Automatic Speech Recognition: Systematic Literature Review
Ieee account.
- Change Username/Password
- Update Address

Purchase Details
- Payment Options
- Order History
- View Purchased Documents
Profile Information
- Communications Preferences
- Profession and Education
- Technical Interests
- US & Canada: +1 800 678 4333
- Worldwide: +1 732 981 0060
- Contact & Support
- About IEEE Xplore
- Accessibility
- Terms of Use
- Nondiscrimination Policy
- Privacy & Opting Out of Cookies
A not-for-profit organization, IEEE is the world's largest technical professional organization dedicated to advancing technology for the benefit of humanity. © Copyright 2024 IEEE - All rights reserved. Use of this web site signifies your agreement to the terms and conditions.
Subscribe to the PwC Newsletter
Join the community, add a new evaluation result row, speech recognition.
1104 papers with code • 234 benchmarks • 87 datasets
Speech Recognition is the task of converting spoken language into text. It involves recognizing the words spoken in an audio recording and transcribing them into a written format. The goal is to accurately transcribe the speech in real-time or from recorded audio, taking into account factors such as accents, speaking speed, and background noise.
( Image credit: SpecAugment )

Benchmarks Add a Result

Most implemented papers
Listen, attend and spell.

Unlike traditional DNN-HMM models, this model learns all the components of a speech recognizer jointly.
Deep Speech 2: End-to-End Speech Recognition in English and Mandarin

We show that an end-to-end deep learning approach can be used to recognize either English or Mandarin Chinese speech--two vastly different languages.
Communication-Efficient Learning of Deep Networks from Decentralized Data
Modern mobile devices have access to a wealth of data suitable for learning models, which in turn can greatly improve the user experience on the device.
Speech Commands: A Dataset for Limited-Vocabulary Speech Recognition
Describes an audio dataset of spoken words designed to help train and evaluate keyword spotting systems.
SpecAugment: A Simple Data Augmentation Method for Automatic Speech Recognition
On LibriSpeech, we achieve 6. 8% WER on test-other without the use of a language model, and 5. 8% WER with shallow fusion with a language model.
Deep Speech: Scaling up end-to-end speech recognition
We present a state-of-the-art speech recognition system developed using end-to-end deep learning.
Conformer: Convolution-augmented Transformer for Speech Recognition
Recently Transformer and Convolution neural network (CNN) based models have shown promising results in Automatic Speech Recognition (ASR), outperforming Recurrent neural networks (RNNs).
wav2vec 2.0: A Framework for Self-Supervised Learning of Speech Representations
We show for the first time that learning powerful representations from speech audio alone followed by fine-tuning on transcribed speech can outperform the best semi-supervised methods while being conceptually simpler.
Recurrent Neural Network Regularization
wojzaremba/lstm • 8 Sep 2014
We present a simple regularization technique for Recurrent Neural Networks (RNNs) with Long Short-Term Memory (LSTM) units.
Split Computing and Early Exiting for Deep Learning Applications: Survey and Research Challenges
Mobile devices such as smartphones and autonomous vehicles increasingly rely on deep neural networks (DNNs) to execute complex inference tasks such as image classification and speech recognition, among others.
Automatic Speech Emotion Recognition: a Systematic Literature Review
- Published: 07 April 2024
- Volume 27 , pages 267–285, ( 2024 )
Cite this article
- Haidy H. Mustafa ORCID: orcid.org/0009-0006-3490-8596 2 ,
- Nagy R. Darwish 2 &
- Hesham A. Hefny 1
171 Accesses
Explore all metrics
Automatic Speech Emotion Recognition (ASER) has recently garnered attention across various fields including artificial intelligence, pattern recognition, and human–computer interaction. However, ASER encounters numerous challenges such as a shortage of diverse datasets, appropriate feature selection, and suitable intelligent recognition techniques. To address these challenges, a systematic literature review (SLR) was conducted following established guidelines. A total of 60 primary research papers spanning from 2011 to 2023 were reviewed to investigate, interpret, and analyze the related literature by addressing five key research questions. Despite being an emerging area with applications in real-life scenarios, ASER still grapples with limitations in existing techniques. This SLR provides a comprehensive overview of existing techniques, datasets, and feature extraction tools in the ASER domain, shedding light on the weaknesses of current research studies. Additionally, it outlines a list of limitations for consideration in future work.
This is a preview of subscription content, log in via an institution to check access.
Access this article
Price includes VAT (Russian Federation)
Instant access to the full article PDF.
