INCREASING DIVERSITY WITH STATISTICAL METHODS IN GENETICS STUDIES
Add to collection, downloadable content.
- Affiliation: Gillings School of Global Public Health, Department of Biostatistics
- With the development of genotyping and sequencing technologies, genetics data have become increasingly available, making genetic studies unprecedentedly flourishing. Genetics data are diverse in all means. At genetic variant level, it covers the whole allele frequency spectrum including those ultra-rare variants as the sample size gets larger and larger, but it is still cost-prohibitive to generate sequencing data for every participant. At sample level, we have genetics data across varying genetic ancestry background including recently-admixed individuals, though their sample sizes are still limited. Statistical methods are warranted to effectively extract maximal information from these increasingly diverse yet still limited data, achieving the goal to further promote diversity in genetic studies. In my first dissertation project, I present MagicalRsq, a novel machine-learning based genotype imputation quality calibration. As an estimate of true imputation quality (true R2), MagicalRsq shows better alignment with true R2 compared to the standard state-of-the-art metric Rsq, especially for low frequency or rare variants. MagicalRsq could also achieve 10,000 to 1 million net gains of variants as a post-imputation quality metric compared to the original Rsq, leading to potential more discoveries in downstream association analysis. In my second dissertation project, I present GAUDI, a novel polygenic risk score (PRS) method focusing on recently-admixed individuals. GAUDI outperforms other PRS methods, especially for traits demonstrating ancestral-differential genetic architectures, even compared with methods utilizing summary statistics from much better-powered genome-wide association studies. In my third dissertation project, I extend MagicalRsq to MagicalRsq-X, which allows cross-population model training and is more broadly applicable in complicated real-life scenarios. MagicalRsq-X shows stable performance across different cohorts, and will be valuable for broader real-life scenarios in downstream analysis.
- Biostatistics
- statistical genetics and genomics
- genome-wide association studies
- Bioinformatics
- genetic studies
- polygenic risk score
- genotype imputation
- https://doi.org/10.17615/nsde-d161
- Dissertation
- In Copyright - Educational Use Permitted
- Love, Michael I.
- Mohlke, Karen L.
- Raffield, Laura M.
- Doctor of Philosophy
- University of North Carolina at Chapel Hill Graduate School
This work has no parents.

Select type of work
Master's papers.
Deposit your masters paper, project or other capstone work. Theses will be sent to the CDR automatically via ProQuest and do not need to be deposited.
Scholarly Articles and Book Chapters
Deposit a peer-reviewed article or book chapter. If you would like to deposit a poster, presentation, conference paper or white paper, use the “Scholarly Works” deposit form.
Undergraduate Honors Theses
Deposit your senior honors thesis.
Scholarly Journal, Newsletter or Book
Deposit a complete issue of a scholarly journal, newsletter or book. If you would like to deposit an article or book chapter, use the “Scholarly Articles and Book Chapters” deposit option.
Deposit your dataset. Datasets may be associated with an article or deposited separately.
Deposit your 3D objects, audio, images or video.
Poster, Presentation, Protocol or Paper
Deposit scholarly works such as posters, presentations, research protocols, conference papers or white papers. If you would like to deposit a peer-reviewed article or book chapter, use the “Scholarly Articles and Book Chapters” deposit option.
The Fundamentals of Modern Statistical Genetics
- © 2011
- Nan M. Laird 0 ,
- Christoph Lange 1
Department of Biostatistics, Harvard University, Boston, USA
You can also search for this author in PubMed Google Scholar
- Provides cutting edge coverage of current gene mapping approaches grounded in a traditional statistical genetics framework, with emphasis on association studies Provides exercises and solutions to reinforce basic concepts for students at all levels Rigorous coverage of key methods
- Includes supplementary material: sn.pub/extras
Part of the book series: Statistics for Biology and Health (SBH)
57 Citations
4 Altmetric
This is a preview of subscription content, log in via an institution to check access.
Access this book
- Available as EPUB and PDF
- Read on any device
- Instant download
- Own it forever
- Compact, lightweight edition
- Dispatched in 3 to 5 business days
- Free shipping worldwide - see info
- Durable hardcover edition
Tax calculation will be finalised at checkout
Other ways to access
Licence this eBook for your library
Institutional subscriptions
Table of contents (12 chapters)
Front matter, introduction to statistical genetics and background in molecular genetics.
Nan M. Laird, Christoph Lange
Principles of Inheritance: Mendel’s Laws and Genetic Models
Some basic concepts from population genetics, aggregation, heritability and segregation analysis: modeling genetic inheritance without genetic data, the general concepts of gene mapping: linkage, association, linkage disequilibrium and marker maps, basic concepts of linkage analysis, the basics of genetic association analysis, population substructure in association studies, association analysis in family designs, advanced topics.
- Genome Wide Association Studies
Looking Toward the Future
Back matter.
- Gene Mapping
- Statistical Genetics
About this book
From the reviews:
“The book covers the historical perspective, covering the standard models and methods. … The presentation of the material is carefully thought through. There are lots of figures, many in colour, a large number of examples, numerous boxes that highlight particular derivations and computations, and exercises at the ends of the chapters. All topics are clearly discussed with due detail. I would say that, for the budding statistical geneticist, this is a must-have.” (Martin Crowder, International Statistical Review, Vol. 79 (3), 2011)
“A book that focuses on statistical methods for finding links between genes and diseases … is timely. … the authors steer us gently and diligently through material that was developed originally for postgraduate students at the Harvard School of Public Health … . ideal for a statistician intending to research in this area or simply for a curious, sufficiently qualified reader. … a lovely book, and essential reading if you are a budding GWASer, or simply interested in where your next disease will come from.” (G. Wood, Australian & New Zealand Journal of Statistics, Vol. 53 (4), 2011)
“The Fundamentals of Modern Statistical Genetics, by Dr. Nan M. Laird and Dr. Christoph Lange, is a timely reference for both researchers and students. … the book is clearly written, and it is useful for colleagues who are interested in the association analysis. Although the book primarily covers the interesting topic of association analysis, it does touch other interesting topics such as joint linkage and association mapping of complex traits.” (Ruzong Fan, Journal of the American Statistical Association, March, 2013)
Authors and Affiliations
About the authors, bibliographic information.
