To read this content please select one of the options below:
Please note you do not have access to teaching notes, a systematic literature review of data science, data analytics and machine learning applied to healthcare engineering systems.
Management Decision
ISSN : 0025-1747
Article publication date: 7 December 2020
Issue publication date: 2 February 2022
The objective of this paper is to assess and synthesize the published literature related to the application of data analytics, big data, data mining and machine learning to healthcare engineering systems.

Design/methodology/approach
A systematic literature review (SLR) was conducted to obtain the most relevant papers related to the research study from three different platforms: EBSCOhost, ProQuest and Scopus. The literature was assessed and synthesized, conducting analysis associated with the publications, authors and content.
From the SLR, 576 publications were identified and analyzed. The research area seems to show the characteristics of a growing field with new research areas evolving and applications being explored. In addition, the main authors and collaboration groups publishing in this research area were identified throughout a social network analysis. This could lead new and current authors to identify researchers with common interests on the field.
Research limitations/implications
The use of the SLR methodology does not guarantee that all relevant publications related to the research are covered and analyzed. However, the authors' previous knowledge and the nature of the publications were used to select different platforms.
Originality/value
To the best of the authors' knowledge, this paper represents the most comprehensive literature-based study on the fields of data analytics, big data, data mining and machine learning applied to healthcare engineering systems.
- Data analytics
- Machine learning
- Healthcare systems
- Systematic literature review
Salazar-Reyna, R. , Gonzalez-Aleu, F. , Granda-Gutierrez, E.M.A. , Diaz-Ramirez, J. , Garza-Reyes, J.A. and Kumar, A. (2022), "A systematic literature review of data science, data analytics and machine learning applied to healthcare engineering systems", Management Decision , Vol. 60 No. 2, pp. 300-319. https://doi.org/10.1108/MD-01-2020-0035
Emerald Publishing Limited
Copyright © 2020, Emerald Publishing Limited
Related articles
We’re listening — tell us what you think, something didn’t work….
Report bugs here
All feedback is valuable
Please share your general feedback
Join us on our journey
Platform update page.
Visit emeraldpublishing.com/platformupdate to discover the latest news and updates
Questions & More Information
Answers to the most commonly asked questions here
Methodology
- Open access
- Published: 28 April 2022
An intelligent literature review: adopting inductive approach to define machine learning applications in the clinical domain
- Renu Sabharwal ORCID: orcid.org/0000-0001-9728-8001 1 &
- Shah J. Miah 1
Journal of Big Data volume 9 , Article number: 53 ( 2022 ) Cite this article
6810 Accesses
9 Citations
Metrics details
Big data analytics utilizes different techniques to transform large volumes of big datasets. The analytics techniques utilize various computational methods such as Machine Learning (ML) for converting raw data into valuable insights. The ML assists individuals in performing work activities intelligently, which empowers decision-makers. Since academics and industry practitioners have growing interests in ML, various existing review studies have explored different applications of ML for enhancing knowledge about specific problem domains. However, in most of the cases existing studies suffer from the limitations of employing a holistic, automated approach. While several researchers developed various techniques to automate the systematic literature review process, they also seemed to lack transparency and guidance for future researchers. This research aims to promote the utilization of intelligent literature reviews for researchers by introducing a step-by-step automated framework. We offer an intelligent literature review to obtain in-depth analytical insight of ML applications in the clinical domain to (a) develop the intelligent literature framework using traditional literature and Latent Dirichlet Allocation (LDA) topic modeling, (b) analyze research documents using traditional systematic literature review revealing ML applications, and (c) identify topics from documents using LDA topic modeling. We used a PRISMA framework for the review to harness samples sourced from four major databases (e.g., IEEE, PubMed, Scopus, and Google Scholar) published between 2016 and 2021 (September). The framework comprises two stages—(a) traditional systematic literature review consisting of three stages (planning, conducting, and reporting) and (b) LDA topic modeling that consists of three steps (pre-processing, topic modeling, and post-processing). The intelligent literature review framework transparently and reliably reviewed 305 sample documents.
Introduction
Organizations are continuously harnessing the power of various big data adopting different ML techniques. Captured insights from big data may create a greater impact to reshape their business operations and processes. As a vital technique, big data analytics methods are used to transform complicated and huge amounts of data, known as ‘Big Data, in order to uncover hidden patterns, new learning, untold facts or associations, anomalies, and other perceptions [ 41 ]. Big Data alludes to the enormous amount of data that a traditional database management system cannot handle. In most of the cases, traditional software functions would be inadequate to analyze or process them. Big data are characterized by the 5 V’s, which refers to volume, variety, velocity, veracity, and value [ 22 ]. ML is a vital approach to design useful big data analytics techniques, which is a rapidly growing sub-field in information sciences that deals with all these characteristics. ML employs numerous methods for machines to learn from past experiences (e.g., past datasets) reducing the extra burden of writing codes in traditional programming [ 7 , 26 ]. Clinical care enterprises face a huge challenge due to the increasing demand of big data processing to improve clinical care outcomes. For example, an electronic health record contains a huge amount of patient information, drug administration, imaging data using various modalities. The variety and quantity of the huge data provide in the clinical domain as an ideal topic to appraise the value of ML in research.
Existing ML approaches, such as Oala et al. [ 35 ] proposed an algorithmic framework that give a path towards the effective and reliable application of ML in the healthcare domain. In conjunction with their systematic review, our research offers a smart literature review that consolidates a traditional literature review followed the PRISMA framework guidelines and topic modeling using LDA, focusing on the clinical domain. Most of the existing literature focused on the healthcare domain [ 14 , 42 , 49 ] are more inclusive and of a broader scope with a requisite of medical activities, whereas our research is primarily focused is clinical, which assist in diagnosing and treating patients as well as includes clinical aspects of medicine.
Since clinical research has developed, the area has become increasingly attractive to clinical researchers, in particular for learning insights of ML applications in clinical practices . This is because of its practical pertinence to clinical patients, professionals, clinical application designers, and other specialists supported by the omnipresence of clinical disease management techniques. Although the advantage is presumed for the target audience, such as self-management abilities (self-efficacy and investment behavior) and physical or mental condition of life amid long-term ill patients, clinical care specialists (such as further developing independent direction and providing care support to patients), their clinical care have not been previously assessed and conceptualized as a well-defined and essential sub-field of health care research. It is important to portray similar studies utilizing different types of review approaches in the aspect of the utilization of ML/DL and its value. Table 1 represents some examples of existing studies with various points and review approaches in the domain.
Although the existing studies included in Table 1 give an understanding of designated aspects of ML/DL utilization in clinical care, they show a lack of focus on how key points addressed in existing ML/DL research are developing. Further to this, they indicate a clear need towards an understanding of multidisciplinary affiliations and profiles of ML/DL that could provide significant knowledge to new specialists or professionals in this space. For instance, Brnabic and Hess [ 8 ] recommended a direction for future research by stating that “ Future work should routinely employ ensemble methods incorporating various applications of machine learning algorithms” (p. 1).
ML tools have become the central focus of modern biomedical research, because of better admittance to large datasets, exponential processing power, and key algorithmic developments allowing ML models to handle increasingly challenging data [ 19 ]. Different ML approaches can analyze a huge amount of data, including difficult and abnormal patterns. Most studies have focused on ML and its impacts on clinical practices [ 2 , 9 , 10 , 24 , 26 , 34 , 43 ]. Fewer studies have examined the utilization of ML algorithms [ 11 , 20 , 45 , 48 ] for more holistic benefits for clinical researchers.
ML becomes an interdisciplinary science that integrates computer science, mathematics, and statistics. It is also a methodology that builds smart machines for artificial intelligence. Its applications comprise algorithms, an assortment of instructions to perform specific tasks, crafted to independently learn from data without human intercession. Over time, ML algorithms improve their prediction accuracy without a need for programming. Based on this, we offer an intelligent literature review using traditional literature review and Latent Dirichlet Allocation (LDA Footnote 1 ) topic modeling in order to meet knowledge demands in the clinical domain. Theoretical measures direct the current study results because previous literature provides a strong foundation for future IS researchers to investigate ML in the clinical sector. The main aim of this study is to develop an intelligent literature framework using traditional literature. For this purpose, we employed four digital databases -IEEE, Google Scholar, PubMed, and Scopus then performed LDA topic modeling, which may assist healthcare or clinical researchers in analyzing many documents intelligently with little effort and a small amount of time.
Traditional systematic literature is destined to be obsolete, time-consuming with restricted processing power, resulting in fewer sample documents investigated. Academic and practitioner-researchers are frequently required to discover, organize, and comprehend new and unexplored research areas. As a part of a traditional literature review that involves an enormous number of papers, the choice for a researcher is either to restrict the number of documents to review a priori or analyze the study using some other methods.
The proposed intelligent literature review approach consists of Part A and Part B, a combination of traditional systematic literature review and topic modeling that may assist future researchers in using appropriate technology, producing accurate results, and saving time. We present the framework below in Fig. 1 .
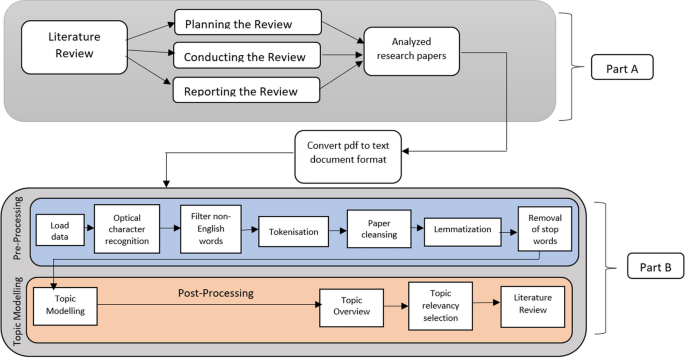
Proposed intelligent literature review framework
The traditional literature review identified 534,327 articles embraces Scopus (24,498), IEEE (2558), PubMed (11,271), and Google Scholar (496,000) articles, which went through three stages–Planning the review, conducting the review, and reporting the review and analyzed 305 articles, where we performed topic modeling using LDA.
We follow traditional systematic literature review methodologies [ 25 , 39 , 40 ] including a PRISMA framework [ 37 ]. We review four digital databases and deliberately develop three stages entailing planning, conducting, and reporting the review (Fig. 2 ).
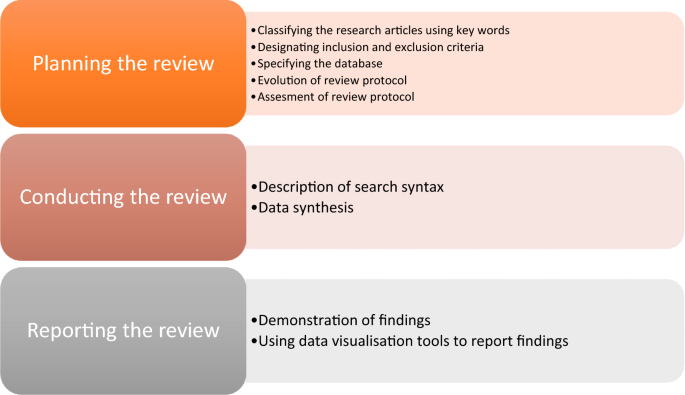
Traditional literature review three stages
Planning the review
Research articles : the research articles are classified using some keywords mentioned below in Tables 2 , 3 .
Digital database : Four databases (IEEE, PubMed, Scopus, and Google Scholar) were used to collect details for reviewing research articles.
Review protocol development : We first used Scopus to search the information and found many studies regarding this review. We then searched PubMed, IEEE, and Google scholar for articles and extracted only relevant papers matching our keywords and review context based on their full-text availability.
Review protocol evaluation : To support the selection of research articles and inclusion and exclusion criteria, the quality of articles was explored and assessed to appraise their suitability and impartiality [ 44 ]. Only articles with keywords “machine learning” and “clinical” in document titles and abstracts were selected.
Conducting the review
The second step is conducting the review, which includes a description of Search Syntax and data synthesis.
Search syntax Table 4 details the syntax used to select research articles.
Data synthesis
We used a qualitative meta-synthesis technique to understand the methodology, algorithms, applications, qualities, results, and current research impediments. Qualitative meta-synthesis is a coherent approach for analyzing data across qualitative studies [ 4 ]. Our first search identified 534,327 papers, comprising Scopus (24,498), IEEE (2,558), PubMed (11,271), and Google Scholar (496,000) articles with the selected keywords. After subjecting this dataset to our inclusion and exclusion criteria, articles were reduced to Scopus (181), IEEE (62), PubMed (37), and Google Scholar (46) (Fig. 3 ).
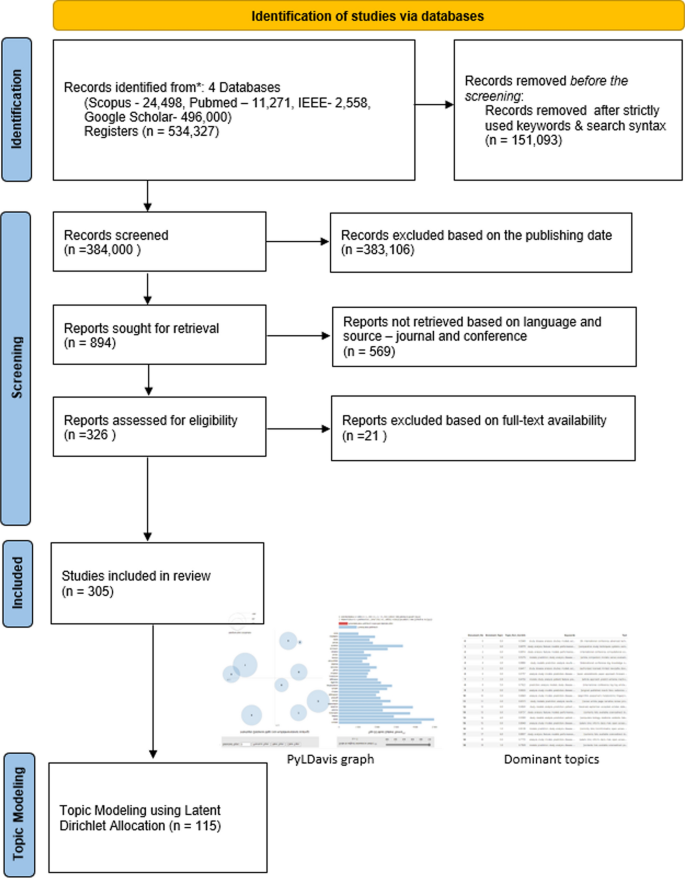
PRISMA framework of traditional literature review
Reporting the review
This section displays the result of the traditional literature review.
Demonstration of findings
A search including linear literature and citation chaining was acted in digital databases, and the resulted papers were thoroughly analyzed to choose only the most pertinent articles, at last, 305 articles were included for the Part B review. Information of such articles were classified, organized, and demonstrated to show the finding.
Report the findings
The word cloud is displayed on the selected 305 research articles which give an overview of the frequency of the word within those 305 research articles. The chosen articles are moved to the next step to perform the conversion of PDF files to text documents for performing LDA topic modeling (Fig. 4 ).
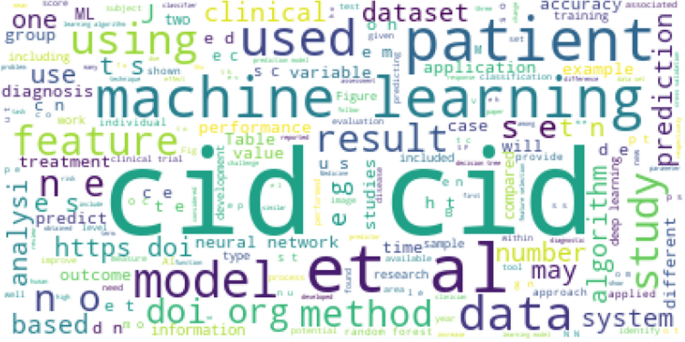
Word cloud on 305 articles
Conversion of pdf files to a text document
The Python coding is used to convert pdf files shared on GitHub https://github.com/MachineLearning-UON/Topic-modeling-using-LDA.git . The one text document is prepared with 305 research papers collected from a traditional literature review.
Topic modelling for intelligent literature review
Our intelligent literature review is developed using a combination of traditional literature review and topic modeling [ 22 ]. We use topic modeling—probability generating, a text-mining technique widely used in computer science for text mining and data recovery. Topic modeling is used in numerous papers to analyze [ 1 , 5 , 17 , 36 ] and use various ML algorithms [ 38 ] such as Latent Semantic Indexing (LSI), Latent Semantic Analysis (LSA), Latent Dirichlet Allocation (LDA), Non-Negative Matrix Factorization (NMF), Parallel Latent Dirichlet Allocation (PLDA), and Pachinko Allocation Model (PAM). We developed the LDA-based methodological framework so it would be most widely and easily used [ 13 , 17 , 21 ] as a very elementary [ 6 ] approach. LDA is an unsupervised and probabilistic ML algorithm that discovers topics by calculating patterns of word co-occurrence across many documents or corpus [ 16 ]. Each LDA topic is distributed across each document as a probability.
While there are numerous ways of conducting a systematic literature review, most strategies require a high expense of time and prior knowledge of the area in advance. This study examined the expense of various text categorization strategies, where the assumptions and cost of the strategy are analyzed [ 5 ]. Interestingly, except manually reading the articles and topic modeling, all the strategies require prior knowledge of the articles' categories and high pre-examination costs. However, topic modeling can be automated, alternate the utilization of researchers' time, demonstrating a perfect match for the utilization of topic modeling as a part of an Intelligent literature review. Topic modeling has been used in a few papers to categorize research papers presented in Table 5 .
The articles/papers in the above table analyzed are speeches, web documents, web posts, press releases, and newspapers. However, none of those have developed the framework to perform traditional literature reviews from digital databases then use topic modeling to save time. However, this research points out the utilization of LDA in academics and explores four parameters—text pre-processing, model parameters selection, reliability, and validity [ 5 ]. Topic modeling identifies patterns of the repetitive word across a corpus of documents. Patterns of word co-occurrence are conceived as hidden ‘topics’ available in the corpus. First, documents must be modified to be machine-readable, with only their most informative features used for topic modeling. We modify documents in a three-stage process entailing pre-processing, topic modeling, and post-processing, as defined in Fig. 1 earlier.
The utilization of topic modeling presents an opportunity for researchers to use advanced technology for the literature review process. Topic modeling has been utilized online and requires many statistical skills, which not all researchers have. Therefore, we have shared the codes in GitHub with the default parameter for future researchers.
Pre-processing
Székely and Brocke [ 46 ] explained that pre-processing is a seven-step process which explored below and mentioned in Fig. 1 as part B:
Load data—The text data file is imported using the python command.
Optical character recognition—using word cloud, characters are recognized.
Filtering non-English words—non-English words are removed.
Document tokenization—Split the text into sentences and the sentences into words. Lowercase the words and remove punctuation.
Text cleaning—the text has been cleaned using portstemmer.
Word lemmatization—words in the third person are changed to the first person, and past and future verb tenses are changed into the present.
Stop word removal—All stop words are removed.
Topic modelling using LDA
Several research articles have been selected to run LDA topic modeling, explained in Table 5 . LDA model results present the coherence score for all the selected topics and a list of the most frequently used words for each.
Post-processing
The goal of the post-processing stage is to identify and label topics and topics relevant for use in the literature review. The result of the LDA model is presented as a list of topics and probabilities of each document (paper). The list is utilized to assign a paper to a topic by arranging the list by the highest probability for each paper for each topic. All the topics contain documents that are like each other. To reduce the risk of error in topic identification, a combination of inspecting the most frequent words for each topic and a paper view is used. After the topic review, it will present in the literature review.
Following the intelligent literature review, results of the LDA model should be approved or validated by statistical, semantic, or predictive means. Statistical validation defines the mutual information tests of result fit to model assumptions; semantics validation requires hand-coding to decide if the importance of specific words varies significantly and as expected with tasks to different topics which is used in the current study to validate LDA model result; and predictive validation refers to checking if events that ought to have expanded the prevalence of particular topic if out interpretations are right, did so [ 6 , 21 ].
LDA defines that each word in each document comes from a topic, and the topic is selected from a set of keywords. So we have two matrices:
ϴtd = P(t|d) which is the probability distribution of topics in documents
Фwt = P(w|t), which is the probability distribution of words in topics
And, we can say that the probability of a word given document, i.e., P(w|d), is equal to:
where T is the total number of topics; likewise, let’s assume there are W keywords for all the documents.
If we assume conditional independence, we can say that
And hence P(w|d) is equal to
that is the dot product of ϴtd and Фwt for each topic t.
Our systematic literature review identified 305 research papers after performing a traditional literature review. After executing LDA topic modeling, only 115 articles show the relevancy with our topic "machine learning application in clinical domain'. The following stages present LDA topic modeling process.
The 305 research papers were stacked into a Python environment then converted into a single text file. The seven steps have been carried out, described earlier in Pre-processing .
- Topic modeling
The two main parameters of the LDA topic model are the dictionary (id2word)-dictionary and the corpus—doc_term_matrix. The LDA model is created by running the command:
# Creating the object for LDA model using gensim library
LDA = gensim.models.ldamodel.LdaModel
# Build LDA model
lda_model = LDA(corpus=doc_term_matrix, id2word = dictionary, num_topics=20, random_state=100,
chunksize = 1000, passes=50,iterations=100)
In this model, ‘num_topics’ = 20, ‘chunksize’ is the number of documents used in each training chunk, and ‘passes’ is the total number of training passes.
Firstly, the LDA model is built with 20 topics; each topic is represented by a combination of 20 keywords, with each keyword contributing a certain weight to a topic. Topics are viewed and interpreted in the LDA model, such as Topic 0, represented as below:
(0, '0.005*"analysis" + 0.005*"study" + 0.005*"models" + 0.004*"prediction" + 0.003*"disease" + 0.003*"performance" + 0.003*"different" + 0.003*"results" + 0.003*"patient" + 0.002*"feature" + 0.002*"system" + 0.002*"accuracy" + 0.002*"diagnosis" + 0.002*"classification" + 0.002*"studies" + 0.002*"medicine" + 0.002*"value" + 0.002*"approach" + 0.002*"variables" + 0.002*"review"'),
Our approach to finding the ideal number of topics is to construct LDA models with different numbers of topics as K and select the model with the highest coherence value. Selecting the ‘K' value that denotes the end of the rapid growth of topic coherence ordinarily offers significant and interpretable topics. Picking a considerably higher value can provide more granular sub-topics if the ‘K’ selection is too large, which can cause the repetition of keywords in multiple topics.
Model perplexity and topic coherence values are − 8.855378536321144 and 0.3724024189689453, respectively. To measure the efficiency of the LDA model is lower the perplexity, the better the model is. Topics and associated keywords were then examined in an interactive chart using the pyLDAvis package, which presents the topics are 20 and most salient terms in those 20 topics, but these 20 topics overlap each other as shown in Fig. 5 , which means the keywords are repeated in these 20 topics and topics are overlapped, which means so decided to use num_topics = 9 and presented PyLDAvis Figure below. Each bubble on the left-hand side plot represents a topic. The bigger the bubble is, the more predominant that topic is. A decent topic will have a genuinely big, non-overlapping bubble dispersed throughout the graph instead of grouped in one quadrant. A topic model with many topics will typically have many overlaps, small-sized bubbles clustered in one locale of the graph, as shown in Fig. 6 .