Rent this article via DeepDyve
Institutional subscriptions
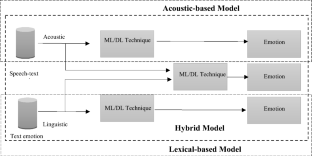
Similar content being viewed by others
A Survey of Human Emotion Recognition Using Speech Signals: Current Trends and Future Perspectives
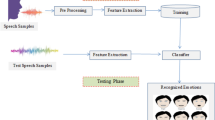
Speech Emotion Recognition Systems: A Comprehensive Review on Different Methodologies
Speech emotion recognition research: an analysis of research focus, data availability.
Most of the datasets and tools presented in this study are available on the internet.
“audeering,” audEERING ® (2023). Retrieved May 23, 2023, from https://www.audeering.com/research/opensmile/
Abdel-Hamid, L. (2020). Egyptian Arabic speech emotion recognition using prosodic, spectral and wavelet features. Speech Communication, 122 , 19–30.
Article Google Scholar
Aldeneh, Z., & Provost, E. M. (2017). Using regional saliency for speech emotion recognition. In 2017 IEEE international conference on acoustics, speech and signal processing (ICASSP) , New Orleans, LA, USA.
Al-Faham, A., & Ghneim, N. (2016). Towards enhanced Arabic speech emotion recognition: Comparison between three methodologies. Asian Journal of Science and Technology, 7 (3), 2665–2669.
Google Scholar
AL-Sarayreh, S., Mohamed, A., & Shaalan, K. (2023). Challenges and solutions for Arabic natural language processing in social media. In Hassanien, A.E., Zheng, D., Zhao, Z., & Fan, Z. (Eds) Business intelligence and information technology. 2022 . Smart innovation, systems and technologies 358. Springer. https://doi.org/10.1007/978-981-99-3416-4_24
An, X. D., & Ruan, Z. (2021). Speech emotion recognition algorithm based on deep learning algorithm fusion of temporal and spatial features. Journal of Physics: Conference Series, 1861 (1), 012064.
Anusha, R., Subhashini, P., Jyothi, D., Harshitha, P., Sushma, J., & Mukesh, N. (2021). Speech emotion recognition using machine learning. In 2021 5th international conference on trends in electronics and informatics (ICOEI) , Tirunelveli, India.
Aouani, H., & Ayed, Y. B. (2020). Speech emotion recognition using deep learning. In 24th international conference on knowledge-based and intelligent information & engineering , Verona, Italy.
Atmaja, B. T., & Sasou, A. (2022a). Evaluating self-supervised speech representations for speech emotion recognition. IEEE Access, 10 , 124396–124407.
Atmaja, B. T., & Sasou, A. (2022b). Effects of data augmentations on speech emotion recognition. Sensors (Basel), 22 (16), 5941.
Atmaja, B. T., & Sasou, A. (2022c). Sentiment analysis and emotion recognition from speech using universal speech representations. Sensors, 22 (17), 6369.
Atmaja, B. T., Shirai, K., & Akagi, M. (2019). Speech emotion recognition using speech feature and word embedding. In Pacific signal and information processing association annual summit and conference (APSIPA ASC) , Lanzhou, China.
Badshah, A. M., Ahmad, J., Rahim, N., & Baik, S. W. (2017). Speech emotion recognition from spectrograms with deep convolutional neural network. In 2017 international conference on platform technology and service (PlatCon) , Busan, Korea (South).
Bertero, D., & Fung, P. (2017). A first look into a convolutional neural network for speech emotion detection. In IEEE international conference on acoustics, speech and signal processing (ICASSP) , New Orleans, LA, USA.
Bojanić, M., Delić, V., & Karpov, A. (2020). Call redistribution for a call center based on speech emotion recognition. Applied Sciences, 10 (13), 4653.
Cho, J., & Kato, S. (2011). Detecting emotion from voice using selective Bayesian pairwise classifiers. In 2011 IEEE symposium on computers & informatics , Kuala Lumpur, Malaysia.
Dangol, R., Alsadoon, A., Prasad, P. W. C., Seher, I., & Alsadoon, O. H. (2020). Speech emotion recognition usingconvolutional neural network and long-short term memory. Multimedia Tools and Applications, 79 , 32917–32934.
Dasgupta, P. B. (2017). Detection and analysis of human emotions through voice and speech pattern processing. International Journal of Computer Trends and Technology (IJCTT), 52 (1), 1–3.
Deng, J., Xu, X., Zhang, Z., Frühholz, S., & Schuller, B. (2017). Semisupervised autoencoders for speech emotion recognition. IEEE/ACM Transactions on Audio, Speech, and Language Processing, 26 (1), 31–43.
Dennis, J., Dat, T. H., & Li, H. (2011). Spectrogram image feature for sound event classification in mismatched conditions. Signal Processing Letters, 18 (2), 130–133.
