited during the preceding recognition memory test; for cross-classification based on not-remembered retrieval trials see Supplementary Fig. 5 ). Applying the decoding procedure to source-space data revealed that these effects might have originated from frontoparietal networks and the right medial temporal lobe (including entorhinal cortex, parahippocampus and hippocampus; see Fig. 2c ).
Finally, we asked whether the oscillatory fingerprint of TMR in the SO-spindle range (Fig. 2a ) would be relevant for the identification of TMR-triggered memory reactivation. To address this question, we correlated, across participants, TMR triggered power as obtained by time-frequency representations (TFR; averaged across the cluster shown in Fig. 2a ) and levels of mean classification performance (averaged across the cluster shown in Fig. 2b ). As shown in Fig. 2d , we observed a significant positive relationship between the two variables (rho = 0.50, p = 0.01; for classification results based on TMR trials exhibiting increased levels of activity in the SO-spindle range see Supplementary Fig. 6 ).
Relationship between behavioral performance and memory reactivation
In an exploratory analysis (see Supplementary Fig. 7 ), we investigated the relationship of confidence ratings and memory reactivation in the scalp EEG study. We found a positive correlation between memory confidence in the pre-sleep memory test and the reactivation score (rho = 0.41; p = 0.038), which was also found when restricting the analysis to remembered and cued trials (rho = 0.43 p = 0.028; see Supplementary Fig. 7a ). Restricting the classification of head orientations to only high confidence trials showed significant above-chance decoding of retrieval-related brain patterns during NREM sleep ( p = 0.025, corrected for multiple comparisons across time; see Supplementary Fig. 7b ), a pattern highly similar to the one found when all trials were included (see Fig. 3b ). In contrast, when restricting the classification of head orientations to low-confidence trials, retrieval-related brain patterns (left vs. right head orientations) were not decodable (see Supplementary Fig. 7c ).
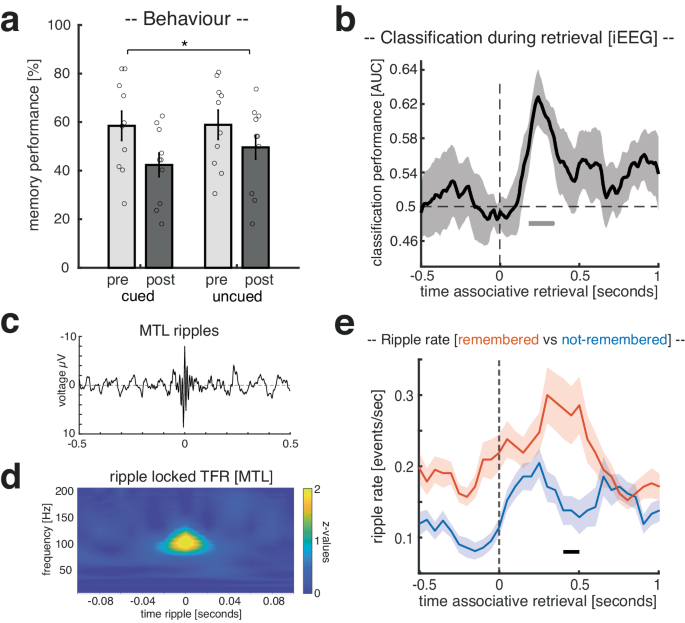
a Behavioral results for both experimental sessions pre- (light gray) and post-sleep (dark gray), separated into cued and uncued trials. Bar graphs show the mean ( ± SEM) percentage of recalled head orientations. Dots indicate individual memory performance of participants ( N = 10). The star denotes the significant interaction (pre vs. post x cued vs. uncued) as derived from a repeated measures ANOVA (F 1,9 = 8.28; p = 0.018). b Later cued head orientation (left vs. right) could be reliably decoded (above chance) from the retrieval data, starting around 190 ms after the onset of the associate prompt. The black solid line indicates decoding performance ( ± SEM across participants). The horizontal dashed line indicates chance level performance (i.e., 0.5). The vertical solid line indicates the onset of associative retrieval trials (time = 0). The lower horizontal gray line shows the temporal extent of significant decoding results as derived from two-sided cluster-based permutation test ( p = 0.019, corrected for multiple comparisons across time, N = 10). c Ripple-triggered grand average of all detected ripples (N contacts = 14; locked to maximal negative amplitude, ( ± SEM across MTL contacts) during retrieval (− 0.5 to 1.5 s). d Time-frequency-representations of ripple-locked MTL data segments; N contacts = 14). e Ripple rates (events per second) for remembered (red) and not-remembered (blue) trials. Ripple rates differed significantly between conditions ( p = 0.047; two-sided cluster-based permutation test corrected for multiple comparisons across time), with MTL ripples peaking during remembered trials (0.4 to 0.5 seconds in relation to the associative memory prompt onset; ± SEM across MTL contacts; N contacts = 14). Source data are provided as a Source Data file.
Results [intracranial EEG - patients]
Ten patients (age: 31.20 ± 3.46; 7 female) took part in the intracranial EEG (iEEG) study. Overall, the procedures of the experiment were highly similar to the above-described scalp EEG study but optimized for patients in a clinical setting (e.g., reduced trial number in the memory task, memory task was split into three consecutive blocks; see “Methods” for details).
First, we tested whether the effects of TMR on memory performance, as reported above, would replicate in the patient sample. Hence, we again tested for differences in memory performance between test times and TMR conditions by conducting an ANOVA for the cued recall (factors: cueing (cued vs. uncued) and test-time (pre- vs. post- sleep)). Results revealed that patients’ memory performance also declined over the course of sleep (main factor test-time: F 1,9 = 32.0; p < 0.001), comparable to the healthy participants’ decline. As in the healthy sample, we found a significant interaction between test time and cueing (F 1,9 = 8.28; p = 0.018), indicating that TMR did modulate memory performance by exerting a detrimental effect on retrieval abilities (cued pre-sleep: 58.47 ± 6.02% vs. cued post-sleep: 42.36 ± 4.89%; uncued pre-sleep: 58.88 ± 5.60% vs. uncued post-sleep: 49.58 ± 5.73%; see Fig. 3a ). While the post-hoc t test (relative change for cued vs. uncued) did not turn out to be significant (t 1,9 = 1.97; p = 0.08), we would still like to emphasize that the overall pattern of behavioral results is highly similar to those of the healthy population. For recognition memory, there was neither a significant main effect of test time (F 1,9 = 0.06; p = 0.08), nor a significant interaction between test-time and cueing (F 1,9 = 2.25; p = 0.16; see Supplementary Fig. 8 for details).
iEEG confirms reactivation of head orientation-related activity during successful retrieval
Next, we assessed whether the intracranial data would reveal evidence for the reactivation of head orientation-related activity during retrieval, similar to the results of the scalp EEG study (see Supplementary Fig. 9 for electrode coverage of intracranial EEG recordings). Again, the associative retrieval data was pooled across pre- and post-sleep sessions, and multivariate classification (LDA) was restricted to correctly remembered items whose associated head orientations were cued during sleep (i.e., one left sided and one right sided head orientation per patient). Using fivefold cross-validation (see “Methods”), significant above-chance classification accuracy emerged after the onset of the associative retrieval prompt (peak at 250 ms; p = 0.019, corrected for multiple comparisons across time, see Fig. 3b ; for classification results based on broadband high-gamma data see Supplementary Fig. 10 ; for results concerning the classification of encoding-related brain patterns during retrieval see Supplementary Fig. 11 ). Hence, similar to scalp EEG recordings, multivariate classification during retrieval using intracranial EEG activity allowed us to isolate brain patterns associated with the putative reactivation of head orientation-related activity.
Given the ascribed role of MTL ripples in memory retrieval 32 , 33 , 34 , 35 , 36 , 37 , we assessed whether ripple rates would differ between remembered and not-remembered trials. First, ripples were extracted (7 patients, 14 contacts) based on established criteria 18 (see “Methods” for details; see Fig. 3 c, d for ripples related EPRs and time-frequency representations; see Supplementary Fig. 12 for exemplary raster plots of retrieval-related ripples during remembered and not-remembered trials). Then peri-event histograms (bin size = 50 ms) of ripple events time-locked to the onset of the associative retrieval prompt were calculated, specifically for remembered and not-remembered trials. Ripple rates (events per second) differed significantly between conditions ( p = 0.047; corrected for multiple comparisons across time), with higher MTL ripple rates for remembered as compared to not-remembered trials (i.e., 400–500 ms after the onset of the associative retrieval prompt; for classification results based on ripple-triggered broadband high-gamma data see Supplementary Fig. 13 ). It should be noted that ripple rates during remembered and not-remembered trials descriptively differed already during the baseline window (−0.5 to 0 sec), with higher ripple rates for remembered trials. This non-significant difference is probably related to the fact that associative memory was always test right after old/new judgments, hence retrieval processes were not exclusively triggered by the associative memory prompt onset.
TMR triggered reactivation of head orientation-related activity is accompanied by elevated levels of SO-spindle and ripple activity
In the first step, we tested whether TMR-triggered power would also distinguish between learning related and control sounds using intracranial EEG recordings (based on frontal, parietal and temporal contacts). In line with the results of the scalp EEG study, learned TMR cues, as compared to control cues, elicited a significant power increase in the SO-spindle range (low-frequency cluster: p < 0.001; spindle cluster: p < 0.001; corrected for multiple comparisons across time and frequency, Fig. 4c ; for raw spectrograms of different TMR conditions see Supplementary Fig. 14 ; for spindle characteristics associated with experimental and control sounds see Supplementary Fig. 15 ).

a Ripple-triggered grand average of all detected ripples (N contacts = 14; locked to maximal negative amplitude) during TMR (− 0.5 to 1.5 s; 138.78 ± 21.72 ripples in 231.14 ± 19.94 trials). A zoomed version of the ripples is illustrated in the inset. b Power spectral density (PSD) averaged across all detected SWRs [± 300 ms] indicating distinct peaks in the SO/delta, spindle, and ripple range (i.e., 3 Hz, 14 Hz, and 84 Hz, ± SEM across MTL contacts; N contacts = 14). c Power difference indicates that retrieval-related TMR cues triggered increased power in intracranial EEG recordings ( p = 0.009, two-sided cluster-based permutation test corrected for multiple comparisons, N contacts = 317 as compared to control cues. d Ripple rates (events per second, ±SEM across MTL contacts) for trials exhibiting high (red) and low power (blue) in the SO-spindle range, respectively. Ripple rate differed significantly between conditions ( p = 0.027, two-sided cluster-based permutation test corrected for multiple comparisons across time, N contacts = 14), with MTL ripples peaking during elevated spindle activity. e Head orientation-related brain patterns (left vs. right) were decodable during TMR when contrasting high and low SO-spindle activity trials ( p = 0.019 two-sided cluster-based permutation test corrected for multiple comparisons; contour lines indicate the extent of the cluster, N = 10, color range (blue to yellow) represents t values against chance level performance). Source data are provided as a Source Data file.
SO-spindles have long been implicated in coordinating the emergence of hippocampal ripples and hippocampal–cortical interactions 17 , 21 , 22 , 24 , 38 . Hence, we next tested whether different levels of cortical SO-spindle activity would influence the emergence of ripples in the medial temporal lobe (MTL). Ripples were extracted in MTL contacts (7 patients, 14 contacts; see “Methods” for details; see Fig. 4a and b ; see Supplementary Fig. 16 for a comparison of ripple rates between NREM and REM sleep 39 ). Then, to investigate whether activity in the SO-spindle range would affect the emergence of ripples, we sorted TMR trials as a function of power in the TFR-related SO-spindle cluster (Fig. 4d ) and divided the trials using a median split (see Supplementary Fig. 17 for TFR differences between high and low SO-spindle activity trials). Next, we created peri-event histograms (bin size = 50 ms) of ripple events time-locked to TMR cues for trials exhibiting high and low activity in the SO-spindle range, respectively. As shown in Fig. 4d , ripple rates (events per second) differed significantly between conditions ( p = 0.027; corrected for multiple comparisons across time), with MTL ripples specifically peaking during elevated spindle activity (i.e., 1100–1250 ms after reminder cue onset; also see Supplementary Fig. 18 ; see Supplementary Fig. 19 for exemplary raster plots of TMR ripples during high and low SO-spindle power trials). However, the overall ripple number did not differ between high and low SO-spindle activity trials (high SO-spindle trials: 66.57 ± 10.57, low SO-spindle trials: 70.35 ± 10.64, t (13) = − 1.1, p = 0.28), indicating that SO-spindle activity coordinates the temporal occurrence of ripples rather than their overall number.
Given that the interaction between SO-spindles and ripples has been tightly linked to memory reactivation and the behavioral expressions of memory consolidation in rodents 40 , 41 , we determined whether TMR-triggered reactivation of head orientation-related activity would be specifically traceable in trials where the probability for SO-spindles and concomitant ripples would be high. Hence, a classifier was trained on the pooled associative retrieval data from both pre- and post-sleep sessions [− 0.5 to 1 s] and tested on the TMR data [− 0.5 to 1.5 s], separately for high SO-spindle activity trials and for low SO-spindle activity trials. The resultant classification performance outcomes were contrasted (see “Methods” for details). We found a cluster of significant classification from 960 to 1410 ms relative to TMR onset ( p = 0.019, corrected for multiple comparisons across time, retrieval time-window [− 150 to 200 ms]; Fig. 4e ; see Supplementary Fig. 20 for results of testing high- and low SO-spindle activity trials against chance-levels and classification results for all TMR segments irrespective of SO-spindle activity). These results indicate that (i) TMR-induced reactivation is related to remembered head orientations and that (ii) reactivation was putatively mediated by SO-spindle and ripple activity (for a localization of the cross-classification result see Supplementary Fig. 21 ). We examine the relation between ripples and memory reactivation in more depth in the next section.
Spindle-locked MTL ripples coincide with memory reactivation
Having established that cardinal sleep oscillations and reactivation of head orientation-related activity co-occur in time, we next assessed whether ripples and their coupling to spindles would be essential for triggering reactivation processes (for time-frequency representations and single trial examples of spindles and ripples see Fig. 5a, b ). First, we tested whether the phase of spindles in cortical contacts would impact ripple band activity in MTL contacts when ripples emerged during high spindle activity (i.e., 700–1400 ms after cue onset; for details see “Methods”) using the Modulation Index 42 . In line with previous findings, results revealed that the phase of sleep spindles robustly influenced the amplitude in the ripple range 17 , 21 (~ 80–120 Hz; p = 0.005, corrected for multiple comparisons across frequencies; see Fig. 5c ). The phase of cortical delta/theta activity also exhibited a significant effect on ripple activity 43 ( p = 0.007, corrected for multiple comparisons across frequencies), while spindle phases additionally modulated low gamma in the MTL (~ 20–40 Hz; p < 0.001, corrected for multiple comparisons across frequencies). When assessing the preferred phase of spindles for their grouping of ripples, we found that ripples were nested towards the trough of cortical spindles (Fig. 5c top inset; V test against ± \({{{\mathbf{\pi}}}}\) : V = 5.29, p = 0.022; mean coupling direction: − 176.67 ± 16.61°; mean vector length = 0.21 ± 0.031) 17 , 21 , while ripples generally tended to emerge towards spindle centers (see Fig. 5c bottom inset).