Book Title : The Fundamentals of Modern Statistical Genetics
Authors : Nan M. Laird, Christoph Lange
Series Title : Statistics for Biology and Health
DOI : https://doi.org/10.1007/978-1-4419-7338-2
Publisher : Springer New York, NY
eBook Packages : Mathematics and Statistics , Mathematics and Statistics (R0)
Copyright Information : The Editor(s) (if applicable) and The Author(s), under exclusive license to Springer Science+Business Media, LLC, part of Springer Nature 2011
Hardcover ISBN : 978-1-4419-7337-5 Published: 02 December 2010
Softcover ISBN : 978-1-4614-2775-9 Published: 28 January 2013
eBook ISBN : 978-1-4419-7338-2 Published: 13 December 2010
Series ISSN : 1431-8776
Series E-ISSN : 2197-5671
Edition Number : 1
Number of Pages : XIV, 226
Topics : Statistics for Life Sciences, Medicine, Health Sciences , Human Genetics , Biometrics , Biostatistics , Epidemiology
- Publish with us
Policies and ethics
- Find a journal
- Track your research
Statistical Genetics
Statistical geneticists at SPH develop statistical methods for understanding the genetic basis of human diseases and traits. These methods involve large-scale data sets from candidate-gene, genome-wide and resequencing studies, using both unrelated and related individuals. SPH statistical geneticists collaborate with other investigators at SPH and around the world on studies of cancer, heart disease, diabetes, respiratory disease, psychiatric disease, and health-related behaviors (e.g. smoking, diet). They have close ties to the Program in Quantitative Genomics and Computational Biology and Bioinformatics group at SPH. Training encompasses basic statistics; Mendelian and population genetics; design and analysis of genetic association studies; gene expression and epigenetic markers; and gene-environment interaction.
Students holding a degree in mathematics, computer science, statistics or a related field and an interest in genetics are invited to apply to our Doctoral or Master’s degree programs. Faculty in the PGSG advise students in both the Epidemiology and Biostatistics departments. Prospective students can apply to either department. While it is possible to apply to both departments, it is typically not recommended. It is Graduate School policy that an individual may submit no more than three applications during the course of his or her academic career. Prospective students are encouraged to discuss which program will best fit their needs with potential advisors. More details about the application process can be found here .
Postdoctoral training positions are also available, with support coming from individual Principal Investigators or appropriate training grants. Prospective students or postdoctoral fellows with an interest in statistical genetics at SPH should contact Alkes Price .
News from the School
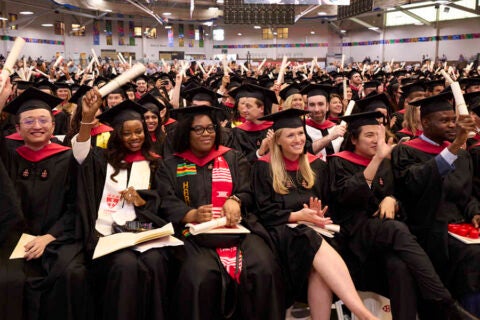
At Convocation, Harvard Chan School graduates urged to meet climate and public health crises with fresh thinking, collective action
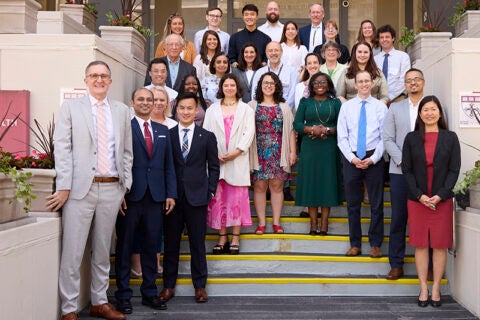
Graduation 2024: Award winners

Once a malaria patient, student now has sights set on stopping the deadly disease
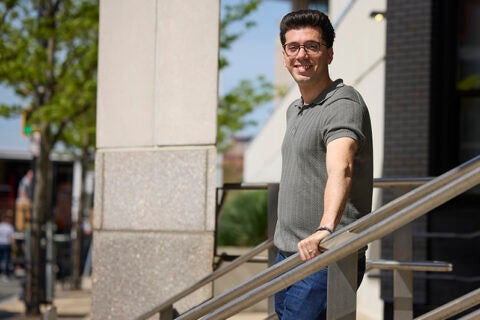
Providing compassionate care to marginalized people
Information for and about students
Applying for study | current students | alumni, uw statistical genetics ph.d. alumni, statistical genetics certificate program alumni.
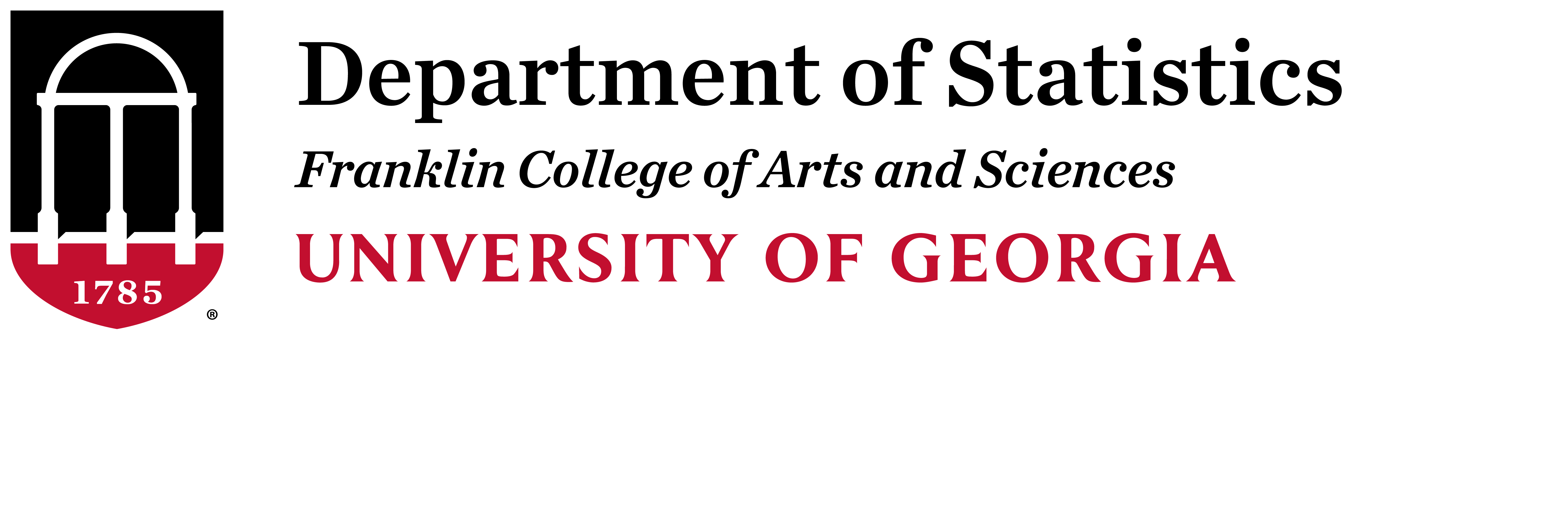
- Statistical Genetics
My research interests include phylogenetics, modeling biological data, statistical analysis of molecular data, and parallel computing. I am particularly interested in reconstructing species phylogenies from multilocus sequences.