Dissanayake, V., Zhang, H., Billinghurst, M., & Nanayakkara, S. (2020). Speech emotion recognition ‘in the wild’ using an Autoencoder. In INTERSPEECH 2020 , Shanghai, China.
Er, M. B. (2020). A novel approach for classification of speech emotions based on deep and acoustic features. IEEE Access, 8 , 221640–221653.
Eskimez, S. E., Imade, K., Yang, N., Sturge-Apple, M., Duan, Z., & Heinzelman, W. (2016). Emotion classification: How does an automated system compare to Naive human coders? In IEEE international conference on acoustics, speech and signal processing (ICASSP) , Shanghai, China.
Etienne, C., Fidanza, G., Petrovskii, A., Devillers, L., & Schmauch, B. (2018). CNN+LSTM architecture for speech emotion recognition with data augmentation. In Workshop on speech, music and mind (SMM 2018) , Hyderabad, India.
Evgeniou, T. P. M. (2001). Machine learning and its applications. In Support vector machines: Theory and applications (ACAI 1999) . Lecture notes in computer science, (vol. 2049). Springer.
Feugère, L., Doval, B., & Mifune, M.-F. (2015). Using pitch features for the characterization of intermediate vocal productions. In 5th international workshop on folk music analysis (FMA) , Paris.
Flower, T. M. L., & Jaya, T. (2022). Speech emotion recognition using Ramanujan Fourier transform. Applied Acoustics, 201 , 109133.
Gamage, K. W., Sethu, V., & Ambikairajah, E. (2017). Salience based lexical features for emotion recognition. In 2017 IEEE international conference on acoustics, speech and signal processing (ICASSP 2017) , New Orleans, LA, USA,
Ghosh, A., Sufian, A., Sultana, F., Chakrabarti, A., & De, D. (2020). Fundamental concepts of convolutional neural network. In Recent trends and advances in artificial intelligence and Internet of Things. Intelligent systems reference . Springer.
“Google Cloud”. Retrieved May 23, 2023, from https://cloud.google.com/speech-to-text/?utm_source=google&utm_medium=cpc&utm_campaign=emea-eg-all-en-dr-bkws-all-all-trial-e-gcp-1011340&utm_content=text-ad-none-any-DEV_c-CRE_495056377393-ADGP_Hybrid%20%7C%20BKWS%20-%20EXA%20%7C%20Txt%20~%20AI%20%26%20M
Gupta, P., & Rajput, N. (2007). Two-stream emotion recognition for call center monitoring. In INTERSPEECH , Antwerp, Belgium.
Hadjadji, I., Falek, L., Demri, L., & Teffahi, H. (2019). Emotion recognition in Arabic speech. In International conference on advanced electrical engineering (ICAEE) , Algiers, Algeria.
Jithendran, A., Pranav Karthik, P., Santhosh, S., & Naren, J. (2020). Emotion recognition on e-learning community to improve the learning outcomes using machine learning concepts: A pilot study . Springer.
Book Google Scholar
Kacur, J., Puterka, B., Pavlovicova, J., & Oravec, M. (2021). On the speech properties and feature extraction methods in speech emotion recognition. Sensors, 21 (5), 1888.
Kannan, V., & Rajamohan, H. R. (2019). Emotion recognition from speech, vol. 10458. arXiV:abs/1912.
Kanwal, S., Asghar, S., & Ali, H. (2022). Feature selection enhancement and feature space visualization for speech-based emotion recognition. PeerJ Computer Science, 8 , e1091.
Khanna, P., & Sasikumar, M. (2011). Recognizing emotions from human speech. In S. J. Pise (Ed.), Thinkquest . Springer.
Kim, E., & Shin, J. W. (2019). DNN-based emotion recognition based on bottleneck acoustic features and lexical features. In 2019 IEEE international conference on acoustics, speech and signal processing (ICASSP) , Brighton, UK.
Kim, E., Song, H., & Shin, J. W. (2020a). Affective latent representation of acoustic and lexical features for emotion recognition. Sensors (Basel), 20 (9), 2614.
Kim, E., Song, H., & Shin, J. W. (2020b). Affective latent representation of acoustic and lexical features for emotion recognition. Sensors, 20 (9), 2614.
Kitchenham, B., & Charters, S. (2007). Guidelines for performing systematic literature reviews in software engineering version 2.3. Engineering, 45 (4), 1051.
Klaylat, S., Osman, Z., Hamandi, L., & Zantout, R. (2018). Emotion recognition in Arabic speech. Analog Integrated Circuits and Signal Processing, 96 , 337–351.
Le, Q. V. (2015). Autoencoders, convolutional neural networks and recurrent neural networks . Google Inc.