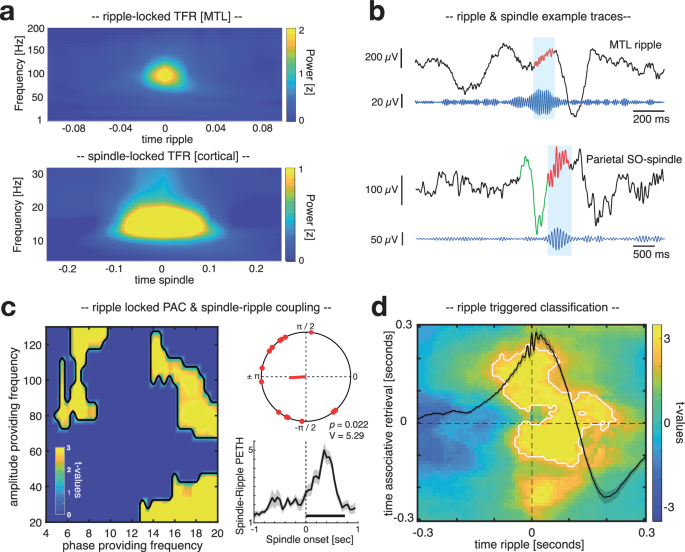
a Time-frequency-representations of ripple-locked MTL data segments (top; N contacts = 14) and spindle-locked data segments from frontal, parietal, and temporal contacts (bottom; N contacts = 317). ( b, top ) Unfiltered iEEG trace from single contact in the MTL with ripple band filtered signal (80 to 120 Hz) shown in blue. Blue shaded area highlights a representative ripple. ( b , bottom ) Unfiltered iEEG trace from single parietal contact with spindle band filtered signal (12 to 15 Hz) shown in blue. Blue shaded area highlights a representative spindle following a SO (green). c Assessing phase-amplitude coupling (PAC) using the Modulation Index revealed that the phase of cortical spindles influenced amplitudes in the ripple range in MTL contacts (~ 80–120 Hz; p = 0.005, two-sided cluster-based permutation test corrected for multiple comparisons, N contacts = 14). In addition, the cortical delta/theta phase exhibited a significant effect on MTL ripple amplitudes ( p = 0.007, two-sided cluster-based permutation test corrected for multiple comparisons, N contacts = 14), while the spindle phase additionally modulated low gamma amplitudes in the MTL ( ~ 20–40 Hz; p = 0.0009; two-sided cluster-based permutation test corrected for multiple comparisons, N contacts = 14). The top inset illustrates phases of the spindle-ripple modulation, indicating a clustering of ripples towards spindle troughs (corresponding to ±π; two-sided V test against ± pi: v = 5.29, p = 0.022 N contacts = 14; mean coupling direction: −176.67 ± 16.61°, mean vector length = 0.21 ± 0.031). The bottom inset illustrates the temporal modulation of MTL ripple onsets by cortical spindle onsets (ripple percentage in relation to spindles ± 1 sec; mean ± SEM across MTL contacts, N contacts = 14). A solid horizontal line indicates mean spindle duration (mean spindle duration: 0.75 ± 0.008 sec; peak latency: 0.37 ± 0.042 sec in relation to spindle onsets). (d) Head orientation-related brain patterns (left vs. right) were decodable during the presence of spindle-locked MTL ripples ( p = 0.007, two-sided cluster-based permutation test corrected for multiple comparisons, N = 7; color range (blue to yellow) represents t values). Source data are provided as a Source Data file.
Finally, we asked whether spindle-locked ripples would be relevant for the identification of memory reactivation. Hence, again a classifier was trained on the pooled associative retrieval data from both pre- and post-sleep sessions [− 0.5 to 1 s], but the resulting training weights were this time specifically applied on intracranial EEG segments centered around spindle-locked MTL ripples (i.e., where MTL ripples were paralleled by cortical spindles in between 700 and 1400 ms). For statistical evaluation, surrogate decoding performance was calculated by centering intracranial EEG segments around time points where no ripple was present during the time window of preferred spindle-ripple interactions (i.e., 700–1400 ms after cue onset). This procedure was repeated 100 times and resulting surrogate performance values were then averaged, providing baseline values for each participant under the null hypothesis that spindle-locked ripples would not be relevant for the classification of stimulus categories. We found a ripple - locked cluster of significant above-chance classification from – 100 to 200 ms relative to ripple centers, indicating that ripples might indeed be associated with memory reactivation during NREM sleep in humans ( p = 0.007, corrected for multiple comparisons across time, associative retrieval time-window [− 120 to 230 ms], Fig. 4b ; see Supplementary Fig. 22 for contrasting ripple triggered classification against chance-level; see Supplementary Fig. 23 for results indicating that uncoupled ripples (i.e., ripples without spindles) did not facilitate multivariate classification).
Our results unveil a key role of spindle-locked ripples in human sleep-based memory reactivation. Specifically, we found that ripples in the MTL, when coupled to cortical spindles, are tightly related to the reprocessing of memories during human NREM sleep, as evidenced by the multivariate classification of prior retrieved head orientations. These findings elucidate the neural processes mediating memory reactivation during human NREM sleep, by establishing MTL ripples and their synchronization with cortical sleep rhythms as crucial cornerstones.
In current models of memory consolidation, ripples are generally considered to be electrophysiological markers of memory reactivation, as they have been suggested to trigger retrieval-related memory reactivation 44 , 45 and the reprocessing of memories during sleep 1 , 20 , 46 . To date, however, even though the role of ripples in memory retrieval has been established in humans 32 , 33 , 34 , 35 , 36 , 37 , direct evidence for a core contribution of ripples to sleep’s memory function has been lacking in humans. We here used multivariate classification to detect human reactivation processes that are timed by ripples identified in the MTL, providing strong support that ripples in humans initiate memory reactivation akin to animal models 6 , 16 , 47 and presumably facilitate the hippocampo-cortical dialog. Interestingly, TMR studies in rodents have not only shown that auditory cues induce memory reactivation in the hippocampus 48 , 49 , but that cortical activity elicited by memory-related cues, predicts hippocampal ripple activity, which in turn biases post-ripple activity in cortical areas, suggesting a loop-like information flow around ripple occurrence 48 , 50 . Our result that ripples in the human MTL were tightly associated with TMR-elicited memory reactivation is in line with the notion that auditory TMR might facilitate the generation of memory-related ripples by biasing hippocampal activity. However, our current data remain agnostic as to whether a cortical-hippocampal-cortical loop of information exchange underlies sleep-related memory consolidation.
One intriguing question is whether all ripples are related to memory reactivation. Our data suggest that, while the ripple rate distinctly peaked during successful remembering in an early time window, only a fraction of NREM ripples, specifically those coupled to cortical spindles, were associated with the decodability of prior retrieved head-orientations (Fig. 4b and Supplementary Fig. 22 ). Spindles are well known to group ripples in the MTL 19 , 21 , 22 , 23 (Fig. 4a ). They have also been shown to induce neural plasticity in cortical target sites 13 , 14 , 51 , ensuring that those areas are optimally tuned for long-term storage when reactivated memory information arrives 1 . Hence, our finding that spindle-locked ripples were key for detecting memory reactivation confirms longstanding theoretical predictions concerning the role of synchronized spindle-ripple activity in this context 20 , 46 , 52 .
Moreover, we show that elevated levels of SO-spindle activity promoted the read-out of prior learned stimulus categories in both scalp and intracranial EEG recordings. The precise interplay between SOs and spindles is believed to regulate the flow of information between the hippocampus and cortical long-term stores, with SO up-states establishing a time window for spindles and ripples to coincide 22 . In addition, earlier studies in healthy participants using scalp EEG established SO-spindles as a necessary pre-requisite for the identification of memory reactivation 18 , 53 , 54 . However, because the poorly conducting skull low-pass filters the scalp EEG 55 , these data remained agnostic to the role of high-frequency signals such as ripples and their potential role in memory reactivation. Our results indicate that ripples in the MTL peaked during the presence of spindles in trials exhibiting high SO-spindle activity (Fig. 3e ), with memory reactivation accompanying those spindle-locked ripples (Fig. 4b ). This might suggest that also in previous studies ripples, without being detected due to methodological reasons, were tightly related to the decodability of prior learned material during SO-spindles.
In the present paradigm, real-world head orientation acted as spatial context in an episodic memory task. By showing that real-world head orientation-related activity is reactivated during successful retrieval and sleep our findings add ecological validity to prior work on the reactivation of memory contexts 56 , 57 , 58 . These findings are important because they indicate that the neural correlates of memory functions generalize from screen-based laboratory settings to more naturalistic behavior incorporating bodily movements 59 . The standard approach to studying the neural basis of human memory requires participants to display minimal bodily movements (e.g., fMRI, MEG), preventing the generation of many self-referential cues, which are thought to play a crucial role in the neural mechanisms underlying memory 59 , 60 . The present approach circumvents these shortcomings by incorporating real-world head rotations that trigger self-referential cues such as motor commands, efference copies, and reafferent feedback. Combining this approach with rare intracranial recordings from core memory regions (e.g., MTL) opens up exciting opportunities to investigate human electrophysiology that would otherwise remain concealed 59 , 61 , 62 .
On a neural level, little is known about how the human brain tracks and maintains information about real-world head orientation but see 62 . Animal research, on the other hand, has successfully identified neurons that act as a neural compass during spatial navigation 63 , 64 , 65 , 66 . During sleep, this neural compass seems to be preserved 67 , 68 . By simultaneously recording hippocampal ripples and activity from thalamic head direction cells in rodents, Viejo & Peyrache 69 showed a specific coupling of the two signals in sleeping rodents that might guide the replay of previously experienced trajectories (even though memory reactivation was not explicitly assessed in their study) 69 . Our results demonstrating ripple-locked memory reactivation connect to these findings on a conceptual level, by showing that ripples are related with the reactivation of memory contexts (i.e., head orientations) that might guide the reactivation of previously experienced events. Going beyond previous work in animal models, we here show that head orientation acts as a memory context in an episodic memory task. Note, however, that the here presented intracranial and surface EEG operate on a meso-/macro scale, compared to the micro-scale of single unit recordings. While recent studies have identified a population-level code for real-world head direction 62 , 70 , 71 , future work is necessary to connect the different levels, see e.g., 72 . On a more general level, implementing real-world navigation into memory paradigms is challenging, but at the same time promises to build bridges between animal research investigating real-world spatial navigation and studies investigating memory processes in humans. Assuming that memory and navigation share neural mechanisms, converging experimental approaches could ultimately foster our understanding of the underlying neural codes in animals and humans 73 .
We here used TMR as an experimental tool to trigger memory reactivation during sleep. It has been shown that TMR modulates memory, leading most often to performance increases 28 , 74 , 75 , 76 , 77 , 78 . Hence, it might seem surprising that TMR did not benefit but deteriorated memory performance both in healthy participants as well as in patients. However, a growing number of studies report TMR-induced impairments, in particular when several targets were associated with a given TMR cue 30 , 79 , 80 . In the present study, multiple images (42 in the scalp EEG study; 36 in the intracranial EEG study) were associated with each of the four head orientations. It has been suggested that if the associations between multiple targets and one cue vary in strength, TMR might elicit the reactivation of targets most strongly associated with the cue 79 , akin to models describing retrieval competition during wake 81 , 82 . Selectively strengthening a subset of strong cue-target associations via TMR, however, might lead to weakly associated targets losing the competition for being reactivated during a subsequent memory test. Depending on the relative amount of cue-target associations in either subset, this might show as a net beneficial, detrimental, or no effect of TMR on memory performance. Interestingly, in an exploratory analysis (see Supplementary Fig. 7 ), we found a positive correlation between memory confidence in the pre-sleep memory test and the effect of TMR in the scalp EEG study (due to time constraints no confidence rating was obtained in the iEEG study). Assuming that confidence ratings in our study are positively related to memory strength 83 , 84 , but see 85 , this relationship indicates that TMR may have mainly reactivated targets that were strongly associated with their cues and further strengthened their association. In turn, these strong targets might have outcompeted weaker ones when competing for being retrieved during post-sleep retrieval, resulting in the observed detrimental effect of TMR on memory performance.
In sum, several lines of argument support the assumption that our classification procedure indeed picked up neural signatures of memory reactivation, despite the unexpected direction of the TMR effect on memory performance. First, TMR did have a robust effect on behavior, both across participants and independent studies (scalp and iEEG), indicating a systematic influence on neural processes during NREM sleep. Second, as outlined in detail above, a growing number of studies reported similar detrimental effects on behavior when several targets were associated with a given TMR cue 30 , 79 , 80 , 86 . Third, multivariate analysis methods (such as MVPA 87 or representational similarity analysis 88 ) have been proven valuable tools to identify memory reactivation during both wakefulness and sleep 5 , 78 . Our cross-classification results, indicating that prior learned stimulus categories were decodable during the presence of sleep spindles, are in line with previous work reporting TMR-induced or endogenous memory reactivation locked to spindles (e.g., 53 , 89 , 90 , 91 ). This consistency in terms of the neural conditions (i.e., the presence of sleep spindles) favoring decodability is also found with studies where TMR exhibited beneficial effects on behavior 90 , 91 , which further supports the notion that our TMR procedure indeed induced memory reactivation, but with opposite consequences on behavior. Finally, the obtained cross-classification results were highly specific with regards to retrieval processes. Retrieval-related neural patterns of head orientations could only be successfully classified during TMR in case of correctly remembered head orientations (see Supplementary Fig. 5 for cross-classification based on not-remembered trials), while decodable time windows with regards to the retrieval data highly overlapped between the cross-classification and within retrieval classification procedures.
To conclude, using invasive and non-invasive human electrophysiology we found an intimate relationship between NREM sleep-related oscillations and memory reactivation. Our findings provide evidence in favor of current models of systems-level consolidation in humans, where spindle-locked ripples synchronize neural population dynamics to reactivate previously formed memories. They establish MTL ripples and their synchronization with cortical sleep rhythms as crucial cornerstones of memory consolidation in humans.
Participants
25 healthy, right-handed participants (mean age: 25.2 ± 0.6; 16 female) with normal or corrected-to-normal vision took part in the EEG experiment. An additional 14 participants had to be excluded due to insufficient sleep or technical problems. The sample size was determined in accordance with previous human sleep and memory studies (e.g., 90 , 91 , 92 ). Pre-study screening questionnaires (including the Pittsburgh Sleep Quality Index (PSQI 93 ), the morningness–eveningness questionnaire 94 , and a self-developed questionnaire querying general health status and the use of stimulants) indicated that participants did not take any medication at the time of the experimental session and did not suffer from any neurological or psychiatric disorders. All participants reported good overall sleep quality. Furthermore, they had not been on a night shift for at least 8 weeks before the experiment. All participants were instructed to wake up by 7 a.m. and avoid alcohol the evening before and caffeine on the day of the experimental sessions. They received course credit or financial reimbursement in return for their participation. All participants gave written informed consent after being informed about the details of the study. The study was approved by the ethics committee of the Department of Psychology (Ludwig–Maximilians-Universität München).