- Big Data Analytics
- Design of Experiments
- Functional Magnetic Residence Imaging (fMRI)
- General Statistics
- Machine Learning
- Statistics and Education
We appreciate your financial support. Your gift is important to us and helps support critical opportunities for students and faculty alike, including lectures, travel support, and any number of educational events that augment the classroom experience. Click here to learn more about giving.
Every dollar given has a direct impact upon our students and faculty.
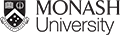
File(s) not publicly available
Statistical and ascertainment problems in human genetics, campus location, year of award, department, school or centre, degree type, usage metrics.
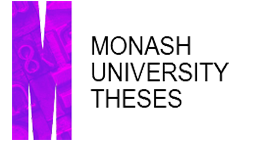
- Public Lectures
- Faculty & Staff Site >>
Statistical Genetics (not admitting students)
The Graduate Certificate in Statistical Genetics has paused admissions as of December 2023.
The Graduate Certificate in Statistical Genetics provides opportunities for concentrated education in statistical genetics to graduate students from a variety of disciplines. While primarily focused towards matriculated PhD and MS students at UW, non-matriculated students may also apply. The classes are taught by faculty in Statistics, Biostatistics, and Genome Sciences.
Program Website
Degree(s)/Certificate(s) offered
- Graduate Certificate in Statistical Genetics
Program director/interdisciplinary group chair
Primary staff contact.
- Mee-Ling Hon, Academic Counselor, Department of Statistics
Interdisciplinary Faculty Group Membership
The following are the core/voting Graduate Faculty members of the interdisciplinary group. For a complete list of faculty active in the program, see the program website.
- Brian Browning, Associate Professor, Department of Medicine
- Sharon Browning, Research Associate Professor, Department of Biostatistics
- Daniel Eisenberg, Assistant Professor, Department of Anthropology
- Joeseph Felsenstein, Professor, Departments of Biology, Genome Sciences, and Molecular Biotechnology
- Philip Green, Professor, Departments of Genome Sciences and Molecular Biotechnology
- Gail Jarvik, Professor, Departments of Medicine, Genome Sciences, and Molecular Biotechnology
- Benjamin Kerr, Professor, Department of Biology
- Kathleen Kerr, Associate Professor, Department of Biostatistics
- Adam Leaché, Assistant Professor, Department of Biology
- Harmit Malik, Affiliate Associate Professor, Departments of Genome Sciences and Molecular Biotechnology
- Frederick Matsen, Affiliate Associate Professor, Department of Statistics
- Barbara McKnight, Professor, Department of Biostatistics
- Daniel Promislow, Professor, Departments of Biology and Pathology
- Kenneth Rice, Associate Professor, Department of Biostatistics
- Ali Shojaie, Assistant Professor, Department of Biostatistics
- Noah Simon, Assistant Professor, Department of Biostatistics
- Elizabeth Thompson, Professor, Department of Statistics
- Jon Wakefield, Professor, Departments of Statistics and Biostatistics
- Bruce Weir, Professor, Department of Biostatistics
- Daniela Witten, Associate Professor, Departments of Biostatistics and Statistics
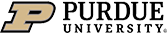
Computational Methods for Population Genetics
Iii: small: novel statistical data analysis approaches for mining human genetics datasets.
Directorate for Computer & Information Science & Engineering
III: Small: Fast and Efficient Algorithms for Matrix Decompositions and Applications to Human Genetics
Bigdata: f: dka: collaborative research: randomized numerical linear algebra (randnla) for multi-linear and non-linear data, degree type.
- Doctor of Philosophy
- Computer Science

Campus location
- West Lafayette
Advisor/Supervisor/Committee Chair
Advisor/supervisor/committee co-chair, additional committee member 2, additional committee member 3, usage metrics.
- Statistical and quantitative genetics
- Genetics not elsewhere classified
- Anthropological genetics
- Behavioural genetics
- Bioinformatics and computational biology not elsewhere classified
- Applications in life sciences
- Knowledge representation and reasoning
- Pattern recognition
- Data mining and knowledge discovery

Introduction to Statistical Genetic Analysis //
Mills, Barban & Tropf

Introducing...
a comprehensive, modern guide to applied statistical genetic data analysis, accessible to those without a background in molecular biology or genetics.
AVAILABLE NOW
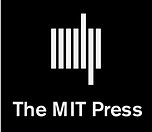
The book is available in a more cost-friendly paperback and is also available for rental as an e-book
ABOUT THE BOOK
Human genetic research is now relevant beyond biology, epidemiology, and the medical sciences, with applications in such fields as psychology, psychiatry, statistics, demography, sociology, and economics. With advances in computing power, the availability of data, and new techniques, it is now possible to integrate large-scale molecular genetic information into research across a broad range of topics. This book offers the first comprehensive introduction to modern applied statistical genetic data analysis that covers theory, data preparation, and analysis of molecular genetic data, with hands-on computer exercises.
It is accessible to students and researchers in any empirically oriented medical, biological, or social science discipline; a background in molecular biology or genetics is not required.
The book first provides foundations for statistical genetic data analysis, including a survey of fundamental concepts, primers on statistics and human evolution, and an introduction to polygenic scores. It then covers the practicalities of working with genetic data, discussing such topics as analytical challenges and data management. Finally, the book presents applications and advanced topics, including polygenic score and gene-environment interaction applications, Mendelian Randomization and instrumental variables, and ethical issues. The software and data used in the book are freely available and can be found on the book's website.