Lee, C. M., & Narayanan, S. S. (2005). Toward detecting emotions in spoken dialogs. IEEE Transactions on Speech and Audio Processing, 13 (2), 293–303.
Lee, Y., Yoon, S., & Jung, K. (2018). Multimodal speech emotion recognition using audio and text. In 2018 IEEE spoken language technology workshop (SLT), Athens, Greece.
Li, B., Dimitriadis, D., & Stolcke, A. (2019). Acoustic and lexical sentiment analysis for customer service calls. In IEEE international conference on acoustics, speech and signal processing (ICASSP) , Brighton, UK.
Li, G. M., Liu, N., & Zhang, J.-A. (2022). Speech emotion recognition based on modified relief. Sensors (Basel), 22 (21), 8152.
Li, Y., Zhang, Y.-T., Ng, G. W., Leau, Y.-B., & Yan, H. (2023). A deep learning method using gender-specific features for emotion recognition: A deep learning method using gender-specific features for emotion recognition. Sensors, 23 (3), 1355–1356.
“librosa”. Retrieved May 23, 2023, from https://librosa.org/doc/latest/index.html
Lieskovska, E., Jakubec, M., & Jarina, R. (2022). RNN with improved temporal modeling for speech emotion recognition. In 2022 32nd international conference radioelektronika (RADIOELEKTRONIKA) , Kosice, Slovakia.
Liu, M. (2022). English speech emotion recognition method based on speech recognition. International Journal of Speech Technology, 25 , 391–398.
Liu, N., Zhang, B., Liu, B., Shi, J., Yang, L., Li, Z., & Zhu, J. (2021). Transfer subspace learning for unsupervised cross-corpus speech emotion recognition. IEEE Access, 9 , 95925–95937.
Lun, X., Wang, F., & Yu, Z. (2021). Human speech emotion recognition via feature selection and analyzing. Journal of Physics Conference Series, 1748 (4), 042008.
Maghilnan, S., & Kumar, M. R. (2017). Sentiment analysis on speaker specific speech data. In 2017 international conference on intelligent computing and control (I2C2) , Coimbatore, India.
Majeed, S. A., Husain, H., Samad, S. A., & Idbeaa, T. F. (2015). Mel frequency cepstral coefficients (MFCC) feature extraction enhancement in theapplication of speech recognition: A comparison study. Journal of Theoretical and Applied Information Technology, 79 (1), 38.
“MathWorks”. Retrieved May 23, 2023, from https://www.mathworks.com/products/matlab.html
Meddeb, M., Karray, H., & Alimi, A. M. (2016). Automated extraction of features from arabic emotional speech corpus. International Journal of Computer Information Systems and Industrial Management Applications, 8 , 184–194.
Mefiah, A., Alotaibi, Y. A., & Selouani, S.-A. (2015). Arabic speaker emotion classification using rhythm metrics and neural networks. In 2015 23rd European signal processing conference (EUSIPCO) , Nice, France.
Meftah, A., Selouani, S.-A., & Alotaibi, Y. A. (2015). Preliminary Arabic speech emotion classification. In 2014 IEEE international symposium on signal processing and information technology (ISSPIT) , Noida, India.
Meftah, A., Qamhan, M., Alotaibi, Y. A., & Zakariah, M. (2020). Arabic speech emotion recognition using KNN and KSU emotions corpus. International Journal of Simulation -- Systems, Science & Technology, 21 (2), 1–5.
Mehmet, B., & Kaya, O. (2020). Speech emotion recognition: Emotional models, databases, features, preprocessing methods, supporting modalities, and classifiers. Speech Communication, 116 , 56–76.
Murugan, H. (2020). Speech emotion recognition using CNN. International Journal of Psychosocial Rehabilitation . https://doi.org/10.37200/IJPR/V24I8/PR280260
Naziya, S., & Ratnadeep, R. D. (2016). Speech recognition system—a review. IOSR Journal of Computer Engineering, 18 (4), 1–9.
Pengfei, X., Houpan, Z., & Weidong, Z. (2020). PAD 3-D speech emotion recognition based on feature fusion. Journal of Physics Conference Series 1616 , 012106.
Płaza, M., Trusz, S., Kęczkowska, J., Boksa, E., Sadowski, S., & Koruba, Z. (2022). Machine learning algorithms for detection and classifications of emotions in contact center applications. Sensors, 22 , 5311.
“python”. Retrieved May 23, 2023, from https://www.python.org/
Rawat, A., & Mishra, P. K. (2015). Emotion recognition through speech using neural network. International Journal of Advanced Research in Computer Science and Software Engineering, 5 (5), 422–428.