For the intracranial EEG study, 10 inpatients from the Epilepsy Center, Department of Neurology, Ludwig–Maximilian Universität (Munich, Germany), all suffering from medically intractable epilepsy, volunteered (7 female; age: 31.20 ± 3.46). An additional four patients had to be excluded due to technical difficulties. The study was approved by the ethics committee of the Medical Faculty of the Ludwig–Maximilians-Universität München.
Stimuli and procedures
On experimental days participants arrived at the sleep laboratory at 7 p.m. The experimental session started with the set-up for polysomnographic recordings during which electrodes for electroencephalographic (EEG) and electrooculography (EOG) were applied. Several days before the experimental session, participants were habituated to the environment by having an adaptation nap in the sleep laboratory. At around 8 p.m. the experiment started with a training task, followed by the memory task (for details see Training and Memory Task below). The sleep period began at ~ 11 p.m. and all participants slept for ~ 7 hours (for sleep characteristics see Supplementary Tables 1 ). During NREM sleep (sleep stages N2 and SWS), some of the animal sounds, which were presented before during the training and the memory task were presented again for 60 min (see Targeted memory reactivation for details). Participants were awoken after ~7 hours of sleep from light sleep (sleep stage N1 or N2) and after 15 min of recovery, memory performance was tested again (see Supplementary Table 1 for sleep characteristics).
For the intracranial EEG study, the general approach was largely similar. Experimental procedures were arranged around the clinical routines. Specifically, the training sessions were executed during the daytime, while the memory task was employed after dinner (i.e., starting between ~ 6-7 pm). Patients went to sleep between 10 and 12 p.m. and slept for ~ 7 hours (for sleep characteristics see Supplementary Tables 2 ). As with the EEG study, animal sounds were presented for 60 min during NREM sleep. Post-sleep memory performance was tested the next morning (see Supplementary Table 2 for sleep characteristics).
A set of in total 336 images of objects and five animal sounds (i.e., a cow’s moo, a parrot’s squawk, a cat’s meow, a sheep’s baa, a cuckoo’s sound) served as experimental stimuli. Objects were images of animals, food, clothing, tools, or household items presented on a plain white background. All images were taken from 95 .
Experimental tasks
For the recording of behavioral responses and the presentation of all experimental tasks, Psychophysics Toolbox Version 3 96 and MATLAB 2018b (MathWorks, Natick, USA) were used. Responses were made via keyboard presses on a dedicated PC. Across all experimental phases, the presentation order of stimuli was randomized across participants/patients.
Participants/patients began by fixating on the center screen (screen size: 28 inch), where a fixation cross was presented for 1.5 ± 0.1 s. The screen to eye distance was ~ 1 meter for the scalp EEG study and ~ 1.20 meter for the iEEG study. The cross disappeared and one of four animal sounds was played (600 ms). Subsequently, the cross appeared on one of four flanking screens (positioned at − 60°, − 30°, + 30° and +60° relative to the center screen, duration: 2.5 s, see Fig. 1a ; the size of flanker screens: 22 inch). Four of the total five sounds were randomly chosen at the start of the experiment and randomly assigned to a single flanking screen. The assignment of sound to screen remained fixed across the whole experiment. Participants/patients were instructed to turn their heads to face the screen which the fixation cross appeared on and maintain fixation upon the cross (duration: 2.5 s). Afterward, the fixation cross re-appeared on the center screen for 1.5 ( ± 0.1) seconds and participants/patients had to bring their head back to the starting (i.e., central) position. The training session consisted of 160 trials, split across 4 blocks (i.e., 40 trials per block). The aim of the session was enabling participants/patients to form strong and stable associations between the sound cues and the corresponding head orientations (i.e., flanking screens).
Memory task [EEG study]
Participants in the EEG study learned to associate 168 images of objects with specific head orientations. Each trial started with a fixation cross, presented for 1.5 ± 0.1 s. Afterward, one of the four animal sounds from the training phase was played (duration 600 ms). Subsequently, an image of an object was presented on the corresponding flanking screen for 4 s (the assignment of sound to screen was known to the participants from the training). Participants were instructed to turn their heads to face the screen which the image appeared on and to remember the images and their position. Afterwards, the participants had to indicate via button press whether the previously seen object was animate or inanimate, with the question being presented on the center screen. The pre-sleep memory test included the 168 images from encoding (old items) intermixed with 84 new images, which were not seen by the participants before (“foils”). Each trial started with a fixation cross, presented for 1.5 ± 0.1 s. After the fixation cross, an image was presented on the center screen. After 1 second, recognition memory was assessed. Participants were asked to indicate whether the image was “old” (i.e., part of the learning material) or “new”’ (i.e., it was not seen during learning) within the next 10 s. In case of “new” responses, participants immediately moved on to the next trial. In the case of “old” responses, participants were required to indicate by button press the related head orientation (i.e., the flanking screen on which the image was presented; for head-movement related data during retrieval see Supplementary Fig. 24 ). Each trial ended with participants indicating how confident they were with their head orientation decision (scale from 0 corresponding to very uncertain to 4, very certain). The post-sleep retrieval followed the same procedures as the pre-sleep memory test with the exception that new foil images were used.
Memory task [intracranial EEG study]
The procedures of the memory task were similar to the EEG study, with some modifications. The stimulus pool comprised 288 objects (drawn from the same selection as used in the EEG study). In order not to overtax patients, the pre-sleep memory task was split into three consecutive encoding - retrieval blocks. During each encoding block patients were presented with 48 images on the flanking screens (please note that the trial level was identical to the one described above). Each encoding block was followed by a retrieval block, where the 48 images from encoding intermixed with 24 new images were presented. Hence, across all blocks, we used 144 images as old items and 72 images as new items. As above, patients had to first indicate whether a given image was old or new and in the case of old items specify the remembered head orientation. Due to time constraints, no confidence rating was obtained. The post-sleep retrieval was executed in one run, meaning that patients were confronted with the 144 images which were part of the learning material and 72 foils.
Targeted memory reactivation
For targeted memory reactivation 2 out of the 4 sounds presented during training and encoding were selected. Specifically, we randomly picked one out of the two sounds associated with the left-sided head orientations (i.e., flanking screens positioned at − 60° and − 30°) and one sound associated with the right-sided head orientations (i.e., flanking screens positioned at +30° and +60°). In addition, the fifth animal sound which was not used during training and encoding served as a control stimulus. The three cues were repeatedly presented during NREM sleep via loudspeaker with an intertrial interval of 5.5 ± 0.2 seconds (~ 50 dB sound pressure level) for a maximum of 60 minutes (EEG study: 182.6 ± 31.41 repetitions per stimulus; intracranial EEG study: 187.1 ± 23.7 repetitions per stimulus). Sound presentation was stopped whenever signs of arousals, awakenings, or REM sleep became visible.
Scalp EEG acquisition
An EEGo 65 channel EEG system (ANT Neuro Enschede, Netherlands) was used to record electro- encephalography (EEG) throughout the experiment. Impedances were kept below 20 kΩ. EEG signals were referenced online to electrode CPz and sampled at a rate of 1000 Hz. Furthermore, horizontal and vertical EOG was recorded for polysomnography. Sleep architecture was determined offline according to standard criteria by two independent raters 97 .
Intracranial EEG acquisition
Intracranial EEG was recorded from Spencer depth electrodes (Ad-Tech Medical Instrument, Racine, Wisconsin, United States) with 4–12 contacts each, 5 mm apart. Data were recorded using XLTEK Neuroworks software (Natus Medical, San Carlos, California, US) and an XLTEK EMU128FS amplifier, with voltages referenced to a parietal electrode site. The sampling rate was set at 1000 Hz.
EEG data analysis
EEG data were preprocessed using the FieldTrip toolbox for EEG/MEG analysis 98 . All data were down-sampled to 200 Hz. Subsequently, the pre- and post-sleep retrieval as well as the TMR data were segmented into epochs. For the retrieval data, we segmented data from the onset of the associative retrieval. We reasoned that memory reactivation of associated head orientations should be particularly strong due to the potential hippocampal dependency (as compared to recognition tests 99 ). The temporal range of the epochs was [– 1 to 3 s] around stimulus onset for retrieval and TMR trials. Noisy EEG channels were identified by visual inspection, discarded, and interpolated, using a weighted average of the neighboring channels. The data were visually inspected and artefactual trials were removed. The retrieval data were additionally subjected to an independent component analysis (ICA) and ICA components associated with eye blinks and eye movements were identified and rejected (for ERPs related to left and right stimulus categories for retrieval and TMR see Supplementary Fig. 25 ).
intracranial EEG data analysis
The preprocessing steps for the intracranial EEG data were identical to the ones described above, just that the original sampling of 1000 Hz was preserved. Intracranial EEG data were additionally inspected for epileptic activity, with data segments comprising epileptic events at any given contact being discarded (26.71 ± 5.24 % of all TMR trials (N allTrials = 561.30 ± 22.49); 29.03 ± 4.68 % of all retrieval trials (N allTrials = 256.8 ± 7.24)); for interictal epileptiform discharge triggered classification see Supplementary Fig. 26 ). In addition, contacts which were contaminated with epileptiform background activity were discarded. Only seizure-free nights were included in the analysis.
Source level
To estimate the sources of the obtained effects in the scalp EEG study, we applied a DICS beamforming method 100 , as implemented in FieldTrip 98 . A spatial filter for each specified location (each grid point; 10 mm 3 grid) was computed based on the cross - spectral density, calculated separately for all retrieval and TMR trials. Electrode locations for the 65 - channel EEGo EEG system were co-registered to the surface of a standard MRI template in MNI (Montreal Neurological Institute) space using the nasion and the left and right preauricular as fiducial landmarks. A standard leadfield was computed using the standard boundary element model 51 . The forward model was created using a common dipole grid (10 mm 3 grid) of the gray matter volume (derived from the anatomical automatic labeling atlas 101 in MNI space, warped onto a standard MRI template, leading to 1457 virtual sensors. Data analysis was accomplished in the same way as on the sensor level.
Time–frequency analysis
Time–frequency analysis of the TMR segments (memory-related and control cues) was performed using FieldTrip. Frequency decomposition of the data, using Fourier analysis based on sliding time windows (moving forward in 50 ms increments). The window length was set to five cycles of a given frequency (frequency range: 1–25 Hz in 1 Hz steps). The windowed data segments were multiplied with a Hanning taper before Fourier analysis. Afterward, power values were z-scored across time [− 1 to 3 s]. The longer time segments were chosen to allow for resolving low-frequency activity within the time windows of interest [− 0.5 to 1.5 s] and avoid edge artifacts. For intracranial EEG data frontal, parietal, and temporal contacts were taken into account.
Multivariate analysis
Multivariate classification of single-trial EEG data was performed using MVPA-Light, a MATLAB-based toolbox for multivariate pattern analysis 102 . For all multivariate analyses, a LDA was used as a classifier. Before classification, data in both studies were re-referenced using a common average reference (CAR).
For classification within the retrieval task, the retrieval data were z-scored across all trials for each time point separately. Next, data from the pre- and the post-sleep retrieval were collapsed and subjected to a principal component analysis (PCA), which transforms the data into linearly uncorrelated components, ordered by the amount of variance explained by each component 103 . PCA was applied to reduce dimensionality and limit over-fitting (PCA) and the first 30 principal components were retained for further analysis (explaining 96.83 ± 0.2 % of the variance in the scalp EEG study and 97.60 ± 0.17 % in the iEEG study). To quantify whether remembered head orientations can be differentiated during retrieval, the classifier was trained and tested to discriminate between the later cued head orientations (i.e., one left sided and one right sided head orientation; see Targeted Memory reactivation for details). Only trials belonging to remembered head orientations entered the analysis. Data were smoothed using a running average window of 150 ms. The EEG channels / intracranial EEG contacts served as features and a different classifier was trained and tested on every time point. As metric, we used Area Under the ROC Curve (AUC), which indexes the mean accuracy with which a randomly chosen pair of Class A and Class B trials could be assigned to their correct classes (0.5 = random performance; 1.0 = perfect performance). To avoid overfitting, data were split into training and test sets using fivefold cross-validation 104 . Since cross-validation results are stochastic due to the random assignment of trials into folds, the analysis was repeated five times and results were averaged. For statistical evaluation, the classification output was tested against chance levels (i.e., 0.5). To resolve the topography of diagnostic features in the scalp EEG data, we conducted a “searchlight decoding procedure” (Fig. 2c ). In brief, PCA components were projected back to sensor space and the classification procedure was repeated across moving kernels of small electrode clusters, with neighboring electrodes being selected as features [feature number range: 5–9]. Finally, classification values were collapsed across our time windows of interest [retrieval time: − 30 to 680 ms;] and tested against chance level (corrected for multiple comparisons across space).
To investigate whether TMR would elicit head orientation-related activity, we used the temporal generalization method 105 . Before decoding, a baseline correction was applied based on the whole trial for retrieval and TMR segments [− 0.5 to 3 s]. Next, retrieval and TMR data were z-scored across trials and collapsed. PCA was applied to the pooled retrieval-TMR data and the first 30 principal components were retained. Retrieval and TMR data were smoothed using a running average window of 150 ms. A classifier was then trained for every time point in the retrieval data and applied on every time point during TMR. No cross-validation was required since retrieval and TMR datasets were independent. As metric, we again used AUC (see above). For statistical evaluation, the classification output was tested against chance levels (i.e., 0.5).
Given that the interaction between SO-spindles and ripples has been tightly linked to memory reactivation 40 , 41 , we determined whether TMR-triggered reactivation of head orientation activity would be traceable in intracranial EEG recordings, specifically in trials where the probability for SO-spindles and concomitant ripples would be high: for each participant, we sorted the TMR trials as a function of power in the clusters obtained in the time-frequency analysis (Fig. 4c ) and divided the trials using a median split. Then, a classifier was trained on the concatenated retrieval data from both pre- and post-sleep sessions [− 0.5 to 1 s], and the resulting training weights were applied to the TMR data [− 0.5 to 1.5 s], either comprising high SO-spindle power trials (i.e., where ripples peaked during spindle activity) or low SO-spindle power trials and contrasted the resultant performance outcomes. For statistical evaluation, the classification performance of both categories was directly compared.
For ripple triggered classification, a classifier was trained on the concatenated retrieval data from both pre- and post-sleep sessions [− 0.5 to 1 s], but the resulting training weights were applied on intracranial EEG segments centered around spindle-locked MTL ripples (i.e., ripples occurring during spindles between 700 to 1400 ms after cue onset). For statistical evaluation, surrogate decoding performance was calculated by centering intracranial EEG segments around time points where no ripple was present during the time window of preferred spindle-ripple interactions (i.e., 700 to 1400 ms after cue onset). This procedure was repeated 100 times and resulting surrogate performance values were then averaged, providing baseline values for each participant under the null hypothesis that spindle-locked ripples would not be relevant for the classification of stimulus categories.