THE AUTHORS
Click Here to Learn More
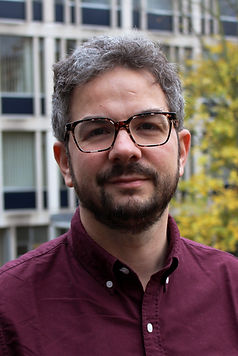
WHAT THE EXPERTS ARE SAYING
"I am regularly asked to recommend a book that provides a comprehensive overview of statistical genetics methods using accessible language with clear applications to important research questions. Look no further. Mills, Barban, and Tropf provide a superb example of such a book with An Introduction to Statistical Genetic Data Analysis."
JASON BOARDMAN Professor of Sociology and Health & Society Program Director at the Institute of Behavioral Science, University of Colorado at Boulder
"Want to run some statistical analysis of the torrent of genetic data that is pouring into science these days? An Introduction to Statistical Genetic Data Analysis is required reading for you. Mills, Barban, and Tropf walk the reader through the basics of what a gene is and march onto advanced data analysis techniques, providing plenty of compelling examples along the way."
DALTON CONLEY Henry Putnam University Professor in Sociology, Princeton University
and author of The Genome Factor
"It is increasingly clear that genetics is not just important for diseases. It contributes to many aspects of human behavior and characteristics. This book is most valuable for those whose basic training was not in statistical genetics, but are starting to incorporate genetic data into their investigations."
AUGUSTINE KONG Professor of Statistical Genetics, University of Oxford
"Contemporary genetic data offers many opportunities, and this book is easily the best available introduction. What is marvelous about the book is how comprehensive and sophisticated it is while remaining clear throughout. The way the book weaves together its explanations with software examples makes it a perfect companion for anyone wanting to better understand what these methods have to offer and how a researcher can actually use them."
JEREMY FREESE Stanford University
If you have any comments about the book, things you would like to see in the future or on this webpage, please contact us at: melinda.mills [at] nuffield.ox.ac.uk
We are human so if you find some errors, let us know and we will post them on this page.
Any other comments or questions, please message below!
Thanks for submitting!
Statistical Genetics and advanced analytical methods
Statistical genetics is a quickly developing field involving massive amounts of data (molecular markers, gene expression, proteomics, metabolomics, DNA and RNA sequences, high-throughput phenotyping, etc) that calls for advanced analytical methods. This is one of the main research topics in our group, aiming to develop methodology for sound inference for genetic, genomic and phenotypic data.
We combine a wide expertise in statistics (linear / non-linear models, mixed models and Bayesian statistics) with a diverse educational background (mathematic, statistics, biology, agronomy).
We research methods applied to a wide range of biological data, including simple and complex populations (NAM, MAGIC, etc), and often under a multivariate setting (multiple environments and/or traits). Our research is done within several national and international projects, collaborating with research teams in the academia and industry..
We also participate in training and education via regular courses at the University (BSc, MSc, and PhD), but also via specific courses targeting the academia and industry (genetic linkage mapping, quantitative trait locus (QTL) mapping and association mapping.
Thank you for visiting nature.com. You are using a browser version with limited support for CSS. To obtain the best experience, we recommend you use a more up to date browser (or turn off compatibility mode in Internet Explorer). In the meantime, to ensure continued support, we are displaying the site without styles and JavaScript.
- View all journals
- Explore content
- About the journal
- Publish with us
- Sign up for alerts
- Perspective
- Published: 13 May 2024
Integrating population genetics, stem cell biology and cellular genomics to study complex human diseases
- Nona Farbehi ORCID: orcid.org/0000-0001-8461-236X 1 , 2 , 3 na1 ,
- Drew R. Neavin ORCID: orcid.org/0000-0002-1783-6491 1 na1 ,
- Anna S. E. Cuomo 1 , 4 ,
- Lorenz Studer ORCID: orcid.org/0000-0003-0741-7987 3 , 5 ,
- Daniel G. MacArthur 4 , 6 &
- Joseph E. Powell ORCID: orcid.org/0000-0002-5070-4124 1 , 3 , 7
Nature Genetics volume 56 , pages 758–766 ( 2024 ) Cite this article
13 Altmetric
Metrics details
- Population genetics
- Transcriptomics
Human pluripotent stem (hPS) cells can, in theory, be differentiated into any cell type, making them a powerful in vitro model for human biology. Recent technological advances have facilitated large-scale hPS cell studies that allow investigation of the genetic regulation of molecular phenotypes and their contribution to high-order phenotypes such as human disease. Integrating hPS cells with single-cell sequencing makes identifying context-dependent genetic effects during cell development or upon experimental manipulation possible. Here we discuss how the intersection of stem cell biology, population genetics and cellular genomics can help resolve the functional consequences of human genetic variation. We examine the critical challenges of integrating these fields and approaches to scaling them cost-effectively and practically. We highlight two areas of human biology that can particularly benefit from population-scale hPS cell studies, elucidating mechanisms underlying complex disease risk loci and evaluating relationships between common genetic variation and pharmacotherapeutic phenotypes.
This is a preview of subscription content, access via your institution
Access options
Access Nature and 54 other Nature Portfolio journals
Get Nature+, our best-value online-access subscription
24,99 € / 30 days
cancel any time
Subscribe to this journal
Receive 12 print issues and online access
195,33 € per year
only 16,28 € per issue
Buy this article
- Purchase on Springer Link
- Instant access to full article PDF
Prices may be subject to local taxes which are calculated during checkout
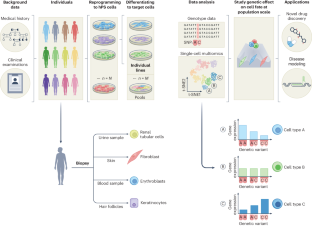
Similar content being viewed by others
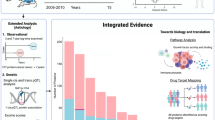
Identifying proteomic risk factors for cancer using prospective and exome analyses of 1463 circulating proteins and risk of 19 cancers in the UK Biobank
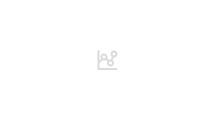
In vitro reconstitution of epigenetic reprogramming in the human germ line
A deep catalogue of protein-coding variation in 983,578 individuals.
Thomson, J. A. Embryonic stem cell lines derived from human blastocysts. Science https://doi.org/10.1126/science.282.5391.1145 (1998).
Takahashi, K. & Yamanaka, S. Induction of pluripotent stem cells from mouse embryonic and adult fibroblast cultures by defined factors. Cell 126 , 663–676 (2006).
Article CAS PubMed Google Scholar
Takahashi, K. et al. Induction of pluripotent stem cells from adult human fibroblasts by defined factors. Cell 131 , 861–872 (2007).