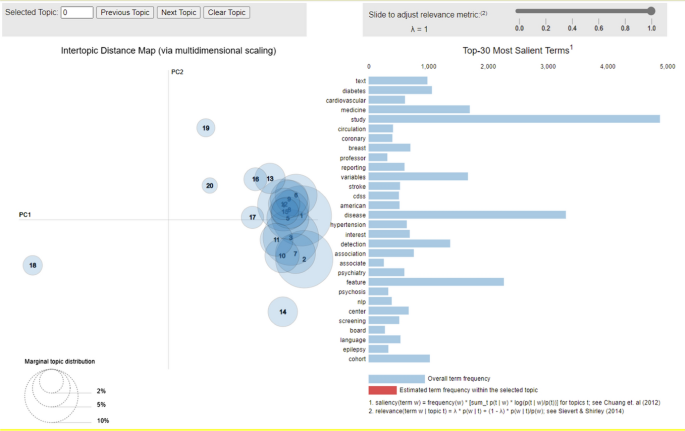
PyLDAvis graph with 20 topics in the clinical domain
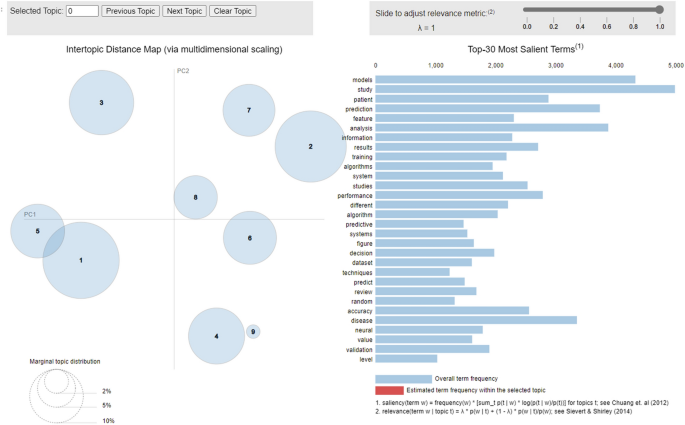
PyLDAvis graph with nine vital topics in the clinical domain
Each bubble addresses a generated topic. The larger the bubble, the higher percentage of the number of keywords in the corpus is about that topic which can be seen on the GitHub file. Blue bars address the general occurrence of each word in the corpus. If no topic is selected, the blue bars of the most frequently used words are displayed, as depicted in Fig. 6 .
The further the bubbles are away from each other, the more various they are. For example, we can tell that topic 1 is about patient information and studies utilized deep learning to analyze the disease, which can be seen in GitHub file codes ( https://github.com/MachineLearning-UON/Topic-modeling-using-LDA.git ) and presented in Fig. 7 .
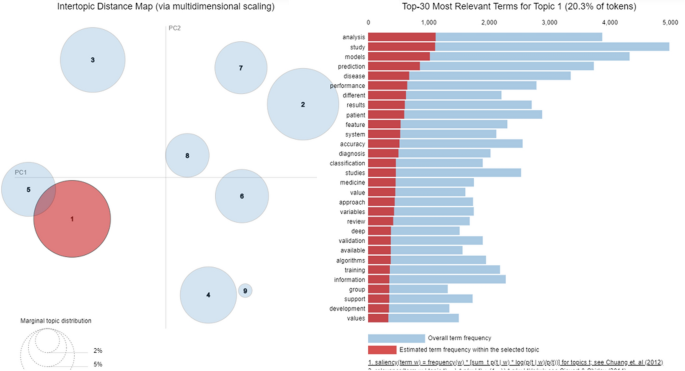
PyLDAvis graph with topic 1
Red bars give the assessed number of times a given topic produced a given term. As you can see from Fig. 7 , there are around 4000 of the word 'analysis', and this term is utilized 1000 times inside topic 1. The word with the longest red bar is the most used by the keywords having a place with that topic.
A good topic model will have big and non-overlapping bubbles dispersed throughout the chart. As we can see from Fig. 6 , the bubbles are clustered within one place. One of the practical applications of topic modeling is discovering the topic in a provided document. We find out the topic number with the highest percentage contribution in that document, as shown in Fig. 8 .
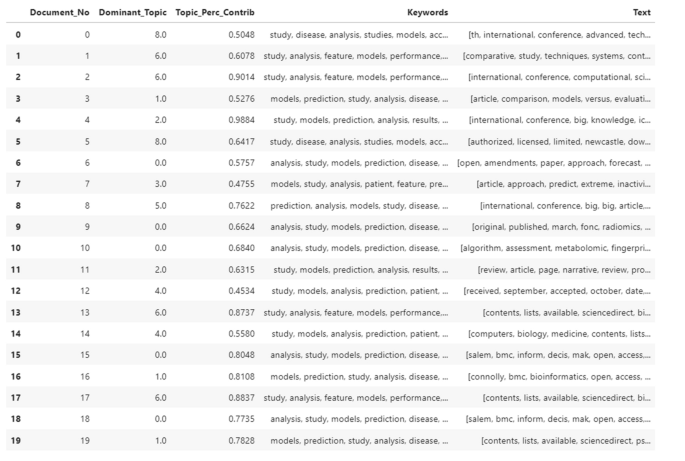
Dominant topics with topic percentage contribution
The next stage is to process the discoveries and find a satisfactory depiction of the topics. A combination of evaluating the most continuous words utilized to distinguish the topic. For example, the most frequent words for the papers in topic 2 are "study" and "analysis", which indicate frequent words for ML usage in the clinical domain.
The topic name is displayed with the topic number from 0 to 8, which represents in the Table 6 , which includes the Topic number and Topic words.
The result represents the percentage of the topics in all documents, which presents that topic 0 and topic 6 have the highest percentage and used in 58 and 57 documents, respectively, with 115 papers. The result of this research was an overview of the exploration areas inside the paper corpus, addressed by 9 topics.
This paper presented a new methodology that is uncommon in scholarly publications. The methodology utilizes ML to investigate sample articles/papers to distinguish research directions. Even though the structure of the ML-based methodology has its restrictions, the outcomes and its ease of use leave a promising future for topic modeling-based systematic literature reviews.
The principal benefit of the methodological framework is that it gives information about an enormous number of papers, with little effort on the researcher's part, before time-exorbitant manual work is to be finished. By utilizing the framework, it is conceivable to rapidly explore a wide range of paper corpora and assess where the researcher's time and concentration should be spent. This is particularly significant for a junior researcher with minimal earlier information on a research field. If default boundaries and cleaning settings can be found for the steps in the framework, a completely programmed gathering of papers could be empowered, where limited works have been introduced to accomplish an overview of research directions.
From a literature review viewpoint, the advantage of utilizing the proposed framework is that the inclusion and exclusion selection of papers for a literature review will be delayed to a later stage where more information is given, resulting in a more educated dynamic interaction. The framework empowers reproducibility, as every step can be reproduced in the systematic review process that ultimately empowers with transparency. The whole process has been demonstrated as a case concept on GitHub by future researchers.
The study has introduced an intelligent literature review framework that uses ML to analyze existing research documents or articles. We demonstrate how topic modeling can assist literature review by reducing the manual screening of huge quantities of literature for more efficient use of researcher time. An LDA algorithm provides default parameters and data cleaning steps, reducing the effort required to review literature. An additional advantage of our framework is that the intelligent literature review offers accurate results with little time, and it comprises traditional ways to analyze literature and LDA topic modeling.
This framework is constructed in a step-by-step manner. Researchers can use it efficiently because it requires less technical knowledge than other ML algorithms. There is no restriction on the quantity of the research papers it can measure. This research extends knowledge to similar studies in this field [ 12 , 22 , 23 , 26 , 30 , 46 ] which present topic modeling. The study acknowledges the inspiring concept of smart literature defined by Asmussen and Møller [ 3 ]. The researchers previously provided a brief description of how LDA is utilized in topic modeling. Our research followed the basic idea but enhanced its significance to broaden its scale and focus on a specific domain such as the clinical domain to produce insights from existing research articles. For instance, Székely and Vom [ 46 ] utilized natural language processing to analyze 9514 sustainability reports published between 1999 and 2015. They identified 42 topics but did not develop any framework for future researchers. This was considered a significant gap in the research. Similarly, Kushwaha et al. [ 22 ] used a network analysis approach to analyze 10-year papers without providing any clear transparent outcome (e.g., how the research step-by-step produces an outcome). Likewise, Asmussen and Møller [ 3 ] developed a smart literature review framework that was limited to analyzing 650 sample articles through a single method. However, in our research, we developed an intelligent literature review that combines traditional and LDA topic modeling, so that future researchers can get assistance to gain effective knowledge regarding literature review when it becomes a state-of-the-art in research domains.
Our research developed a more effective intelligent framework, which combines traditional literature review and topic modeling using LDA, which provides more accurate and transparent results. The results are shared via public access on GitHub using this link https://github.com/MachineLearning-UON/Topic-modeling-using-LDA.git .
This paper focused on creating a methodological framework to empower researchers, diminishing the requirement for manually scanning documents and assigning the possibility to examine practically limitless. It would assist in capturing insights of an enormous number of papers quicker, more transparently, with more reliability. The proposed framework utilizes the LDA's topic model, which gathers related documents into topics.
A framework employed topic modeling for rapidly and reliably investigating a limitless number of papers, reducing their need to read individually, is developed. Topic modeling using the LDA algorithm can assist future researchers as they often need an outline of various research fields with minimal pre-existing knowledge. The proposed framework can empower researchers to review more papers in less time with more accuracy. Our intelligent literature review framework includes a holistic literature review process (conducting, planning, and reporting the review) and an LDA topic modeling (pre-processing, topic modeling, and post-processing stages), which conclude the results of 115 research articles are relevant to the search.
The automation of topic modeling with default parameters could also be explored to benefit non-technical researchers to explore topics or related keywords in any problem domain. For future directions, the principal points should be addressed. Future researchers in other research fields should apply the proposed framework to acquire information about the practical usage and gain ideas for additional advancement of the framework. Furthermore, research in how to consequently specify model parameters could extraordinarily enhance the ease of use for the utilization of topic modeling for non-specialized researchers, as the determination of model parameters enormously affects the outcome of the framework.
Future research may be utilized more ML analytics tools as complete solution artifacts to analyze different forms of big data. This could be adopting design science research methodologies for benefiting design researchers who are interested in building ML-based artifacts [ 15 , 28 , 29 , 31 , 32 , 33 ].
Availability of data and materials
Data will be supplied upon request.
LDA is a probabilistic method for topic modeling in text analysis, providing both a predictive and latent topic representation.
Abbreviations
The Institute of Electrical and Electronics Engineers
- Machine learning
- Latent Dirichlet Allocation
Organizational Capacity
Latent Semantic Indexing
Latent Semantic Analysis
Non-Negative Matrix Factorization
Parallel Latent Dirichlet Allocation
Pachinko Allocation Model
Abuhay TM, Kovalchuk SV, Bochenina K, Mbogo G-K, Visheratin AA, Kampis G, et al. Analysis of publication activity of computational science society in 2001–2017 using topic modelling and graph theory. J Comput Sci. 2018;26:193–204.
Article Google Scholar
Adlung L, Cohen Y, Mor U, Elinav E. Machine learning in clinical decision making. Med. 2021;2(6):642–65.
Asmussen CB, Møller C. Smart literature review: a practical topic modeling approach to exploratory literature review. J Big Data. 2019;6(1):1–18.
Beck CT. A meta-synthesis of qualitative research. MCN Am J Mater Child Nurs. 2002;27(4):214–21.
Behera RK, Bala PK, Dhir A. The emerging role of cognitive computing in healthcare: a systematic literature review. Int J Med Informatics. 2019;129:154–66.
Blei DM. Probabilistic topic models. Commun ACM. 2012;55(4):77–84.
Blei DM, Ng AY, Jordan MI. Latent Dirichlet allocation. J Mach Learn Res. 2003;3:993–1022.
MATH Google Scholar
Brnabic A, Hess LM. Systematic literature review of machine learning methods used in the analysis of real-world data for patient-provider decision making. BMC Med Inform Decis Mak. 2021;21(1):1–19.
Cabitza F, Locoro A, Banfi G. Machine learning in orthopedics: a literature review. Front Bioeng Biotechnol. 2018;6:75.
Chang C-H, Lin C-H, Lane H-Y. Machine learning and novel biomarkers for the diagnosis of Alzheimer’s disease. Int J Mol Sci. 2021;22(5):2761.
Connor KL, O’Sullivan ED, Marson LP, Wigmore SJ, Harrison EM. The future role of machine learning in clinical transplantation. Transplantation. 2021;105(4):723–35.
Dias R, Torkamani A. Artificial intelligence in clinical and genomic diagnostics. Genome Med. 2019;11(1):1–12.
DiMaggio P, Nag M, Blei D. Exploiting affinities between topic modeling and the sociological perspective on culture: application to newspaper coverage of US government arts funding. Poetics. 2013;41(6):570–606.
Forest P-G, Martin D. Fit for Purpose: Findings and recommendations of the external review of the Pan-Canadian Health Organizations: Summary Report: Health Canada Ottawa, ON; 2018.
Genemo H, Miah SJ, McAndrew A. A design science research methodology for developing a computer-aided assessment approach using method marking concept. Educ Inf Technol. 2016;21(6):1769–84.
Greene D, Cross JP. Exploring the political agenda of the european parliament using a dynamic topic modeling approach. Polit Anal. 2017;25(1):77–94.
Grimmer J. A Bayesian hierarchical topic model for political texts: measuring expressed agendas in Senate press releases. Polit Anal. 2010;18(1):1–35.
Grimmer J, Stewart BM. Text as data: the promise and pitfalls of automatic content analysis methods for political texts. Polit Anal. 2013;21(3):267–97.
Hassan N, Slight R, Weiand D, Vellinga A, Morgan G, Aboushareb F, et al. Preventing sepsis; how can artificial intelligence inform the clinical decision-making process? A systematic review. Int J Med Inform. 2021;150:104457.
Hirt R, Koehl NJ, Satzger G, editors. An end-to-end process model for supervised machine learning classification: from problem to deployment in information systems. Designing the Digital Transformation: DESRIST 2017 Research in Progress Proceedings of the 12th International Conference on Design Science Research in Information Systems and Technology Karlsruhe, Germany 30 May-1 Jun; 2017: Karlsruher Institut für Technologie (KIT).
Koltsova O, Koltcov S. Mapping the public agenda with topic modeling: the case of the Russian live journal. Policy Internet. 2013;5(2):207–27.
Kushwaha AK, Kar AK, Dwivedi YK. Applications of big data in emerging management disciplines: a literature review using text mining. Int J Inf Manag Data Insights. 2021;1(2):100017.
Google Scholar
Li S, Wang H. Traditional literature review and research synthesis. The Palgrave handbook of applied linguistics research methodology. 2018:123–44.
Magrabi F, Ammenwerth E, McNair JB, De Keizer NF, Hyppönen H, Nykänen P, et al. Artificial intelligence in clinical decision support: challenges for evaluating AI and practical implications. Yearb Med Inform. 2019;28(01):128–34.
Maier D, Waldherr A, Miltner P, Wiedemann G, Niekler A, Keinert A, et al. Applying LDA topic modeling in communication research: toward a valid and reliable methodology. Commun Methods Meas. 2018;12(2–3):93–118.
Mårtensson G, Ferreira D, Granberg T, Cavallin L, Oppedal K, Padovani A, et al. The reliability of a deep learning model in clinical out-of-distribution MRI data: a multicohort study. Med Image Anal. 2020;66:101714.
Mendo IR, Marques G, de la Torre DI, López-Coronado M, Martín-Rodríguez F. Machine learning in medical emergencies: a systematic review and analysis. J Med Syst. 2021;45(10):1–16.
Miah SJ. An ontology based design environment for rural business decision support. Nathan: Griffith University Nathan; 2008.
Miah SJ, A new semantic knowledge sharing approach for e-government systems. 4th IEEE International Conference on Digital Ecosystems and Technologies; 2010: IEEE.
Miah SJ, Camilleri E, Vu HQ. Big Data in healthcare research: a survey study. J Comput Inf Syst. 2021. https://doi.org/10.1080/08874417.2020.1858727 .
Miah SJ, Gammack J, Kerr D, Ontology development for context-sensitive decision support. Third International Conference on Semantics, Knowledge and Grid (SKG 2007); 2007: IEEE.
Miah SJ, Gammack JG. Ensemble artifact design for context sensitive decision support. Australas J Inf Syst. 2014. https://doi.org/10.3127/ajis.v18i2.898 .
Miah SJ, Gammack JG, McKay J. A metadesign theory for tailorable decision support. J Assoc Inf Syst. 2019;20(5):4.
Mimno D, Blei D, editors. Bayesian checking for topic models. Proceedings of the 2011 conference on empirical methods in natural language processing; 2011.
Oala L, Murchison AG, Balachandran P, Choudhary S, Fehr J, Leite AW, et al. Machine learning for health: algorithm auditing & quality control. J Med Syst. 2021;45(12):1–8.
Ouhbi S, Idri A, Fernández-Alemán JL, Toval A. Requirements engineering education: a systematic mapping study. Requir Eng. 2015;20(2):119–38.
Page MJ, McKenzie JE, Bossuyt PM, Boutron I, Hoffmann TC, Mulrow CD, et al. The PRISMA 2020 statement: an updated guideline for reporting systematic reviews. BMJ. 2020;372:n71.
Quinn KM, Monroe BL, Colaresi M, Crespin MH, Radev DR. How to analyze political attention with minimal assumptions and costs. Am J Polit Sci. 2010;54(1):209–28.
Rowley J, Slack F. Conducting a literature review. Management research news. 2004.
Rozas LW, Klein WC. The value and purpose of the traditional qualitative literature review. J Evid Based Soc Work. 2010;7(5):387–99.
Sabharwal R, Miah SJ. A new theoretical understanding of big data analytics capabilities in organizations: a thematic analysis. J Big Data. 2021;8(1):1–17.
Salazar-Reyna R, Gonzalez-Aleu F, Granda-Gutierrez EM, Diaz-Ramirez J, Garza-Reyes JA, Kumar A. A systematic literature review of data science, data analytics and machine learning applied to healthcare engineering systems. Management Decision. 2020.
Shah P, Kendall F, Khozin S, Goosen R, Hu J, Laramie J, et al. Artificial intelligence and machine learning in clinical development: a translational perspective. NPJ Digit Med. 2019;2(1):1–5.
Sone D, Beheshti I. Clinical application of machine learning models for brain imaging in epilepsy: a review. Front Neurosci. 2021;15:761.
Spasic I, Nenadic G. Clinical text data in machine learning: systematic review. JMIR Med Inform. 2020;8(3):e17984.
Székely N, Vom Brocke J. What can we learn from corporate sustainability reporting? Deriving propositions for research and practice from over 9,500 corporate sustainability reports published between 1999 and 2015 using topic modelling technique. PLoS ONE. 2017;12(4):e0174807.
Verma D, Bach K, Mork PJ, editors. Application of machine learning methods on patient reported outcome measurements for predicting outcomes: a literature review. Informatics; 2021: Multidisciplinary Digital Publishing Institute.
Weng W-H. Machine learning for clinical predictive analytics. Leveraging data science for global health. Cham: Springer; 2020. p. 199–217.
Book Google Scholar
Yin Z, Sulieman LM, Malin BA. A systematic literature review of machine learning in online personal health data. J Am Med Inform Assoc. 2019;26(6):561–76.
Download references
Acknowledgements
Not applicable.
Author information
Authors and affiliations.
Newcastle Business School, The University of Newcastle, Newcastle, NSW, Australia
Renu Sabharwal & Shah J. Miah
You can also search for this author in PubMed Google Scholar
Contributions
The first author conducted the research, while the second author has ensured quality standards and rewritten the entire findings linking to underlying theories. Both authors read and approved the final manuscript.
Corresponding author
Correspondence to Renu Sabharwal .
Ethics declarations
Ethics approval and consent to participate, consent for publication, competing interests, additional information, publisher's note.
Springer Nature remains neutral with regard to jurisdictional claims in published maps and institutional affiliations.
Rights and permissions
Open Access This article is licensed under a Creative Commons Attribution 4.0 International License, which permits use, sharing, adaptation, distribution and reproduction in any medium or format, as long as you give appropriate credit to the original author(s) and the source, provide a link to the Creative Commons licence, and indicate if changes were made. The images or other third party material in this article are included in the article's Creative Commons licence, unless indicated otherwise in a credit line to the material. If material is not included in the article's Creative Commons licence and your intended use is not permitted by statutory regulation or exceeds the permitted use, you will need to obtain permission directly from the copyright holder. To view a copy of this licence, visit http://creativecommons.org/licenses/by/4.0/ .
Reprints and permissions
About this article
Cite this article.
Sabharwal, R., Miah, S.J. An intelligent literature review: adopting inductive approach to define machine learning applications in the clinical domain. J Big Data 9 , 53 (2022). https://doi.org/10.1186/s40537-022-00605-3
Download citation
Received : 18 November 2021
Accepted : 06 April 2022
Published : 28 April 2022
DOI : https://doi.org/10.1186/s40537-022-00605-3
Share this article
Anyone you share the following link with will be able to read this content:
Sorry, a shareable link is not currently available for this article.
Provided by the Springer Nature SharedIt content-sharing initiative
- Clinical research
- Systematic literature review
Thank you for visiting nature.com. You are using a browser version with limited support for CSS. To obtain the best experience, we recommend you use a more up to date browser (or turn off compatibility mode in Internet Explorer). In the meantime, to ensure continued support, we are displaying the site without styles and JavaScript.
- View all journals
- Explore content
- About the journal
- Publish with us
- Sign up for alerts
- CAREER FEATURE
- 04 December 2020
- Correction 09 December 2020
How to write a superb literature review
Andy Tay is a freelance writer based in Singapore.
You can also search for this author in PubMed Google Scholar
Literature reviews are important resources for scientists. They provide historical context for a field while offering opinions on its future trajectory. Creating them can provide inspiration for one’s own research, as well as some practice in writing. But few scientists are trained in how to write a review — or in what constitutes an excellent one. Even picking the appropriate software to use can be an involved decision (see ‘Tools and techniques’). So Nature asked editors and working scientists with well-cited reviews for their tips.
Access options
Access Nature and 54 other Nature Portfolio journals
Get Nature+, our best-value online-access subscription
24,99 € / 30 days
cancel any time
Subscribe to this journal
Receive 51 print issues and online access
185,98 € per year
only 3,65 € per issue
Rent or buy this article
Prices vary by article type
Prices may be subject to local taxes which are calculated during checkout
doi: https://doi.org/10.1038/d41586-020-03422-x
Interviews have been edited for length and clarity.
Updates & Corrections
Correction 09 December 2020 : An earlier version of the tables in this article included some incorrect details about the programs Zotero, Endnote and Manubot. These have now been corrected.
Hsing, I.-M., Xu, Y. & Zhao, W. Electroanalysis 19 , 755–768 (2007).
Article Google Scholar
Ledesma, H. A. et al. Nature Nanotechnol. 14 , 645–657 (2019).
Article PubMed Google Scholar
Brahlek, M., Koirala, N., Bansal, N. & Oh, S. Solid State Commun. 215–216 , 54–62 (2015).
Choi, Y. & Lee, S. Y. Nature Rev. Chem . https://doi.org/10.1038/s41570-020-00221-w (2020).
Download references
Related Articles
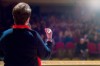
- Research management
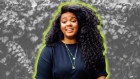
Defying the stereotype of Black resilience
Career Q&A 30 MAY 24
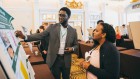
How I overcame my stage fright in the lab
Career Column 30 MAY 24
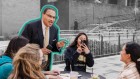
I had my white colleagues walk in a Black student’s shoes for a day
Career Q&A 28 MAY 24
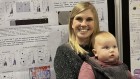
Researcher parents are paying a high price for conference travel — here’s how to fix it
Career Column 27 MAY 24
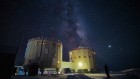
How researchers in remote regions handle the isolation
Career Feature 24 MAY 24
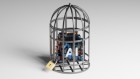
Guidelines for academics aim to lessen ethical pitfalls in generative-AI use
Nature Index 22 MAY 24
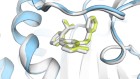
Who will make AlphaFold3 open source? Scientists race to crack AI model
News 23 MAY 24
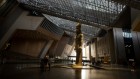
Egypt is building a $1-billion mega-museum. Will it bring Egyptology home?
News Feature 22 MAY 24
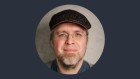
Pay researchers to spot errors in published papers
World View 21 MAY 24
Postdoctoral Associate - Amyloid Strain Differences in Alzheimer's Disease
Houston, Texas (US)
Baylor College of Medicine (BCM)
Postdoctoral Associate- Bioinformatics of Alzheimer's disease
Postdoctoral associate- alzheimer's gene therapy, postdoctoral associate, kaust global postdoctoral fellowship.
The KAUST Global Fellowship Program is designed to attract emerging research leaders working across areas under the four research priorities of KAUST.
Saudi Arabia (SA)
King Abdullah University of Science and Technology (KAUST
Sign up for the Nature Briefing newsletter — what matters in science, free to your inbox daily.
Quick links
- Explore articles by subject
- Guide to authors
- Editorial policies
Literature review: your definitive guide