For the scalp EEG data, head orientations were decoded in source space using searchlight analysis 106 . A sphere of radius 2 cm was centered on each of the 1467 voxels in the brain. All voxels within the sphere that were inside the brain volume (10-26 voxels) were selected as features. Identical to the sensor level analysis a classifier was trained for every time point in the retrieval data and applied on every time point during TMR. Finally, classification values were collapsed across our time windows of interest [retrieval time: − 110 to 330 ms] and tested against chance level (corrected for multiple comparisons across space).
Ripple detection
Ripple events in the medial temporal lobe (MTL) depth recordings (7 patients, 14 contacts in total: 4 hippocampal, 7 parahippocampal, and 3 entorhinal contacts) were detected during artifact-free retrieval and TMR segments using offline algorithms 18 , 107 . The intracranial EEG signal (sampling rate 1000 Hz) was band-pass filtered from 80 to 120 Hz and the root mean square signal (RMS) was calculated based on a 20 ms windows followed by an additional smoothing with the same window length. A ripple event was identified whenever the smoothed RMS-signal exceed a threshold, defined by the mean plus 2 times the standard deviation of the RMS-signal across all TMR data points. Potential ripple events shorter than 25 ms or longer than 300 ms were rejected. All ripple events were required to exhibit a minimum of three cycles in the raw signal (for cross-classification based on raw, non artifact-rejected data, where ripples were detected using a threshold of 3 SD, see Supplementary Fig. 27 ).
Peri-event histograms of ripple occurrence
For retrieval trials, peri-event histograms (bin size = 50 ms) of ripple events (per second) time-locked to the associative memory prompt onset were computed for remembered and not-remembered trials. To investigate the timing of MTL ripples (centered at the maximal negative amplitude) with regards to TMR cues, we first sorted for each participant the TMR trials as a function of power in the clusters obtained in the time-frequency analysis (Fig. 4c ) and divided the trials using a median split. We then created for each condition peri-event histograms (bin size = 50 ms) of ripple events (per second) time-locked to TMR cues.
Modulation Index
Phase amplitude coupling was assessed with the Modulation Index (MI) 42 . We first isolated in each patient the cortical contact exhibiting the strongest power in the spindle band (12–15 Hz; 0 – 1.5 seconds after cues onset; see Supplementary Table 3 for an overview). All intracranial EEG data segments were centered in relation to MTL-detected ripple maxima, focusing on ripples during high spindle activity (i.e., ripples emerging in a time window from 700 to 1400 ms after cue onset). Low frequencies in cortical contacts (4 – 20 Hz) were filtered with a window of 0.3 times the frequency of interest, centered on each frequency step. High frequencies in MTL contacts (20 – 130 Hz) were filtered with a window of 0.7 times the frequency of interest. To compute the MI (for a given frequency pair), we divided the phase signal into 18 bins (20° each), and then, computed for each bin the mean amplitude. This yielded a distribution of amplitude as a function of phase. The MI is defined as the Kullback-Leibler distance between that distribution and the uniform distribution (over the same number of bins). To assess the statistical significance of the MI values, we randomly shuffled the trials of the amplitude providing contacts and computed the MI using the shuffled data. We repeated this procedure 100 times, resulting in a MI-level reference distribution.
Spindle-ripple coupling
For the analysis of the coupling between cortical spindles and MTL ripples, we first isolated in each patient the cortical contact exhibiting the strongest power in the spindle band (12–15 Hz; 0 – 1.5 seconds after cues onset). We then filtered the data (12–15 Hz, two-pass Butterworth bandpass filter) and applied a Hilbert transform. The instantaneous phase angle of cortical recordings at the time of MTL detected ripple maxima was extracted. We specifically focused on ripples which occurred during high spindle activity (i.e., ripples emerging in a time window from 700 to 1400 ms after cue onset). The preferred phase of spindle-ripple coupling for each cortical contact was then obtained by taking the circular mean of all individual events’ preferred phases.
Behavioral retrieval data were subjected to a 2 (TMR: cued/uncued) × 2 (Test-Time: Pre-sleep/Post-sleep) repeated measures ANOVA. The statistical significance thresholds for all behavioral analyses were set at p < .05. FieldTrip’s cluster-based permutation test 98 was used to deal with the multiple comparisons problem for all classification analyses. A dependent-samples t-test was used at the sample level to identify clusters of contiguous time points across participants and values were thresholded at p = 0.05. Monte Carlo simulations were used to calculate the cluster p value (alpha = 0.05, two-tailed) under the permutation distribution. Analyses were performed at the group level. The input data were either classification values across time (Figs. 1 c– 3b ) or time x time classification values (Figs. 2 b– 3c ). In all cases a two-sided cluster-based permutation test with 1000 randomizations was used to contrast classification accuracy against chance performance (except for Supplementary Fig. 2 , where a one-sided cluster permutation test was used). The same statistical rationale was implemented for the statistical assessment of time-frequency data with time × frequency values as input, as well as for phase-amplitude data (frequency x frequency as input) and peri-event histograms (time as input). Statistical analysis of TFR data in the intracranial EEG study was performed at the individual electrode/contact level (fixed-effects analysis), considering all intracranial EEG contacts ( N = 390; see Supplementary Fig. 8 for coverage), while statistical analysis of phase-amplitude and peri-event histogram data considered all possible cortical-MTL contact pairs ( N = 14, fixed effects; with chosen cortical contacts showing the strongest spindle power). Pearson correlation was used to assess the relationship between (i) classification performance and time-frequency power (Fig. 2d ). For circular statistics (Fig. 5c ), the phase distributions across all cortical-MTL contact pairs ( N = 14) were tested against uniformity with a specified mean direction (i.e., ± π corresponding to the spindle through) using the V test (CircStat toolbox 108 ).
Reporting summary
Further information on research design is available in the Nature Portfolio Reporting Summary linked to this article.
Data availability
Data acquired from the healthy participants are available at Ludwig- Maximilians-Universität via https://data.ub.uni-muenchen.de/487/ 109 . Due to privacy laws, data acquired from the patients are not openly available, though (subject to privacy laws) can be provided by contacting the corresponding author. Source data are provided with this paper.
Code availability
Openly available at Ludwig- Maximilians-Universität via https://data.ub.uni-muenchen.de/487/ 109 .
Klinzing, J. G., Niethard, N. & Born, J. Mechanisms of systems memory consolidation during sleep. Nat. Neurosci. 22 , 1598–1610 (2019).
Article CAS PubMed Google Scholar
Frankland, P. W. & Bontempi, B. The organization of recent and remote memories. Nat. Rev. Neurosci. 6 , 119–130 (2005).
Dudai, Y., Karni, A. & Born, J. The Consolidation and Transformation of Memory. Neuron 88 , 20–32 (2015).
Navarrete, M., Valderrama, M. & Lewis, P. A. The role of slow-wave sleep rhythms in the cortical-hippocampal loop for memory consolidation. Curr. Opin. Behav. Sci. 32 , 102–110 (2020).
Article Google Scholar
Schreiner, T. & Staudigl, T. Electrophysiological signatures of memory reactivation in humans. Philos. Trans. R. Soc. Lond. B Biol. Sci. 375 , 20190293 (2020).
Article PubMed PubMed Central Google Scholar
Girardeau, G. & Lopes-Dos-Santos, V. Brain neural patterns and the memory function of sleep. Science 374 , 560–564 (2021).
Article ADS CAS PubMed PubMed Central Google Scholar
Timofeev, I. Neuronal plasticity and thalamocortical sleep and waking oscillations. Prog. Brain Res. 193 , 121–144 (2011).
Fernandez, L. M. J. & Lüthi, A. Sleep Spindles: Mechanisms and Functions. Physiol. Rev. 100 , 805–868 (2020).
Article PubMed Google Scholar
Isomura, Y. et al. Integration and segregation of activity in entorhinal-hippocampal subregions by neocortical slow oscillations. Neuron 52 , 871–882 (2006).
Schreiner, T., Kaufmann, E., Noachtar, S., Mehrkens, J.-H. & Staudigl, T. The human thalamus orchestrates neocortical oscillations during NREM sleep. Nat. Commun. 13 , 5231 (2022).
Mak-McCully, R. A. et al. Coordination of cortical and thalamic activity during non-REM sleep in humans. Nat. Commun. 8 , 15499 (2017).
Rosanova, M. & Ulrich, D. Pattern-specific associative long-term potentiation induced by a sleep spindle-related spike train. J. Neurosci. 25 , 9398–9405 (2005).
Article CAS PubMed PubMed Central Google Scholar
Seibt, J. et al. Cortical dendritic activity correlates with spindle-rich oscillations during sleep in rodents. Nat. Commun. 8 , 684 (2017).
Article ADS PubMed PubMed Central Google Scholar
Niethard, N., Ngo, H.-V. V., Ehrlich, I. & Born, J. Cortical circuit activity underlying sleep slow oscillations and spindles. Proc. Natl. Acad. Sci. USA 115 , E9220–E9229 (2018).
Buzsáki, G. Hippocampal sharp wave-ripple: A cognitive biomarker for episodic memory and planning. Hippocampus 25 , 1073–1188 (2015).
O’Neill, J., Pleydell-Bouverie, B., Dupret, D. & Csicsvari, J. Play it again: reactivation of waking experience and memory. Trends Neurosci. 33 , 220–229 (2010).
Helfrich, R. F. et al. Bidirectional prefrontal-hippocampal dynamics organize information transfer during sleep in humans. Nat. Commun. 10 , 3572 (2019).
Ngo, H.-V., Fell, J. & Staresina, B. Sleep spindles mediate hippocampal-neocortical coupling during long-duration ripples. ELife 9 , e57011 (2020).
Siapas, A. G. & Wilson, M. A. Coordinated interactions between hippocampal ripples and cortical spindles during slow-wave sleep. Neuron 21 , 1123–1128 (1998).
Brodt, S., Inostroza, M., Niethard, N. & Born, J. Sleep—A brain-state serving systems memory consolidation. Neuron 111 , 1050–1075 (2023).
Staresina, B. P. et al. Hierarchical nesting of slow oscillations, spindles and ripples in the human hippocampus during sleep. Nat. Neurosci. 18 , 1679–1686 (2015).
Staresina, B. P., Niediek, J., Borger, V., Surges, R. & Mormann, F. How coupled slow oscillations, spindles and ripples coordinate neuronal processing and communication during human sleep. Nat. Neurosci. 26 , 1429–1437 (2023).
van Schalkwijk, F. J. et al. An evolutionary conserved division-of-labor between archicortical and neocortical ripples organizes information transfer during sleep. Prog. Neurobiol. 227 , 102485 (2023).
Jiang, X., Gonzalez-Martinez, J. & Halgren, E. Coordination of human hippocampal sharpwave ripples during NREM sleep with cortical theta bursts, spindles, downstates, and upstates. J. Neurosci. 39 , 8744–8761 (2019).
Jiang, X., Gonzalez-Martinez, J. & Halgren, E. Posterior hippocampal spindle ripples co-occur with neocortical theta bursts and downstates-upstates, and phase-lock with parietal spindles during NREM sleep in humans. J. Neurosci. 39 , 8949–8968 (2019).
Geva-Sagiv, M. et al. Augmenting hippocampal–prefrontal neuronal synchrony during sleep enhances memory consolidation in humans. Nat. Neurosci. 26 , 1100–1110 (2023).
Oudiette, D. & Paller, K. A. Upgrading the sleeping brain with targeted memory reactivation. Trends Cogn. Sci. 17 , 142–149 (2013).
Hu, X., Cheng, L. Y., Chiu, M. H. & Paller, K. A. Promoting memory consolidation during sleep: A meta-analysis of targeted memory reactivation. Psychol. Bull. 146 , 218–244 (2020).
Göldi, M., van Poppel, E. A. M., Rasch, B. & Schreiner, T. Increased neuronal signatures of targeted memory reactivation during slow-wave up states. Sci. Rep. 9 , 2715 (2019).
Oyarzún, J. P., Morís, J., Luque, D., Diego-Balaguer, Rde & Fuentemilla, L. Targeted memory reactivation during sleep adaptively promotes the strengthening or weakening of overlapping memories. J. Neurosci. 37 , 7748–7758 (2017).
Schechtman, E. et al. Multiple memories can be simultaneously reactivated during sleep as effectively as a single memory. Commun. Biol. 4 , 1–13 (2021).
Vaz, A. P., Inati, S. K., Brunel, N. & Zaghloul, K. A. Coupled ripple oscillations between the medial temporal lobe and neocortex retrieve human memory. Science 363 , 975–978 (2019).
Norman, Y. et al. Hippocampal sharp-wave ripples linked to visual episodic recollection in humans. Science 365 , eaax1030 (2019).
Article ADS CAS PubMed Google Scholar
Norman, Y., Raccah, O., Liu, S., Parvizi, J. & Malach, R. Hippocampal ripples and their coordinated dialogue with the default mode network during recent and remote recollection. Neuron 109 , 2767–2780.e5 (2021).
Henin, S. et al. Spatiotemporal dynamics between interictal epileptiform discharges and ripples during associative memory processing. Brain 144 , 1590–1602 (2021).
Kunz, L. et al. Ripple-locked coactivity of stimulus-specific neurons and human associative memory. Nat Neurosci 27 , 587–599 (2024).
Dickey, C. W. et al. Widespread ripples synchronize human cortical activity during sleep, waking, and memory recall. Proc. Natl. Acad. Sci. USA 119 , e2107797119 (2022).
Clemens, Z. et al. Temporal coupling of parahippocampal ripples, sleep spindles and slow oscillations in humans. Brain 130 , 2868–2878 (2007).
Chen, Y. Y. et al. Stability of ripple events during task engagement in human hippocampus. Cell Rep. 35 , 109304 (2021).
Maingret, N., Girardeau, G., Todorova, R., Goutierre, M. & Zugaro, M. Hippocampo-cortical coupling mediates memory consolidation during sleep. Nat. Neurosci. 19 , 959–964 (2016).
Latchoumane, C.-F. V., Ngo, H.-V. V., Born, J. & Shin, H.-S. Thalamic spindles promote memory formation during sleep through triple phase-locking of cortical, thalamic, and hippocampal rhythms. Neuron 95 , 424–435.e6 (2017).
Tort, A. B. L., Komorowski, R., Eichenbaum, H. & Kopell, N. Measuring phase-amplitude coupling between neuronal oscillations of different frequencies. J. Neurophysiol. 104 , 1195–1210 (2010).
Cox, R., Rüber, T., Staresina, B. P. & Fell, J. Heterogeneous profiles of coupled sleep oscillations in human hippocampus. Neuroimage 202 , 116178 (2019).
Joo, H. R. & Frank, L. M. The hippocampal sharp wave–ripple in memory retrieval for immediate use and consolidation. Nat. Rev. Neurosci. 19 , 744–757 (2018).
Yang, W. et al. Selection of experience for memory by hippocampal sharp wave ripples. Science 383 , 1478–1483 (2024).