Liu, G., David, B. T., Trawczynski, M. & Fessler, R. G. Advances in pluripotent stem cells: history, mechanisms, technologies, and applications. Stem Cell Rev. Rep. 16 , 3–32 (2020).
Article PubMed Google Scholar
Efrat, S. Epigenetic memory: lessons from iPS cells derived from human β cells. Front. Endocrinol. 11 , 614234 (2020).
Article Google Scholar
Anderson, R. H. & Francis, K. R. Modeling rare diseases with induced pluripotent stem cell technology. Mol. Cell. Probes 40 , 52–59 (2018).
Article CAS PubMed PubMed Central Google Scholar
Spitalieri, P., Talarico, V. R., Murdocca, M., Novelli, G. & Sangiuolo, F. Human induced pluripotent stem cells for monogenic disease modelling and therapy. World J. Stem Cells 8 , 118–135 (2016).
Article PubMed PubMed Central Google Scholar
Passier, R., Orlova, V. & Mummery, C. Complex tissue and disease modeling using hiPSCs. Cell Stem Cell 18 , 309–321 (2016).
Warren, C. R., Jaquish, C. E. & Cowan, C. A. The NextGen genetic association studies consortium: a foray into in vitro population genetics. Cell Stem Cell 20 , 431–433 (2017).
Visscher, P. M., Brown, M. A., McCarthy, M. I. & Yang, J. Five years of GWAS discovery. Am. J. Hum. Genet. 90 , 7–24 (2012).
Tak, Y. G. & Farnham, P. J. Making sense of GWAS: using epigenomics and genome engineering to understand the functional relevance of SNPs in non-coding regions of the human genome. Epigenetics Chromatin 8 , 57 (2015).
Umans, B. D., Battle, A. & Gilad, Y. Where are the disease-associated eQTLs? Trends Genet. 37 , 109–124 (2021).
Yazar, S. et al. Single-cell eQTL mapping identifies cell type–specific genetic control of autoimmune disease. Science 376 , eabf3041 (2022).
Jerber, J. et al. Population-scale single-cell RNA-seq profiling across dopaminergic neuron differentiation. Nat. Genet. 53 , 304–312 (2021).
Neavin, D. et al. Single cell eQTL analysis identifies cell type-specific genetic control of gene expression in fibroblasts and reprogrammed induced pluripotent stem cells. Genome Biol. 22 , 76 (2021).
Cuomo, A. S. E. et al. Single-cell RNA-sequencing of differentiating iPS cells reveals dynamic genetic effects on gene expression. Nat. Commun. 11 , 810 (2020).
Warren, C. R. et al. Induced pluripotent stem cell differentiation enables functional validation of GWAS variants in metabolic disease. Cell Stem Cell 20 , 547–557 (2017).
Kishore, S. et al. A non-coding disease modifier of pancreatic agenesis identified by genetic correction in a patient-derived iPSC line. Cell Stem Cell 27 , 137–146 (2020).
Magdy, T. et al. RARG variant predictive of doxorubicin-induced cardiotoxicity identifies a cardioprotective therapy. Cell Stem Cell 28 , 2076–2089 (2021).
Bourgeois, S. et al. Towards a functional cure for diabetes using stem cell-derived beta cells: are we there yet? Cells 10 , 191 (2021).
Sharma, A., Sances, S., Workman, M. J. & Svendsen, C. N. Multi-lineage human iPSC-derived platforms for disease modeling and drug discovery. Cell Stem Cell 26 , 309–329 (2020).
Volpato, V. & Webber, C. Addressing variability in iPSC-derived models of human disease: guidelines to promote reproducibility. Dis. Model. Mech. 13 , dmm042317 (2020).
Banovich, N. E. et al. Impact of regulatory variation across human iPSCs and differentiated cells. Genome Res. 28 , 122–131 (2018).
Kilpinen, H. et al. Common genetic variation drives molecular heterogeneity in human iPSCs. Nature 546 , 370–375 (2017).
Panopoulos, A. D. et al. iPSCORE: a resource of 222 iPSC lines enabling functional characterization of genetic variation across a variety of cell types. Stem Cell Rep. 8 , 1086–1100 (2017).
Article CAS Google Scholar
Chen, G., Ning, B. & Shi, T. Single-cell RNA-seq technologies and related computational data analysis. Front. Genet. 10 , 317 (2019).
Elorbany, R. et al. Single-cell sequencing reveals lineage-specific dynamic genetic regulation of gene expression during human cardiomyocyte differentiation. PLoS Genet. 18 , e1009666 (2022).
Ward, M. C., Banovich, N. E., Sarkar, A., Stephens, M. & Gilad, Y. Dynamic effects of genetic variation on gene expression revealed following hypoxic stress in cardiomyocytes. eLife 10 , e57345 (2021).
Shi, Z.-D. et al. Genome editing in hPSCs reveals GATA6 haploinsufficiency and a genetic interaction with GATA4 in human pancreatic development. Cell Stem Cell 20 , 675–688 (2017).
Strober, B. J. et al. Dynamic genetic regulation of gene expression during cellular differentiation. Science 364 , 1287–1290 (2019).
González, F. et al. An iCRISPR platform for rapid, multiplexable, and inducible genome editing in human pluripotent stem cells. Cell Stem Cell 15 , 215–226 (2014).
Barbeira, A. N. et al. Exploiting the GTEx resources to decipher the mechanisms at GWAS loci. Genome Biol. 22 , 49 (2021).
Hamazaki, T., El Rouby, N., Fredette, N. C., Santostefano, K. E. & Terada, N. Concise review: induced pluripotent stem cell research in the era of precision medicine. Stem Cells 35 , 545–550 (2017).
Cuomo, A. S. E. et al. CellRegMap: a statistical framework for mapping context-specific regulatory variants using scRNA-seq. Mol. Syst. Biol. 18 , e10663 (2022).
Cuomo, A. S. E., Nathan, A., Raychaudhuri, S., MacArthur, D. G. & Powell, J. E. Single-cell genomics meets human genetics. Nat. Rev. Genet. 24 , 535–549 (2023).
Mirauta, B. A. et al. Population-scale proteome variation in human induced pluripotent stem cells. eLife 9 , e57390 (2020).
Findley, A. S. et al. Functional dynamic genetic effects on gene regulation are specific to particular cell types and environmental conditions. eLife 10 , e67077 (2021).