Joanna Wilkinson
This is our ultimate guide on how to write a narrative literature review. It forms part of our Research Smarter series .
How do you write a narrative literature review?
Researchers worldwide are increasingly reliant on literature reviews. That’s because review articles provide you with a broad picture of the field, and help to synthesize published research that’s expanding at a rapid pace .
In some academic fields, researchers publish more literature reviews than original research papers. The graph below shows the substantial growth of narrative literature reviews in the Web of Science™, alongside the percentage increase of reviews when compared to all document types.
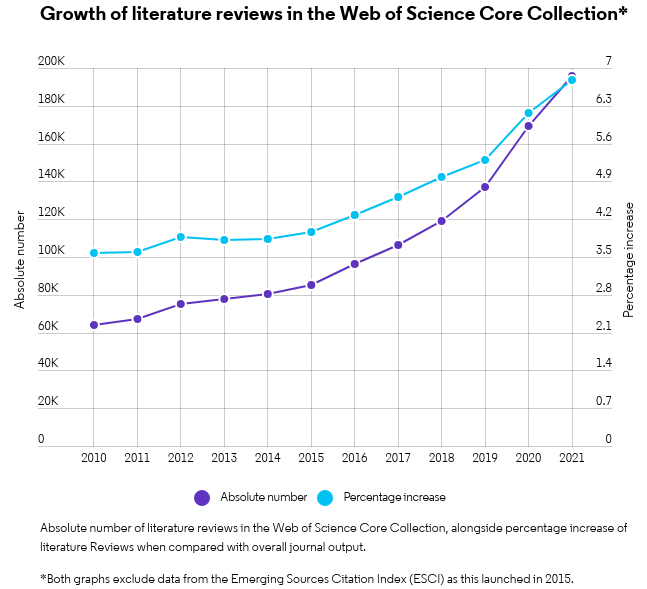
It’s critical that researchers across all career levels understand how to produce an objective, critical summary of published research. This is no easy feat, but a necessary one. Professionally constructed literature reviews – whether written by a student in class or an experienced researcher for publication – should aim to add to the literature rather than detract from it.
To help you write a narrative literature review, we’ve put together some top tips in this blog post.
Best practice tips to write a narrative literature review:
- Don’t miss a paper: tips for a thorough topic search
- Identify key papers (and know how to use them)
- Tips for working with co-authors
- Find the right journal for your literature review using actual data
- Discover literature review examples and templates
We’ll also provide an overview of all the products helpful for your next narrative review, including the Web of Science, EndNote™ and Journal Citation Reports™.
1. Don’t miss a paper: tips for a thorough topic search
Once you’ve settled on your research question, coming up with a good set of keywords to find papers on your topic can be daunting. This isn’t surprising. Put simply, if you fail to include a relevant paper when you write a narrative literature review, the omission will probably get picked up by your professor or peer reviewers. The end result will likely be a low mark or an unpublished manuscript, neither of which will do justice to your many months of hard work.
Research databases and search engines are an integral part of any literature search. It’s important you utilize as many options available through your library as possible. This will help you search an entire discipline (as well as across disciplines) for a thorough narrative review.
We provide a short summary of the various databases and search engines in an earlier Research Smarter blog . These include the Web of Science , Science.gov and the Directory of Open Access Journals (DOAJ).
Like what you see? Share it with others on Twitter:
[bctt tweet=”Writing a #LiteratureReview? Check out the latest @clarivateAG blog for top tips (from topic searches to working with coauthors), examples, templates and more”]
Searching the Web of Science
The Web of Science is a multidisciplinary research engine that contains over 170 million papers from more than 250 academic disciplines. All of the papers in the database are interconnected via citations. That means once you get started with your keyword search, you can follow the trail of cited and citing papers to efficiently find all the relevant literature. This is a great way to ensure you’re not missing anything important when you write a narrative literature review.
We recommend starting your search in the Web of Science Core Collection™. This database covers more than 21,000 carefully selected journals. It is a trusted source to find research papers, and discover top authors and journals (read more about its coverage here ).
Learn more about exploring the Core Collection in our blog, How to find research papers: five tips every researcher should know . Our blog covers various tips, including how to:
- Perform a topic search (and select your keywords)
- Explore the citation network
- Refine your results (refining your search results by reviews, for example, will help you avoid duplication of work, as well as identify trends and gaps in the literature)
- Save your search and set up email alerts
Try our tips on the Web of Science now.
2. Identify key papers (and know how to use them)
As you explore the Web of Science, you may notice that certain papers are marked as “Highly Cited.” These papers can play a significant role when you write a narrative literature review.
Highly Cited papers are recently published papers getting the most attention in your field right now. They form the top 1% of papers based on the number of citations received, compared to other papers published in the same field in the same year.
You will want to identify Highly Cited research as a group of papers. This group will help guide your analysis of the future of the field and opportunities for future research. This is an important component of your conclusion.
Writing reviews is hard work…[it] not only organizes published papers, but also positions t hem in the academic process and presents the future direction. Prof. Susumu Kitagawa, Highly Cited Researcher, Kyoto University
3. Tips for working with co-authors
Writing a narrative review on your own is hard, but it can be even more challenging if you’re collaborating with a team, especially if your coauthors are working across multiple locations. Luckily, reference management software can improve the coordination between you and your co-authors—both around the department and around the world.
We’ve written about how to use EndNote’s Cite While You Write feature, which will help you save hundreds of hours when writing research . Here, we discuss the features that give you greater ease and control when collaborating with your colleagues.
Use EndNote for narrative reviews
Sharing references is essential for successful collaboration. With EndNote, you can store and share as many references, documents and files as you need with up to 100 people using the software.
You can share simultaneous access to one reference library, regardless of your colleague’s location or organization. You can also choose the type of access each user has on an individual basis. For example, Read-Write access means a select colleague can add and delete references, annotate PDF articles and create custom groups. They’ll also be able to see up to 500 of the team’s most recent changes to the reference library. Read-only is also an option for individuals who don’t need that level of access.
EndNote helps you overcome research limitations by synchronizing library changes every 15 minutes. That means your team can stay up-to-date at any time of the day, supporting an easier, more successful collaboration.
Start your free EndNote trial today .
4.Finding a journal for your literature review
Finding the right journal for your literature review can be a particular pain point for those of you who want to publish. The expansion of scholarly journals has made the task extremely difficult, and can potentially delay the publication of your work by many months.
We’ve written a blog about how you can find the right journal for your manuscript using a rich array of data. You can read our blog here , or head straight to Endnote’s Manuscript Matcher or Journal Citation Report s to try out the best tools for the job.
5. Discover literature review examples and templates
There are a few tips we haven’t covered in this blog, including how to decide on an area of research, develop an interesting storyline, and highlight gaps in the literature. We’ve listed a few blogs here that might help you with this, alongside some literature review examples and outlines to get you started.
Literature Review examples:
- Aggregation-induced emission
- Development and applications of CRISPR-Cas9 for genome engineering
- Object based image analysis for remote sensing
(Make sure you download the free EndNote™ Click browser plugin to access the full-text PDFs).
Templates and outlines:
- Learn how to write a review of literature , Univ. of Wisconsin – Madison
- Structuring a literature review , Australian National University
- Matrix Method for Literature Review: The Review Matrix , Duquesne University
Additional resources:
- Ten simple rules for writing a literature review , Editor, PLoS Computational Biology
- Video: How to write a literature review , UC San Diego Psychology
Related posts
Journal citation reports 2024 preview: unified rankings for more inclusive journal assessment.

Introducing the Clarivate Academic AI Platform

Reimagining research impact: Introducing Web of Science Research Intelligence
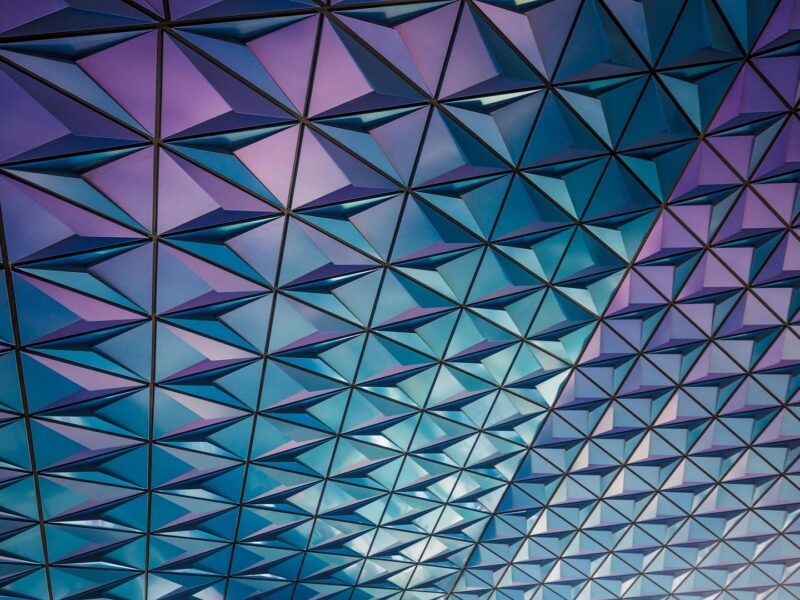

An official website of the United States government
The .gov means it’s official. Federal government websites often end in .gov or .mil. Before sharing sensitive information, make sure you’re on a federal government site.
The site is secure. The https:// ensures that you are connecting to the official website and that any information you provide is encrypted and transmitted securely.
- Publications
- Account settings
Preview improvements coming to the PMC website in October 2024. Learn More or Try it out now .
- Advanced Search
- Journal List
- Sensors (Basel)
- PMC10255695

Data Science Methods and Tools for Industry 4.0: A Systematic Literature Review and Taxonomy
Helder moreira arruda.
1 Applied Computing Graduate Program, University of Vale do Rio dos Sinos, 950, Unisinos Av., São Leopoldo 93022-000, RS, Brazil; rb.sonisinu.ude@bnomisr (R.S.B.); rb.sonisinu@tsnukleafar (R.K.)
Rodrigo Simon Bavaresco
Rafael kunst, elvis fernandes bugs.
2 HT Micron Semiconductors S.A., 1550, Unisinos Av., São Leopoldo 93022-750, RS, Brazil; [email protected] (E.F.B.); [email protected] (G.C.P.)
Giovani Cheuiche Pesenti
Jorge luis victória barbosa.
The Fourth Industrial Revolution, also named Industry 4.0, is leveraging several modern computing fields. Industry 4.0 comprises automated tasks in manufacturing facilities, which generate massive quantities of data through sensors. These data contribute to the interpretation of industrial operations in favor of managerial and technical decision-making. Data science supports this interpretation due to extensive technological artifacts, particularly data processing methods and software tools. In this regard, the present article proposes a systematic literature review of these methods and tools employed in distinct industrial segments, considering an investigation of different time series levels and data quality. The systematic methodology initially approached the filtering of 10,456 articles from five academic databases, 103 being selected for the corpus. Thereby, the study answered three general, two focused, and two statistical research questions to shape the findings. As a result, this research found 16 industrial segments, 168 data science methods, and 95 software tools explored by studies from the literature. Furthermore, the research highlighted the employment of diverse neural network subvariations and missing details in the data composition. Finally, this article organized these results in a taxonomic approach to synthesize a state-of-the-art representation and visualization, favoring future research studies in the field.
1. Introduction
A way of better understanding the current civilization is through the industrial revolution timeline. The first phase of this movement began in the late 18th century, based on the evolution of mechanical equipment for manufacturing and the emergence of steam machines. Then, at the beginning of the 20th century, the possibility of implementing large-scale production based on task division started the second phase of the industrial revolution with the advent of electricity. Afterward, in the early 1970s, the usage of electronics associated with information technology enabled the automation of manufacturing processes, establishing the third phase of this movement [ 1 ]. Today, the world lives the so-called new wave of the industrial revolution which started in Europe and spread worldwide [ 2 ]. The fourth phase of this revolution, named Industry 4.0, employs technological advances and concepts such as the Internet of things (IoT) and cyberphysical systems (CPS) to assist in the development of smart factories [ 3 , 4 ].
Along with the aforesaid advances, the expression “Data Science” began to be discussed by the information technology community in the first decade of the 21st century. Data scientists are people who deal with significant quantities of data from different sources to extract relevant information in decision-making [ 5 ]. One of data science’s main goals is to predict outcomes considering the domain knowledge of interest [ 6 ]. A successful data scientist must have a perspective of business problems, in addition to the knowledge of data mining algorithms, computational methods, and software tools to extract knowledge and insights from big datasets [ 7 ].
Frequently, these datasets organize observations in high dimensionality with various data types, formats, and sizes. In this sense, one of the most frequent ways to deal with this information is in the time domain. Observations sampled in the time domain constitute a sequence of information named time series [ 8 ]. Time series may receive diverse processing methods to understand machinery maintenance, production life cycle, and industrial and business processes to generate valuable outcomes for companies. Moreover, time series allow the aggregation, combination, and computational processing of data to create higher information levels, such as contextual data [ 9 ]. Context, in turn, features a situation regarding individuals, applications, and the surrounding environment. Contexts represent the time and the state of something that can be an object, a machine, a system, a person, or a group.
In this regard, the literature presents systematic reviews encompassing the aforementioned scope similar to this study. Manufacturing has generated research studies to deal with decision-making problems using analytical techniques, data mining, and machine learning [ 10 ]. Moreover, a review of big data tools and applications for manufacturing presented the essential components to create complete solutions [ 11 ]. In addition to case studies applied to a chemical company, a review of data mining and analytical categories such as predictive, inquisitive, descriptive, and prescriptive categories focused on manufacturing processes [ 12 ]. However, these reviews do not retrieve and analyze data science methods and software tools focused on general industrial applications. This article proposes a systematic literature review of data science methods and tools employed in distinct segments of the industry. Moreover, the study analyses the usage of different time series levels and data quality concerning data science applications. In this sense, the article provides the answers to three general, two focused, and two statistical questions to synthesize the literature through a taxonomy, favoring the findings’ representation.
The remainder of this article has the following structure. Section 2 describes related works and how this study differentiates from them. Section 3 explains the methodology employed in the systematic review. Section 4 presents the results and the findings based on the research questions, highlighting industrial segments, data science methods, and software tools. Section 5 depicts the proposed taxonomy to represent the findings covered by the literature, and Section 6 discusses the findings. Finally, Section 7 approaches the limitations, future work, and conclusions of this study.
2. Related Work
This section analyzes surveys and reviews in comparison to the proposed work. Over the last years, some authors have reviewed the literature, aiming to exploit the best techniques used by smart factories that correspond to the data science field. This is because Industry 4.0 allows the employment of multiple types of technologies in different segments of manufacturing.
Mazzei and Ramjattan [ 13 ] used natural language processing techniques to review machine learning methods used in Industry 4.0 cases. The authors stated questions regarding Industry 4.0 main problems, which machine learning methods were used in these situations, and how the areas focused on the academic literature and white papers. The systematic review focused on two databases using the topic modeling technique BERTopic. The most recurrent problems regarded security, smart production, IoT connectivity, service optimization, robotic automation, and logistics optimization. Convolutional neural networks were the most frequent machine learning method.
Wolf et al. [ 10 ] studied the lack of management tools oriented toward decision-making problems in the manufacturing domain. The work provided a systematic mapping review that identified seven application areas for data analytics and had advanced analytical techniques associated with each area. The mapping originated a novel tool to ease decision-making that identified promising analytic projects. Moreover, the management tool employed data mining techniques and machine learning algorithms.
Cui et al. [ 11 ] published a systematic literature review aiming to classify big data tools with similarities and identify the differences among them. The work took into account industrial data, big data technologies, and data applications in manufacturing. The conceptual framework of the systematic literature review had three perspectives: data source, big data ecosystem, and the data consumer. Data types, source devices, data dynamics, data formats, and systems composed the data source perspective. The big data ecosystem perspective presented data aspects as storage, resource management, visualization, analysis, database, data warehouse, search, query, processing, ingestion, data flow, workflow, and management. Prediction, optimization, monitoring, design, decision support, data analytics, scheduling, data management, simulation, and quality control were part of the components of the data consumer perspective. Four research questions featured the drivers and requirements for big data applications, the essential components of the big data ecosystem, the capabilities of big data ecosystems, and the future directions of big data applications. In conclusion, the authors found six key drivers and nine essential components of the big data ecosystem. The study did not find any enterprise-ready big data solution in the literature.
Belhadi et al. [ 12 ] systematically reviewed the literature regarding big data analytics in manufacturing processes in addition to multiple case studies applied to a leading chemical company. The three cases were part of a digital transformation project, the first case being an implementation of big data analytics in a fertilizer plant, the second in a phosphoric acid company, and the third one, an intelligent and self-controlled production unit. The article classified the selected works according to data mining and analytics categories: predictive, inquisitive, descriptive, and prescriptive. Moreover, the implemented techniques categorized papers into offline and real-online. Moreover, the work established the following research trends: real-time data mining approaches, big data analytics enabler architecture, integrated human-data intelligence, and prescriptive analytics. Each research trend pointed to the research questions regarding performance management, production control, and maintenance in manufacturing processes. The authors realized that the emergence of advanced technologies, particularly sensors, generated data with a wide variability, large variety, high velocity, intense volatility, high volume, unascertained veracity, and low value. Furthermore, the study proposed a framework of big data analytics in the manufacturing process, which presented the process challenges, faculties, and capabilities of big data analytics.
None of the related works retrieved and analyzed data science methods and software tools focused on industrial applications ( Table 1 ). Therefore, this article identifies and organizes industrial segments, data science methods, and software tools employed in industrial environments to produce a taxonomy. In turn, the taxonomy synthesizes the literature favoring the representation of the findings. For this, the article describes a systematic literature review converging towards three main themes: Industry 4.0, data science, and time series. These themes are the basis to create general, focused, and statistical questions that shape this work’s investigation. In this sense, the study also investigates specific approaches derived from these themes, particularly the usage of context and the data quality employed in studies. These aspects provide the differential approach of this article regarding the aforementioned reviews.
Related works and the presence of data science methods and tools compared to this work.
3. Methodology
This section presents the research methods employed in this work. The structure follows the methodology proposed by Petersen [ 14 ]. Figure 1 summarizes the stages organized into four steps with three substeps each. First, the stages encompass the research planning, followed by the execution of the systematic review, analysis of the data, and reporting of the results.

Sequence of the four stages of the research: planning, execution, analysis and reporting. Each stage is organized into three substeps.
3.1. Research Planning
The research planning establishes the objectives, defines the research questions, and plans the selection of the studies. The following subsections explain each step in detail.
3.1.1. Objectives
A systematic review of the state of the art in data science methods and tools employed in Industry 4.0 is the central aspect of this article. The goal was to find studies that employ Industry 4.0, data science, and time series to produce useful insights for the industrial field. After collecting the papers, the objectives concerned the classification of each study according to the industrial segments, data science methods, and software tools. Afterward, this work synthesized the results with graphics, tables, and a taxonomy of the findings to ease the data analysis.
3.1.2. Research Questions
The research questions focused on the three main themes of the review: “Industry 4.0”, “Data Science” and “Time Series”. The seven research questions had the following division: three general questions (GQ), two focused questions (FQ), and two statistical questions (SQ), as shown in Table 2 .
The research questions divided into general questions (GQ), focused questions (FQ), and statistical questions (SQ).
The motivation to look for the industrial segments involved with data science was to find out where big quantities of data needed to be analyzed and show new work opportunities (GQ1), the kinds of methods used for this purpose (GQ2), and what were the techniques employed in industry (GQ3). Moreover, understanding how the data are used over time is key to choosing the best technique to use in specific situations (FQ1). Furthermore, the quality of the datasets available is important to analyze how well an algorithm performs related to data gaps and balance (FQ2). Finally, the sources (SQ1) and the number of publications over time (SQ2) help the research process.
3.1.3. Studies Selection
The process of selecting the studies involved five relevant databases in the field of research: ACM, IEEE, Scopus, Springer, and Wiley. A study regarding the research questions helped to define the search string. Moreover, the usage of synonyms and related words allowed the search to get more embracing results. Table 3 shows the organization of the search string considering three themes.
The search string and its three themes: “Industry 4.0”, “Data Science” and “Time Series”.
The refining of the search occurred using six exclusion criteria (EC). First, the filtering process disregarded the papers not written in English (EC1) and not found in journals, conferences, or workshops (EC2). Next, the titles (EC3) and abstracts (EC4) analysis only considered the works in agreement with the research questions. Then, the filtering excluded duplicated papers (EC5). Finally, the last filtering criteria (EC6) was the three-pass approach. This approach uses the analysis of the title, abstract, introduction, title of sections and subsections, mathematical content, and conclusions in the first pass. The second pass is the observation of the images, diagrams, and illustrations. At last, the third pass searches the entire text [ 15 ].
3.2. Execution
After the planning phase, the execution of the planned steps occurred according to the search string’s insertion in the selected databases. Further, the usage of the Zotero tool and an SQL database allowed us to organize the results.
3.2.1. Search String
The databases’ initial search occurred with no filters, using the proposed search string and organizing the data gathered in collections named according to each database. The filtering process occurred all in the “zotero.sqlite” file, which is the SQL database generated by Zotero. The chosen search databases were ACM, IEEE, Scopus, Springer, and Wiley. Figure 2 shows the name of the databases and the number of papers retrieved from the initial search and after applying each exclusion criterion.

The number of papers retrieved from each database: ( a ) from the initial search; ( b ) after exclusion criteria 1 and 2; ( c ) after exclusion criterion 3; ( d ) after exclusion criterion 4; ( e ) after exclusion criterion 5; ( f ) after exclusion criterion 6. Exclusion criterion 4 discarded the remaining papers from Wiley. Scopus had the greatest number of works selected for the corpus, followed by Springer, IEEE, and ACM.
3.2.2. Zotero Tool
A single management tool’s usage aims to ease the collecting process, smoothing the papers’ search and classification. A tool with open access to its database is preferable. At the beginning of this study, tests were conducted with the Mendeley ( https://www.mendeley.com ; accessed on 17 May 2023) and Zotero ( https://www.zotero.org ; accessed on 17 May 2023) reference management tools. Zotero was chosen, due to the authors’ need of accessing the SQL database with no restrictions, since it is an open-access database. Zotero is a reference manager tool that provides a practical way of gathering papers. It organizes the search results thanks to the possibility of using a browser connector that makes the process faster, by allowing the metadata gathering of a set of papers instead of one by one. Moreover, the use of the ZotFile ( http://zotfile.com ; accessed on 17 May 2023) browser plugin in the individual analysis of the selected papers eased the extraction of highlighted sentences [ 16 ].
Table 4 presents the exclusion criteria used in the filtering process with the Zotero tool. In the main screen of Zotero, the field called “Extra” allows the user to insert additional information about the papers. The appending of the pipe symbol (“|”) to the end of the “Extra” field created a new field to be used by SQL queries called “Status”. This new field used along the filtering process assigned a different “Status” to every paper after applying each exclusion criterion. Before the application of the exclusion criteria, all the papers had the “Status” set to empty (“ ”). The usage of SQL sentences in the Zotero database provided a practical way to apply the first two exclusion criteria at the same time, filtering papers not written in English (EC1) and not found in journals, conferences, or workshops (EC2). The papers that met these exclusion criteria had their “Status” set to “ec”, which meant excluded by EC1 or EC2. The remaining papers with an empty status underwent a filtering by the third exclusion criterion, the title analysis (EC3). The discarded papers had their status changed to “ec3”, and the accepted ones to the next step gained the status “ec3_next”. The filtering process continued with the papers with the status “ec3_next”, which had their abstracts analyzed in the fourth exclusion criterion (EC4), and accepted to the next phase (“ec4_next”) or rejected (“ec4”). The next filter eliminated duplicated works, representing the fifth exclusion criterion (EC5), by setting the status to “ec5” or keeping the paper in the next phase, setting the status to “ec5_next”. The last exclusion criterion (EC6) applied the three-pass approach and changed the status of the discarded papers to “ec6” and of the accepted papers to “final”.
Exclusion criteria and status filters used during the corpus selection.
3.2.3. SQL Database
The SQL database allowed an organization of the data extracted during the process. Furthermore, the relational model enabled us to organize the data collected over the development of the systematic review and eased the generation of graphics and the extraction of information. Nine tables and a database view of the Zotero tool composed the model. Figure 3 depicts the relational model, developed with the QuickDBD ( https://app.quickdatabasediagrams.com ; accessed on 17 May 2023) diagram tool.