Rasch, B. & Born, J. About sleep’s role in memory. Physiol. Rev. 93 , 681–766 (2013).
Chen, Z. S. & Wilson, M. A. Now and Then: How Our Understanding of Memory Replay Evolves. J. Neurophysiol. 93 , 681–766 (2023).
Rothschild, G., Eban, E. & Frank, L. M. A cortical-hippocampal-cortical loop of information processing during memory consolidation. Nat. Neurosci. 20 , 251–259 (2017).
Bendor, D. & Wilson, M. A. Biasing the content of hippocampal replay during sleep. Nat. Neurosci. 15 , 1439–1444 (2012).
Rothschild, G. The transformation of multi-sensory experiences into memories during sleep. Neurobiol. Learn Mem. 160 , 58–66 (2019).
Oostenveld, R., Stegeman, D. F., Praamstra, P. & van Oosterom, A. Brain symmetry and topographic analysis of lateralized event-related potentials. Clin. Neurophysiol. 114 , 1194–1202 (2003).
Helfrich, R. F., Lendner, J. D. & Knight, R. T. Aperiodic sleep networks promote memory consolidation. Trends Cogn. Sci. 25 , 648–659 (2021).
Schreiner, T., Petzka, M., Staudigl, T. & Staresina, B. P. Endogenous memory reactivation during sleep in humans is clocked by slow oscillation-spindle complexes. Nat. Commun. 12 , 3112 (2021).
Schechtman, E., Heilberg, J. & Paller, K. A. Memory consolidation during sleep involves context reinstatement in humans. Cell Rep. 42 , 112331 (2023).
Nunez, P. L. & Srinivasan, R. Electric Fields of the Brain: The Neurophysics of Eeg . (Oxford University Press, Oxford; New York, 2005).
Staudigl, T., Vollmar, C., Noachtar, S. & Hanslmayr, S. Temporal-pattern similarity analysis reveals the beneficial and detrimental effects of context reinstatement on human memory. J. Neurosci. 35 , 5373–5384 (2015).
Miller, J. F. et al. Neural activity in human hippocampal formation reveals the spatial context of retrieved memories. Science 342 , 1111–1114 (2013).
Schechtman, E., Heilberg, J. & Paller, K. A. Memory consolidation during sleep involves context reinstatement in humans. Cell reports 42 , 112331 (2023).
Stangl, M., Maoz, S. L. & Suthana, N. Mobile cognition: imaging the human brain in the ‘real world’. Nat. Rev. Neurosci. 24 , 347–362 (2023).
Taube, J. S., Valerio, S. & Yoder, R. M. Is navigation in virtual reality with FMRI really navigation? J. Cogn. Neurosci. 25 , 1008–1019 (2013).
Stangl, M. et al. Boundary-anchored neural mechanisms of location-encoding for self and others. Nature 589 , 420–425 (2021).
Griffiths, B. J. et al. Electrophysiological signatures of veridical head direction in humans. Nat. Hum. Behav. 1–17 https://doi.org/10.1038/s41562-024-01872-1 (2024).
Taube, J., Muller, R. & Ranck, J. Head-direction cells recorded from the postsubiculum in freely moving rats. I. description and quantitative analysis. J. Neurosci. 10 , 420–435 (1990).
Cullen, K. E. & Taube, J. S. Our sense of direction: progress, controversies and challenges. Nat. Neurosci. 20 , 1465–1473 (2017).
Hulse, B. K. & Jayaraman, V. Mechanisms underlying the neural computation of head direction. Annu. Rev. Neurosci. 43 , 31–54 (2020).
Geva-Sagiv, M., Las, L., Yovel, Y. & Ulanovsky, N. Spatial cognition in bats and rats: from sensory acquisition to multiscale maps and navigation. Nat. Rev. Neurosci. 16 , 94–108 (2015).
Peyrache, A., Lacroix, M. M., Petersen, P. C. & Buzsáki, G. Internally organized mechanisms of the head direction sense. Nat. Neurosci. 18 , 569–575 (2015).
Chaudhuri, R., Gerçek, B., Pandey, B., Peyrache, A. & Fiete, I. The intrinsic attractor manifold and population dynamics of a canonical cognitive circuit across waking and sleep. Nat. Neurosci. 22 , 1512–1520 (2019).
Viejo, G. & Peyrache, A. Precise coupling of the thalamic head-direction system to hippocampal ripples. Nat. Commun. 11 , 2524 (2020).
Ajabi, Z., Keinath, A. T., Wei, X.-X. & Brandon, M. P. Population dynamics of head-direction neurons during drift and reorientation. Nature 615 , 892–899 (2023).
Zirkelbach, J., Stemmler, M. & Herz, A. V. M. Anticipatory neural activity improves the decoding accuracy for dynamic head-direction signals. J. Neurosci. 39 , 2847–2859 (2019).
Kunz, L. et al. Mesoscopic neural representations in spatial navigation. Trends Cogn. Sci. 23 , 615–630 (2019).
Buzsáki, G. & Moser, E. I. Memory, navigation and theta rhythm in the hippocampal-entorhinal system. Nat. Neurosci. 16 , 130–138 (2013).
Schreiner, T. & Rasch, B. Boosting vocabulary learning by verbal cueing during sleep. Cereb. Cortex 25 , 4169–4179 (2015).
Schreiner, T., Lehmann, M. & Rasch, B. Auditory feedback blocks memory benefits of cueing during sleep. Nat. Commun. 6 , 8729 (2015).
Antony, J. W., Gobel, E. W., O’Hare, J. K., Reber, P. J. & Paller, K. A. Cued memory reactivation during sleep influences skill learning. Nat. Neurosci. 15 , 1114–1116 (2012).
Schönauer, M., Geisler, T. & Gais, S. Strengthening procedural memories by reactivation in sleep. J. Cogn. Neurosci. 26 , 143–153 (2014).
Lewis, P. A. & Bendor, D. How targeted memory reactivation promotes the selective strengthening of memories in sleep. Curr. Biol. 29 , R906–R912 (2019).
Joensen, B. H. et al. Targeted memory reactivation during sleep can induce forgetting of overlapping memories. Learn Mem. 29 , 401–411 (2022).
Antony, J. W., Cheng, L. Y., Brooks, P. P., Paller, K. A. & Norman, K. A. Competitive learning modulates memory consolidation during sleep. Neurobiol. Learn. Mem. 155 , 216–230 (2018).
Mensink, G.-J. & Raaijmakers, J. G. A model for interference and forgetting. Psychol. Rev. 95 , 434–455 (1988).
Anderson, M. C., Bjork, R. A. & Bjork, E. L. Remembering can cause forgetting: retrieval dynamics in long-term memory. J. Exp. Psychol. Learn. Mem. Cogn. 20 , 1063–1087 (1994).
Yonelinas, A. P. Receiver-operating characteristics in recognition memory: evidence for a dual-process model. J. Exp. Psychol. Learn. Mem. Cogn. 20 , 1341–1354 (1994).
Squire, L. R., Wixted, J. T. & Clark, R. E. Recognition memory and the medial temporal lobe: a new perspective. Nat. Rev. Neurosci. 8 , 872–883 (2007).
DeSoto, K. A. & Roediger, H. L. Positive and negative correlations between confidence and accuracy for the same events in recognition of categorized Lists. Psychol. Sci. 25 , 781–788 (2014).
Witkowski, S. et al. Does memory reactivation during sleep support generalization at the cost of memory specifics? Neurobiol. Learn Mem. 182 , 107442 (2021).
Grootswagers, T., Wardle, S. G. & Carlson, T. A. Decoding dynamic brain patterns from evoked responses: A tutorial on multivariate pattern analysis applied to time series neuroimaging data. J. Cogn. Neurosci. 29 , 677–697 (2017).
Kriegeskorte, N., Mur, M. & Bandettini, P. Representational similarity analysis - connecting the branches of systems neuroscience. Front. Syst. Neurosci. 2 , 4 (2008).
PubMed PubMed Central Google Scholar
Wang, B. et al. Targeted memory reactivation during sleep elicits neural signals related to learning content. J. Neurosci. 39 , 6728–6736 (2019).
Cairney, S. A., Guttesen, A. Á. V., El Marj, N. & Staresina, B. P. Memory consolidation is linked to spindle-mediated information processing during sleep. Curr. Biol. 28 , 948–954.e4 (2018).
Ngo, H.-V. V. & Staresina, B. P. Shaping overnight consolidation via slow-oscillation closed-loop targeted memory reactivation. Proc. Natl. Acad. Sci. USA 119 , e2123428119 (2022).
Helfrich, R. F., Mander, B. A., Jagust, W. J., Knight, R. T. & Walker, M. P. Old brains come uncoupled in sleep: slow wave-spindle synchrony, brain atrophy, and forgetting. Neuron 97 , 221–230.e4 (2018).
Buysse, D. J., Reynolds, C. F., Monk, T. H., Berman, S. R. & Kupfer, D. J. The Pittsburgh sleep quality index: A new instrument for psychiatric practice and research. Psychiatry Res. 28 , 193–213 (1989).
Horne, J. A. & Ostberg, O. A self-assessment questionnaire to determine morningness-eveningness in human circadian rhythms. Int. J. Chronobiol. 4 , 97–110 (1976).
CAS PubMed Google Scholar
Konkle, T., Brady, T. F., Alvarez, G. A. & Oliva, A. Conceptual distinctiveness supports detailed visual long-term memory for real-world objects. J. Exp. Psychol. Gen. 139 , 558–578 (2010).
Brainard, D. H. The psychophysics toolbox. Spat. Vis. 10 , 433–436 (1997).
Iber, C., Ancoli-Israel, S., Chesson, A. L. & Quan, S. F. The AASM Manual for the Scoring of Sleep and Associated Events: Rules, Terminology, and Technical Specification . (American Academy of Sleep Medicine, 2007). https://doi.org/10.1007/978-3-7985-1852-0 .
Oostenveld, R., Fries, P., Maris, E. & Schoffelen, J.-M. FieldTrip: Open source software for advanced analysis of MEG, EEG, and invasive electrophysiological data. Comput. Intell. Neurosci. 2011 , 156869 (2011).
Davachi, L. Item, context and relational episodic encoding in humans. Curr. Opin. Neurobiol. 16 , 693–700 (2006).
Gross, J. et al. Dynamic imaging of coherent sources: Studying neural interactions in the human brain. Proc. Natl. Acad. Sci. USA 98 , 694–699 (2001).
Tzourio-Mazoyer, N. et al. Automated anatomical labeling of activations in SPM using a macroscopic anatomical parcellation of the MNI MRI single-subject brain. Neuroimage 15 , 273–289 (2002).
Treder, M. S. MVPA-Light: A classification and regression toolbox for multi-dimensional data. Front. Neurosci. 14 , 289 (2020).
Jackson, J. E. A User’s Guide to Principal Components . (Wiley-Interscience, Hoboken, N.J, 2003).
Lemm, S., Blankertz, B., Dickhaus, T. & Müller, K.-R. Introduction to machine learning for brain imaging. Neuroimage 56 , 387–399 (2011).
King, J.-R. & Dehaene, S. Characterizing the dynamics of mental representations: the temporal generalization method. Trends Cogn. Sci. 18 , 203–210 (2014).
Kriegeskorte, N. Pattern-information analysis: from stimulus decoding to computational-model testing. Neuroimage 56 , 411–421 (2011).
Zhang, H., Fell, J. & Axmacher, N. Electrophysiological mechanisms of human memory consolidation. Nat. Commun. 9 , 4103 (2018).
Berens, P. CircStat: A MATLAB toolbox for circular statistics. J. Stat. Softw. 31 , 1–21 (2009).
Staudigl, T. Data for: Schreiner et al. Spindle-locked ripples mediate memory reactivation during human NREM sleep. Nat. Commun. https://doi.org/10.5282/ubm/data.487 (2024).
Download references
Acknowledgements
This work was supported by the European Research Council ( https://erc.europa.eu/ , Starting Grant 802681 awarded to T.St). T.S. is supported by the Emmy Noether program of the German Research Foundation (492835154). We are indebted to all patients and participants who volunteered to participate in this study. We thank the staff and physicians at the Epilepsy Center, Department of Neurology, Ludwig-Maximilians-Universität München for assistance.
Open Access funding enabled and organized by Projekt DEAL.
Author information
Authors and affiliations.
Department of Psychology, Ludwig-Maximilians-Universität München, Munich, Germany
Thomas Schreiner, Benjamin J. Griffiths, Merve Kutlu & Tobias Staudigl
Centre for Human Brain Health, University of Birmingham, Birmingham, UK
Benjamin J. Griffiths
Epilepsy Center, Department of Neurology, Ludwig-Maximilians-Universität München, Munich, Germany
Christian Vollmar, Elisabeth Kaufmann, Jan Remi & Soheyl Noachtar
Department of Neurosurgery, University Hospital Munich, Ludwig-Maximilians-Universität München, Munich, Germany
Stefanie Quach
You can also search for this author in PubMed Google Scholar
Contributions
Conceptualization: T.S., T.St.; Data acquisition: T.S, T.St., M.K., B.G; Resources: C.V., E.K., S.Q., J.R., S.N.; Formal Analysis: T.S., T.St., B.G.; Funding acquisition: T.St.; Writing – original draft: T.S., T.St.; Writing– review & editing: T.S., T.St., M.K., B.G.
Corresponding author
Correspondence to Tobias Staudigl .
Ethics declarations
Competing interests.
The authors declare no competing interests.
Peer review
Peer review information.
Nature Communications thanks Yitzhak Norman, and the other, anonymous, reviewers for their contribution to the peer review of this work. A peer review file is available.
Additional information
Publisher’s note Springer Nature remains neutral with regard to jurisdictional claims in published maps and institutional affiliations.
Supplementary information
Supplementary information, peer review file, reporting summary, source data, source data, rights and permissions.
Open Access This article is licensed under a Creative Commons Attribution 4.0 International License, which permits use, sharing, adaptation, distribution and reproduction in any medium or format, as long as you give appropriate credit to the original author(s) and the source, provide a link to the Creative Commons licence, and indicate if changes were made. The images or other third party material in this article are included in the article’s Creative Commons licence, unless indicated otherwise in a credit line to the material. If material is not included in the article’s Creative Commons licence and your intended use is not permitted by statutory regulation or exceeds the permitted use, you will need to obtain permission directly from the copyright holder. To view a copy of this licence, visit http://creativecommons.org/licenses/by/4.0/ .
Reprints and permissions
About this article
Cite this article.
Schreiner, T., Griffiths, B.J., Kutlu, M. et al. Spindle-locked ripples mediate memory reactivation during human NREM sleep. Nat Commun 15 , 5249 (2024). https://doi.org/10.1038/s41467-024-49572-8
Download citation
Received : 30 October 2023
Accepted : 11 June 2024
Published : 19 June 2024
DOI : https://doi.org/10.1038/s41467-024-49572-8
Share this article
Anyone you share the following link with will be able to read this content:
Sorry, a shareable link is not currently available for this article.