Kimura, M. et al. En masse organoid phenotyping informs metabolic-associated genetic susceptibility to NASH. Cell https://doi.org/10.1016/j.cell.2022.09.031 (2022).
Llufrio, E. M., Wang, L., Naser, F. J. & Patti, G. J. Sorting cells alters their redox state and cellular metabolome. Redox Biol. 16 , 381–387 (2018).
Shen, S. et al. Integrating single-cell genomics pipelines to discover mechanisms of stem cell differentiation. Trends Mol. Med. https://doi.org/10.1016/j.molmed.2021.09.006 (2021).
van der Wijst, M. et al. The single-cell eQTLGen consortium. eLife 9 , e52155 (2020).
Soskic, B. et al. Immune disease risk variants regulate gene expression dynamics during CD4 + T cell activation. Nat. Genet. 54 , 817–826 (2022).
Daniszewski, M. et al. Retinal ganglion cell-specific genetic regulation in primary open-angle glaucoma. Cell Genomics 2 , 100142 (2022).
Senabouth, A. et al. Transcriptomic and proteomic retinal pigment epithelium signatures of age-related macular degeneration. Nat. Commun. 13 , 4233 (2022).
Benaglio, P. et al. Mapping genetic effects on cell type-specific chromatin accessibility and annotating complex immune trait variants using single nucleus ATAC-seq in peripheral blood. PLoS Genet. 19 , e1010759 (2023).
Baysoy, A., Bai, Z., Satija, R. & Fan, R. The technological landscape and applications of single-cell multi-omics. Nat. Rev. Mol. Cell Biol. 24 , 695–713 (2023).
Weinshilboum, R. M. & Wang, L. Pharmacogenomics: precision medicine and drug response. Mayo Clin. Proc. 92 , 1711–1722 (2017).
Pirmohamed, M. Personalized pharmacogenomics: predicting efficacy and adverse drug reactions. Annu. Rev. Genom. Hum. Genet. 15 , 349–370 (2014).
Nelson, M. R. et al. The support of human genetic evidence for approved drug indications. Nat. Genet. 47 , 856–860 (2015).
Hay, M., Thomas, D. W., Craighead, J. L., Economides, C. & Rosenthal, J. Clinical development success rates for investigational drugs. Nat. Biotechnol. 32 , 40–51 (2014).
Holmgren, G. et al. Long-term chronic toxicity testing using human pluripotent stem cell-derived hepatocytes. Drug Metab. Dispos. 42 , 1401–1406 (2014).
Kim, J.-H., Kang, M., Jung, J.-H., Lee, S.-J. & Hong, S.-H. Human pluripotent stem cell-derived alveolar epithelial cells as a tool to assess cytotoxicity of particulate matter and cigarette smoke extract. Dev. Reprod. 26 , 155–163 (2022).
Sharma, A. et al. High-throughput screening of tyrosine kinase inhibitor cardiotoxicity with human induced pluripotent stem cells. Sci. Transl. Med. 9 , eaaf2584 (2017).
Han, Y. et al. Identification of SARS-CoV-2 inhibitors using lung and colonic organoids. Nature 589 , 270–275 (2021).
Lam, C. K. & Wu, J. C. Clinical trial in a dish: using patient-derived induced pluripotent stem cells to identify risks of drug-induced cardiotoxicity. Arterioscler. Thromb. Vasc. Biol. 41 , 1019–1031 (2021).
Iwata, R. et al. Mitochondria metabolism sets the species-specific tempo of neuronal development. Science 379 , eabn4705 (2023).
Miller, J. D. et al. Human iPSC-based modeling of late-onset disease via progerin-induced aging. Cell Stem Cell 13 , 691–705 (2013).
Hergenreder, E. et al. Combined small-molecule treatment accelerates maturation of human pluripotent stem cell-derived neurons. Nat. Biotechnol. https://doi.org/10.1038/s41587-023-02031-z (2024).
Fowler, J. L., Ang, L. T. & Loh, K. M. A critical look: challenges in differentiating human pluripotent stem cells into desired cell types and organoids. Wiley Interdiscip. Rev. Dev. Biol. 9 , e368 (2020).
Jiang, S., Feng, W., Chang, C. & Li, G. Modeling human heart development and congenital defects using organoids: how close are we? J. Cardiovasc. Dev. Dis. 9 , 125 (2022).
CAS PubMed PubMed Central Google Scholar
Tremmel, D. M. et al. Validating expression of beta cell maturation-associated genes in human pancreas development. Front. Cell Dev. Biol. 11 , 1103719 (2023).
Washer, S. J. et al. Single-cell transcriptomics defines an improved, validated monoculture protocol for differentiation of human iPSC to microglia. Sci. Rep. 12 , 19454 (2022).
Wolf, F. A., Angerer, P. & Theis, F. J. SCANPY: large-scale single-cell gene expression data analysis. Genome Biol. 19 , 15 (2018).
Wilson, S. B. et al. DevKidCC allows for robust classification and direct comparisons of kidney organoid datasets. Genome Med. 14 , 19 (2022).
Subramanian, A. et al. Single cell census of human kidney organoids shows reproducibility and diminished off-target cells after transplantation. Nat. Commun. 10 , 5462 (2019).
Kammers, K. et al. Gene and protein expression in human megakaryocytes derived from induced pluripotent stem cells. J. Thromb. Haemost. 19 , 1783–1799 (2021).
De Sousa, P. A. et al. Rapid establishment of the European Bank for induced Pluripotent Stem Cells (EBiSC)—the Hot Start experience. Stem Cell Res. 20 , 105–114 (2017).
Morrison, M. et al. StemBANCC: governing access to material and data in a large stem cell research consortium. Stem Cell Rev. Rep. 11 , 681–687 (2015).
The GTEx Consortium The GTEx Consortium atlas of genetic regulatory effects across human tissues. Science 369 , 1318–1330 (2020).
Article PubMed Central Google Scholar
Mitchell, J. M., Nemesh, J., Ghosh, S. & Handsaker, R. E. Mapping genetic effects on cellular phenotypes with ‘cell villages’. Preprint at bioRxiv https://doi.org/10.1101/2020.06.29.174383 (2020).
Neavin, D. R. et al. A village in a dish model system for population-scale hiPSC studies. Nat. Commun. 14 , 3240 (2023).
Kang, H. M. et al. Multiplexed droplet single-cell RNA-sequencing using natural genetic variation. Nat. Biotechnol. 36 , 89–94 (2018).