The diagram shows nine tables created to support the systematic review and a view with the essential data of the Zotero database. The table “Paper” is the central entity and has a one-to-one relationship with the view “Sysmap”. The other main tables are “Industry”, “Question”, “Tool”, and “Method”, besides the auxiliary tables “PaperIndustry”, “PaperQuestion”, “PaperTool”, and “PaperMethod”.
The table “Paper” had four attributes, a unique identifier of the paper (field “idPaper”), a field to store the title of the work (“title”), an identifier code of the work in the Zotero tool (“idZotero”), and a field with the order of the article in the corpus (“idCorpus”). This table had a one-to-one relationship with the view “Sysmap”, which represented the most relevant data used from the Zotero database.
The field “itemID”, of the view “Sysmap”, was the unique identifier of the paper used by Zotero and it was related to the field “idZotero”, of the table “Paper”. The field “typeName” represented the type of publication (book section, journal article, conference paper, manuscript, book, or report). This work only considered journal articles, conference papers, and workshops, which are a variant of conferences. The field “collectionName” was the name of the collection chosen to organize the documents. This work used the names of the search databases and an identifier representing the search round. The field “author” was the name of the first author. The field “year” was the year of publication, “title” was the title of the article, and “abstract” was the abstract of the paper. The field “keywords” organized the keywords of the work separated by a comma. The “language” was the writing language of the paper. The field “extra” was used to set a status for each paper using a pipe character followed by a code. Another attribute called “status” showed the status code. Papers from a conference or workshop used the fields “conferenceName” and “proceedingsTitle” to store the conference or workshop name and the title of the proceedings. Finally, the field “venue” indicated whether the paper was from a journal, conference, or workshop.
The main tables “Industry”, “Question”, “Tool”, and “Methods” related to the table “Paper” in a disjoint many-to-many relationship into one-to-many relationships with auxiliary tables. The table “Industry” had the register of the industrial segments used in the review. “Question” stored the research questions of the paper. The table “Tool” held the software tools used in the selected papers. The table “Method” had the data science methods implemented by the works. The auxiliary tables “PaperIndustry”, “PaperQuestions”, “PaperTool”, and “PaperMethod” had the primary keys of the main tables. The auxiliary table “PaperIndustry” had two extra fields. One of them was responsible for indicating when a specific industrial segment acted in a simulated environment (field “simulated”) and the other one for storing the time period of the data used in the work (field “timePeriod”).
3.3. Analysis
The selected works were carefully investigated looking for data to answer the research questions and classify each work in a specific industry segment. Moreover, the investigation allowed the identification of the data science methods and software tools applied in the studies. Although some papers mentioned the industrial segment, their data actually resulted from a simulation environment. Furthermore, the time duration of data used in the studies, when available, appeared in hours, days, months, or years.
3.4. Reporting
The reporting provided results in different ways. The creation of graphics favored the analysis process providing information in figures with data grouped and organized. In addition, the creation of a taxonomy synthesized a general view of the results. Furthermore, the research questions had the answers discussed which produced research highlights.
This section presents the results of the systematic literature review. Figure 4 shows each step of the process with the number of papers from each database used along the process. Moreover, the figure depicts the number of papers discarded by the exclusion criteria.

The figure shows the five databases used in the study (ACM, IEEE, Scopus, Springer, and Wiley) with the number of papers discarded after each one of the exclusion criteria applied. The number of papers after the initial search, the combination, and the final step is shown in blue. The number of papers discarded by the exclusion criteria is displayed in red.
First, the initial search returned 10,456 papers from the five databases. With the aim of finding the first years that matched the string, the search did not use any filter besides the keywords present in the search string, which meant no cut by years. Then, the two initial exclusion criteria (EC1 and EC2) removed the papers not written in English and the ones not found in journals, conferences, or workshops (22.61%). The third exclusion criterion (EC3) removed the papers which did not pass the title analysis (67.36%). The fourth exclusion criterion (EC4) excluded papers according to the abstract analysis (7.90%). The combination of the remaining papers resulted in 223 works, representing 2.14% of the initial search. The fifth exclusion criterion (EC5) removed 19 duplicated studies. Finally, the sixth exclusion criteria (EC6) excluded 101 papers using the three-pass approach, leaving 103 works in the corpus, which corresponded to 0.99% of the initial search. Table A1 , of Appendix A , shows the selected papers and the corpus identification codes.
The next step consisted of a thorough analysis of the corpus aiming to answer each research question, showing the results with graphics and tables. The rest of this section presents the research questions and respective answers.
4.1. GQ1: Which Industrial Segments Applied Data Science Techniques?
Aiming to standardize the industrial segments present in the corpus, these results considered the classification proposed by the International Labour Organization ( https://www.ilo.org ; accessed on 17 May 2023), a United Nations agency. This classification presents 22 industrial segments, of which 15 were in the corpus. Table 5 shows the industrial segments and each paper’s corpus identification code, besides an extra segment for papers with segments fitted in the general-purpose use segment.
Industrial segments and the identification codes of the papers in the corpus.
The general purpose/others industrial segment represented the major number of papers with 24.04% related to the corpus’s total. After, mechanical and electrical engineering was the second industrial segment with 19.23%, followed by transport equipment manufacturing with 15.38%. The other segments represented less than 10% of the total each. Luo et al. [ 17 ] used two industrial segments: transport equipment manufacturing and Utilities (water, gas, and electricity) . That paper was accounted twice for percentage analysis purposes.
Utilities represented 8.65% of the corpus. basic metal production approached 6.73% of the corpus. Oil and gas represented 5.77% of the corpus. Health services and mining encompassed 3.85% each. Food represented 2.88% of the corpus. Agriculture , postal and telecommunications services , and textiles encompassed 1.92% of the corpus each. Chemical industries , construction , forestry , and media approached 0.96% of the corpus each.
4.2. GQ2: What Are the Data Science Methods Used in the Studies?
A primordial aspect of the successful use of data science is the choice of suitable methods. Table 6 shows the abbreviations of the data science methods used in each paper, ordered by the corpus identification code, and Table A2 of Appendix B contains the names of the methods. Long short-term memory (LSTM) was the most used data science method, appearing in 22 papers, followed by support vector machine (SVM), with 19 appearances, and random forest (RF), which appeared 14 times. Convolutional neural network (CNN) appeared 11 times. Recurrent neural network (RNN) appeared nine times. Multilayer perceptron (MLP) and Principal component analysis (PCA) appeared eight times each. Neural network (NN) appeared seven times. Autoregressive integrated moving average (ARIMA) and logistic regression (LR) appeared six times each. Autoencoder (AE), deep neural network (DNN), local outlier factor (LOF), and synthetic minority oversampling technique (SMOTE) appeared five times each. Convolutional neural network–long short-term memory (CNN-LSTM), density-based spatial clustering of applications with noise (DBSCAN), gated recurrent unit (GRU), K-means (KM), K-nearest neighbor (KNN), one-class SVM (OCSVM), support vector regression (SVR), and XGBoost (XGB) appeared four times each. AdaBoost (AB), bidirectional long short-term memory (BLSTM), backpropagation neural network (BPNN), decision tree (DT), gradient boosting decision tree (GBDT), Gaussian mixture models (GMM), hidden Markov models (HMM), linear regression model (LRM), and isolation forest (iForest) appeared three times each. Agglomerative hierarchical clustering (AHC), attention-based long short-term memory (ALSTM), artificial neural network (ANN), bidirectional gated recurrent unit (BGRU), Bayesian ridge/regularization (BR), classification and regression tree (CART), fault detection and classification convolutional neural network (FDC-CNN), gradient boosting machine (GBM), hierarchical clustering algorithm/analysis (HCA), linear discriminant analysis (LDA), matrix profile (MP), ontology (Ontology), self-organizing maps (SOM), short-term Fourier transform (STFT), visual analytics (VA), and wide-first kernel and deep convolutional neural network (WDCNN) appeared two times each. The other data science methods appeared just one time each over the corpus.
Identification codes of the papers at the corpus and the data science methods used by each one.
Furthermore, to better follow the evolution over the timeline, Figure 5 shows how many times a data science method appeared over the years of publication. Long short-term memory (LSTM) networks were the method that most appeared in the corpus, with 22 occurrences. Then, support vector machine (SVM) had 19 occurrences. Next, the random forest (RF) method appeared 14 times. The years 2019, 2020, and 2021 presented the highest concentration of data science methods.

Data science methods grouped by year. The definition of each method is in Table A2 . Long short-term memory—LSTM was the method with the most occurrences (22), followed by support vector machine—SVM (19), and random forest—RF (14). For better visualization, only methods with more than two occurrences appear in the picture.
4.3. GQ3: What Are the Software Tools Used in the Studies?
Implementing data science methods requires proper software tools such as programming languages, databases, and toolkits. Table 7 shows the abbreviation of the software tools used in each paper of the corpus, and Table A3 of Appendix C , contains the complete names of the tools. Python was the most used software tool, appearing in 20 papers, followed by Keras, in 15 papers, and Tensorflow in 13. MATLAB appeared in eight works and the R language appeared in six. Hadoop and SKLEARN appeared in five studies each. Kafka and MongoDB appeared in four papers each. Spark appeared in three studies. doParallel, fastcluster, foreach, InfluxDB, JavaScript, Jupyter, Knime, MES, MSSQL, PyTorch, rpud, SQL, Storm, and SWRL appeared in two papers each. The remaining software tools appeared just once in the corpus.
Identification codes of the papers in the corpus and the software tools used by each one.
Moreover, Figure 6 shows the software tools grouped by years. The Python programming language was the most used tool, appearing in 20 papers, followed by Keras, which appeared in 15 papers, and Tensorflow which appeared in 13 articles.

Software tools grouped by year. The definition of each tool is in Table A3 . Python was the tool with the most occurrences (20), followed by Keras (15), and Tensorflow (13). For a better visualization, only tools with more than one occurrence appear in the picture.
4.4. FQ1: How Do the Studies Employ Contextual Time Series?
Eleven papers used the concept of context in some way. The works approached ontologies, visual analytics, dynamic Bayesian networks, context-aware cyberphysical systems, convolutional neural networks, recurrent neural networks, and long short-term memory networks.
Wu et al. [ 18 ] used context information to develop an interactive visual analytics system for a petrochemical plant. The system worked in the operation stage, using time-series data from 791 sensors which provided the status of different parts of the factory. Tripathi and Baruah et al. [ 19 ] proposed a method to identify contextual anomalies in a time-series-modifying dynamic Bayesian network (DBN) method to support context information, named contextual DBN. The tests of the new method efficacy occurred in oil well drilling data. Majdani et al. [ 20 ] developed a framework for cyberphysical systems using machine learning and computational intelligence. The framework used context data from 25 sensors of different parts of a gas turbine. Canizo et al. [ 21 ] proposed a convolutional neural network–recurrent neural network (CNN-RNN) architecture to extract features and learn the temporal patterns of context-specific time-series data from 20 sensors installed at a service elevator.
Jiang et al. [ 22 ] used two deep learning methods to predict the remaining useful life (RUL) of bearings. The methods employed context vectors in time-series multiple-channel networks for convolutional neural networks (TSMC-CNN) and extended the method to attention-based long short-term memory networks (TSMC-CNN-ALSTM). Stahl et al. [ 23 ] presented a case of steel sheets’ failure detection using bidirectional recurrent neural networks (RNN) with an attention mechanism. The method used context vectors to represent each state of the process. Ma et al. [ 24 ] proposed a predictive production planning architecture based on big data for a ceramic manufacturing company. The architecture used cube-based models to deal with context-aware historical data using LSTM networks. Yasaei et al. [ 25 ] developed an adaptive context-aware and data-driven model using measures from 62 heterogeneous sensors of a wastewater plant. The model used LSTM networks to detect sensing device anomalies and environmental anomalies.
Abbasi et al. [ 26 ] developed an ontology for aquaponic systems called AquaONT, using the methontology approach to formulate and evaluate the model. The ontology used contextual data from a standard farm to provide information on the optimal operation of IoT devices. Bagozi et al. [ 27 ] proposed an approach focused on resilient cyberphysical production systems (R-CPPS), exploiting big data and the human-in-the-loop perspective. The study used context-aware data stream partitioning, processing data streams collected in the same context, which means the same smart machine and the same type of process to produce the same kind of product. Kim et al. [ 28 ] conducted an experiment to observe the participants’ attentiveness in a repeated workplace hazard, using virtual reality to avoid the risk of injuries. The experiment used a construction task to measure the participants’ biosignals by means of eye-tracking sensors and a wearable device to measure the electrodermal activity, together with contextual features.
4.5. FQ2: What Is the Data Quality over Time Used in the Studies?
Data quality is primordial for all types of industrial segments, including the assembly lines of industries. Knowing the quantity of data over time used in an experiment is fundamental for a better understanding of the data analysis. Out of one hundred and three papers in the corpus, the equivalent of 39.81% (41 papers) mentioned the quantity of data used over a certain period of time. Table 8 presents this information along with the paper identification. Despite mentioning the quantity of data, the units of measure appeared in different forms. The years represent the quantity of data in 14 studies, months in 17 works, days express data in 7 papers, and hours in 3 works.
Quantity of data over time employed in each paper as described by the authors, identified by the ID of the paper in the corpus. The quantity of data appears in years, months, days, and hours.
Another crucial point regarding data quality is the origin of the datasets used in the experiments. Table 9 shows ten papers of the corpus that made their datasets available to public. Three papers used the same repository, although two of them focused on Turbofan engine degradation (Lu et al. [ 29 ] and Wu et al. [ 30 ]), and the other one on bearings (Ding et al. [ 31 ]). Shenfield et al. [ 32 ] and Kancharla et al. [ 33 ], which worked with two datasets, also used bearings but from different repositories. Moreover, Apiletti et al. [ 34 ] used data from hard-drives, Mohsen et al. [ 35 ] worked on a human activity dataset, Zvirblis et al. [ 36 ] used data from conveyor belts, Wahid et al. [ 37 ] worked with a component failure dataset, and Zhan et al. [ 38 ] used data from wind turbines.
The papers whose datasets are available to the public, identified by the ID of the paper in the corpus, the author, and the URL where the data can be downloaded. Ten papers presented the dataset used. Accessed on 17 May 2023.
4.6. SQ1: In Which Databases Are the Studies Published?
The review applied the searches to five databases: ACM, IEEE, Scopus, Springer, and Wiley. However, only four databases had studies selected into the corpus, as shown in Figure 7 . Scopus had the great majority of papers (71.84%), followed by Springer (24.27%), IEEE (2.91%), and ACM (0.97%).

The number of papers in each database by year. Of the five databases used in this work, only four had papers in the corpus. Scopus was the database with the greatest number of studies (74), followed by Springer (25), IEEE (3), and ACM (1). Wiley stayed out of the corpus with no papers selected.
4.7. SQ2: What Is the Number of Publications per Year?
Over the last five years, the publications related to this study increased, doubling from 2018 (10 papers) to 2019 (23 papers). Figure 8 shows the annual progress of the publications, taking into account the date of publishing. The first publication that fit the selection criteria was in 2013 and the last in 2022. Only fourteen works emerged until the end of June 2022 because this was the date when the searches were executed.

The number of publications present in corpus per year. The years with the higher number of works published were 2019, 2020, and 2021 with 23, 22, and 29 papers, respectively. The years refer to the papers’ publication date.
Regarding the types of publications, Figure 9 shows the paper identification code inside a geometric shape. Conference works use a square symbol, journal papers use a circle, and workshop papers use a diamond symbol. Journals had the greatest number of papers (63.11%), followed by conferences (31.07%) and workshops (5.83%).

Types of publication by year, classified as conference, journal, or workshop. The number inside the geometric shapes is the identification code of the paper in the corpus. The years 2019, 2020, and 2021 with 23, 22, and 29 papers, respectively, had the biggest number of publications. Overall, there were 65 publications from journals, 32 from conferences, and 6 presented in workshops.
5. Taxonomy
This section summarizes the answers to the three general research questions, previously presented in Table 2 , using a taxonomic approach to better visualize and understand the results. Figure 10 depicts a taxonomy that hierarchically organizes, classifies, and synthesizes the industrial segments (GQ1), data science methods (GQ2), and software tools (GQ3) found in the corpus with the nodes industry [ 39 ], methods [ 40 , 41 , 42 ], and tools [ 43 , 44 ], respectively. Industrial segments featured sixteen classes, data science methods organized algorithms and techniques into nine branches, and software tools presented applications and libraries organized into nine components.

The taxonomy has three main branches: industry, methods, and tools. Industry organizes the papers into industrial segments, according to the International Labour Organization. Methods depict the data science methods employed in the papers. Tools organize the software tools used in the works.
The industrial segments used in this work originated from the International Labour Organization (ILO) ( https://www.ilo.org/global/industries-and-sectors ; accessed on 17 May 2023), an agency of the United Nations, which classifies industries and sectors into 22 segments. The 103 papers resulted from the systematic review fell into 15 of the 22 segments proposed by the ILO: agriculture , basic metal production , chemical industries , construction , food , forestry , health services , mining , mechanical and electrical engineering , media , oil and gas , postal and telecommunications services , textiles , transport equipment manufacturing , and utilities . These different segments complement those industries with general purpose .
The data science methods found included data structure , machine learning , mathematical , metric , statistical , symbolic , visual analytics , process , and combinatorial search , as shown in the taxonomy and more detailed in Figure 11 . Due to the significant number of methods and their variations, the machine learning branch had a separated taxonomy shown in Figure 12 . The machine learning method long short-term memory (LSTM) networks represented the most used method, with 22 occurrences. Furthermore, there were ten LSTM variations: attention-based long short-term memory (ALSTM), which uses a context vector to infer different attention degrees of distinct data features at specific time points [ 22 ]; bidirectional long short-term memory (BLSTM), which processes data both in chronological order, from start to end, and in the opposite direction, the reverse order [ 21 , 23 ]; deep long short-term memory (DeepLSTM), an LSTM network with stacked layers connected to a dense layer distributed over time [ 45 ]; long short-term memory with nonparametric dynamic thresholding (LSTM-NDT) [ 38 ]; long short-term memory variational autoencoder (LSTM-VAE) [ 38 ]; singular spectrum analysis bidirectional long short-term memory (SSA-BLSTM) [ 46 ]; long short-term memory autoencoder (LSTMAE) [ 47 ]; long short-term memory anomaly detection (LSTM-AD) [ 48 ]. encoder–decoder anomaly detection (EncDec-AD) [ 48 ]; and the ontology-based LSTM neural network (OntoLSTM), which implements semantics concepts using an ontology to learn the representation of a production line, together with an LSTM network for temporal dependencies learning [ 49 ].

The methods branch presents the data science methods split into data structure, machine learning, mathematical, metric, statistical, symbolic, visual analytics, process, and combinatorial search. As a result of the significant number of specialized methods, the machine learning branch is presented in more detail in Figure 12 .

Machine learning branch has the following organization: clustering, decision trees, ensemble, Gaussian processes, linear models, naive Bayes, nearest neighbors, neural networks, reinforcement learning, support vector machines, transfer learning, genetic algorithm, and AutoML.
The second most used data science method was the support vector machine (SVM) method, representing 19 occurrences. Moreover, the method had four variations: fast Fourier transform based support vector machines (FFT-SVM), a version of SVM which uses a fast Fourier transform to extract features [ 32 ]; one-class SVM (OCSVM), an unsupervised version of SVM using a single class to identify similar or different data [ 50 ]; support vector classification (SVC), a variation used for classification tasks [ 34 ]; and the support vector regression (SVR) variation, which implements a linear regression function to the mapped data [ 51 ].
The data science method that was the third-most used was the decision tree method random forest (RF), accumulating 14 occurrences, followed by convolutional neural network (CNN), with 11 occurrences, and recurrent neural network (RNN), with 9 occurrences. Twelve CNN variations stood out as branches: fault detection and classification convolutional neural network (FDC-CNN), designed to detect multivariate sensor signals’ faults over a time axis, extracting fault features; multichannel deep convolutional neural networks (MC-DCNN), whose objective is to deal with multiple sensors that generate data with different lengths; multiple-time-series convolution neural network (MTS-CNN), designed for diagnosis and fault detection of time series, uses a multichannel CNN to extract important data features [ 52 ]; temporal convolutional network (TCN), which works by summarizing signals in time steps, using a maximum and minimum value per step [ 53 ]; residual neural networks (ResNet) [ 54 ]; residual-squeeze Net (RSNet) [ 45 ]; stacked residual dilated convolutional neural network (SRDCNN) [ 32 ]; wide first kernel and deep convolutional neural network (WDCNN) [ 32 , 55 ]; convolutional neural network maximum mean discrepancy (CNN-MMD) [ 33 ]; deep convolutional transfer learning network (DCTLN) [ 55 ]; attention fault detection and classification convolutional neural network (AFDC-CNN) [ 48 ]; and the time-series multiple-channel convolutional neural network (TSMC-CNN), which uses as inputs N-variate time series split into segments, smoothing the extraction of data points [ 22 ]. RNN represented three branches: gated recurrent unit (GRU), long short-term memory (LSTM), and bidirectional recurrent neural network (BRNN).
Regarding the software tools, nine main classes appeared in the taxonomy: anomaly detection , databases , distributed computing , model , prediction , programming languages , toolkits , visualization , and reasoner , as depicted in Figure 13 . The Python language was the most used software tool, with 20 occurrences, followed by Keras (15 occurrences), and Tensorflow (13 toccurrences). Keras is a deep learning framework, and Tensorflow is a machine learning back end [ 32 ], and both are branches of Python in the taxonomy hierarchy.