Provided by the Springer Nature SharedIt content-sharing initiative
By submitting a comment you agree to abide by our Terms and Community Guidelines . If you find something abusive or that does not comply with our terms or guidelines please flag it as inappropriate.
Quick links
- Explore articles by subject
- Guide to authors
- Editorial policies
Sign up for the Nature Briefing newsletter — what matters in science, free to your inbox daily.

LAist is part of Southern California Public Radio, a member-supported public media network.

Scientists zap sleeping humans' brains with electricity to improve their memory

A little brain stimulation at night appears to help people remember what they learned the previous day.
A study of 18 people with severe epilepsy found that they scored higher on a memory test if they got deep brain stimulation while they slept, a team reports in the journal Nature Neuroscience .
The stimulation was delivered during non-REM sleep, when the brain is thought to strengthen memories it expects to use in the future. It was designed to synchronize the activity in two brain areas involved in memory consolidation : the hippocampus and the prefrontal cortex.
"Some improved by 10% or 20%, some improved by 80%," depending on the level of synchrony, says Dr. Itzhak Fried , an author of the study and a professor of neurosurgery at the University of California, Los Angeles.
The results back a leading theory of how the brain transforms a daily event into a memory that can last for days, weeks, or even years. They also suggest a new approach to helping people with a range of sleep and memory problems.
"We know for instance that in patients with dementia, with Alzheimer, sleep is not working very well at all," Fried says. "The question is whether by changing the architecture of sleep, you can help memory."
Although the results are from a small study of people with a specific disorder (epilepsy), they are "reason to celebrate," says Dr. György Buzsáki , a professor of neuroscience at New York University who was not involved in the research.
Rhythms in the brain
During sleep, brain cells fire in rhythmic patterns. Scientists believe that when two brain areas synchronize their firing patterns, they are able to communicate.
Studies suggest that during non-REM sleep, the hippocampus, found deep in the brain, synchronizes its activity with the prefrontal cortex, which lies just behind the forehead. That process appears to help transform memories from the day into memories that can last a lifetime.
So Fried and his team wanted to know whether increasing synchrony between the two brain areas could improve a person's memory of facts and events.
Their study involved epilepsy patients who already had electrodes in their brains as part of their medical evaluation. This gave the scientists a way to both monitor and alter a person's brain rhythms.
They measured memory using a "celebrity pet" test in which participants were shown a series of images that matched a particular celebrity with a specific animal. The goal was to remember which animal went with which celebrity.
Patients saw the images before going to bed. Then, while they slept, some of them got tiny pulses of electricity through the wires in their brains.
"We were measuring the activity in one area deep in the brain [the hippocampus], and then, based on this, we were stimulating in a different area [the prefrontal cortex]," Fried says.
In patients who got the stimulation, rhythms in the two brain areas became more synchronized. And when those patients woke up they did better on the celebrity pet test.
The results back decades of research on animals showing the importance of rhythm and synchrony in forming long-term memories.
"If you would like to talk to the brain, you have to talk to it in its own language," Buzsáki says.
But altering rhythms in the brain of a healthy person might not improve their memory, he says, because those communication channels are already optimized.
The epilepsy patients may have improved because they started out with sleep and memory problems caused by both the disorder and the drugs used to treat it.
"Maybe what happened here is just making worse memories better," Buzsáki says.
Even so, he says, the approach has the potential to help millions of people with impaired memory. And brain rhythms probably play an important role in many other problems.
"They are not specific to memory. They are doing a lot of other things," Buzsáki says, like regulating mood and emotion.
So tweaking brain rhythms might also help with disorders like depression, he says.

An official website of the United States government
The .gov means it’s official. Federal government websites often end in .gov or .mil. Before sharing sensitive information, make sure you’re on a federal government site.
The site is secure. The https:// ensures that you are connecting to the official website and that any information you provide is encrypted and transmitted securely.
- Publications
- Account settings
Preview improvements coming to the PMC website in October 2024. Learn More or Try it out now .
- Advanced Search
- Journal List
- Proc Natl Acad Sci U S A
- v.119(44); 2022 Nov 1

Sleep, Brain, and Cognition Special Feature
Contributions of memory and brain development to the bioregulation of naps and nap transitions in early childhood, rebecca m. c. spencer.
a Department of Psychological & Brain Sciences, University of Massachusetts, Amherst, MA 01003;
b Neuroscience & Behavior Program, University of Massachusetts, Amherst, MA 01003;
c Institute for Applied Life Sciences, University of Massachusetts, Amherst, MA 01003;
Tracy Riggins
d Department of Psychology, University of Maryland, College Park, MD 20742
Author contributions: R.M.C.S. and T.R. designed research, performed research, and wrote the paper.
Associated Data
There are no data underlying this work.
The transition from multiple sleep bouts each day to a single overnight sleep bout (i.e., nap transition) is a universal process in human development. Naps are important during infancy and early childhood as they enhance learning through memory consolidation. However, a normal part of development is the transition out of naps. Understanding nap transitions is essential in order to maximize early learning and promote positive long-term cognitive outcomes. Here, we propose a novel hypothesis regarding the cognitive, physiological, and neural changes that accompany nap transitions. Specifically, we posit that maturation of the hippocampal-dependent memory network results in more efficient memory storage, which reduces the buildup of homeostatic sleep pressure across the cortex (as reflected by slow-wave activity), and eventually, contributes to nap transitions. This hypothesis synthesizes evidence of bioregulatory mechanisms underlying nap transitions and sheds new light on an important window of change in development. This framework can be used to evaluate multiple untested predictions from the field of sleep science and ultimately, yield science-based guidelines and policies regarding napping in childcare and early education settings.
Children spend as much as half of their early years asleep ( 1 ). Sleep promotes healthy brain and cognitive development ( 2 ). However, the mechanisms underlying these relations are poorly understood. Understanding children’s sleep needs can be used to promote optimal cognitive function and is deemed a priority in sleep research ( 3 ). Additionally, whether and for how long sleep needs must be met with naps are concerns for parents and early childhood educators.
With growing investments in universal early education in the United States, there is increasing scrutiny and awareness of how time is spent in these settings in order to maximize academic success. Should children be sleeping or learning in the early education setting? Ample data in adults and children support a role of sleep in solidifying memories, and yet, the intuition is that classroom time should be spent learning, not sleeping ( 4 , 5 ). Moreover, some children within the preschool age range seem unable to nap, while others, at the same age, can be hard to keep awake ( 6 ). Because there are currently no formal recommendations regarding nap timing/length or nap transitions (e.g., from the American Academy of Pediatrics or the National Sleep Foundation), some have devalued daytime naps for children over the age of 2 y ( 7 ), even proposing the elimination of naps in publicly funded preschools ( 4 ). Related, sleep advice for parents at this age is provided by a growing number of sleep coaches; however, these individuals lack evidence-based information and guidance on such issues ( 8 ). Therefore, in order to promote optimal learning and development through sleep in infancy and early childhood, a scientific understanding of nap transitions is essential as this will inform guidelines regarding nap needs and transition points.
The need to nap is driven by the bioregulation of sleep and wakefulness ( 9 ). Sleep regulation, which can be thought of as “the drive to sleep when we do,” underlies the need for naps as well as the ability to adjust to schedule changes (e.g., daylight savings, jetlag). Disorders of sleep regulation result in insufficient sleep, which is associated with depression and anxiety, even in childhood ( 10 ). Sleep regulation is also altered in neurodevelopmental disorders (e.g., autism, Down syndrome, Attention-deficit/hyperactivity disorder or ADHD) ( 11 , 12 ). Here, we describe what is known regarding the bioregulation of sleep in early development. We first discuss the relation between nap transitions and sleep regulation, including underlying neural and physiological mechanisms. Next, we consider how changes in the underlying neural substrates are related to changes in early learning and memory. Taken together, these data lead to our novel prediction that nap transitions are driven by changes in memory that are related to brain development.
1. Nap Transitions in Infants and Children
Newborns sleep up to 20 h each day, with sleep distributed across multiple sleep bouts (polyphasic), largely reflecting the need to feed frequently. Overnight sleep becomes distinct from daytime naps between 4 and 6 mo in most infants, but sleep remains polyphasic, with three or more naps in addition to the overnight sleep bout ( 13 ).
Sleep becomes triphasic by 9 mo of age, consisting of two daytime naps and an overnight sleep bout ( 14 ). Between the first and second years of life, the morning nap fades, and sleep becomes biphasic ( 1 , 14 ). The transition to adult-like monophasic sleep most commonly occurs in the early childhood years ( 13 – 15 ). However, there is significant variability in the timing of the transition from triphasic and biphasic sleep (ranging from 6 to 18 mo) and even more variability in the transition out of biphasic sleep (which can be as early as 2 y and as late as 8 y of age) ( 13 , 16 ).
We opt to use the terminology “nap transition” (as opposed to “nap cessation”) to capture the fact that changes in nap patterns are not instantaneous. A longitudinal study of early nap transitions ( 17 ) and meta-analyses of cross-sectional data support that transitions take place over a number of months, with naps gradually reducing in frequency and length over time ( 6 , 13 , 15 ). Central to our research is that these sleep transition periods are interesting windows of change in brain and memory development.
2. Bioregulation of Sleep and Naps
2.1. bioregulation of sleep..
The two-process model of sleep regulation is a prominent conceptual model, which posits that sleep regulation emerges from the interaction of two distinct biological processes ( 18 , 19 ). The first process is the circadian control of sleep, termed Process C, which underlies the 24-h pattern in sleep behaviors. The second process is the homeostatic regulation of sleep through a mechanism termed Process S or the homeostatic sleep drive. This is the process that allows for the self-regulation of sleep, adjusting to changing conditions while preserving sleep.
2.1.1. Circadian regulation of sleep (Process C).
Underlying Process C is a central circadian pacemaker that synchronizes multiple physiological processes to the same 24-h rhythm. Located in the suprachiasmatic nucleus (SCN) of the anterior hypothalamus, the circadian pacemaker is entrained to external cues or zeitgebers. Social cues, such as routine mealtimes and exercise, can serve as zeitgebers ( 20 ). However, the most common zeitgebers are light/dark cues. The SCN signals the pineal gland to synthesize the hormone melatonin on a circadian schedule. Although melatonin does not promote sleep per se, it is released from the pineal gland in darkness and reciprocally inhibits the SCN, dampening its alertness-promoting actions and ultimately, demoting wakefulness ( 21 ).
2.1.2. Homeostatic regulation of sleep (Process S).
If left to the circadian system alone, we would fall asleep at the same time and wake up at the same time regardless of sleep history; overnight sleep timing would be the same following a night of poor sleep and following a luxurious afternoon nap (one not taken to compensate for sleep loss). Rather, the common experience is that it is tempting to fall asleep much earlier following a night of poor sleep and hard to fall asleep at the usual time following an afternoon nap. This reflects the second aspect of sleep regulation, Process S or the homeostatic mechanism that drives sleep. Homeostatic sleep pressure accumulates across periods of wake and dissipates with subsequent sleep ( 19 ). The mechanism underlying homeostatic control is complex, and current evidence suggests that it may be a distributed process across multiple pathways (reviewed in ref. 22 ).
A neurobiological marker of homeostatic sleep pressure is slow-wave activity (SWA), activity in the delta-frequency band (0.5 to 4.5 Hz) in the sleep electroencephalogram (EEG) thought to be cortically generated ( 23 ). SWA reflects the frequency of the high-amplitude slow waves characteristic of slow-wave sleep. SWA is highest early in a sleep bout and dissipates across the sleep bout. Moreover, SWA is higher following sleep deprivation, supporting the use of SWA changes as a measure of the accumulation of homeostatic sleep pressure ( 24 ). Wake-related increases in SWA may result from global increases in synaptic strength related to learning. Slow waves emerge from bistability of cortical neurons in non-rapid eye movement (non-REM) sleep, oscillating between wake-like tonic firing (up state) and neuronal silence (down state) ( 25 ). Following long periods of wake, increased global synaptic strength may contribute to higher neural synchrony or a greater number of neurons contributing to the oscillation. Collectively, there is clear support that SWA is functionally involved in maintaining sleep homeostasis, but little more is understood regarding this mechanism.
2.2. Bioregulation of Naps and Nap Transitions in Early Childhood.
Naps and nap transitions are influenced by multiple factors, including genes (although minimally) ( 17 , 26 ), environment ( 27 , 28 ), and culture ( 29 – 31 ). The two-process model provides a framework to understand how these factors exert their influence on sleep. For example, parents may choose to promote a consistent nap time ( 28 ), reflecting circadian contributions to nap patterns. However, many children will nap even in the absence of nap promotion, and some children are unable to nap even in sleep-promoting conditions ( 6 ), likely reflecting homeostatic processes (i.e., variability in the accumulation of homeostatic sleep pressure).
2.2.1. Circadian contribution to nap patterns.
Consistency of nap times is recommended as it is thought to promote stable and predictable sleep patterns ( 32 ). As such, parents and childcare providers institute many zeitgebers (such as light availability), which may then reinforce a circadian drive to sleep at these times during the day. There is evidence that these changes in environment alter the onset of melatonin and ultimately, timing of sleep ( 33 ). Conversely, parents with negative views toward naps in this age group likely provide no zeitgebers or no nap opportunity, and as such, those children have fewer naps and naps of shorter duration ( 28 ). Cultural variation in naps may likewise reflect the use of light and rule setting, which may promote or demote daytime sleep ( 16 , 33 ).
2.2.2. Homeostatic contributions to nap patterns.
Whereas parent-guided zeitgebers can shift the circadian timing of sleep bouts, homeostatic control of sleep offers an explanation as to why naps can result from late bedtimes. Late bedtimes are often followed by too-early wake times due to parent or childcare schedules. The resulting shortened overnight sleep creates additional sleep pressure or the need to nap the subsequent day. However, while this provides an explanation of how environmental and cultural influences contribute to the presence of napping, they do not provide an obvious explanation of how and when nap transitions may occur. In other words, they do not explain the persistence of napping.
Nap transitions have most commonly been ascribed to Process S ( 34 , 35 ). Specifically, more rapid accumulation of homeostatic sleep pressure in young compared with older children is thought to create the need to more frequently nap to release this sleep pressure. Preliminary support of this comes from a study of a small sample ( n = 8) of 2- to 5-y-old children ( 36 ). Sleep pressure was varied by altering the amount of time that the child was awake before a nap was permitted (4, 7, or 10 h after waking). Consistent with the view that SWA serves as a proxy for sleep pressure, SWA in the nap was greater following longer intervals awake. However, this difference dissipated with age; the difference in SWA across conditions was less in younger compared with older children. This suggests that sleep pressure accumulates more rapidly in young children, who are also more likely to be habitual nappers.