Sahu, S., Mitra, V., Seneviratne, S., & Espy-Wilson, C. (2019). Multi-modal learning for speech emotion recognition: An analysis and comparison of ASR outputs with ground truth transcription. In Proceedings of Interspeech (pp. 3302–3306).
Sato, S., Kimura, T., Horiuchi, Y., & Nishida, M. (2008). A method for automatically estimating F0 model parameters and a speech re-synthesis tool using F0 model and STRAIGHT. In INTERSPEECH 2008, 9th annual conference of the international speech communication association , Brisbane, Australia.
Schuller, B., Rigoll, G., &. Manfred, L. (2004). Speech emotion recognition combining acoustic features and linguistic information in a hybrid support vector machine-belief network architecture. In IEEE international conference on acoustics, speech, and signal processing (ICASSP) , Montreal, QC, Canada.
Seknedy, M. E., & Fawzi, S. (2021). Speech emotion recognition system for human interaction applications. In 10th international conference on intelligent computing and information systems (ICICIS) , Cairo, Egypt.
Selvara, M., Bhuvana, R., & Padmaja, S. (2016). Human speech emotion recognition. International Journal of Engineering and Technology (IJET), 8 (1), 311–323.
Shixin, P., Kai, C., Tian, T., & Jingying, C. (2022). An autoencoder-based feature level fusion for speech emotion recognition. Digital Communications and Networks . https://doi.org/10.1016/j.dcan.2022.10.018
Singh, Y. B., & Goel, S. (2022). A systematic literature review of speech emotion recognition approaches. Neurocomputing, 492 , 245–263.
Srivastava, B. M. L., Kajarekar, S., & Murthy, H. A. (2019). Challenges in automatic transcription of real-world phone calls. In Proceedings of Interspeech , Graz, Austria.
Sun, C., Li, H., & Ma, L. (2023). Speech emotion recognition based on improved masking EMD and convolutional recurrent neural network. Frontiers in Psychology, 13 , 1075624.
Sun, L., Fu, S., & Wang, F. (2019). Decision tree SVM model with Fisher feature selection for speech emotion recognition. EURASIP Journal on Audio, Speech, and Music Processing, 2 , 1–14.
Tacconi, D., Mayora, O., Lukowicz, P., Arnrich, B., Setz, C., Troster, G., & Haring, C. (2008). Activity and emotion recognition to support early diagnosis of psychiatric diseases. In International conference on pervasive computing technologies for healthcare .
“The University of Waikato”. Retrieved May 23, 2023, from https://www.cs.waikato.ac.nz/ml/weka/
Trigeorgis, G., Ringeval, F., Brueckner, R., Marchi, E., Nicolaou, M. A., Schuller, B., & Zafeiriou, S. (2016). Adieu features? End-to-end speech emotion recognition using a deep convolutional recurrent network. In IEEE international conference on acoustics, speech and signal processing (ICASSP) , Shanghai, China.
Wani, T. M., Gunawan, T. S., Qadri, S. A. A., Mansor, H., Kartiwi, M., & Ismail, N. (2020). Speech emotion recognition using convolution neural networks and deep stride convolutional neural networks. In 6th international conference on wireless and telematics (ICWT) , Yogyakarta, Indonesia.
“WavePad Audio Editing Software”. Retrieved May 23, 2023, from https://www.nch.com.au/wavepad/index.html
Yang, N., Yuan, J., Zhou, Y., Demirkol, I., Duan, Z., Heinzelman, W., & Sturge-Apple, M. (2017). Enhanced multiclass SVM with thresholding fusion for speech-based emotion classification. International Journal of Speech Technology, 20 , 27–41.
Yazdani, A., Simchi, H., & Shekofteh, Y. (2021). Emotion recognition in persian speech using deep neural networks. In 11th international conference on computer engineering and knowledge (ICCKE) , Mashhad, Iran.
Yu, Y., & Kim, Y.-J. (2020). Attention-LSTM-attention model for speech emotion recognition and analysis of IEMOCAP database. Electronics, 9 (5), 713.
Zhang, Y., & Srivastava, G. (2022). Speech emotion recognition method in educational scene based on machine learning. EAI Endorsed Transactions on Scalable Information Systems, 9 (5), e9.
Zhao, J., Mao, X., & Chen, L. (2018). Speech emotion recognition using deep 1D & 2D CNN LSTM networks. Biomedical Signal Processing and Control, 47 , 312–323.
Zheng, C., Wang, C., & Jia, N. (2020). An ensemble model for multi-level speech emotion recognition. Applied Sciences, 10 (1), 205–224.
ZiaUddin, M., & Nilsson, E. G. (2020). Emotion recognition using speech and neural structured learning to facilitate edge intelligence. Engineering Applications of Artificial Intelligence, 94 , 103775.