The tools branch presents the software tools used by the authors, split into anomaly detection, databases, distributed computing, model, prediction, programming languages, toolkits, visualization, and reasoner. All the branches represent one or more ramifications.
Despite covering industrial segments, data science methods, and software tools hierarchically, the taxonomy did not link them horizontally. These relations are in Table 5 , representing industrial segments, Table 6 showing data science methods, and Table 7 providing software tools.
6. Discussion
The results presented in this study originated from a systematic review process focused on Industry 4.0, data science and time series. There was no restriction regarding the publication year to provide a whole spectrum of literature in these aforementioned fields. With this, the review showed industrial segment applications both from real cases and simulated environments, in addition to identifying data science methods, software tools, and the data quality used by the experiments.
Several industrial segments are interested in analyzing data, and more and more data analysis is crucial for companies. This contributes to decision-making in the function of historical data generated by each industry. Moreover, these data analytical processes contribute to the companies’ specific needs since previous experiences are substantial to improve future outcomes.
The industrial segments explored by the literature were classified and grouped according to the International Labour Organization pattern. This provided a better way of visualization in the taxonomy ( Figure 10 ). The general purpose/others industrial segment appeared in 25 papers, being the most present in the corpus. The mechanical and electrical engineering industrial segment was the second most common one (20 papers). The segment includes industries strictly connected to technology, such as semiconductors, computers, and electronics, which explains why it was the most frequent segment in the study, after general purpose/others . Furthermore, this industry usually has controlled environments and employees trained to work with technology, making the collection of data simpler. This favors the execution of studies because those industrial environments are already prepared to produce data combinations toward high-level decision-making.
The majority of studies used real industrial facilities in the experiments (81 papers). However, some papers employed simulated environments (23 works). The work of Luo et al. [ 17 ] appeared twice in the simulated cases due to the presence of two industrial segments in the paper. The usage of real data in most papers provides evidence of the evolution of data science applications in the industry’s production line. This is because sensors and database tools have evolved and become more affordable in the last years. Moreover, the quality of real datasets is a positive point for the training of machine learning algorithms since it can improve the accuracy of predictive models and substantiate future applications that use the same type of data. This is also positive because it reflects real industrial scenarios and potentially provides technology for real-world problems.
Furthermore, the literature presents a wide usage of different technologies, which can hinder the right choice of a suitable method since there is a chance of empirically employing the methods. Aside from the methods, choosing the right tool is another challenge due to different implementations of the same method in distinct tools, e.g., programming languages which present alternative values to initialize the weights of a neural network. A couple of tools rely on specific methods, such as the Keras tool, which deals with deep learning applications employing LSTM and GRU methods. Moreover, it is common to see Keras and Tensorflow tools used together [ 21 , 32 , 54 , 56 , 57 , 58 ]. Both Keras and Tensorflow support the Python language, which is widely used for scientific purposes, appearing in 20 papers of the corpus, as presented in Table 7 . On the other hand, regarding the usage of data combination to create high-level information, the corpus included 11 papers that mentioned contextual data [ 18 , 19 , 20 , 21 , 22 , 23 , 24 , 25 , 26 , 27 , 28 ].
In addition to the aforesaid technologies, neural networks were among the 13 variations of machine learning methods according to the taxonomy. On the other hand, neural networks themselves presented 31 subvariations. With this machine learning method’s improvement, three approaches stood out: attention-based, bidirectional, and autoencoder networks. The attention-based mechanism acts like the human visual attention behavior, using a context vector and focusing on the importance of different features over distinct time steps to improve the prediction accuracy. The studies which focused on this attention-based mechanism explored the usage of, for example, ALSTM and AGRU. Bidirectional models work as two different neural networks walking through a data sequence in both directions to avoid forgotten data. One network goes from the start to the end of the sequence, and the other one comes from the opposite direction. In this respect, studies encompassed the usage of BLSTM, BGRU, and BRNN. An autoencoder is an unsupervised feed-forward neural network commonly used for feature extraction and dimensionality reduction, composed of an encoder and a decoder. The encoder compresses the data to a hidden layer, and the decoder reassembles it to the original input data. In particular, studies used 2-DConvLSTMAE, AEWGAN, AE-GRU, and AE. Hence, these techniques focused on novel combinations and variations of neural networks, which provide versatile methods to exploit problems and questions within the scope of data science in industries.
More specifically, the data quality analysis is critical to ensure a proper functioning of the above-mentioned data science methods. Missing details in the data composition can hamper the paper’s understanding and the reproducibility of the experiment. The quantity of data over time is not enough to supply all the information needed since the frequency can vary during the same period. For example, it is possible to measure the air temperature every hour or every minute of the day. If the measurement occurs every hour, it results in 24 rows. On the other hand, if the measurement occurs every minute, it results in 1440 rows. Therefore, these measurements provide different data granularity, which consequently affects the way results are described. More importantly, these cases require an adequate exposure to methodologies and discussions considering the method’s specificity.
Regarding data structures found in the methods, ontologies provide an advanced way to retrieve information. Classes and relations organize data as a taxonomy but with the possibility to query and reason. The SPARQL is the language used to retrieve information and Hermit, Pallet, and RDFox are examples of reasoners found in the review. An important aspect of ontologies is that they are extendable and reusable [ 26 , 49 , 59 ].
In addition, another crucial piece of information that studies should clearly provide is the percentage of data used for training and testing the model because this strategy of data splitting directly affects the results. Moreover, to guarantee the experiment’s reproducibility, some specific details of the methods are of significant importance, for example, the number of hidden layers of a neural network, or the type of kernel used by a support vector machine, or even the number of interactions used by a random forest. In this sense, there is a need for studies to present more about the data organization and how the data science methods were employed. Papers must include all details of the implementation, such as the architecture and parameters of the machine learning methods and the whole composition of feature vectors. With this, the practitioners will find the methodologies clearer to understand and reproduce in their studies. Hence, this will benefit the community, ensuring potential common situations among different segments to avoid technical and managerial aspects.
7. Conclusions
This article presented a systematic literature review focused on Industry 4.0, data science, and time series. This work investigated the usage of data science methods and software tools in several industrial segments, taking into account the implementation of time series and the data quality employed by the authors. Furthermore, a taxonomy organized the industrial segments, data science methods, and software tools in a hierarchical and synthesized way, which eased the reading of how studies from Industry 4.0 have employed these technologies.
The literature presented several mature methods which covered vast possibilities for industrial analysis. This strengthens both the market and academia because the more companies employ the technologies, the more researchers and practitioners become experts in those methods and tools. In this sense, the industrial investment in these analyses is beneficial because it provides empirical results for the community about applicable use cases in several segments. Moreover, it contributes to the maturity and evolution of the technological methods and tools employed in the process of industrial data analysis.
Even with efforts to reduce biases, this review has limitations as any other systematic review. The search string was applied to five research databases intending to use different academic sources, which potentially decreased the source bias. The search string’s conception used three axes employing respective known keywords and synonyms for each axis, focusing on reducing keywords biases. Moreover, six exclusion criteria filtered the resulting papers, providing the corpus. Accordingly, these exclusion criteria and the remaining filtering process followed Petersen et al.’s [ 14 ] guidelines to reduce process bias.
The taxonomy represents an important contribution to further research since the organization of data science methods and software tools helps the visual search in categories, assisting in discovering research gaps. In addition, the variation of a specific method or tool into a node points to trends in the use of that technology, which is important when choosing what technique to use. Therefore, the taxonomy’s faculty of organizing and classifying the results in hierarchical classes constitutes a relevant achievement of this work. Moreover, the class industry was an attempt to standardize the segments according to the International Labour Organization. Hence, the visualization of the outcomes in the form of a taxonomy increases the possibilities of new research.
Finally, this research study did not focus on how the works dealt with data treatment before applying data science methods to datasets. This situation constitutes an additional limitation, and hence, it is suggested as future work. Moreover, how the software tools are linked to the data science methods is another potential future work. Furthermore, the last topic suggested for future work is to specifically correlate the most used methods and tools with each industrial segment.
Acknowledgments
We are also grateful to Unisinos (University of Vale do Rio dos Sinos— http://www.unisinos.br ; accessed on 17 May 2023) and HT Micron Semiconductors ( http://www.htmicron.com.br ; accessed on 17 May 2023) for embracing this research.
Appendix A. Corpus
Corpus of articles derived from this research.
Appendix B. Methods
Appendix c. tools, funding statement.
The authors wish to acknowledge that this work was supported by CNPq (National Council for Scientific and Technological Development— http://www.cnpq.br ; accessed on 17 May 2023, grant numbers 23/2018 and 306395/2017-7), CAPES (Coordenação de Aperfeiçoamento de Pessoal de Nível Superior-Brasil-Finance Code 001), and FAPERGS (Foundation for the Supporting of Research in the State of Rio Grande do Sul— http://www.fapergs.rs.gov.br ; accessed on 17 May 2023).
Author Contributions
Conceptualization, H.M.A. and R.S.B.; methodology, H.M.A. and R.S.B.; writing—original draft, H.M.A. and R.S.B.; writing—review and editing, R.K., E.F.B., G.C.P. and J.L.V.B.; supervision, R.K., G.C.P. and J.L.V.B. All authors have read and agreed to the published version of the manuscript.
Conflicts of Interest
The authors declare no conflict of interest.
Disclaimer/Publisher’s Note: The statements, opinions and data contained in all publications are solely those of the individual author(s) and contributor(s) and not of MDPI and/or the editor(s). MDPI and/or the editor(s) disclaim responsibility for any injury to people or property resulting from any ideas, methods, instructions or products referred to in the content.
Help | Advanced Search
Computer Science > Databases
Title: wrangling data issues to be wrangled: literature review, taxonomy, and industry case study.
Abstract: Data quality is vital for user experience in products reliant on data. As solutions for data quality problems, researchers have developed various taxonomies for different types of issues. However, although some of the existing taxonomies are near-comprehensive, the over-complexity has limited their actionability in data issue solution development. Hence, recent researchers issued new sets of data issue categories that are more concise for better usability. Although more concise, modern data issue labeling's over-catering to the solution systems may sometimes cause the taxonomy to be not mutually exclusive. Consequently, different categories sometimes overlap in determining the issue types, or the same categories share different definitions across research. This hinders solution development and confounds issue detection. Therefore, based on observations from a literature review and feedback from our industry partner, we propose a comprehensive taxonomy of data quality issues from two distinct dimensions: the attribute dimension represents the intrinsic characteristics and the outcome dimension that indicates the manifestation of the issues. With the categories redefined, we labeled the reported data issues in our industry partner's data warehouse. The labeled issues provide us with a general idea of the distributions of each type of problem and which types of issues require the most effort and care to deal with. Our work aims to address a widely generalizable taxonomy rule in modern data quality issue engineering and helps practitioners and researchers understand their data issues and estimate the efforts required for issue fixing.
Submission history
Access paper:.
- Other Formats

References & Citations
- Google Scholar
- Semantic Scholar
BibTeX formatted citation

Bibliographic and Citation Tools
Code, data and media associated with this article, recommenders and search tools.
- Institution
arXivLabs: experimental projects with community collaborators
arXivLabs is a framework that allows collaborators to develop and share new arXiv features directly on our website.
Both individuals and organizations that work with arXivLabs have embraced and accepted our values of openness, community, excellence, and user data privacy. arXiv is committed to these values and only works with partners that adhere to them.
Have an idea for a project that will add value for arXiv's community? Learn more about arXivLabs .
- Software Development
- Data Science and Business Analytics
- Press Announcements
- Scaler Academy Experience
Statistics for Data Science: A Complete Guide
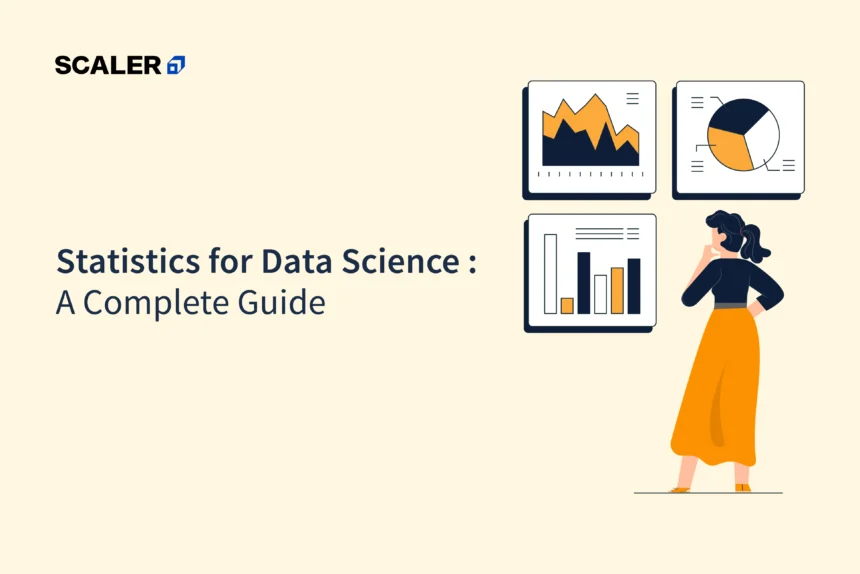
#ezw_tco-2 .ez-toc-title{ font-size: 120%; font-weight: 500; color: #000; } #ezw_tco-2 .ez-toc-widget-container ul.ez-toc-list li.active{ background-color: #ededed; } Contents
Data science is all about finding meaning in data, and statistics is the key to unlocking those insights. Consider statistics as the vocabulary that data scientists employ to comprehend and analyze data. Without it, data is just a jumble of numbers.
A strong background in statistics is essential for anyone hoping to work as a data scientist. It’s the tool that empowers you to turn raw data into actionable intelligence, make informed decisions, and drive real-world impact. In this guide, we’ll break down the key concepts, tools, and applications of statistics in data science, providing you with the knowledge you need to succeed in this exciting field.
Fundamentals of Statistics
Statistics provides the framework for understanding and interpreting data. It enables us to calculate uncertainty, spot trends, and draw conclusions about populations from samples. In data science, a strong grasp of statistical concepts is crucial for making informed decisions, validating findings, and building robust models.
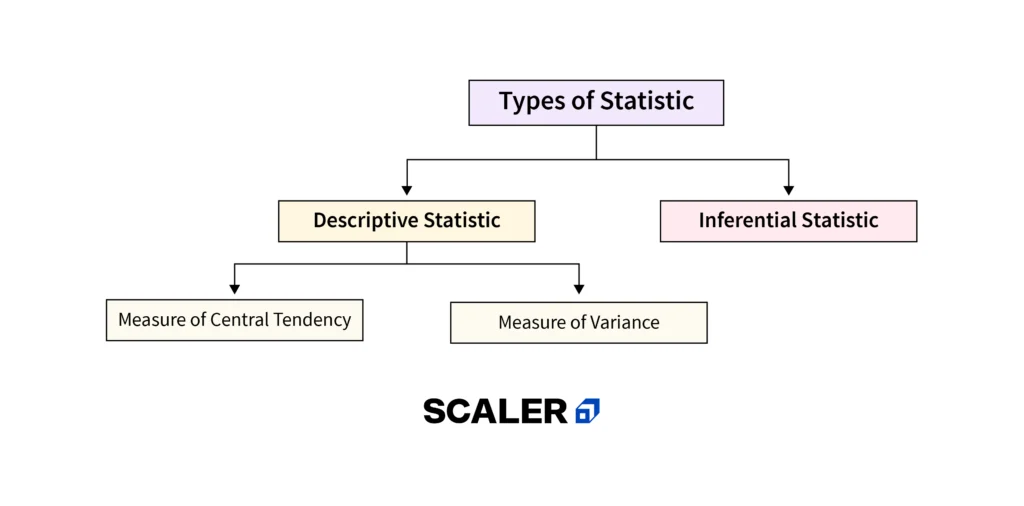
1. Descriptive Statistics
Descriptive statistics help us summarize and describe the key characteristics of a dataset. This includes measures of central tendency like mean (average), median (middle value), and mode (most frequent value), which tell us about the typical or central value of a dataset. We also use measures of variability, such as range (difference between maximum and minimum values), variance , and standard deviation , to understand how spread out the data is. Additionally, data visualization techniques like histograms, bar charts, and scatter plots provide visual representations of data distributions and relationships, making it easier to grasp complex patterns.
2. Inferential Statistics
Inferential statistics, on the other hand, allow us to make generalizations about a population based on a sample. This involves understanding how to select representative samples and how they relate to the overall population. Hypothesis testing is a key tool in inferential statistics, allowing us to evaluate whether a hypothesis about a population is likely to be true based on sample data. We also use confidence intervals to estimate the range of values within which a population parameter is likely to fall. Finally, p-values and significance levels help us determine the statistical significance of results and whether they are likely due to chance.
Why Does Statistics Matter in Data Science?
Statistics is the foundation of the entire field of data science, not just a theoretical subject found in textbooks. It’s the engine that drives data-driven decision-making, allowing you to extract meaningful insights, test hypotheses, and build reliable models.
Applications of Statistics in Data Science Projects:
Statistics is an integral part of data science projects and finds numerous applications at each stage of such projects, from data exploration to model building and validation. Here’s how:
- Data Collection: Designing surveys or experiments to gather representative samples that accurately reflect the target population.
- Data Cleaning: Identifying and handling outliers, missing values, and anomalies using statistical techniques.
- Exploratory Data Analysis (EDA): Summarizing data, visualizing distributions, and identifying relationships between variables using descriptive statistics and graphs.
- Feature engineering: Selecting and transforming variables to improve model performance, often based on statistical insights.
- Model Building: Using statistical models like linear regression, logistic regression, or decision trees to make predictions or classify data.
- Model Evaluation: Assessing the accuracy and reliability of models using statistical metrics like R-squared, precision, recall, and F1 score.
- Hypothesis Testing: Formulating and testing hypotheses about relationships between variables to draw valid conclusions.
- A/B Testing: Comparing the performance of different versions of a product or website to determine which one is more effective, using statistical significance tests.
Examples of Statistical Methods in Real-world Data Analysis:
Here are some examples of how statistical methods are applied in real-world data analysis:
- Healthcare: Statistical methods can be used for analyzing clinical trial data to determine the effectiveness of a new drug or treatment.
- Finance: Building risk models to assess the creditworthiness of borrowers.
- Marketing: Identifying customer segments and predicting their buying behaviour.
- E-commerce: Personalizing product recommendations based on customer preferences.
- Manufacturing: Optimizing production processes to reduce defects and improve efficiency.
By applying statistical methods, data scientists can uncover hidden patterns in data, make accurate predictions, and drive data-driven decision-making across various domains. Whether it’s predicting customer churn, optimizing pricing strategies, or detecting fraudulent activity, statistics play a pivotal role in transforming raw data into actionable insights.
The Fundamental Statistics Concepts for Data Science
Statistics provides the foundation for extracting meaningful insights from data. Understanding these key concepts will empower you to analyze data effectively, build robust models, and make informed decisions in the field of data science.
1. Correlation
Correlation quantifies the relationship between two variables. The correlation coefficient, a value between -1 and 1, indicates the strength and direction of this relationship. A positive correlation means that as one variable increases, so does the other, while a negative correlation means that as one variable increases, the other decreases. Pearson correlation measures linear relationships, while Spearman correlation assesses monotonic relationships.
2. Regression
Regression analysis is a statistical method used to model the relationship between a dependent variable and one or more independent variables. Linear regression models a linear relationship, while multiple regression allows for multiple independent variables. Logistic regression is used when the dependent variable is categorical, such as predicting whether a customer will churn or not.
Bias refers to systematic errors in data collection, analysis, or interpretation that can lead to inaccurate conclusions. Selection, measurement, and confirmation bias are examples of different types of bias. Mitigating bias requires careful data collection and analysis practices, such as random sampling, blinding, and robust statistical methods.
4. Probability
Probability is the study of random events and their likelihood of occurrence. Expected values, variance, and probability distributions are examples of fundamental probability concepts. Conditional probability and Bayes’ theorem allow us to update our beliefs about an event based on new information.
5. Statistical Analysis
Statistical analysis is the process of testing hypotheses and making inferences about data using statistical techniques. Analysis of variance (ANOVA) compares means between multiple groups, while chi-square tests assess the relationship between categorical variables.
6. Normal Distribution
Numerous natural phenomena can be described by the normal distribution, commonly referred to as the bell curve. It is a common probability distribution. It’s characterized by its mean and standard deviation. Z-scores standardize values relative to the mean and standard deviation, allowing us to compare values from different normal distributions.
By mastering these fundamental statistical concepts, you will be able to analyze data, identify patterns, make predictions, and draw meaningful conclusions that will aid in data science decision-making.
Statistics in Relation To Machine Learning
While machine learning frequently takes center stage in data science, statistics is its unsung hero. Statistical concepts underpin the entire machine learning process, from model development and training to evaluation and validation. Understanding this connection is essential for aspiring data scientists and anyone seeking to harness the power of machine learning.
The Role of Statistics in Machine Learning:
Statistics and machine learning are closely intertwined disciplines. Here’s how they relate:
- Model Development: Machine learning models are created and designed using statistical methods such as regression and probability distributions. These models are essentially mathematical representations of relationships within data.
- Training and Optimization: Statistical optimization techniques, such as gradient descent, are used to fine-tune the parameters of machine learning models, enabling them to learn from data and make accurate predictions.
- Model Evaluation: Statistical metrics like accuracy, precision, recall, and F1 score are used to assess the performance of machine learning models. These metrics help data scientists select the best-performing model and identify areas for improvement.
- Hypothesis Testing: Statistical hypothesis testing determines whether the observed results of a machine learning model are statistically significant or simply random.
- Data Preprocessing: Statistical techniques like normalization and standardization are applied to prepare data for machine learning algorithms.
Examples of Statistical Techniques Used in Machine Learning:
Certainly, many statistical techniques form the backbone of machine learning algorithms. Here are a few examples:
- Linear Regression: A statistical model used for predicting a continuous outcome variable based on one or more predictor variables.
- Logistic Regression: A statistical model used for predicting a binary outcome (e.g., yes/no, true/false) based on one or more predictor variables.
- Bayesian Statistics: A probabilistic framework that combines prior knowledge with observed data to make inferences and predictions.
- Hypothesis Testing: A statistical method for evaluating whether a hypothesis about a population is likely to be true based on sample data.
- Cross-Validation: A technique for assessing how well a machine learning model will generalize to new, unseen data.
Statistical Software Used in Data Science
Data scientists have access to a vast collection of statistical software, each with its own set of strengths and capabilities. Whether you’re just starting your data science journey or you’re a seasoned professional, familiarizing yourself with these tools is essential for efficient and effective data analysis.
- Excel: While often overlooked, Excel remains a powerful tool for basic data analysis and visualization. Its user-friendly interface and built-in functions make it accessible for beginners, while its flexibility allows for custom calculations and data manipulation.
- R: It is a statistical programming language specifically designed for data analysis and visualization. It boasts a vast collection of packages and libraries for various statistical techniques, making it a favorite among statisticians and data analysts.
- Python: Known for its versatility and ease of use, Python has become the go-to language for data science. It offers a rich ecosystem of libraries like NumPy (for numerical operations), pandas (for data manipulation and analysis), SciPy (for scientific computing), and stats models (for statistical modeling), making it a powerful tool for data scientists.
- MySQL: It is a popular open-source relational database management system (RDBMS), is widely used to store and manage structured data. Its ability to handle large datasets and perform complex queries makes it essential for data scientists working with relational data.
- SAS: It is a comprehensive statistical analysis software suite used in various industries for tasks like business intelligence, advanced analytics, and predictive modeling. It offers a wide range of statistical procedures, data management tools, and reporting capabilities.
- Jupyter Notebook: A web-based interactive computing environment that allows data scientists to create and share documents that combine code, visualizations, and narrative text. It’s a popular tool for data exploration, prototyping, and collaboration.
The software used is frequently determined by the task at hand, the type of data, and personal preferences. Many data scientists use a combination of these tools to leverage their strengths and tackle diverse challenges.
Practical Applications and Case Studies
Statistics isn’t just theoretical; it’s the engine powering many of the most impactful data science applications across industries. Here are a few examples where statistical methods play a pivotal role:
1. Customer Churn Prediction (Telecommunications):
A telecommunications company was experiencing a high rate of customer churn, losing valuable revenue. Data scientists tackled this problem by building a logistic regression model using historical customer data. This model analyzed various factors, including call patterns, data usage, customer service interactions, and billing history, to predict the likelihood of each customer churning. Armed with these predictions, the company could proactively reach out to high-risk customers with personalized retention offers and tailored services, ultimately reducing churn and improving customer loyalty.
2. Fraud Detection (Finance):
A financial institution was losing millions of dollars annually due to fraudulent transactions. To combat this, data scientists implemented anomaly detection algorithms based on statistical distributions and probability theory. These algorithms continuously monitored transaction data, flagging unusual patterns or outliers that could indicate fraudulent activity. This allowed the institution to investigate and block potentially fraudulent transactions in real time, significantly reducing financial losses.