In sum, there is evidence that both circadian (Process C) and homeostatic (Process S) sleep regulation processes contribute to whether a child naps ( Fig. 1 A ). To date, most research regarding these processes and individual differences in nap transitions has focused on external factors, such as the extent to which parents choose to promote napping ( 28 ), a factor that varies across cultures and socioeconomic groups ( 27 , 29 ). Despite these external influences, many children will nap even in the absence of nap promotion, and some children are unable to nap even in sleep-promoting conditions ( 6 ). These differences likely stem from internal processes and reflect variability in the accumulation of homeostatic sleep pressure ( 35 ). However, the biological mechanisms underlying these internal processes remain unclear. Specifically, what underlies the accumulation of homeostatic sleep pressure, and why does it vary developmentally? Additionally, how do these developmental changes contribute to changes in accumulation of homeostatic sleep pressure and ultimately, the transition out of naps? In the following section, we present a novel hypothesis that may provide answers to these questions by linking what we currently know about nap transitions with what we know about the development of the brain and its capacity to learn and remember at an early age.

Understanding nap transitions through the two-process model of sleep regulation. ( A ) Although environmental and cultural factors (yellow; e.g., caregiver schedules and the use of light) may influence the presence of naps, they are unlikely to explain the persistence of naps and nap transitions. Rather, nap transitions are posited to largely be related to homeostatic processes (indicated by the pink arrow), with greater accumulation of sleep pressure in habitually compared with nonhabitually napping children. ( B ) We hypothesize that brain development and memory development underlie this difference in homeostatic sleep pressure. Specifically, as the hippocampal-dependent memory network develops, memories can be held for longer without interference, making more space and/or using less energy resources, which may (directly or indirectly) yield sleep pressure as measured by SWA and result in nap transitions.
3. A Novel Hypothesis of Nap Transitions
A unique problem exists early in human development; there is a massive amount of information that must be learned, yet the neural systems that support learning are still under construction. Schema and semantic networks that support learning in adults ( 37 , 38 ) are nascent at very young ages and must be built from scratch. Moreover, the structural and functional neural circuitry that supports memory is also immature. Specifically, the hippocampus—a brain region associated with learning and memory—undergoes a period of “learning to learn” ( 39 ). Finally, to support early learning, there is overproduction of synapses across the brain, which is most prolonged in prefrontal or higher cortical areas. The increased firing rate that accompanies high synaptic density escalates energy demand ( 40 ).
Seen in this light, naps provide a solution to what is essentially a memory problem; high learning demands on an immature system create an overproduction of synapses that reaches its peak in early childhood. Sleep has been argued to help meet space and energy challenges associated with development ( 40 , 41 ). Thus, we posit that early childhood is a time of competing demands of learning, which loads the brain (the hippocampus in particular), and sleeping, which may unload synapses across the cortex ( 42 ) and free up the hippocampus for ongoing learning ( 43 ).
This leads to our hypothesis that maturation of the brain, particularly the hippocampal-dependent memory network, during early childhood results in more efficient memory storage, which reduces the buildup of homeostatic sleep pressure (as reflected by SWA) and eventually, contributes to nap transitions ( Fig. 1 B ). In the following sections, we provide motivation for this novel hypothesis and review initial support for this view.
3.1. Role of the Hippocampus in Learning and Memory in Early Development.
The ability to learn novel information and recall it later relies on a network of brain regions, including the hippocampus and neocortex. Together, these regions provide “complementary learning systems” that allow for the rapid learning of new information while both preserving existing knowledge and integrating new knowledge into these existing frameworks ( 41 ). The hippocampus is particularly important for the early stages of memory, including formation and consolidation (or stabilization) ( 44 ). In short, this structure provides short-term storage and initially works together with the neocortex to support memory of new information across long delays. Over time, connectivity among distributed cortical regions strengthens, and the role of the hippocampus gradually declines. Although learning initiates memory processes in the hippocampus, these memory traces are vulnerable to interference and forgetting. Once memories are consolidated and stabilized in the cortex, they are more robust against disruption ( 41 ).
The hippocampus is a complex structure composed of multiple subfields (cornu ammonis [CA] areas 1 to 4, dentate gyrus, and subiculum) that are distributed disproportionately along its longitudinal axis (head, body, tail) ( 45 , 46 ). These regions show protracted development as a result of prolonged neurogenesis, synaptic growth, dendritic arborization, pruning, vascularization, and myelination ( 47 – 49 ). Data from nonhuman primates (e.g., ref. 50 ) and human children (e.g., ref. 51 ) suggest that the developmental trajectory of these subfields and their connectivity with each other are related to age-related improvements in memory, which is consistent with theoretical proposals of brain and memory development ( 52 ). Specifically, within the hippocampus, although immature cells continue to accrue within the dentate gyrus throughout the first year of postnatal life [and may be related to the onset of other cognitive abilities, such as spatial navigation ( 50 )], elevated rates of dendritic development and synapse formation persist until at least 5 y of age ( 53 , 54 ). During early childhood (∼3 to 5 y of age), neuronal connections between granule cells of the dentate gyrus and pyramidal neurons of Ammon’s horn form, which alter the functional circuits of the hippocampus ( 54 ) and regions located downstream from the dentate gyrus, particularly CA3 ( 50 ). Because circuitry in the dentate gyrus is critical for adult-like memory formation, its protracted developmental profile suggests that adult-like memory formation in humans may not be expected before 5 y, as morphological development is likely correlated with functional capability ( 54 ).
Prolonged development of memory due to the long maturational time line of neural circuitry is supported by behavioral research. Specifically, as children mature, they are better able to remember individual items as well as associations between items ( 55 , 56 ). In fact, children’s ability to remember such details shows accelerated rates of change between 5 and 7 y of age ( 55 ). These findings fit well with cross-sectional research suggesting that children’s ability to bind information increases between 4 and 6 y of age (e.g., refs. 57 – 59 ). Moreover, these improvements in memory are associated with variations in hippocampal development (as indexed by volume). Across two different studies and two different memory paradigms, brain–behavior relations have been found to change around 6 y of age—following the final nap transition. Specifically, in younger (4- to 6-y) children, better memory performance is associated with larger hippocampal subfield volumes, whereas the opposite is true in older (6- to 8-y) children (i.e., better memory performance is associated with smaller volumes). This difference in brain–behavior relations may be the result of synaptic pruning within the dentate gyrus → CA3 → CA1 circuit, which is critical for binding and ultimately, yields a more efficient system ( 50 , 54 ).
In summary, ample evidence supports the role of the hippocampus (and its associated neocortical network) in learning and memory even from an early age [e.g., infants 3 to 24 mo ( 60 )]. Critical to the present hypothesis, across early childhood, the hippocampus is learning to learn in conjunction with protracted development, a challenge that we posit is met by naps ( Fig. 2 ).

Growth trajectories across nap transitions (gray shading). Synaptic density [prefrontal cortex ( 113 )], hippocampal volume ( 114 , 115 ), cortical gray matter ( 116 ), and SWA ( 117 ) are illustrated.
3.2. SWA Supports Hippocampal-Dependent Memory.
3.2.1. evidence of swa-related memory processing in adults..
Memories from the day benefit from subsequent sleep. Recall is better following sleep compared with an equivalent interval awake, with memories protected by the sleep interval compared with the decay that typically occurs over wake. For example, in one study, adults retained memory for more word pairs following an interval with sleep compared with an equivalent interval awake ( 61 ). Notably, memory retention was associated with time spent in slow-wave sleep, suggesting an active role of this sleep stage in memory processing as opposed to passive protection of memories during the sleep interval as a whole. Further support for the role of slow-wave sleep is provided by studies that represent auditory or odor cues associated with learned material during subsequent slow-wave sleep, which results in enhanced consolidation of cue-related material ( 62 ). Conversely, when SWA, the primary spectral power of slow-wave sleep, is reduced, performance benefits following sleep are absent ( 63 ). Such results support that consolidation of memories is enhanced by sleep and that SWA in particular supports memory consolidation.
Notably, not all memories benefit from sleep. Rather, engagement of the hippocampus during encoding seems to be a necessary condition for sleep-dependent memory consolidation ( 64 – 66 ). For example, memory for novel objects alone is not reliant on the hippocampus, whereas memory requiring binding of an object with a location or ordering with another object does ( 67 ). When rats performed a task requiring memory for novel objects (“what” memory), object location (“where” memory), or temporal order (“when” memory), performance was better following an interval of sleep compared with wake for the object placement and temporal order tasks. Memory for novel objects did not differ for sleep and wake conditions. This is taken as evidence that sleep specifically supports memory for tasks engaging the hippocampus ( 64 ).
Mechanistically, the synaptic homeostasis hypothesis contends that the association between SWA and memory consolidation over the sleep interval reflects synaptic downscaling over sleep, with superfluous synapses (incidental memories) being downscaled, while intentionally learned memories are benefited by reduced signal to noise ( 38 ). According to the active systems consolidation view, synchronous slow waves aligned with hippocampal activity (i.e., hippocampal sharp-wave ripples) support a hippocampal–neocortical shift of the memory representation. Numerous studies in animals have demonstrated that patterns of hippocampal activation associated with a learning episode are seen again in subsequent sleep and not in the prior sleep bouts ( 68 ). Such “replay events” undergo developmental changes early in life ( 69 ) and have been linked with the emergence of episodic-like memory in rodents ( 70 ). The timing of these developmental changes coincides with the transition to monophasic sleep in humans [i.e., roughly during the early childhood years ( 13 – 15 )]. Replay activity is associated with hippocampal ripples, which co-occur with sleep spindles and slow oscillations ( 71 ). Although hippocampal ripples cannot be measured noninvasively in humans, spindle–slow oscillation coupling predicts performance changes over sleep in adults ( 72 , 73 ). Growing support for both the synaptic homeostasis hypothesis and the active systems consolidation view has brought many to agree that these memory-supporting mechanisms in sleep are not mutually exclusive ( 74 , 75 ). Although both of these theories have influenced our thinking, our proposal intentionally does not ascribe to either one specifically, nor is our work intended to provide direct evidence supporting one or the other. Rather, we focus on the importance of sleep for memory—as it both stabilizes existing memories in cortical regions and optimizes synaptic organization ( 76 ), which ultimately frees up the hippocampus for ongoing learning.
3.2.2. Evidence of SWA-related memory processing in children.
Sleep benefits memory consolidation from a very young age. Benefits of sleep relative to wake have been observed in the performance of children ranging from 6 mo through adolescence, particularly for declarative learning tasks (reviewed in ref. 77 ). Under normal conditions, motor procedural learning does not benefit from sleep in childhood. However, when children receive additional training, performance improves following sleep relative to wake ( 78 ), which may suggest that, like adults, hippocampal engagement with encoding may be essential to sleep-dependent memory consolidation. Moreover, a study in toddlers supports that hippocampal-based memories are reactivated during a nap even in children as young as 2 y ( 79 ), providing evidence that the hippocampus is functional during sleep and directly related to memory at an early age.
Interestingly, SWA in children’s naps predicts the overnap protection of memory for emotional faces ( 80 ) and also, predicts reductions in the emotional attention bias following a nap in young children ( 81 ). We posit that consolidation of emotional memories from the morning decreases emotional load, and as a result, children are less reactive to emotional stimuli thereafter. This provides a potential explanation of the oft observed phenomena that habitually napping children are emotionally dysregulated at the end of the day if they do not nap (the “witching hour”).
Sleep-related declarative memory benefits in children have also been associated with sleep spindles and accompanying slow oscillations in children 3 to 5 y ( 82 , 83 ). Moreover, a longitudinal study of older children (9 y) who were tested subsequently at 16 y of age showed that developmental increases in coupling strength between spindles and slow oscillations provided a strong predictor of developmental changes in sleep-related benefits on a word-pair learning task ( 84 ). This provides additional support for the cortical stabilization of memories, initially encoded in the hippocampus, in the cortex during sleep and the theory that this process is present in children and strengthens with development.
Notably, whether such benefits reflect hippocampal–neocortical transition of memory representations as proposed by the active systems consolidation theory, cortical stabilization via synaptic downscaling as proposed by the synaptic homeostasis hypothesis, or both is unknown. It has been hypothesized that active systems consolidation would be unlikely before 18 to 24 mo due to underdevelopment of the hippocampus ( 85 ). Although few studies in this young age group have included polysomnography ( 77 ), there is some evidence that sleep-dependent memory processing is associated with sleep spindles, even at this young age. For instance, 9- and 16-mo-old infants who learned word–object pairs prior to a nap or wake interval generalized to category exemplars only after sleep. Notable here is that nap-related generalization was associated with the EEG sigma power, the frequency range of sleep spindles (10 to 15 Hz) ( 86 , 87 ). This lends support that key brain areas for active systems consolidation may be sufficiently developed in infancy ( 60 ). An alternative is that synaptic downscaling oversleep accounts for memory benefits at a very young age, while active systems consolidation accounts for sleep-related benefits later on ( 85 ). Importantly, the present hypothesis can accommodate either mechanism.
3.3. Evidence Supporting the Relation between Brain Development and Nap Transitions.
Support for the present hypothesis can be drawn from the coincident development of brain, memory, and sleep described above. Additionally, a growing number of behavioral and neural developmental studies provide more direct support for the relation between brain development and nap transitions, although to date, this work has been focused on the biphasic to monophasic sleep transition.
3.3.1. Behavioral studies.
At a behavioral level, support for relations between brain development and nap transitions can be drawn from studies comparing cognitive performance in habitually and nonhabitually napping children who are the same age. In one such study, children who performed better on a cognitive battery were found to take fewer naps than those with lower performance ( 88 ). In particular, fewer naps corresponded to enhanced memory span for auditory number sequences and larger vocabulary. One interpretation of this result is that those who nap have insufficient overnight sleep (supported by a negative correlation between nighttime sleep and nap length), and this may explain both lower cognitive performance and nap frequency. However, an alternative is that nap habituality is related to brain maturation; children who have more mature brains need to nap less often and also, perform better on cognitive assessments.
Supporting the latter interpretation, our recent study provided experimental evidence of differences in memory performance around the nap transition. In this study, we taught habitually napping and nonhabitually napping children a visuospatial task prior to an afternoon nap and again prior to an equivalent interval awake (within subjects) ( 82 ). Memory for item locations was probed again after the nap or wake interval and once more the following morning. We found that memories were protected by the nap; accuracy following the nap did not differ from immediate recall. However, when children stayed awake during the nap opportunity, recall accuracy was reduced by ∼12% compared with immediate recall. The benefit of the nap remained when performance was assessed again the following morning. We then considered whether the nap benefit varied for children who napped habitually (greater than or equal to five naps per week) compared with those who no longer napped (zero to time per week but were nap promoted for the experiment). Memory consolidation over the nap did not differ for habitually and nonhabitually napping children; naps protected memories regardless of nap habituality. Rather, what differed was how much memories decayed over the waking interval; memory decay over an afternoon awake was greater for children who napped habitually and minimal for those who no longer napped, even when controlling for age. We interpret this as evidence that in habitual nappers, sleep needs to occur more frequently in order to prevent the catastrophic interference between memories that occurs when kept awake. Nonhabitual nappers, on the other hand, may have more developed memory storage and thus, be able to hold memories for longer without interference. Subsequently, other studies have found similar differences between habitually and nonhabitually napping children with a word-learning task ( 89 ) and an emotional face-learning task ( 80 ). Collectively, these studies provide evidence that naps are similarly beneficial regardless of nap habituality but that memory of habitually napping children is much more damaged by a missed nap compared with nonhabitually napping children.