Zvarevashe, K., & Olugbara, O. O. (2020). Recognition of speech emotion using custom 2D-convolution neural network deep learning algorithm. Intelligent Data Analysis, 24 (5), 1065–1086.
Download references
Acknowledgements
The authors thank the Associate Editor and the reviewers for their insightful remarks, which greatly improved the paper's clarity.
Not applicable.

Author information
Authors and affiliations.
Computer Science Department, Faculty of Graduate Studies for Statistical Research, Cairo University, Giza, 12613, Egypt
Hesham A. Hefny
Information Systems and Technology Department, Faculty of Graduate Studies for Statistical Research, Cairo University, Giza, 12613, Egypt
Haidy H. Mustafa & Nagy R. Darwish
You can also search for this author in PubMed Google Scholar
Contributions
Haidy was the main contributor in preparing and writing the research. Nagy and Hesham revised the research. Three authors read and revised the research paper.
Corresponding author
Correspondence to Haidy H. Mustafa .
Ethics declarations
Competing interests.
None of the authors have any competing interests.
Additional information
Publisher's note.
Springer Nature remains neutral with regard to jurisdictional claims in published maps and institutional affiliations.
Rights and permissions
Springer Nature or its licensor (e.g. a society or other partner) holds exclusive rights to this article under a publishing agreement with the author(s) or other rightsholder(s); author self-archiving of the accepted manuscript version of this article is solely governed by the terms of such publishing agreement and applicable law.
Reprints and permissions
About this article
Mustafa, H.H., Darwish, N.R. & Hefny, H.A. Automatic Speech Emotion Recognition: a Systematic Literature Review. Int J Speech Technol 27 , 267–285 (2024). https://doi.org/10.1007/s10772-024-10096-7
Download citation
Received : 19 December 2023
Accepted : 18 February 2024
Published : 07 April 2024
Issue Date : March 2024
DOI : https://doi.org/10.1007/s10772-024-10096-7
Share this article
Anyone you share the following link with will be able to read this content:
Sorry, a shareable link is not currently available for this article.
Provided by the Springer Nature SharedIt content-sharing initiative
- Speech recognition
- Speech emotion recognition
- Automatic speech recognition
- Emotional speech
- Find a journal
- Publish with us
- Track your research
speech recognition Recently Published Documents
Total documents.
- Latest Documents
- Most Cited Documents
- Contributed Authors
- Related Sources
- Related Keywords
Improving Deep Learning based Automatic Speech Recognition for Gujarati
We present a novel approach for improving the performance of an End-to-End speech recognition system for the Gujarati language. We follow a deep learning-based approach that includes Convolutional Neural Network, Bi-directional Long Short Term Memory layers, Dense layers, and Connectionist Temporal Classification as a loss function. To improve the performance of the system with the limited size of the dataset, we present a combined language model (Word-level language Model and Character-level language model)-based prefix decoding technique and Bidirectional Encoder Representations from Transformers-based post-processing technique. To gain key insights from our Automatic Speech Recognition (ASR) system, we used the inferences from the system and proposed different analysis methods. These insights help us in understanding and improving the ASR system as well as provide intuition into the language used for the ASR system. We have trained the model on the Microsoft Speech Corpus, and we observe a 5.87% decrease in Word Error Rate (WER) with respect to base-model WER.
Dereverberation of autoregressive envelopes for far-field speech recognition
A computational look at oral history archives.
Computational technologies have revolutionized the archival sciences field, prompting new approaches to process the extensive data in these collections. Automatic speech recognition and natural language processing create unique possibilities for analysis of oral history (OH) interviews, where otherwise the transcription and analysis of the full recording would be too time consuming. However, many oral historians note the loss of aural information when converting the speech into text, pointing out the relevance of subjective cues for a full understanding of the interviewee narrative. In this article, we explore various computational technologies for social signal processing and their potential application space in OH archives, as well as neighboring domains where qualitative studies is a frequently used method. We also highlight the latest developments in key technologies for multimedia archiving practices such as natural language processing and automatic speech recognition. We discuss the analysis of both visual (body language and facial expressions), and non-visual cues (paralinguistics, breathing, and heart rate), stating the specific challenges introduced by the characteristics of OH collections. We argue that applying social signal processing to OH archives will have a wider influence than solely OH practices, bringing benefits for various fields from humanities to computer sciences, as well as to archival sciences. Looking at human emotions and somatic reactions on extensive interview collections would give scholars from multiple fields the opportunity to focus on feelings, mood, culture, and subjective experiences expressed in these interviews on a larger scale.