3. Disease Prediction (Healthcare):
In the realm of healthcare, data scientists are using survival analysis and predictive modeling techniques to predict the risk of diseases like diabetes and heart disease. By analyzing patient data, including demographics, medical history, lifestyle factors, and genetic information, these models can identify high-risk individuals. Armed with this knowledge, healthcare providers can offer personalized preventive care and early interventions, potentially saving lives and improving overall health outcomes.
4. Recommender Systems (e-commerce):
E-commerce giants like Amazon and Netflix rely heavily on recommender systems to drive customer engagement and sales. These systems use collaborative filtering and matrix factorization, statistical techniques that analyze vast amounts of user behavior and product/content data. By understanding user preferences and item characteristics, recommender systems can suggest products or movies that are most likely to resonate with each individual, resulting in personalized shopping experiences and increased revenue.
These case studies demonstrate how statistics enables data scientists to tackle complex problems, uncover hidden patterns, and provide actionable insights that drive business value across industries. By leveraging statistical methods, you can create innovative solutions that have a real-world impact, from improving customer satisfaction to saving lives.
Read More Article:
- Data Science Roadmap
- How to Become a Data Scientist
- Career Transition to Data Science
- Data Science Career Opportunities
- Best Data Science Courses Online
Statistics is the foundation on which data science is built. It provides the essential tools for understanding, analyzing, and interpreting data, allowing us to uncover hidden patterns, make informed decisions, and drive innovation.
From the fundamental concepts of descriptive and inferential statistics to the advanced techniques used in machine learning, statistics empowers data scientists to transform raw data into actionable insights. By mastering the concepts discussed in this guide, you’ll be well-equipped to tackle the challenges of data analysis, build robust models, and make data-driven decisions that have a real-world impact. Remember, statistics is not just a subject to be studied; it’s a powerful tool that can unlock the full potential of data and propel your career in data science to new heights.
If you’re ready to dive deeper into the world of data science, consider exploring Scaler’s comprehensive Data Science Course . They offer a well-structured curriculum, expert instruction, and career support to help you launch your career in this exciting field.
What statistics are needed for data science?
Data science requires a solid foundation in descriptive and inferential statistics, including measures of central tendency and variability, probability distributions, hypothesis testing, regression analysis, and sampling techniques.
What are the branches of statistics?
The two primary branches of statistics are descriptive statistics, which summarize and describe data, and inferential statistics, which draw conclusions about populations from samples. Other branches include Bayesian statistics, non-parametric statistics, and robust statistics.
What is the importance of statistics in data science?
Statistics is important in data science because it provides tools for analyzing and interpreting data, developing reliable models, making informed decisions, and effectively communicating findings. It’s the backbone of the entire data science process, from data collection to model evaluation.
Can I learn statistics for data science online?
Yes, numerous online courses and resources are available to learn statistics for data science. Platforms such as Coursera, edX, and Udemy provide courses ranging from beginner to advanced levels, which are frequently taught by experienced professionals and academics.
How do I apply statistical concepts in data science projects?
Statistical concepts are used throughout the data science workflow. You can use descriptive statistics to summarize data, inferential statistics to test hypotheses, regression analysis to predict outcomes, and various other techniques depending on the specific project and its goals.
Leave a Reply Cancel reply
Your email address will not be published. Required fields are marked *
Save my name, email, and website in this browser for the next time I comment.
Get Free Career Counselling
By continuing, I have read and agree to Scaler’s Terms and Privacy Policy
Get Free Career Counselling ➞
- Systematic Review
- Open access
- Published: 30 May 2024
Patient experiences: a qualitative systematic review of chemotherapy adherence
- Amineh Rashidi 1 ,
- Susma Thapa 1 ,
- Wasana Sandamali Kahawaththa Palliya Guruge 1 &
- Shubhpreet Kaur 1
BMC Cancer volume 24 , Article number: 658 ( 2024 ) Cite this article
Metrics details
Adherence to chemotherapy treatment is recognized as a crucial health concern, especially in managing cancer patients. Chemotherapy presents challenges for patients, as it can lead to potential side effects that may adversely affect their mobility and overall function. Patients may sometimes neglect to communicate these side effects to health professionals, which can impact treatment management and leave their unresolved needs unaddressed. However, there is limited understanding of how patients’ experiences contribute to improving adherence to chemotherapy treatment and the provision of appropriate support. Therefore, gaining insights into patients’ experiences is crucial for enhancing the accompaniment and support provided during chemotherapy.
This review synthesizes qualitative literature on chemotherapy adherence within the context of patients’ experiences. Data were collected from Medline, Web of Science, CINAHL, PsychINFO, Embase, Scopus, and the Cochrane Library, systematically searched from 2006 to 2023. Keywords and MeSH terms were utilized to identify relevant research published in English. Thirteen articles were included in this review. Five key themes were synthesized from the findings, including positive outlook, receiving support, side effects, concerns about efficacy, and unmet information needs. The review underscores the importance for healthcare providers, particularly nurses, to focus on providing comprehensive information about chemotherapy treatment to patients. Adopting recommended strategies may assist patients in clinical practice settings in enhancing adherence to chemotherapy treatment and improving health outcomes for individuals living with cancer.
Peer Review reports
Introduction
Cancer can affect anyone and is recognized as a chronic disease characterized by abnormal cell multiplication in the body [ 1 ]. While cancer is prevalent worldwide, approximately 70% of cancer-related deaths occur in low- to middle-income nations [ 1 ]. Disparities in cancer outcomes are primarily attributed to variations in the accessibility of comprehensive diagnosis and treatment among countries [ 1 , 2 ]. Cancer treatment comes in various forms; however, chemotherapy is the most widely used approach [ 3 ]. Patients undergoing chemotherapy experience both disease-related and treatment-related adverse effects, significantly impacting their quality of life [ 4 ]. Despite these challenges, many cancer patients adhere to treatment in the hope of survival [ 5 ]. However, some studies have shown that concerns about treatment efficacy may hinder treatment adherence [ 6 ]. Adherence is defined as “the extent to which a person’s behaviour aligns with the recommendations of healthcare providers“ [ 7 ]. Additionally, treatment adherence is influenced by the information provided by healthcare professionals following a cancer diagnosis [ 8 ]. Patient experiences suggest that the decision to adhere to treatment is often influenced by personal factors, with family support playing a crucial role [ 8 ]. Furthermore, providing adequate information about chemotherapy, including its benefits and consequences, can help individuals living with cancer gain a better understanding of the advantages associated with adhering to chemotherapy treatment [ 9 ].
Recognizing the importance of adhering to chemotherapy treatment and understanding the impact of individual experiences of chemotherapy adherence would aid in identifying determinants of adherence and non-adherence that are modifiable through effective interventions [ 10 ]. Recently, systematic reviews have focused on experiences and adherence in breast cancer [ 11 ], self-management of chemotherapy in cancer patients [ 12 ], and the influence of medication side effects on adherence [ 13 ]. However, these reviews were narrow in scope, and to date, no review has integrated the findings of qualitative studies designed to explore both positive and negative experiences regarding chemotherapy treatment adherence. This review aims to synthesize the qualitative literature on chemotherapy adherence within the context of patients’ experiences.
This review was conducted in accordance with the Joanna Briggs Institute [ 14 ] guidelines for systemic review involving meta-aggregation. This review was registered in PROSPERO (CRD42021270459).
Search methods
The searches for peer reviewed publications in English from January 2006-September 2023 were conducted by using keywords, medical subject headings (MeSH) terms and Boolean operators ‘AND’ and ‘OR’, which are presented in the table in Appendix 1 . The searches were performed in a systematic manner in core databases such including Embase, Medline, PsycINFO, CINAHL, Web of Science, Cochrane Library, Scopus and the Joanna Briggs Institute (JBI). The search strategy was developed from keywords and medical subject headings (MeSH) terms. Librarian’s support and advice were sought in forming of the search strategies.
Study selection and inclusion criteria
The systematic search was conducted on each database and all articles were exported to Endnote and duplicates records were removed. Then, title and abstract of the full text was screened by two independent reviewers against the inclusion criteria. For this review, populations were patients aged 18 and over with cancer, the phenomenon of interest was experiences on chemotherapy adherence and context was considered as hospitals, communities, rehabilitation centres, outpatient clinics, and residential aged care. All peer-reviewed qualitative study design were also considered for inclusion. Studies included in this review were classified as primary research, published in English since 2006, some intervention implemented to improve adherence to treatment. This review excluded any studies that related to with cancer and mental health condition, animal studies and grey literature.
Quality appraisal and data extraction
The JBI Qualitative Assessment and Review Instrument for qualitative studies was used to assess the methodological quality of the included studies, which was conducted by the primary and second reviewers independently. There was no disagreement between the reviews. The qualitative data on objectives, study population, context, study methods, and the phenomena of interest and findings form the included studies were extracted.
Data synthesis
The meta-aggregation approach was used to combine the results with similar meaning. The primary and secondary reviewers created categories based on the meanings and concept. These categories were supported by direct quotations from participants. The findings were assess based on three levels of evidence, including unequivocal, credible, and unsupported [ 15 , 16 ]. Findings with no quotation were not considered for synthesis in this review. The categories and findings were also discussed by the third and fourth reviewers until a consensus was reached. The review was approved by the Edith Cowan University Human Research Ethics Committee (2021–02896).
Study inclusion
A total of 4145 records were identified through a systematic search. Duplicates ( n = 647) were excluded. Two independent reviewers conducted screening process. The remaining articles ( n = 3498) were examined for title and abstract screening. Then, the full text screening conducted, yielded 13 articles to be included in the final synthesis see Appendix 2 .
Methodological quality of included studies
All included qualitative studies scored between 7 and 9, which is displayed in Appendix 3 . The congruity between the research methodology and the research question or objectives, followed by applying appropriate data collection and data analysis were observed in all included studies. Only one study [ 17 ] indicated the researcher’s statement regarding cultural or theoretical perspectives. Three studies [ 18 , 19 , 20 ] identified the influence of the researcher on the research and vice-versa.
Characteristics of included studies
Most of studies conducted semi-structured and in-depth interviews, one study used narrative stories [ 19 ], one study used focus group discussion [ 21 ], and one study combined focus group and interview [ 22 ] to collect data. All studies conducted outpatient’s clinic, community, or hospital settings [ 17 , 18 , 19 , 20 , 21 , 22 , 23 , 24 , 25 , 26 , 27 , 28 , 29 ]. The study characteristics presented in Appendix 4 .
Review findings
Eighteen findings were extracted and synthesised into five categories: positive outlook, support, side effects, concern about efficacy and unmet information needs.
Positive outlook
Five studies discussed the link between positivity and hope and chemotherapy adherence [ 19 , 20 , 23 , 27 , 28 ]. Five studies commented that feeling positive and avoid the negativity and worry could encourage people to adhere in their mindset chemotherapy: “ I think the main thing for me was just keeping a positive attitude and not worrying, not letting myself worry about it ” [ 20 ]. Participants also considered the positive thoughts as a coping mechanism, that would help them to adhere and complete chemotherapy: “ I’m just real positive on how everything is going. I’m confident in the chemo, and I’m hoping to get out of her soon ” [ 23 ]. Viewing chemotherapy as part of their treatment regimen and having awareness of negative consequences of non-adherence to chemotherapy encouraged them to adhere chemotherapy: “ If I do not take medicine, I do not think I will be able to live ” [ 28 ]. Adhering chemotherapy was described as a survivor tool which helped people to control cancer-related symptoms: “ it is what is going to restore me. If it wasn’t this treatment, maybe I wasn’t here talking to you. So, I have to focus in what he is going to give me, life !” [ 27 ]. Similarly, people accepted the medical facts and prevent their life from worsening; “ without the treatment, it goes the wrong way. It is hard, but I have accepted it from the beginning, yes. This is how it is. I cannot do anything about it. Just have to accept it ” [ 19 ].
Finding from six studies contributed to this category [ 20 , 21 , 23 , 24 , 25 , 29 ]. Providing support from families and friends most important to the people. Receiving support from family members enhanced a sense responsibility towards their families, as they believed to survive for their family even if suffered: “ yes, I just thought that if something comes back again and I say no, then I have to look my family and friends in the eye and say I could have prevented it, perhaps. Now, if something comes back again, I can say I did everything I could. Cancer is bad enough without someone saying: It’s your own fault!!” [ 29 ]. Also, emotional support from family was described as important in helping and meeting their needs, and through facilitation helped people to adhere chemotherapy: “ people who genuinely mean the support that they’re giving […] just the pure joy on my daughter’s face for helping me. she was there day and night for me if I needed it, and that I think is the main thing not to have someone begrudgingly looking after you ” [ 20 ]. Another study discussed the role family, friends and social media as the best source of support during their treatment to adhere and continue “ I have tons of friends on Facebook, believe it or not, and it’s amazing how many people are supportive in that way, you know, just sending get-well wishes. I can’t imagine going through this like 10 years ago whenever stuff like that wasn’t around ” [ 23 ]. Receiving support from social workers was particularly helpful during chemotherapy in encouraging adherence to the chemotherapy: “ the social worker told me that love is courage. That was a huge encouragement, and I began to encourage myself ” [ 25 ].
Side effects
Findings from five studies informed this category [ 17 , 21 , 22 , 25 , 26 ]. Physical side effects were described by some as the most unpleasure experience: “ the side effects were very uncomfortable. I felt pain, fatigue, nausea, and dizziness that limited my daily activities. Sometimes, I was thinking about not keeping to my chemotherapy schedule due to those side effect ” [ 17 ]. The impact of side effects affected peoples’ ability to maintain their independence and self-care: “ I couldn’t walk because I didn’t have the energy, but I wouldn’t have dared to go out because the diarrhoea was so bad. Sometimes I couldn’t even get to the toilet; that’s very embarrassing because you feel like you’re a baby ” [ 26 ]. Some perceived that this resulted in being unable to perform independently: “ I was incredibly weak and then you still have to do things and you can’t manage it ” [ 22 ]. These side effect also decreased their quality of life “ I felt nauseated whenever I smelled food. I simply had no appetite when food was placed in front of me. I lost my sense of taste. Food had no taste anymore ” [ 25 ]. Although, the side effects impacted on patients´ leisure and free-time activities, they continued to undertake treatment: “ I had to give up doing the things I liked the most, such as going for walks or going to the beach. Routines, daily life in general were affected ” [ 21 ].
Concern about efficacy
Findings form four studies informed this category [ 17 , 18 , 24 , 28 ]. Although being concerned about the efficacy of the chemotherapy and whether or not chemotherapy treatment would be successful, one participant who undertook treatment described: “the efficacy is not so great. It is said to expect about 10% improvement, but I assume that it declines over time ” [ 28 ]. People were worried that such treatment could not cure their cancer and that their body suffered more due to the disease: “ I was really worried about my treatment effectiveness, and I will die shortly ” [ 17 ]. There were doubts expressed about remaining the cancer in the body after chemotherapy: “ there’s always sort of hidden worries in there that whilst they’re not actually taking the tumour away, then you’re wondering whether it’s getting bigger or what’s happening to it, whether it’s spreading or whatever, you know ” [ 24 ]. Uncertainty around the outcome of such treatment, or whether recovering from cancer or not was described as: “it makes you feel confused. You don’t know whether you are going to get better or else whether the illness is going to drag along further” [ 18 ].
Unmet information needs
Five studies contributed to this category [ 17 , 21 , 22 , 23 , 26 ]. The need for adequate information to assimilate information and provide more clarity when discussing complex information were described. Providing information from clinicians was described as minimal: “they explain everything to you and show you the statistics, then you’re supposed to take it all on-board. You could probably go a little bit slower with the different kinds of chemo and grappling with these statistics” [ 26 ]. People also used the internet search to gain information about their cancer or treatments, “I’ve done it (consult google), but I stopped right away because there’s so much information and you don’t know whether it’s true or not ” [ 21 ]. The need to receive from their clinicians to obtain clearer information was described as” I look a lot of stuff up online because it is not explained to me by the team here at the hospital ” [ 23 ]. Feeling overwhelmed with the volume of information could inhibit people to gain a better understanding of chemotherapy treatment and its relevant information: “ you don’t absorb everything that’s being said and an awful lot of information is given to you ” [ 22 ]. People stated that the need to know more information about their cancer, as they were never dared to ask from their clinicians: “ I am a low educated person and come from a rural area; I just follow the doctor’s advice for my health, and I do not dare to ask anything” [ 17 ].
The purpose of this review was to explore patient’s experiences about the chemotherapy adherence. After finalizing the searches, thirteen papers were included in this review that met the inclusion criteria.
The findings of the present review suggest that social support is a crucial element in people’s positive experiences of adhering to chemotherapy. Such support can lead to positive outcomes by providing consistent and timely assistance from family members or healthcare professionals, who play vital roles in maintaining chemotherapy adherence [ 30 ]. Consistent with our study, previous research has highlighted the significant role of family members in offering emotional and physical support, which helps individuals cope better with chemotherapy treatment [ 31 , 32 ]. However, while receiving support from family members reinforces individuals’ sense of responsibility in managing their treatment and their family, it also instils a desire to survive cancer and undergo chemotherapy. One study found that assuming self-responsibility empowers patients undergoing chemotherapy, as they feel a sense of control over their therapy and are less dependent on family members or healthcare professionals [ 33 ]. A qualitative systematic review reported that support from family members enables patients to become more proactive and effective in adhering to their treatment plan [ 34 ]. This review highlights the importance of maintaining a positive outlook and rational beliefs as essential components of chemotherapy adherence. Positive thinking helps individuals recognize their role in chemotherapy treatment and cope more effectively with their illness by accepting it as part of their treatment regimen and viewing it as a tool for survival. This finding is supported by previous studies indicating that positivity and positive affirmations play critical roles in helping individuals adapt to their reality and construct attitudes conducive to chemotherapy adherence [ 35 , 36 ]. Similarly, maintaining a positive mindset can foster more favourable thoughts regarding chemotherapy adherence, ultimately enhancing adherence and overall well-being [ 37 ].
This review identified side effects as a significant negative aspect of the chemotherapy experience, with individuals expressing concerns about how these side effects affected their ability to perform personal self-care tasks and maintain independent living in their daily lives. Previous studies have shown that participants with a history of chemotherapy drug side effects were less likely to adhere to their treatment regimen due to worsening symptoms, which increased the burden of medication side effects [ 38 , 39 ]. For instance, cancer patients who experienced minimal side effects from chemotherapy were at least 3.5 times more likely to adhere to their treatment plan compared to those who experienced side effects [ 40 ]. Despite experiencing side effects, patients were generally willing to accept and adhere to their treatment program, although one study in this review indicated that side effects made some patients unable to maintain treatment adherence. Side effects also decreased quality of life and imposed restrictions on lifestyle, as seen in another study where adverse effects limited individuals in fulfilling daily commitments and returning to normal levels of functioning [ 41 ]. Additionally, unmet needs regarding information on patients’ needs and expectations were common. Healthcare professionals were considered the most important source of information, followed by consultation with the internet. Providing information from healthcare professionals, particularly nurses, can support patients effectively and reinforce treatment adherence [ 42 , 43 ]. Chemotherapy patients often preferred to base their decisions on the recommendations of their care providers and required adequate information retention. Related studies have highlighted that unmet needs among cancer patients are known factors associated with chemotherapy adherence, emphasizing the importance of providing precise information and delivering it by healthcare professionals to improve adherence [ 44 , 45 ]. Doubts about the efficacy of chemotherapy treatment, as the disease may remain latent, were considered negative experiences. Despite these doubts, patients continued their treatment, echoing findings from a study where doubts regarding efficacy were identified as a main concern for chemotherapy adherence. Further research is needed to understand how doubts about treatment efficacy can still encourage patients to adhere to chemotherapy treatment.
Strengths and limitation
The strength of this review lies in its comprehensive search strategy across databases to select appropriate articles. Additionally, the use of JBI guidelines provided a comprehensive and rigorous methodological approach in conducting this review. However, the exclusion of non-English studies, quantitative studies, and studies involving adolescents and children may limit the generalizability of the findings. Furthermore, this review focuses solely on chemotherapy treatment and does not encompass other types of cancer treatment.
Conclusion and practical implications
Based on the discussion of the findings, it is evident that maintaining a positive mentality and receiving social support can enhance chemotherapy adherence. Conversely, experiencing treatment side effects, concerns about efficacy, and unmet information needs may lead to lower adherence. These findings present an opportunity for healthcare professionals, particularly nurses, to develop standardized approaches aimed at facilitating chemotherapy treatment adherence, with a focus on providing comprehensive information. By assessing patients’ needs, healthcare professionals can tailor approaches to promote chemotherapy adherence and improve the survival rates of people living with cancer. Raising awareness and providing education about cancer and chemotherapy treatment can enhance patients’ understanding of the disease and its treatment options. Utilizing videos and reading materials in outpatient clinics and pharmacy settings can broaden the reach of educational efforts. Policy makers and healthcare providers can collaborate to develop sustainable patient education models to optimize patient outcomes in the context of cancer care. A deeper understanding of individual processes related to chemotherapy adherence is necessary to plan the implementation of interventions effectively. Further research examining the experiences of both adherent and non-adherent patients is essential to gain a comprehensive understanding of this topic.