3.3.2. Neural studies.
At a neural level, SWA, the marker of the accumulation of sleep pressure that contributes to naps ( 36 , 90 ), has also been related to brain development (as reviewed in ref. 91 ). Slow-wave amplitude increases during childhood and is highest shortly before puberty. This parallels findings of developmental changes in synaptic density ( Fig. 2 ) ( 92 ). SWA is highly predictive of decreases in gray matter volume, a relation that is strongest in areas undergoing maturation at this age ( 93 ). In addition, the distribution (or topography) of SWA also tracks with the development of underlying cortical areas ( 93 , 94 ). Specifically, SWA, which peaks maximally over occipital regions in younger children, shifts to a peak over frontal regions by adolescence, a trajectory that mirrors that of cortical maturation [cortical thickness ( 93 , 94 )] and is predictive of brain myelin ( 95 ). Taken together, these studies support a link between brain development and global SWA in early childhood.
Finally and more directly, we recently reported a difference in hippocampal volume for habitually and nonhabitually napping children. We compared hippocampal subfield volumes in 4- to 6-y-old children who napped habitually with those who did not nap habitually. Habitually napping children had larger CA1 hippocampal subfield volumes in the hippocampal body compared with nonnapping children ( 96 ). This study provides the first direct evidence for a difference in the hippocampus between habitually napping and nonhabitually napping children that cannot be accounted for by age. Critically, prior reports on this same sample of children have linked volume of the CA1 to children’s memory performance, and this region also shows developmental change across the early childhood period ( 51 , 97 ). Most germane to our hypothesis is the finding that across all children in the study (4 to 8 y, regardless of nap status), smaller CA1 was associated with better memory performance ( 53 ).
3.4. Summary, Caveats, and Future Research Directions.
Collectively, we provide support for a relation between nap transitions and underlying memory and brain development. Together with studies relating nap habituality to SWA and memory to SWA, we provide a parsimonious hypothesis suggesting that maturation of the hippocampal-dependent memory network during early childhood results in more efficient memory storage, which reduces the buildup of homeostatic sleep pressure and in turn, contributes to nap transitions ( Fig. 1 B ).
However, there are some caveats given the limited body and nature of the current literature. First, much of the data supporting our hypothesis lack causality. While we posit that reduced need for memory consolidation is related to changing accumulation of sleep pressure (and related SWA), whether these are related directly (e.g., SWA changes could be related to simultaneous development in cortical regions in the hippocampal–memory network where SWA may be generated) or indirectly (e.g., less adenosine accumulation in the developing hippocampus benefiting memory and less adenosine accumulation in the cortex reducing SWA) is important to consider. We also interpret associations between the development of the hippocampal-dependent memory network and sleep, particularly nap habituality, to indicate that development of the brain drives nap habituality. An alternative developmental time line is that changes in sleep are a necessary precursor to brain development. Indeed, changes in SWA have been shown to precede improvements in some motor skills, which in turn, preceded decreases in gray matter in a cross-sectional sample of those 2 to 26 y of age ( 98 ). It will also be important to consider the development of other brain regions associated with the bioregulation of sleep. For example, hypothalamic development may follow a similar time line ( 99 ). Although this is unlikely to account for the differences in behavior of habitual and nonhabitual nappers described above, it will be important for future work to consider interactions in the development of multiple brain areas, which may support the bioregulation of sleep in early development and their causal role in nap habituality.
Second, while we posit that brain and memory development may underlie the transition from triphasic to biphasic sleep, current data in support of the hypothesis are based on the biphasic to monophasic sleep transition. Moreover, the present hypothesis is based on mostly cross-sectional data supporting individual relationships between sleep–brain, sleep–memory, or brain–memory. It is essential to acquire longitudinal data that capture sleep physiology, structural and functional brain development, and memory changes across the nap transitions, starting at 12 mo to capture both critical transitions, within a single sample that is large enough to overcome individual differences and tease apart general maturation from nap-related change. Such longitudinal data can be probed using latent change score modeling to assess the relations between these factors. Latent change score models evaluate ways in which variables are recursively associated over time in order to isolate temporal components of change within a person or group in order to specify lead vs. lag ( 100 ). For example, latent change score modeling has been used successfully in studies of memory decline to better understand the dynamics among processes, including changes at the neural level (e.g., ref. 101 ).
Third, there is considerable variability in the experimental methods used to examine the impact of sleep on memory early in life. This variation applies to the stimuli used (words vs. pictures), age ranges explored, and durations over which information is retained, as well as other factors. These differences likely contribute to the lack of consensus in results. For example, one study that used a verb-learning task, which required generalization to a novel exemplar, failed to find a difference in performance between habitual and nonhabitual nappers following intervals of wake and sleep (which benefited both groups) ( 102 ). However, it is possible that measurement limitations (as change in performance over the interval cannot be assessed) or other task differences may account for these discrepancies. Moreover, there are clear task nuances that can vary sleep’s function in memory consolidation even in the adult literature ( 103 , 104 ). Better understanding of these tasks and related neural underpinnings is essential for a mechanistic understanding of nap function and nap transitions. Thus, it is important for future work to also consider memory consolidation with various types of tasks as it relates to brain development.
Finally, several factors contribute to the presence of naps. Cultural (including parenting practices) and environmental factors likely play a role ( 105 – 107 ). Although many of these are related to circadian and homeostatic sleep regulation ( section 2 ), many other factors could be considered [e.g., diet, illness ( 108 )] that are likely to contribute to the presence of naps but not the persistence of naps and nap transitions. Importantly, while we posit that brain maturation is a strong factor underlying nap transitions, these factors are not mutually exclusive [e.g., environmental factors can also contribute to brain development ( 109 )]. Moreover, whether other species demonstrate similar nap transitions and factors that may affect these transitions have received little attention and would contribute greatly to a broader understanding of sleep regulation. Identifying factors that moderate or interact with brain development and sleep is an important goal for future research.
4. Conclusions and Implications
Here, we present a first review of the bioregulatory processes that contribute to the napping patterns of young children. Every young child naps and transitions out of naps at some point in early childhood—but the age at which this transition takes places varies dramatically ( 14 , 15 , 110 ).
Better understanding nap transitions would allow educators and caregivers to support these transitions, thereby strengthening children’s health and cognition. Scientific evidence showing that nap transitions are a product of brain development that is quite variable between individual children would help parents and providers appreciate that nap transitions cannot be determined by age and that the opportunity to nap should be protected for those that need it. This would also have substantial policy implications for early education, suggesting that nap opportunities should not only be protected for some children but actively supported and of sufficient length. Moreover, understanding typical sleep development is essential to identifying disorders of sleep as well as the extent of the impact associated with insufficient sleep. Thirty percent of children aged 6 to 11 y have insufficient sleep ( 111 ). Unfortunately, the extent of this deficiency in younger children is unclear due to a dearth of data on sleep in early childhood ( 1 ). This is egregiously problematic as younger children are at greater risk for problems stemming from insufficient sleep due to their immature brain development. Moreover, sleep is particularly reduced in low-income and atypically developing children ( 112 ) of this age and thus, contributes to known health disparities that are widespread and have far-reaching impacts. Given the prevalence of naps, the importance of naps to early cognition, and the policy ramifications, a scientific understanding is essential, as these periods may provide unique windows of opportunity to promote healthy development and optimize cognitive abilities.
Acknowledgments
Support for this work is provided by NSF Grant BCS 1749280 (to R.M.C.S. and T.R.), NIH Grant R21 HD094758 (to R.M.C.S. and T.R.), and NIH Grant R01 HL111695 (to R.M.C.S.). We thank Dr. Marc Weissbluth for dialogue and feedback on this work and Neely Miller, Gina Mason, Bethany Jones, Tamara Allard, and our laboratory members for feedback.
The authors declare no competing interest.
This article is a PNAS Direct Submission. K.S. is a guest editor invited by the Editorial Board.
Data Availability

IMAGES
VIDEO
COMMENTS
Advances in research on memory and sleep can thus shed light on how this processing influences our waking life, which can further inspire the development of novel strategies for decreasing detrimental rumination-like activity during sleep and for promoting beneficial sleep cognition. Keywords: learning, ...
There is a growing body of evidence suggesting a critical role of sleep in learning and memory (Diekelmann & Born, 2010).On the one hand, offline memory consolidation during sleep benefits both declarative and procedural memories acquired during preceding wake (Klinzing et al., 2019).On the other hand, memory encoding capacity has been argued to saturate gradually during wake, with sleep ...
Sleep across the Life Span. One pressing question about the sleep-memory link concerns how it manifests over one's lifetime. Spencer and Riggins examined this link at the younger end of the age spectrum.They review evidence that naps in early childhood are essential for memory consolidation, presenting a fascinating new hypothesis connecting the psychological, physiological, and ...
Over more than a century of research has established the fact that sleep benefits the retention of memory. In this review we aim to comprehensively cover the field of "sleep and memory" research by providing a historical perspective on concepts and a discussion of more recent key findings. Whereas initial theories posed a passive role for sleep enhancing memories by protecting them from ...
Consolidation during sleep not only strengthens memory traces quantitatively but can also produce qualitative changes in memory representations. An active process of re-organization enables the ...
One pressing question about the sleep-memory link concerns how it manifests over one's lifetime. Spencer and Riggins examined this link at the younger end of the age spectrum.They review evidence that naps in early childhood are essential for memory consolidation, presenting a fascinating new hypothesis connecting the psychological, physiological, and neurobiological changes that accompany ...
However, sleep and memory research lags behind other subfields of psychology and cognitive neuroscience in adopting open science practices 176, despite positive examples emerging 177,178,179.
Decades of research have sought to connect large-scale patterns of brain activity during sleep to mechanisms of memory storage. Many of the findings suggest that sleep plays an active role in revisiting waking experiences and consolidating them into long-term memories ( 2 ).
Over more than a century of research has established the fact that sleep benefits the retention of memory. In this review we aim to comprehensively cover the field of "sleep and memory" research by providing a historical perspective on concepts and a discussion of more recent key findings. Whereas initial theories posed a passive role for sleep ...
Walker and colleagues review neuroimaging studies on the consequences of sleep deprivation on cognition and emotion — with specific focuses on attention and working memory, positive and negative ...
A study in a 2018 issue of the Journal of Sleep Research, for example, indicates that one night of sleep loss can impair working memory, which is important for reasoning and planning.
Introduction. Several studies primarily in adults have shown that sleep improves procedural memory, i.e. skills and procedures [1], [2] as well as declarative memory [3]. REM and slow-wave sleep (SWS) have been implicated in memory consolidation [3] - [5]. Lack of REM sleep is associated with poor recall of visual location [6].
Sleep's Role in Memory Consolidation . Sleep and memory Trusted Source National Institutes of Health (NIH) The NIH, a part of the U.S. Department of Health and Human Services, is the nation's medical research agency — making important discoveries that improve health and save lives. View Source share a complex relationship. Getting enough rest helps you process new information Trusted ...
Sleep provides a window of opportunity for the brain to sort and reinforce newly encoded memories in absence of the incessant barrage of external information. This process, called "consolidation", leads to the generation of long-lasting "memory traces" or "engrams" whose activation during wakefulness supports the recall of information.
Recent research has led scientists to hypothesize that Stage 3 (deep non-Rapid Eye Movement sleep, or Slow Wave Sleep) may be especially important for the improvement of memory retention and recall [2]. How does sleep improve long-term memory? Scientists hypothesize that sleep also plays a major role in forming long-term memories.
Poor sleep impairs memory consolidation by disrupting the normal process that draws on both NREM and REM sleep for building and retaining memories. ... Ganesan, S., Leong, R. L., & Chee, M. W. (2016). Sleep deprivation increases formation of false memory. Journal of sleep research, 25(6), 673-682.
Research suggests that sleep deprivation both before and after encoding has a detrimental effect on memory for newly learned material. However, there is as yet no quantitative analyses of the size of these effects. We conducted two meta-analyses of studies published between 1970 and 2020 that investigated effects of total, acute sleep deprivation on memory (i.e., at least one full night of ...
Sleep and brain/cognitive/neural reserve significantly impact well-being and cognition throughout life. This review aims to explore the intricate relationship between such factors, with reference to their effects on human cognitive functions. The specific goal is to understand the bidirectional influence that sleep and reserve exert on each other. Up to 6 February 2024, a methodical search of ...
Even after the sleep-deprived mice were allowed to sleep again, their SWRs never quite reached the strength and consistency found in mice that had normal sleep. The results in this study further demonstrate how critical sleep is for memory and suggest that long term sleep deprivation could have a lasting effect on memory.
healthy sleep helps to consolidate new memories, and that this pro-cess is impaired for procedural memories in patients with primary insomnia (Nissen et al., 2011). Sleep quality and memory can be assessed in a number of ways. One way that sleep quality has been measured in previous research is with a Basic Mini-Motionlogger actigraph, a device
Sleep deficit has been associated with lack of concentration and attention during class. 19 While a few studies report null effects, 20,21 most studies looking at the effects of sleep quality and ...
Sleep also is important to the ability to recall memories. Research indicates that recall of both short- and long-term memory is impaired by lack of sleep. A sleep-deprived brain is less effective ...
Research has also shown that sleep strengthens memory for the most important aspects of an experience, restructures our memories to form more cohesive narratives and helps us come up with ...
For example, previous research on sleep and memory emphasized comparisons between sleep and wakeful retention intervals to draw inferences about sleep's influence. The waking condition could consist of an ordinary period of wakefulness or a night of total sleep deprivation. ... Sleep memory processing: the sequential hypothesis. Frontiers in ...
Lack of sleep is known to affect the brain, including memory, in mice and in humans, but research is beginning to show that these memories are not lost, they are just 'hidden' in the brain and ...
Exploring LAUREATE - the Longitudinal multimodAl stUdent expeRience datasEt for AffecT and mEmory Research. In Adjunct Proceedings of the 2022 ACM International Joint Conference on Pervasive and Ubiquitous Computing and the 2022 ACM International Symposium on Wearable Computers (UbiComp/ISWC '22 Adjunct).
Contemporary models propose that memory consolidation, i.e., the strengthening of memories during sleep, is achieved in part by reactivating experiences that were encoded during prior wakefulness ...
Scientists have shown that deep brain stimulation during sleep can help people retain new information. The approach could help people with memory problems related to disorders like Alzheimer's.
Central to our research is that these sleep transition periods are interesting windows of change in brain and memory development. 2. ... we focus on the importance of sleep for memory—as it both stabilizes existing memories in cortical regions and optimizes synaptic organization , which ultimately frees up the hippocampus for ongoing learning.
We are pleased to announce that Kristine Wilckens, PhD, has been promoted to Associate Professor of Psychiatry by the University of Pittsburgh School of Medicine. Dr. Wilckens received her PhD in cognitive psychology from the University of Pittsburgh, and completed postdoctoral research training in the Department's National Institute of Mental Health (NIMH)-funded Clinical and Translational ...