Contribution of frequency compressed temporal fine structure cues to the speech recognition in noise: An implication in cochlear implant signal processing
Supplemental material for song properties and familiarity affect speech recognition in musical noise, noise-robust speech recognition in mobile network based on convolution neural networks, optical laser microphone for human-robot interaction: speech recognition in extremely noisy service environments, improving low-resource tibetan end-to-end asr by multilingual and multilevel unit modeling.
AbstractConventional automatic speech recognition (ASR) and emerging end-to-end (E2E) speech recognition have achieved promising results after being provided with sufficient resources. However, for low-resource language, the current ASR is still challenging. The Lhasa dialect is the most widespread Tibetan dialect and has a wealth of speakers and transcriptions. Hence, it is meaningful to apply the ASR technique to the Lhasa dialect for historical heritage protection and cultural exchange. Previous work on Tibetan speech recognition focused on selecting phone-level acoustic modeling units and incorporating tonal information but underestimated the influence of limited data. The purpose of this paper is to improve the speech recognition performance of the low-resource Lhasa dialect by adopting multilingual speech recognition technology on the E2E structure based on the transfer learning framework. Using transfer learning, we first establish a monolingual E2E ASR system for the Lhasa dialect with different source languages to initialize the ASR model to compare the positive effects of source languages on the Tibetan ASR model. We further propose a multilingual E2E ASR system by utilizing initialization strategies with different source languages and multilevel units, which is proposed for the first time. Our experiments show that the performance of the proposed method-based ASR system exceeds that of the E2E baseline ASR system. Our proposed method effectively models the low-resource Lhasa dialect and achieves a relative 14.2% performance improvement in character error rate (CER) compared to DNN-HMM systems. Moreover, from the best monolingual E2E model to the best multilingual E2E model of the Lhasa dialect, the system’s performance increased by 8.4% in CER.
Background Speech Synchronous Recognition Method of E-commerce Platform Based on Hidden Markov Model
In order to improve the effect of e-commerce platform background speech synchronous recognition and solve the problem that traditional methods are vulnerable to sudden noise, resulting in poor recognition effect, this paper proposes a background speech synchronous recognition method based on Hidden Markov model. Combined with the principle of speech recognition, the speech feature is collected. Hidden Markov model is used to input and recognize high fidelity speech filter to ensure the effectiveness of signal processing results. Through the de-noising of e-commerce platform background voice, and the language signal cache and storage recognition, using vector graph buffer audio, through the Ethernet interface transplant related speech recognition sequence, thus realizing background speech synchronization, so as to realize the language recognition, improve the recognition accuracy. Finally, the experimental results show that the background speech synchronous recognition method based on Hidden Markov model is better than the traditional methods.
Massive Speech Recognition Resource Scheduling System based on Grid Computing
Nowadays, there are too many large-scale speech recognition resources, which makes it difficult to ensure the scheduling speed and accuracy. In order to improve the effect of large-scale speech recognition resource scheduling, a large-scale speech recognition resource scheduling system based on grid computing is designed in this paper. In the hardware part, microprocessor, Ethernet control chip, controller and acquisition card are designed. In the software part of the system, it mainly carries out the retrieval and exchange of information resources, so as to realize the information scheduling of the same type of large-scale speech recognition resources. The experimental results show that the information scheduling time of the designed system is short, up to 2.4min, and the scheduling accuracy is high, up to 90%, in order to provide some help to effectively improve the speed and accuracy of information scheduling.
Export Citation Format
Share document.
Help | Advanced Search
Electrical Engineering and Systems Science > Audio and Speech Processing
Title: robust speech recognition via large-scale weak supervision.
Abstract: We study the capabilities of speech processing systems trained simply to predict large amounts of transcripts of audio on the internet. When scaled to 680,000 hours of multilingual and multitask supervision, the resulting models generalize well to standard benchmarks and are often competitive with prior fully supervised results but in a zero-shot transfer setting without the need for any fine-tuning. When compared to humans, the models approach their accuracy and robustness. We are releasing models and inference code to serve as a foundation for further work on robust speech processing.
Submission history
Access paper:.
- Other Formats
References & Citations
- Google Scholar
- Semantic Scholar
BibTeX formatted citation

Bibliographic and Citation Tools
Code, data and media associated with this article, recommenders and search tools.
- Institution
arXivLabs: experimental projects with community collaborators
arXivLabs is a framework that allows collaborators to develop and share new arXiv features directly on our website.
Both individuals and organizations that work with arXivLabs have embraced and accepted our values of openness, community, excellence, and user data privacy. arXiv is committed to these values and only works with partners that adhere to them.
Have an idea for a project that will add value for arXiv's community? Learn more about arXivLabs .