Wells, M. F. et al. Natural variation in gene expression and viral susceptibility revealed by neural progenitor cell villages. Cell Stem Cell 30 , 312–332 (2023).
Neavin, D. et al. Demuxafy : improvement in droplet assignment by integrating multiple single-cell demultiplexing and doublet detection methods. Genome Biol. 25 , 94 (2024).
Xu, J. et al. Genotype-free demultiplexing of pooled single-cell RNA-seq. Genome Biol. 20 , 290 (2019).
Heaton, H. et al. Souporcell: robust clustering of single-cell RNA-seq data by genotype without reference genotypes. Nat. Methods 17 , 615–620 (2020).
Huang, Y., McCarthy, D. J. & Stegle, O. Vireo: Bayesian demultiplexing of pooled single-cell RNA-seq data without genotype reference. Genome Biol. 20 , 273 (2019).
Hindson, B. J. et al. High-throughput droplet digital PCR system for absolute quantitation of DNA copy number. Anal. Chem. 83 , 8604–8610 (2011).
Dong, X. et al. powerEQTL: an R package and shiny application for sample size and power calculation of bulk tissue and single-cell eQTL analysis. Bioinformatics https://doi.org/10.1093/bioinformatics/btab385 (2021).
Schmid, K. T. et al. scPower accelerates and optimizes the design of multi-sample single cell transcriptomic studies. Nat. Commun. 12 , 6625 (2021).
Camp, J. G., Platt, R. & Treutlein, B. Mapping human cell phenotypes to genotypes with single-cell genomics. Science 365 , 1401–1405 (2019).
Datlinger, P. et al. Pooled CRISPR screening with single-cell transcriptome readout. Nat. Methods 14 , 297–301 (2017).
Dixit, A. et al. Perturb-Seq: dissecting molecular circuits with scalable single-cell RNA profiling of pooled genetic screens. Cell 167 , 1853–1866 (2016).
Rubin, A. J. et al. Coupled single-cell CRISPR screening and epigenomic profiling reveals causal gene regulatory networks. Cell 176 , 361–376 (2019).
Schraivogel, D. et al. Targeted Perturb-seq enables genome-scale genetic screens in single cells. Nat. Methods 17 , 629–635 (2020).
Download references
Acknowledgements
Figures were generated with BioRender.com and further developed by A. Garcia, a scientific illustrator from Bio-Graphics. This research was supported by a National Health and Medical Research Council (NHMRC) Investigator grant (J.E.P., 1175781), research grants from the Australian Research Council (ARC) Special Research Initiative in Stem Cell Science, an ARC Discovery Project (190100825), an EMBO Postdoctoral Fellowship (A.S.E.C.) and an Aligning Science Across Parkinson’s Grant (J.E.P., N.F., D.R.N. and L.S.). J.E.P. is supported by a Fok Family Fellowship.
Author information
These authors contributed equally: Nona Farbehi, Drew R. Neavin.
Authors and Affiliations
Garvan Weizmann Center for Cellular Genomics, Garvan Institute of Medical Research, Sydney, New South Wales, Australia
Nona Farbehi, Drew R. Neavin, Anna S. E. Cuomo & Joseph E. Powell
Graduate School of Biomedical Engineering, University of New South Wales, Sydney, New South Wales, Australia
Nona Farbehi
Aligning Science Across Parkinson’s Collaborative Research Network, Chevy Chase, MD, USA
Nona Farbehi, Lorenz Studer & Joseph E. Powell
Centre for Population Genomics, Garvan Institute of Medical Research, University of New South Wales, Sydney, New South Wales, Australia
Anna S. E. Cuomo & Daniel G. MacArthur
The Center for Stem Cell Biology and Developmental Biology Program, Sloan-Kettering Institute for Cancer Research, New York, NY, USA
Lorenz Studer
Centre for Population Genomics, Murdoch Children’s Research Institute, Melbourne, Victoria, Australia
Daniel G. MacArthur
UNSW Cellular Genomics Futures Institute, University of New South Wales, Sydney, New South Wales, Australia
Joseph E. Powell
You can also search for this author in PubMed Google Scholar
Contributions
All authors conceived the topic and wrote and revised the manuscript.
Corresponding author
Correspondence to Joseph E. Powell .
Ethics declarations
Competing interests.
D.G.M. is a founder with equity in Goldfinch Bio, is a paid advisor to GSK, Insitro, Third Rock Ventures and Foresite Labs, and has received research support from AbbVie, Astellas, Biogen, BioMarin, Eisai, Merck, Pfizer and Sanofi-Genzyme; none of these activities is related to the work presented here. J.E.P. is a founder with equity in Celltellus Laboratory and has received research support from Illumina. The other authors declare no conflict of interest.
Peer review
Peer review information.
Nature Genetics thanks Kelly Frazer, Gosia Trynka and the other, anonymous, reviewer(s) for their contribution to the peer review of this work.
Additional information
Publisher’s note Springer Nature remains neutral with regard to jurisdictional claims in published maps and institutional affiliations.
Supplementary information
Supplementary information.
Supplementary Table 1.
Rights and permissions
Springer Nature or its licensor (e.g. a society or other partner) holds exclusive rights to this article under a publishing agreement with the author(s) or other rightsholder(s); author self-archiving of the accepted manuscript version of this article is solely governed by the terms of such publishing agreement and applicable law.
Reprints and permissions
About this article
Cite this article.
Farbehi, N., Neavin, D.R., Cuomo, A.S.E. et al. Integrating population genetics, stem cell biology and cellular genomics to study complex human diseases. Nat Genet 56 , 758–766 (2024). https://doi.org/10.1038/s41588-024-01731-9
Download citation
Received : 24 January 2023
Accepted : 20 March 2024
Published : 13 May 2024
Issue Date : May 2024
DOI : https://doi.org/10.1038/s41588-024-01731-9
Share this article
Anyone you share the following link with will be able to read this content:
Sorry, a shareable link is not currently available for this article.
Provided by the Springer Nature SharedIt content-sharing initiative
Quick links
- Explore articles by subject
- Guide to authors
- Editorial policies
Sign up for the Nature Briefing newsletter — what matters in science, free to your inbox daily.

- Courses & Registration
Office of Neuroscience Research
Thesis Defense: Kara Quaid (Human and Statistical Genetics Program) – “Understanding the Relationship between Genetic and Epigenetic Variation at a Species and Individual Level”
Thesis lab: Ting Wang (WashU Genetics)
For inquiries contact Kara at [email protected] .