Data availability
The datasets used and/or analysed during the current study available from the corresponding author on reasonable request. on our submission system as well.
World Health Organization. Cancer 2021 [ https://www.who.int/news-room/fact-sheets/detail/cancer .
Klapheke A, Yap SA, Pan K, Cress RDDHSDCA. Sociodemographic disparities in chemotherapy treatment and impact on survival among patients with metastatic bladder cancer. Urologic Oncology: Seminars Original Investigations. 2018;36(6):19–308.
Article Google Scholar
Moth EB, Kiely BE, Naganathan V, Martin A, Blinman P. How do oncologists make decisions about chemotherapy for their older patients with cancer? A survey of Australian oncologists. Support Care Cancer. 2018;26(2):451–60.
Article CAS PubMed Google Scholar
Khamboon T, Pakanta I. Intervention for symptom cluster management of fatigue, loss of appetite, and anxiety among patients with lung cancer undergoing chemotherapy. Asia-Pacific J Oncol Nurs. 2021;8(3):267–75.
Garcia ACM, Camargos Junior JB, Sarto KK, Silva Marcelo CAd, Paiva EMC, Nogueira DA, Mills J. Quality of life, self-compassion and mindfulness in cancer patients undergoing chemotherapy: a cross-sectional study. Eur J Oncol Nurs. 2021;51:N.PAG-N.PAG.
Horne R, Chapman SCE, Parham R, Freemantle N, Forbes A, Cooper V. Understanding patients’ adherence-related beliefs about Medicines prescribed for long-term conditions: a Meta-Analytic Review of the necessity-concerns Framework. PLoS ONE. 2013;8(12):e80633.
Article PubMed PubMed Central Google Scholar
WHO. Adherence to long-term therapies: evidence for action. Geneva, Switzerland: World Health Organisation; 2003.
Google Scholar
Warby A, Dhillon HM, Kao S, Vardy JL. A survey of patient and caregiver experience with malignant pleural mesothelioma. Support Care Cancer. 2019;27(12):4675–86.
Article PubMed Google Scholar
Arunachalam SS, Shetty AP, Panniyadi N, Meena C, Kumari J, Rani B, et al. Study on knowledge of chemotherapy’s adverse effects and their self-care ability to manage - the cancer survivors impact. Clin Epidemiol Global Health. 2021;11:100765.
Article CAS Google Scholar
Nizet P, Touchefeu Y, Pecout S, Cauchin E, Beaudouin E, Mayol S, et al. Exploring the factors influencing adherence to oral anticancer drugs in patients with digestive cancer: a qualitative study. Support Care Cancer. 2022;30(3):2591–604.
Clancy C, Lynch J, Oconnor P, Dowling M. Breast cancer patients’ experiences of adherence and persistence to oral endocrine therapy: a qualitative evidence synthesis. Eur J Oncol Nurs. 2020;44.
Magalhães B, Fernandes C, Lima L, Martinez-Galiano JM, Santos C. Cancer patients’ experiences on self-management of chemotherapy treatment-related symptoms: A systematic review and thematic synthesis. Eur J Oncol Nurs. 2020;49.
Peddie N, Agnew S, Crawford M, Dixon D, MacPherson I, Fleming L. The impact of medication side effects on adherence and persistence to hormone therapy in breast cancer survivors: a qualitative systematic review and thematic synthesis. Breast. 2021;58:147–59.
Moher D, Liberati A, Tetzlaff J, Altman DG. Preferred reporting items for systematic reviews and meta-analyses: the PRISMA statement. BMJ: Br Med J. 2009;339(7716):332–6.
Joanna Briggs Institute. The Joanna Briggs Institute critical appraisal tools for use in JBI systematic reviews. Checklist for qualitative research. 2017.
Zachary M, Kylie P, Craig L, Edoardo A, Alan P. Establishing confidence in the output of qualitative research synthesis: the ConQual approach. BMC Med Res Methodol [Internet]. 2014;14(1):108.
Iskandarsyah A, de Klerk C, Suardi DR, Soemitro MP, Sadarjoen SS, Passchier J. Psychosocial and cultural reasons for Delay in seeking help and Nonadherence to treatment in Indonesian women with breast Cancer: a qualitative study. Health Psychol. 2014;33(3):214–21.
Chircop D, Scerri J. The lived experience of patients with Non-hodgkin’s lymphoma undergoing chemotherapy. Eur J Oncol Nurs. 2018;35:117–21.
Kvåle K, Synnes O. Living with life-prolonging chemotherapy—control and meaning‐making in the tension between life and death. Eur J Cancer Care. 2018;27(1):1.
Staneva AA, Beesley VL, Niranjan N, Gibson AF, Rowlands I, Webb PM. I wasn’t gonna let it stop me: exploring women’s experiences of getting through chemotherapy for ovarian cancer. Cancer Nurs. 2019;42(2):E31–8.
Talens A, Guilabert M, Lumbreras B, Aznar MT, López-Pintor E. Medication Experience and Adherence to Oral Chemotherapy: A Qualitative Study of Patients’ and Health Professionals’ Perspectives. Int J Environ Res Public Health. 2021;18(8).
Dumas L, Lidington E, Appadu L, Jupp P, Husson O, Banerjee S, et al. Exploring older women’s attitudes to and experience of treatment for advanced ovarian cancer: a qualitative phenomenological study. Cancers. 2021;13(6):1207.
Albrecht TA, Keim-Malpass J, Boyiadzis M, Rosenzweig M. Psychosocial experiences of young adults diagnosed with acute leukemia during hospitalization for induction chemotherapy treatment. J Hospice Palliat Nurs. 2019;21(2):167–73.
Beaver K, Williamson S, Briggs J. Exploring patient experiences of neo-adjuvant chemotherapy for breast cancer. Eur J Oncol Nurs. 2016;20:77–86.
Chou J-F, Lu YY. Intraperitoneal chemotherapy: the lived experiences of Taiwanese patients with ovarian cancer. Clin J Oncol Nurs. 2019;23(6):E100–6.
Farrell C, Heaven C. Understanding the impact of chemotherapy on dignity for older people and their partners. Eur J Oncol Nurs. 2018;36:82–8.
Wakiuchi J, Silva Marcon S, de Oliveira DC, Aparecida Sales C. Rebuilding subjectivity from the experience of cancer and its treatment. Revista Brasileira De Enfermagem. 2019;72(1):125–33.
Yagasaki K, Komatsu H, Takahashi T. Inner conflict in patients receiving oral anticancer agents: a qualitative study. BMJ Open [Internet]. 2015; 5(4).
Gassmann C, Kolbe N, Brenner A. Experiences and coping strategies of oncology patients undergoing oral chemotherapy: first steps of a grounded theory study. Eur J Oncol Nurs. 2016;23:106–14.
Tang GX, Yan PP, Yan CL, Fu B, Zhu SJ, Zhou LQ, et al. Determinants of suicidal ideation in gynecological cancer patients. Psycho-oncology. 2016;25(1):97–103.
Oven Ustaalioglu B, Acar E, Caliskan M. The predictive factors for perceived social support among cancer patients and caregiver burden of their family caregivers in Turkish population. Int J Psychiatry Clin Pract. 2018;22(1):63–9.
Levkovich I, Cohen M, Karkabi K. The experience of fatigue in breast Cancer patients 1–12 Month Post-chemotherapy: a qualitative study. Behav Med. 2019;45(1):7–18.
Simchowitz B, Shiman L, Spencer J, Brouillard D, Gross A, Connor M, Weingart SN. Perceptions and experiences of patients receiving oral chemotherapy. Clin J Oncol Nurs. 2010;14(4):447–53.
Rashidi A, Kaistha P, Whitehead L, Robinson S. Factors that influence adherence to treatment plans amongst people living with cardiovascular disease: a review of published qualitative research studies. Int J Nurs Stud 2020;110(103727).
Aydogan U, Doganer YC, Komurcu S, Ozturk B, Ozet A, Saglam K. Coping attitudes of cancer patients and their caregivers and quality of life of caregivers. Indian J Palliat Care. 2016;22(2):150–6.
Langford DJ, Morgan S, Cooper B, Paul S, Kober K, Wright F, et al. Association of personality profiles with coping and adjustment to cancer among patients undergoing chemotherapy. Psycho-oncology. 2020;29(6):1060–7.
Jamie MJ, Pensak NA, Sporn NJ, MacDonald JJ, Lennes IT, Safren SA et al. Treatment satisfaction and adherence to oral chemotherapy in patients with Cancer. J Oncol Pract. 2017;13(2).
Tsai Y-F, Huang W-C, Cho S-F, Hsiao H-H, Liu Y-C, Lin S-F, et al. Side effects and medication adherence of tyrosine kinase inhibitors for patients with chronic myeloid leukemia in Taiwan. Medicine. 2018;97(26):415.
D S, M P, G R, S H. Importance of medication adherence and factors affecting it. IP Int J Compr Adv Pharmacolog. 2020;3(2):69–77.
Bekalu YE, Wudu MA, Gashu AW. Adherence to Chemotherapy and Associated factors among patients with Cancer in Amhara Region, Northeastern Ethiopia, 2022. A cross-sectional study. Cancer Control. 2023;30.
Hsu H-C, Liou W-S, Chiang A-J, Tsai S-Y, Jeang S-R, Wu S-L, et al. Longitudinal perceptions of the side effects of chemotherapy in patients with gynecological cancer. Support Care Cancer. 2017;25(11):3457–64.
Gow K, Rashidi A, Whithead L. Factors influencing medication adherence among adults living with diabetes and comorbidities: a qualitative systematic review. Curr Diab Rep. 2023:1–7.
Rashidi A, Whitehead L, Kaistha P. Nurses’ perceptions of factors influencing treatment engagement among patients with cardiovascular diseases: a systematic review. BMC Nurs. 2021;20(1):251.
Zebrack BJ, Block R, Hayes-Lattin B, Embry L, Aguilar C, Meeske KA, et al. Psychosocial service use and unmet need among recently diagnosed adolescent and young adult cancer patients. Cancer. 2013;119(1):201–14.
Timmers L, Boons CCLM, van den Verbrugghe M, Van Hecke A, Hugtenburg JG. Supporting adherence to oral anticancer agents: clinical practice and clues to improve care provided by physicians, nurse practitioners, nurses and pharmacists. BMC Cancer. 2017;17(1).
Download references
Acknowledgements
Not applicable.
Author information
Authors and affiliations.
School of Nursing and Midwifery, Edith Cowan University, 270 Joondalup Drive, Joondalup, Perth, WA, 6027, Australia
Amineh Rashidi, Susma Thapa, Wasana Sandamali Kahawaththa Palliya Guruge & Shubhpreet Kaur
You can also search for this author in PubMed Google Scholar
Contributions
First author (AR) and second author (ST) conceived the review and the second author oversight for all stages of the review provided by the second author. All authors (AR), (ST), (WG) and (SK) undertook the literature search. Data extraction, screening the included papers and quality appraisal were undertaken by all authors (AR), (ST), (WG) and (SK). First and second authors (AR) and (ST) analysed the data and wrote the first draft of the manuscript and revised the manuscript and all authors (AR), (ST), (WG) and (SK) approved the final version of the manuscript.
Corresponding author
Correspondence to Amineh Rashidi .
Ethics declarations
Ethics approval and consent to participate.
The review was approved by the Edith Cowan University Human Research Ethics Committee (2021–02896). A proposal for the systematic review was assessed by the Edith Cowan University Human Research Ethics Committee and deemed not appropriate for full ethical review. However, a Data Management Plan (2021-02896-RASHIDI) was approved and monitored as part of this procedure. Raw data was extracted from the published manuscripts and authors could not identify individual participants during or after this process.
Consent for publication
Competing interests.
The authors declare no competing interests.
Additional information
Publisher’s note.
Springer Nature remains neutral with regard to jurisdictional claims in published maps and institutional affiliations.
Electronic supplementary material
Below is the link to the electronic supplementary material.
Supplementary Material 1
Supplementary material 2, supplementary material 3, supplementary material 4, supplementary material 5, rights and permissions.
Open Access This article is licensed under a Creative Commons Attribution 4.0 International License, which permits use, sharing, adaptation, distribution and reproduction in any medium or format, as long as you give appropriate credit to the original author(s) and the source, provide a link to the Creative Commons licence, and indicate if changes were made. The images or other third party material in this article are included in the article’s Creative Commons licence, unless indicated otherwise in a credit line to the material. If material is not included in the article’s Creative Commons licence and your intended use is not permitted by statutory regulation or exceeds the permitted use, you will need to obtain permission directly from the copyright holder. To view a copy of this licence, visit http://creativecommons.org/licenses/by/4.0/ . The Creative Commons Public Domain Dedication waiver ( http://creativecommons.org/publicdomain/zero/1.0/ ) applies to the data made available in this article, unless otherwise stated in a credit line to the data.
Reprints and permissions
About this article
Cite this article.
Rashidi, A., Thapa, S., Kahawaththa Palliya Guruge, W. et al. Patient experiences: a qualitative systematic review of chemotherapy adherence. BMC Cancer 24 , 658 (2024). https://doi.org/10.1186/s12885-024-12353-z
Download citation
Received : 17 November 2023
Accepted : 07 May 2024
Published : 30 May 2024
DOI : https://doi.org/10.1186/s12885-024-12353-z
Share this article
Anyone you share the following link with will be able to read this content:
Sorry, a shareable link is not currently available for this article.
Provided by the Springer Nature SharedIt content-sharing initiative
- Chemotherapy treatment
- Medication adherence
- Qualitative research
- Patients experiences
ISSN: 1471-2407
- Submission enquiries: [email protected]
- General enquiries: [email protected]
- Scoping Review
- Open access
- Published: 24 May 2024
Impact of climate change on the global circulation of West Nile virus and adaptation responses: a scoping review
- Hao-Ran Wang 1 , 2 ,
- Tao Liu 1 , 2 ,
- Xiang Gao 1 , 2 ,
- Hong-Bin Wang 1 , 2 &
- Jian-Hua Xiao ORCID: orcid.org/0000-0003-1109-9133 1 , 2
Infectious Diseases of Poverty volume 13 , Article number: 38 ( 2024 ) Cite this article
277 Accesses
1 Altmetric
Metrics details
West Nile virus (WNV), the most widely distributed flavivirus causing encephalitis globally, is a vector-borne pathogen of global importance. The changing climate is poised to reshape the landscape of various infectious diseases, particularly vector-borne ones like WNV. Understanding the anticipated geographical and range shifts in disease transmission due to climate change, alongside effective adaptation strategies, is critical for mitigating future public health impacts. This scoping review aims to consolidate evidence on the impact of climate change on WNV and to identify a spectrum of applicable adaptation strategies.
We systematically analyzed research articles from PubMed, Web of Science, Scopus, and EBSCOhost. Our criteria included English-language research articles published between 2007 and 2023, focusing on the impacts of climate change on WNV and related adaptation strategies. We extracted data concerning study objectives, populations, geographical focus, and specific findings. Literature was categorized into two primary themes: 1) climate-WNV associations, and 2) climate change impacts on WNV transmission, providing a clear understanding. Out of 2168 articles reviewed, 120 met our criteria. Most evidence originated from North America (59.2%) and Europe (28.3%), with a primary focus on human cases (31.7%). Studies on climate-WNV correlations ( n = 83) highlighted temperature (67.5%) as a pivotal climate factor. In the analysis of climate change impacts on WNV ( n = 37), most evidence suggested that climate change may affect the transmission and distribution of WNV, with the extent of the impact depending on local and regional conditions. Although few studies directly addressed the implementation of adaptation strategies for climate-induced disease transmission, the proposed strategies ( n = 49) fell into six categories: 1) surveillance and monitoring (38.8%), 2) predictive modeling (18.4%), 3) cross-disciplinary collaboration (16.3%), 4) environmental management (12.2%), 5) public education (8.2%), and 6) health system readiness (6.1%). Additionally, we developed an accessible online platform to summarize the evidence on climate change impacts on WNV transmission ( https://2xzl2o-neaop.shinyapps.io/WNVScopingReview/ ).
Conclusions
This review reveals that climate change may affect the transmission and distribution of WNV, but the literature reflects only a small share of the global WNV dynamics. There is an urgent need for adaptive responses to anticipate and respond to the climate-driven spread of WNV. Nevertheless, studies focusing on these adaptation responses are sparse compared to those examining the impacts of climate change. Further research on the impacts of climate change and adaptation strategies for vector-borne diseases, along with more comprehensive evidence synthesis, is needed to inform effective policy responses tailored to local contexts.
West Nile virus (WNV), the most widely distributed flavivirus globally, is a significant mosquito-borne virus [ 1 ]. It was first isolated in 1937 from the blood of a febrile woman in the West Nile region of Uganda. The earliest reported outbreaks occurred in the 1950s near Haifa, Israel [ 2 ]. Since the 1950s, WNV outbreaks have primarily occurred in Israel and various African countries [ 3 , 4 ]. However, the epidemiology of WNV appears to have shifted since the 1990s due to the globalization of human trade and travel [ 1 ]. WNV was first detected in New York City in 1999 and subsequently spread rapidly throughout the entire Western Hemisphere, including the United States (US), Canada, and Argentina [ 5 , 6 , 7 ]. Concurrently, epidemic activity increased in Europe, the Middle East, and Russia [ 3 , 4 , 8 ]. In 2018, Europe experienced an unprecedented WNV epidemic, with human cases exceeding 1900, seven times higher than in previous seasons [ 9 ]. In 2020, locally transmitted human cases of WNV were reported for the first time in the Netherlands and Germany [ 10 , 11 ]. Evidence suggests interactive WNV cycles on all continents except Antarctica [ 1 ].
The establishment of ongoing WNV transmission relies on the interactions among the virus, vectors, hosts, and environmental factors [ 12 ]. WNV can infect a wide range of vertebrate species, including most mammals, birds, and some reptiles and amphibians [ 13 , 14 ]. Birds, serving as the primary amplifying hosts, play a crucial role in WNV proliferation. While humans and horses are susceptible to WNV, they are considered dead-end hosts [ 15 ]. In humans, WNV often results in asymptomatic or mild illness, but approximately 1 in 150 cases progress to neuroinvasive disease, potentially leading to encephalitis or death [ 16 ]. The primary vectors for WNV transmission are mosquitoes, particularly those belonging to the Culex genus. Mosquito bites are responsible for the vast majority of human WNV infections, although the virus can also spread through blood transfusions, organ transplantations, and potentially breastfeeding [ 17 ]. Given that WNV is transmitted by mosquitoes, its distribution depends on environmental conditions and is susceptible to the impacts of climate change [ 18 ]. For example, higher temperatures can accelerate viral replication, shorten the extrinsic incubation period in mosquitoes, promote vector abundance, enhance transmission efficiency, expand the suitability of vector habitats, and increase the probability of avian migration across regions [ 19 , 20 ]. Additionally, precipitation patterns have a significant impact on mosquito breeding and abundance, thus affecting the spread and geographical distribution of WNV [ 18 ].
The current body of evidence strongly indicates that climate change directly impacts the spread and proliferation of vector-borne illnesses, including WNV [ 21 ]. Numerous studies have demonstrated that areas vulnerable to WNV transmission could expand or shift due to climate elements. This encompasses projecting future global climate change scenarios, examining how vector species respond to environmental shifts in laboratory settings, and conducting field research in regions where outbreaks occur. There is some evidence of WNV emerging or re-emerging in high-latitude regions and at the edges of current endemic zones [ 22 , 23 , 24 , 25 , 26 ]. For example, in North America, the suitable range for WNV is projected to extend northward and to higher altitudes by 2050 and 2080, potentially leading to new infections in both native and non-native species [ 22 ]. In Europe, increased WNV cases and new outbreak locations are predicted under future climate scenarios, especially at the margin of current transmission areas [ 23 ]. In South America, high risk areas for WNV might shift between 2046–2065 and 2081–2100, with more pronounced changes under high greenhouse gas emission scenarios, potentially altering the current WNV distributions in some countries (e.g., parts of Bolivia, Paraguay, and Brazil) [ 24 ]. Moreover, existing surveillance data support the overall trend of heightened WNV risk due to climate change. For instance, in the Powder River Basin of Montana and Wyoming, US, the WNV mortality rate in the wild bird population was significantly higher in 2003 (the sixth most sweltering summer historically) than in 2004 and 2005 [ 25 ]. In Germany, the extreme heat in the summer of 2018 (the second most sweltering and desiccated summer historically) theoretically played a pivotal role in reducing the average extrinsic incubation period in mosquitoes, resulting in rapid viral amplification and increased transmission risks to vertebrate hosts [ 26 ]. However, the impact of climate change on WNV distribution may vary geographically, and some areas may see a decrease in cases. For example, while Keyel et al. predicted a general increase in WNV cases in 2021, a subsequent study indicated that future cases may decrease in areas outside the boundaries of the original study area in New York [ 27 , 28 ].
While efforts to mitigate climate change are essential to reduce CO 2 emissions and lessen potential future impacts, there is an increasing need to focus on adaptation strategies as well. These include various short-term measures at different levels to address the immediate effects of climate change [ 29 ]. Adaptation approaches aim to enhance resilience in health systems, preparing them to manage and minimize the health consequences of climate change [ 29 ]. Given the commitments countries have made to the Paris Agreement and Sustainable Development Goals, along with the growing global evidence base for climate change's impact on disease spread, nations have begun developing and implementing policy responses as components of national climate adaptation plans [ 30 ]. Insights into the expected magnitude of climate change impacts on WNV and associated adaptive responses can help inform best practices to mitigate public health impacts from the climate-induced spread of disease.
Contemporary prioritization in Canada of investigative pursuits on emerging human and animal diseases under climate change scenarios indicated that WNV is a disease requiring primary attention [ 31 , 32 ]. Since the Intergovernmental Panel on Climate Change (IPCC) Fourth Assessment Report in 2007, the health impacts of climate change have garnered significant research focus [ 33 ]. This attention has increased further following the Fifth Assessment Report in 2014 and the 2015 Lancet Commission on Climate Change and Health, leading to a growth in the number of related publications [ 34 , 35 ]. In addition to highlighting the impacts of climate change, these articles also emphasize to some degree specific interventions or policy responses within defined countries and regions. To our knowledge, a comprehensive review of the global impacts and adaptation responses related to climate change and WNV has not been conducted. Such a review is necessary to consolidate existing evidence, explore how climate change influences the spread of WNV, and identify the most effective strategies for developing adaptation policies.
In summary, this scoping review aims to address two core questions:
What types of evidence exist regarding the impact of climate change on the global transmission of WNV?
What adaptation measures have been proposed or implemented in response to climate change?
Our primary focus is to elucidate the climatic drivers of WNV to better inform these strategies. This approach is intended to serve as a foundation for future research that may delve into comprehensive public health policies and adaptation measures.
Protocol and registration
We used a scoping review methodology to select studies for inclusion in this synthesis. Our review followed an established protocol, guided by the PRISMA Scoping Review Extension (PRISMA-SCR) and published scoping review methodology [ 36 , 37 , 38 ]. It was registered with the OSF Registries ( https://osf.io/9j2as ) on December 25, 2023, to ensure transparency [ 39 , 40 ].
Search strategy
We conducted systematic searches across four databases—PubMed (MEDLINE), Web of Science, Scopus, and EBSCOhost—to identify relevant peer-reviewed publications on climate change and WNV between January 2007 and December 2023 without imposing language restrictions. Our literature searches employed terminology related to climate change and the diseases of interest. Terms for climate change were taken from the search strategy used in Sweileh’s (2020) bibliometric analysis of climate change and health publications: “climat* Change” OR “global warming” OR “changing climate” OR “climate variability” OR “greenhouse gas” OR “rising temperature” OR “extreme weather” OR “greenhouse effect” [ 41 ]. Disease-specific terms included were: “West Nile virus” OR “WNV host” OR “WNV vector”. Full search strategies for each database are provided in supplementary materials (Additional file 1 ).
This search strategy was designed to comprehensively capture all original studies examining the associations between meteorological, climatological, ecological, or environmental change factors and the transmission dynamics, outbreaks, risks, or adaptations of WNV. By conducting systematic searches across key databases, supplemented by targeted topic strings, our strategy ensures reproducibility and effectively summarizes contemporary evidence illuminating the connections between WNV and climate amidst escalating changes.
Eligibility criteria