IMAGES
VIDEO
COMMENTS
A huge amount of research has been done in the field of speech signal processing in recent years. In particular, there has been increasing interest in the automatic speech recognition (ASR) technology field. ASR began with simple systems that responded to a limited number of sounds and has evolved into sophisticated systems that respond fluently to natural language. This systematic review of ...
ABSTRACT Over the past decades, a tremendous amount of research has been done on the use of machine. learning for speech processing applications, especially speech recognition. However, in the ...
1104 papers with code • 234 benchmarks • 87 datasets. Speech Recognition is the task of converting spoken language into text. It involves recognizing the words spoken in an audio recording and transcribing them into a written format. The goal is to accurately transcribe the speech in real-time or from recorded audio, taking into account ...
Recently, the speech community is seeing a significant trend of moving from deep neural network based hybrid modeling to end-to-end (E2E) modeling for automatic speech recognition (ASR). While E2E models achieve the state-of-the-art results in most benchmarks in terms of ASR accuracy, hybrid models are still used in a large proportion of commercial ASR systems at the current time. There are ...
Speech Recognition is a t echnology with the help of which a machine can. acknowledge the spoken word s and phrases, which can further be used to. generate text. Speech Recognition System works ...
1. Introduction. Automatic Speech Recognition (ASR) converts speech signals to corresponding text via algorithms. This paper examines the history of ASR research, exploring why many ASR design choices were made, how ASR is currently done, and which changes may achieve significantly better results.
We present a state-of-the-art speech recognition system developed using end-to-end deep learning. Our architecture is significantly simpler than traditional speech systems, which rely on laboriously engineered processing pipelines; these traditional systems also tend to perform poorly when used in noisy environments. In contrast, our system does not need hand-designed components to model ...
Scaling Automatic Speech Recognition Beyond 100 Languages ... We next present the key findings of our research, provide an overall view of the report, and review related work. ... We review the architecture and the methods used in the paper. We provide brief summaries of the Conformer [9], BEST-RQ [10], text-injection [12,13] used for MOST, and ...
Table 2 presents that we have shortlisted 148 research papers in this study to review. The primary studies having major terms such as speech recognition along with Deep Neural Network, Recurrent Neural Network, Convolution Neural Network, Long Short-term Memory, Denoising, Neural Network, Deep Learning, Transfer Learning, End-to-End ASR have been included.
pre-training has been underappreciated so far for speech recognition. We achieve these results without the need for the self-supervision or self-training techniques that have been a mainstay of recent large-scale speech recognition work. To serve as a foundation for further research on robust speech recognition, we release inference code and ...
used a deep MLP network to perform speech emotion recognition. The research used the speech data present in the IEMOCAP database . The network was composed of an input layer, five hidden layers, and three output layers, one layer for each metric. ... Rathore Y A Survey Paper on Automatic Speech Recognition by Machine. Davis KH, Biddulph R ...
Automatic Speech Emotion Recognition (ASER) has recently garnered attention across various fields including artificial intelligence, pattern recognition, and human-computer interaction. ... a systematic literature review (SLR) was conducted following established guidelines. A total of 60 primary research papers spanning from 2011 to 2023 were ...
widely used, state-of-the-art commercial speech systems. 1 Introduction Top speech recognition systems rely on sophisticated pipelines composed of multiple algorithms and hand-engineered processing stages. In this paper, we describe an end-to-end speech system, called "Deep Speech", where deep learning supersedes these processing stages.
A key source of emotional information is the spoken expression, which may be part of the interaction between the human and the machine. Speech emotion recognition (SER) is a very active area of research that involves the application of current machine learning and neural networks tools. This ongoing review covers recent and classical approaches ...
AbstractConventional automatic speech recognition (ASR) and emerging end-to-end (E2E) speech recognition have achieved promising results after being provided with sufficient resources. However, for low-resource language, the current ASR is still challenging. The Lhasa dialect is the most widespread Tibetan dialect and has a wealth of speakers ...
This paper presents fundamental concept of speech processing systems. It explores the pattern matching techniques in speech recognition system in noisy as well as in noise less environment. A ...
Speech emotion recognition (SER) as a Machine Learning (ML) problem continues to garner a significant amount of research interest, especially in the affective computing domain. This is due to its increasing potential, algorithmic advancements, and applications in real-world scenarios. Human speech contains para-linguistic information that can ...
We study the capabilities of speech processing systems trained simply to predict large amounts of transcripts of audio on the internet. When scaled to 680,000 hours of multilingual and multitask supervision, the resulting models generalize well to standard benchmarks and are often competitive with prior fully supervised results but in a zero-shot transfer setting without the need for any fine ...
In this paper, we propose a simple, yet efficient, method for speech to text recognition based on a machine learning approach, using a Romanian speech corpus. View full-text Article