COMMENTS
1947-2022. Harvard affiliates with an ID number and PIN can get free download of dissertations, both Harvard and other, on the Digital Access to Scholarship at Harvard site via DASH . Click on "By Collections" in the left hand menu under Statistics, and select "FAS Theses and Dissertations" in the list. Choose the option "This Collection" under ...
One current study is the NHLBI Trans-Omics for Precision Medicine (TOPMed) project. www.nhlbiwgs.org For data freeze 5 of this study: Sequence analysis identified 410,323,831 genetic variants (381,343,078 SNVs and 28,980,753 indels), corresponding to an average of one variant per 7 bp throughout the reference genome.
PhD with the Statistical Genetics Pathway. Students in our Doctor of Philosophy (PhD) in Biostatistics program may choose a pathway in statistical genetics which provides rigorous training in the areas of statistical genetics, population genetics, and computational molecular biology. Learn more.
At sample level, we have genetics data across varying genetic ancestry background including recently-admixed individuals, though their sample sizes are still limited. Statistical methods are warranted to effectively extract maximal information from these increasingly diverse yet still limited data, achieving the goal to further promote ...
To do so, we build on the classical linear mixed model (LMM), a widely adopted framework for genetic studies. The first contribution of this thesis is mtSet, an efficient mixed-model approach that ...
Dr. Laird is a Professor of Biostatistics in the Biostatistics Department at the Harvard School of Public Health. Dr. Laird has contributed to methodology in many different fields, including missing data, EM-algorithm, meta-analysis, statistical genetics, and has coauthored a book with Garrett Fitzmaurice and James Ware on Applied Longitudinal Analysis.
Statistical geneticists at SPH develop statistical methods for understanding the genetic basis of human diseases and traits. These methods involve large-scale data sets from candidate-gene, genome-wide and resequencing studies, using both unrelated and related individuals. SPH statistical geneticists collaborate with other investigators at SPH ...
Statistical Bioinformatics. In no particular order, I would also like to thank my thesis committee panel members viz, Dr. Jonathon E. Mohl, Dr. Amy Wagler and Dr. Lela Vukovic for their invaluable co-operation and support towards the success of this thesis dissertation. Worth
We offer an accelerated 18-month MS capstone program, and a traditional MS thesis program. Doctor of Philosophy. Earn a PhD in Biostatistics to prepare for careers such as an independent investigator, educator, or highly-qualified practitioner of biostatistics. We offer a standard pathway and a statistical genetics pathway.
Students interested in graduate study in Statistical Genetics should apply directly to the Statistics or Biostatistics department in the usual way. At the University of Washington, these two departments maintain a close partnership in their graduate programs, and the Statistical Genetics curriculum is a joint endeavor. ... , Thesis adviser ...
Thesis and Dissertations >> MS Thesis; PhD Dissertation; Statistics Club; Slideshow. Statistical Genetics. Personnel. Liang Liu Professor. My research interests include phylogenetics, modeling biological data, statistical analysis of molecular data, and parallel computing. I am particularly interested in reconstructing species phylogenies from ...
The thesis contains a paper intended for publication in a peer reviewed journal. The paper contains a description of the data and the genetic association analysis results, along with details of the overall statistical modeling framework that includes steps for data curation, demographic analysis, assumption checking, the linear mixed modeling
A timely update of a highly popular handbook on statistical genomics This new, two-volume edition of a classic text provides a thorough introduction to statistical genomics, a vital resource for advanced graduate students, early-career researchers and new entrants to the field. It introduces new and updated information on developments that have occurred since the 3rd edition. Widely regarded ...
This thesis was scanned from the print manuscript for digital preservation and is copyright the author. Researchers can access this thesis by asking their local university, institution or public library to make a request on their behalf. Monash staff and postgraduate students can use the link in the References field.
A comprehensive tutorial on imputing HLA alleles from genotype data, offering best practices for association testing and defining HLA's role in diseases.
Three Minute Thesis (3MT) ... The Graduate Certificate in Statistical Genetics has paused admissions as of December 2023. The Graduate Certificate in Statistical Genetics provides opportunities for concentrated education in statistical genetics to graduate students from a variety of disciplines. While primarily focused towards matriculated PhD ...
The field of population genetics has seen an unprecedented growth driven by the advancement of sequencing technologies, resulting in volumes of massive datasets. As a result, efficient computational methods backed by theoretical foundations are required to analyze and understand the intricate details of complex biological processes captured in the genetic code. To this end, we developed novel ...
To satisfy certificate requirements, you must complete: At least 15 credit hours, with at least 9 credit hours from courses numbered 500 and above. Required courses include BIOST 550, BIOST 551 and 3 credits of BIOST 581 (Statistical Genetics Seminar). Completion of a statistical genetics capstone experience.
The book first provides foundations for statistical genetic data analysis, including a survey of fundamental concepts, primers on statistics and human evolution, and an introduction to polygenic scores. It then covers the practicalities of working with genetic data, discussing such topics as analytical challenges and data management.
Statistical genetics is a quickly developing field involving massive amounts of data (molecular markers, gene expression, proteomics, metabolomics, DNA and RNA sequences, high-throughput phenotyping, etc) that calls for advanced analytical methods. This is one of the main research topics in our group, aiming to develop methodology for sound inference for genetic, genomic and phenotypic data.
A master's thesis is an independent scientific work and is meant to prepare students for future professional or academic work. Largely, the thesis is expected to be similar to papers published in statistical journals. It is not set in stone exactly how the thesis should be organized. The following outline should however be followed. Title Page
A final challenge is the necessity of performing genome-wide hPS cell studies with adequate sample sizes to detect genetic effects with statistical significance. Population genomic approaches ...
BIOST 516. Theory and application of statistical techniques used in genetic epidemiology. Includes discussion of association studies, linkages and segregation analyses. Examples stressed with reference to assumptions and limitations. Prerequisites: either BIOST 513 or BIOST 518; PHG 511/EPI 517; or permission of instructor.
Thesis Defense: Kara Quaid (Human and Statistical Genetics Program) - "Understanding the Relationship between Genetic and Epigenetic Variation at a Species and Individual Level" June 10, 2024. 2:00 pm - 3:00 pm. Thesis Defenses, Upcoming. Zoom/Holden Auditorium (Farrell LTC, Medical Campus) ...