The criteria for including and excluding articles in our analysis are outlined in Table 1 . We examined literature since 2007 to capture research conducted after the IPCC Fourth Assessment Report’s release, representing a milestone driving expanded climate-health investigations [ 33 , 41 ]. Focusing on this period enhances relevance and rigor by concentrating on studies consciously examining climate-related impacts during intensifying change. Further augmenting stringency, we concentrated solely on original quantitative and qualitative investigations published in English-language peer-reviewed academic journals. Together these boundaries help systematically extract recent high-quality evidence elucidating shifting WNV transmission dynamics amidst climate change while delineating adaptations instituted since an authoritative global assessment.
Screening and study selection
We used the systematic review software NoteExpress 3.8.0.9455 (Beijing Aegean Sea Software Company, Beijing, China) to implement standardized screening and selection procedures. Two independent reviewers carried out an initial screening of titles and abstracts to filter articles that met basic eligibility criteria, with a third reviewer resolving any discrepancies. Subsequently, these two reviewers conducted full-text evaluations of the retained articles to ensure compliance with all inclusion criteria as outlined in the predefined protocol. Any disputes again triggered third-reviewer arbitration to achieve consensus.
Data extraction
We used a predefined covidence data extraction framework to systematically characterize key article features including 1) identifiers like title, author(s), and year; 2) specific objectives, study populations, WNV research priority (primary/secondary), and geographic focus; and 3) findings of the paper, such as nature of the evidence for climate change impacts on disease emergence, transmission or spread and/or policy responses, interventions or adaptations [ 42 , 43 ].
We categorized the geographic focus of articles into six regions: North America, South America, Europe, Africa, Asia, and Oceania, with multi-regional studies classified as global. The study populations analyzed included humans, mosquitoes, birds, and horses. Investigations encompassing more than one species were labeled as ‘multiple species’, and studies that did not specify their focus were marked as ‘unspecified’. The central disease under investigation in all articles was WNV. Articles primarily focused on WNV dynamics were categorized under ‘primary’ interest level, while those analyzing WNV in conjunction with other vector-borne diseases were deemed of ‘secondary’ interest.
The findings of the paper regarding evidence or arguments presented on the impacts of climate change (including extreme weather, rising temperatures, and/or climate variability) on WNV emergence, transmission, or spread were recorded. To clearly understand the impacts of climate change on WNV, articles were grouped into two main categories: 1) climate-WNV associations, and 2) climate change impacts on WNV, as categorized by Kulkarni et al. in their study of the impact of climate change on global malaria and dengue fever [ 38 ]. The articles defined as climate-WNV associations mainly refer to the impacts of climatic and seasonal factors (e.g. temperature, precipitation, and seasonal variations) on WNV transmission and spread within a certain time frame. Articles defined as climate change impacts on WNV are further categorized into two types: those with clear evidence of climate change or climate anomalies during the study period affecting WNV transmission and spread, and those with projections of future WNV transmission and spread under climate change scenarios.
The findings of the paper pertaining to evidence for policy responses, interventions, or adaptive measures addressing the impacts of climate change on disease emergence, transmission, or spread were documented. Specifically, the nature of the evidence or arguments presented regarding policy measures, interventions, and/or adaptations to mitigate the effects of climate change on the emergence, propagation, or spread of WNV were recorded. The United Nations Environmental Program (UNEP) handbook on methodologies for assessing climate change impacts and adaptation strategies outlines a typology of adaptation measures to safeguard human health from climate change [ 44 ]. These encompassed five categories of measures: (1) surveillance and monitoring, (2) infrastructure development, (3) public education, (4) technology or engineering strategies, and (5) medical interventions. The content of the article on adaptation strategies is categorized according to the UNEP manual and in the context of the WNV case.
Quality assessment of included literature
The quality of the included articles was assessed using the Joanna Briggs Institute Prevalence Critical Appraisal Tool [ 45 ]. All selected studies were scored using the 10 quality control items suggested by the tool. A score of one was awarded for each item fulfilled while a zero score was awarded for each unmet item. Score aggregates were generated and studies were classified as either low (0–3), moderate (4–6), or high (7–10) quality.
Web development
Most reviews traditionally present evidence in a tabular format, which consumes a considerable portion of the article’s space and often hinders easy navigation through the key information [ 36 , 38 ]. In this study, we used the R Shiny interactive web application framework to develop an online-accessible website that presents evidence on the impact of climate change on WNV transmission and dissemination [ 46 ]. This website allows visitors to query and download information on the effects of climate change on WNV transmission and spread at any time and from any location. This method provides a novel way to access and understand the synthesized evidence in a clearer and more convenient manner.
Characteristics of included studies
Initially, 2168 articles were retrieved from four databases: Web of Science, PubMed, Scopus, and EBSCOhost. After removing 896 duplicates, 1272 articles remained (Fig. 1 ). Following title and abstract screening, 1068 articles were excluded as irrelevant, leaving 204 for full-text review. This resulted in 105 articles meeting inclusion criteria, focusing on the association between climate/weather and WNV or its transmission due to climate changes.
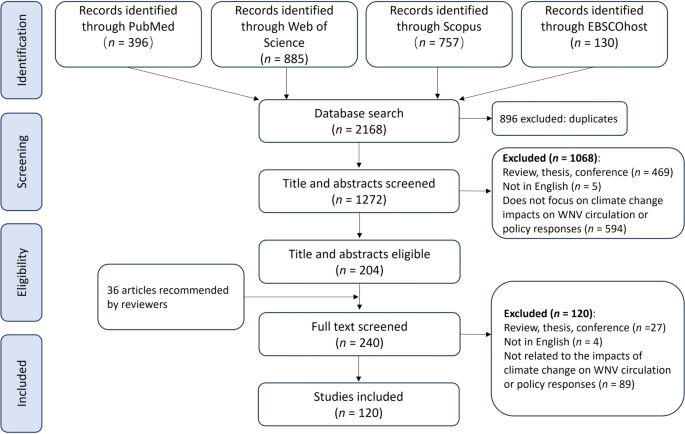
Flowchart diagram illustrating the article search and selection process
To comprehensively cover literature on the impact of climate change on WNV, we used specific search terms based on key themes from prior studies [ 37 , 38 ]. Although these terms helped in retrieving targeted and relevant literature, their specificity might have restricted the scope, possibly excluding significant studies that broader terms could have included. Hence, the reviewers recommended 36 relevant articles, which we screened and retained 15 articles according to the inclusion criteria.
The comprehensive review included 120 studies divided into two categories: 83 studies focused on the associations between climate/weather and WNV, and 37 studies examined the impacts of climate change on WNV transmission. All the reviewed evidence and related adaptation responses are available for exploration and download through a dedicated Shiny web application ( https://2xzl2o-neaop.shinyapps.io/WNVScopingReview/ ).
Publication year
The number of published studies on climate change and WNV has increased over time, with a sharp rise observed after 2013 (Fig. 2 ). Regarding the temporal distribution of relevant literature, two key observations can be made.
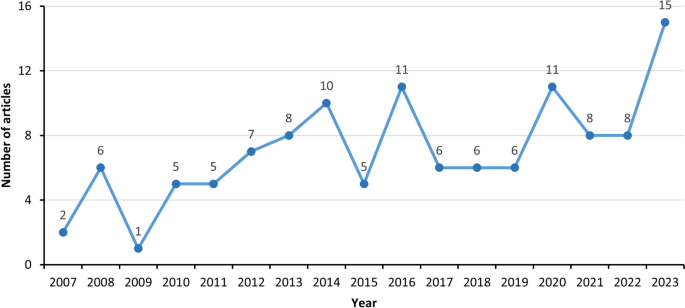
Distribution of the publication years in all articles included from 2007 to 2023
First, only 26 articles were published between 2007 and 2012, of which 21 articles focused on the associations between climate/weather [ 47 , 48 , 49 , 50 , 51 , 52 , 53 , 54 , 55 , 56 , 57 , 58 , 59 , 60 , 61 , 62 , 63 , 64 , 65 , 66 , 67 ] and 5 articles examined the impacts of climate change on WNV [ 25 , 68 , 69 , 70 , 71 ]. The earliest study on climate/weather factors and WNV, published in 2007, analyzed the association between precipitation and human WNV incidence in the US during 2002–2004. The first article on the impacts of climate change on WNV, published in 2007, investigated WNV prevalence in wild Greater Sage-Grouse populations across Montana and Wyoming during 2003–2005. The relatively small number of studies before 2013 indicates that relevant research was still in its infancy stage.
Second, most studies on this topic ( n = 94) emerged after 2013, corresponding to the release of the IPCC Fifth Assessment Report in 2014 and the Lancet Commission on Climate and Health in 2015 [ 34 , 35 ]. As authoritative reviews synthesizing the state-of-the-art science on anthropogenic climate change and its health consequences, these landmark reports have stimulated new research assessing climate impacts on infectious diseases like WNV.
Study location
The geographical distribution of study locations examined in the articles is shown in Fig. 3 . The most frequently studied region was North America, representing 59.2% of articles ( n = 71). Within North America, 53 articles focused on the US [ 25 , 27 , 28 , 47 , 48 , 49 , 50 , 51 , 52 , 53 , 54 , 55 , 56 , 57 , 58 , 59 , 60 , 61 , 62 , 68 , 72 , 73 , 74 , 75 , 76 , 77 , 78 , 79 , 80 , 81 , 82 , 83 , 84 , 85 , 86 , 87 , 88 , 89 , 90 , 91 , 92 , 93 , 94 , 95 , 96 , 97 , 98 , 99 , 100 , 101 , 102 , 103 , 104 ], 17 on Canada [ 31 , 32 , 63 , 64 , 69 , 105 , 106 , 107 , 108 , 109 , 110 , 111 , 112 , 113 , 114 , 115 , 116 ], and 1 covered the entire continent [ 22 ]. Europe was the second most studied region, accounting for 28.3% of articles ( n = 34) [ 23 , 26 , 65 , 70 , 71 , 117 , 118 , 119 , 120 , 121 , 122 , 123 , 124 , 125 , 126 , 127 , 128 , 129 , 130 , 131 , 132 , 133 , 134 , 135 , 136 , 137 , 138 , 139 , 140 , 141 , 142 , 143 , 144 , 145 ]. The other world regions assessed were Asia ( n = 4; 3.3%) [ 66 , 146 , 147 , 148 ], Africa ( n = 4; 3.3%) [ 67 , 149 , 150 , 151 ], South America ( n = 2; 1.7%) [ 24 , 152 ], and Oceania ( n = 1; 0.8%) [ 153 ]. Only 4 articles (3.3%) [ 51 , 154 , 155 , 156 ] included multiple global regions and were classified as the “global” studies.
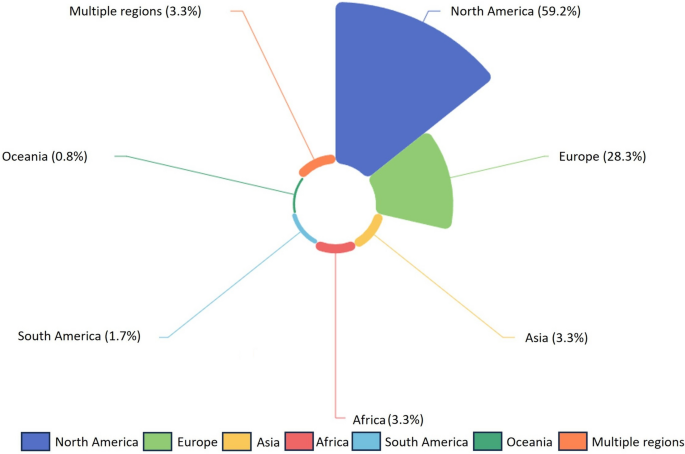
Geographical distribution of the study areas in all articles included from 2007 to 2023
Research on WNV has focused on two regions, North America and Europe, which corresponds to the high incidence and disease burden from epidemics reported in these two regions over the past two decades. In the US, between 2007 and 2022, there were 32,600 confirmed or suspected human WNV cases reported to the Centers for Disease Control and Prevention, particularly concentrated in California, Colorado, and Texas [ 157 ]. WNV remains the leading cause of mosquito-borne disease in the US, accounting for 83.0% of the reported cases in 2020 [ 158 ]. In Canada, since the virus's emergence in 2001, there have been over 5000 lab-confirmed human cases, with around 20.0% of patients experiencing neurological complications [ 159 , 160 ]. Additionally, it is estimated that up to 27,000 cases may have gone unreported, given the largely asymptomatic nature of WNV infection [ 160 ]. Similarly severe WNV outbreaks have hit Europe in recent years — its 2018 epidemic exceeded 1900 confirmed human cases, surpassing all previous years in scale and distribution [ 161 ]. The heavy health and economic toll has reasonably triggered intensive research interests in examining environmental risk factors such as climate change. Study interests and public health priorities understandably tend to align with acute epidemic events and tangible disease burden.
Research on WNV in regions like Asia, Africa, South America, and Oceania has been comparatively sparse. This imbalance may stem from various factors, such as a lower prioritization due to limited epidemiological data and clinical cases, often attributed to suboptimal surveillance systems. Additionally, the allocation of public health resources in these regions might be challenged by competing health issues, alongside barriers to conducting coordinated multi-national research. For example, in South America, inconsistencies between actual and reported WNV cases arise from symptomatic similarity with other arboviruses and limitations in differential laboratory diagnostics [ 24 ]. Moreover, mild and self-resolving cases may remain undocumented. Meanwhile, more severe cases can also be under-diagnosed, owing to a lack of accessible healthcare facilities and logistical constraints on sample transportation and testing [ 12 ].
Regional differences in climate, vector ecology, and host community characteristics contribute to variations in WNV transmission patterns and health impacts. For example, the primary vectors of WNV display distinct seasonality under varying climatic conditions [ 68 ]. Furthermore, viral strains may evolve different levels of pathogenicity in diverse host species and environmental settings [ 84 ]. Consequently, collaborative multi-regional research is essential to formulate prevention policies that are specifically tailored to different regions. Additionally, integrating knowledge and assessment tools is crucial to further understand the environmental and social factors driving WNV transmission.
Study population
The majority of research articles ( n = 103; 85.8%) focused exclusively on WNV, its vectors, or hosts. The remaining 17 articles (14.2%) examined WNV in conjunction with other mosquito-borne diseases, such as dengue fever and Rift Valley fever. The most studied subject was human WNV cases (Fig. 4 ), examined in 31.7% of articles ( n = 38) [ 23 , 27 , 47 , 48 , 52 , 53 , 54 , 56 , 58 , 59 , 62 , 66 , 71 , 73 , 78 , 82 , 83 , 90 , 96 , 98 , 102 , 109 , 118 , 122 , 124 , 125 , 126 , 128 , 130 , 131 , 132 , 133 , 134 , 135 , 140 , 143 , 145 , 148 ]. Mosquito vectors[ 49 , 55 , 57 , 61 , 65 , 68 , 69 , 72 , 74 , 80 , 84 , 87 , 88 , 91 , 92 , 93 , 95 , 97 , 101 , 103 , 105 , 107 , 108 , 110 , 111 , 116 , 121 , 127 , 129 , 136 , 139 , 144 , 150 , 152 , 153 , 155 ] and multi-species [ 22 , 26 , 28 , 51 , 60 , 64 , 67 , 70 , 75 , 76 , 77 , 79 , 81 , 86 , 94 , 99 , 100 , 106 , 117 , 119 , 123 , 141 , 142 , 146 ] were investigated in 36 (30.0%) and 24 (20.0%) studies, respectively. A smaller percentage of articles ( n = 10, 8.3%) failed to specify the study population [ 24 , 31 , 32 , 51 , 112 , 113 , 120 , 151 , 154 , 156 ]. Limited studies focused solely on bird hosts ( n = 7, 5.8%) [ 25 , 50 , 89 , 104 , 114 , 115 , 138 ] or equine hosts ( n = 5, 4.2%) [ 63 , 85 , 137 , 147 , 149 ].
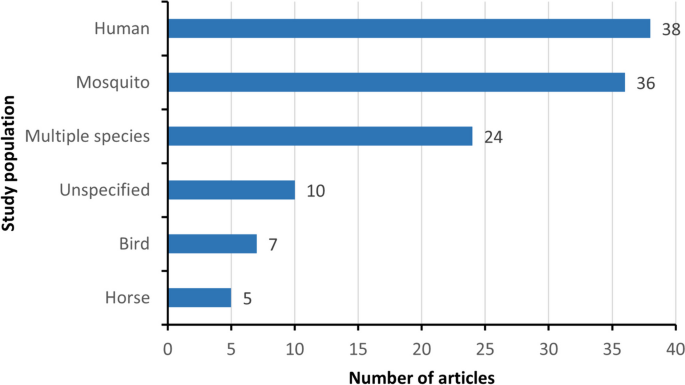
Distribution of the study populations in all articles included from 2007 to 2023
The majority of WNV research has focused on human infection. It's estimated that about 1 in 150 infected individuals develop a severe, long-lasting illness [ 162 ]. High incidence rates in humans have been linked to environmental factors such as extensive irrigated croplands and rural settings [ 54 ]. Mosquito vectors, particularly Culex species, play a crucial role in WNV transmission cycles, with their abundance influenced by factors like the urban heat island effect, the presence of water bodies, and the extent of irrigated farmland [ 54 , 129 ].
However, there is a significant gap in the number of animal-focused studies compared to human studies. In North America, over 28,000 equine cases of WNV have been reported since 1999 [ 163 ]. Additionally, in the US alone, the virus has impacted over 300 bird species, with estimated deaths in the millions [ 164 ]. Juvenile dispersing birds have been demonstrated to play a vital role in the long-distance dispersal and rapid spatial spread of introduced WNV strains across North America [ 165 ]. Given the importance of the role of animals in the transmission and evolution of WNV, there is a need to strengthen research on the impacts of climate change on the transmission and spread of WNV in animals.
Climate-WNV associations
Among the 83 articles examining climate/weather associations with WNV, temperature was the most studied factor ( n = 56, 67.5%) [ 28 , 47 , 49 , 51 , 52 , 54 , 55 , 58 , 59 , 60 , 61 , 63 , 64 , 66 , 72 , 75 , 76 , 79 , 80 , 81 , 82 , 84 , 86 , 87 , 88 , 89 , 92 , 93 , 94 , 98 , 105 , 107 , 108 , 109 , 110 , 117 , 118 , 119 , 120 , 121 , 122 , 123 , 124 , 125 , 126 , 127 , 128 , 129 , 130 , 132 , 134 , 137 , 138 , 139 , 147 , 150 ]. All these studies showed increased WNV transmission probabilities or cases within certain temperature ranges. Precipitation was assessed in 34 studies (41.0%), with 13 showing a positive correlation, 13 indicating a negative correlation, 7 revealing mixed positive/negative correlations, and 1 indicating no correlation with WNV risk [ 28 , 47 , 48 , 52 , 53 , 54 , 55 , 58 , 63 , 67 , 72 , 77 , 79 , 81 , 82 , 86 , 87 , 88 , 92 , 93 , 98 , 105 , 109 , 110 , 125 , 126 , 128 , 131 , 136 , 137 , 139 , 146 , 149 , 150 ]. Drought events and warmer winters were investigated less frequently, in 8 (9.7%) [ 28 , 56 , 72 , 74 , 85 , 119 , 126 , 146 ] and 5 (6.0%) [ 50 , 65 , 83 , 92 , 106 ] studies, respectively. Four articles (4.8%) showed a correlation between humidity and WNV risk, with 3 [ 47 , 80 , 91 ] showing a positive correlation and 1 [ 119 ] showing a negative correlation. Nine studies (10.8%) found links between WNV activity and climate-driven seasonal shifts [ 57 , 61 , 62 , 73 , 78 , 90 , 95 , 96 , 133 ], while 2 (2.4%) reported increased transmission associated with flooding events [ 135 , 153 ]. Three studies (3.6%) reported the correlation between WNV risk and winds/hurricanes [ 91 , 97 , 119 ].
Temperature and WNV
Ambient temperature is a critical driver influencing WNV transmission through direct and indirect impacts on vectors and hosts [ 49 , 71 ]. Specifically, higher temperatures accelerate viral replication and shorten the incubation period in mosquitoes, fuel vector population growth, increase transmission efficiency, and expand vector habitat suitability [ 49 ]. In Israel, positive temperature anomalies were linked to greater mosquito abundance and ensuing human cases [ 66 ]. Similarly in Canada, higher mean temperatures are associated with increased Culex populations and elevated WNV infections [ 129 ]. Moreover, there is a trend towards increased risk around large metropolitan areas characterized by urban heat islands, for example in the United Kingdom [ 129 ]. Phenomena such as warm winters and hot summers due to increased temperatures have also contributed to the rise in WNV infection rates [ 50 , 65 , 83 , 106 ]. The mean temperature is a strong predictor of the presence of WNV in Culex mosquitoes, and this relationship is unimodal [ 76 ]. The optimal temperature range for WNV transmission is identified as 22.7–30.2 °C [ 75 ]. Outside this range, particularly at temperatures below 17.0 °C, vector competence significantly declines, reaching a relative risk near zero [ 76 ]. It is important to note that this lower temperature threshold can vary among different vector species. Moreover, extreme heat events may further amplify outbreak magnitude [ 65 , 66 ]. However, it is important to note that an increase in temperature does not necessarily mean an increase in disease incidence altogether. For example, temperatures above 30 °C reduced survival of Culex tarsalis and slowed the growth of WNV in Culex mosquito [ 166 ].
In addition, ambient temperature rise under climate change may indirectly alter WNV transmission by shifting bird host ecology and associated vector exposures. Models project warming could expand bird infection prevalence to higher latitudes as longer activity seasons enable more transmission events [ 114 ]. While temperature alone allows increased vector habitat suitability and viral replication at mid-range optima, cascading impacts on avian immunity, migration timing, vector-host overlaps, and habitat ranges could potentially override direct effects. For instance, warmer climates have prompted earlier nesting in British birds, potentially leading to offspring hatching during peak mosquito seasons, increasing young birds’ exposure to vectors [ 167 ]. This phenomenon is exemplified in Caillouët et al.’s study, which demonstrates how the end of the nesting season aligns with higher mosquito populations, potentially escalating WNV transmission risks during these periods [ 168 ].
Precipitation and WNV
The positive correlation between elevated rainfall pre-outbreak and intensified WNV vector abundance/infection has been well documented [ 47 , 125 , 126 ]. For example, a 10 cm rise in summer precipitation was associated with 0.39 more WNV-positive Culex mosquitoes per 1000 tested in South Africa [ 67 ]. In the US, every 1 cm precipitation increase was linked to a 15% greater WNV incidence [ 86 ]. In Australia and Srpska, flooding due to extreme precipitation events creates favorable conditions for WNV transmission, as waterlogged environments can support larger populations of waterbirds and mosquitoes, increasing the likelihood of virus spread [ 135 , 153 ]. However, precipitation effects on WNV vary across regions and timescales, likely due to place-based differences in viral strain, vector, and host ecology. For example, a negative correlation between total monthly precipitation and the number of WNV cases was observed in Europe [ 128 ]. Similarly, in years of increased human WNV incidence in Israel, there was a significant decrease in spring precipitation [ 146 ]. In America, extreme drought caused by extremely low precipitation is a potential amplifier of WNV virus transmission and can further increase the risk of WNV transmission [ 56 , 85 ]. The cause of this phenomenon may be related to the fact that below-average precipitation creates limited water resources for mosquitoes, thereby increasing close contact between hosts and infected mosquitoes at remaining water sources [ 169 ]. In addition, both positive and negative correlations of precipitation on WNV incidence have been observed in the eastern and western parts of the US at different time scales [ 53 , 82 ].
Humidity, wind speed and WNV
Humidity and wind speed play important and complex roles in WNV transmission dynamics, but the impacts vary widely across ecosystems. For instance, higher humidity increased the probability of human infection with WNV in the US [ 47 ], and positive correlations were found between soil moisture and vector indices [ 80 ]. However, a Greek study conversely found negative relative humidity-WNV case correlations [ 119 ]. A study in New York and Connecticut showed an inverse U-shaped relationship between soil moisture and WNV-infected mosquitoes, with high infection associated with drought, but also an increase associated with wetter conditions—both patterns can be present at the same time [ 27 ]. Meanwhile, wind may impact disease transmission by influencing mosquito movement. For example, low wind speeds were found to be associated with the capture of WNV-infected mosquitoes during the same week that human cases of WNV emerged in Greece [ 119 ]. This may be related to the fact that high wind speeds reduce the chances of a mosquito blood meal, thus reducing the chances of human WNV cases [ 119 ]. Additional hypotheses, including storm roles in bird migration contributing to WNV transmission [ 170 ], require further investigation.
Climate-driven seasonal shifts and WNV
Climate-driven seasonal shifts are also important factors influencing WNV spread and outbreak magnitudes. For example, Texan counties experience major spikes following wet springs and hot, dry summers [ 73 ]. In Suffolk County, warm and dry conditions in early spring have been shown to increase WNV infection in Culex mosquitoes [ 74 ]. Patterns of dry, hot temperatures following wet years also increase WNV infections [ 78 ]. Broader European analyses suggest that anomalous seasonal temperatures and dry winters exacerbate seasonal amplification and drive WNV outbreaks [ 133 ]. These climate-mediated seasonal effects likely arise through multiple mechanisms affecting vector reproduction, host immunity, viral replication rates, and transmission efficiency at different phases [ 170 ]. As climate change intensifies precipitation variability and seasonal temperature extremes, such seasonal shift tipping points may become more frequent. Therefore, improved surveillance programs that are responsive to emerging seasonal shifts remain essential for predicting and mitigating transmission at fine geographic and temporal scales.
While climatic factors have a significant impact on the spread and transmission of WNV, many other factors also influence the complexity of the transmission dynamics. Land use, global trade, bird migration patterns, landscape features, and socioeconomics also partially determine the geographic distribution of infections [ 50 , 80 , 86 ]. For example, areas with older infrastructure, lower incomes, high percentages of cropland, and large rural populations have more landscape features and environmental conditions favorable to vector habitat, which increases local WNV risk [ 54 , 80 ]. Therefore, operationalizing the “One Health” paradigm through collaborative surveillance, modeling, and mitigation across veterinary, human, wildlife and environmental health remains imperative for fully anticipating and responding to shifting WNV.
Climate change impacts on WNV
Among the 37 articles examining climate change impacts on WNV, the majority ( n = 28; 75.7%) predicted the impact of climate change on WNV [ 22 , 23 , 24 , 27 , 31 , 32 , 69 , 70 , 99 , 100 , 101 , 102 , 103 , 104 , 111 , 112 , 113 , 114 , 115 , 116 , 141 , 142 , 143 , 145 , 151 , 152 , 155 , 156 ]. Specifically, high latitude regions, areas with immunocompromised populations, locations prone to extreme weather events, and marginalized communities were expected to be more affected [ 22 , 24 , 103 ]. Additionally, 8 articles (21.6%) provided substantial evidence that climatic variability phenomena have already affected the transmission and distribution of WNV during recent outbreaks [ 25 , 26 , 68 , 71 , 140 , 144 , 148 , 154 ]. Only 1 article (2.7%) focused on developing a national indicator framework for monitoring climate change impacts on infectious diseases [ 51 ].
Evidence of future climate change impacts on WNV
In the review, most evidence predicts that future climate change may affect the spread and distribution of WNV [ 22 , 23 , 24 , 111 , 114 , 151 , 155 , 156 ]. In North America, the projected climatic suitability range for WNV in 2050 and 2080 is expected to expand northward and into high-altitude areas, potentially leading to infections in novel and native hosts [ 22 ]. In Europe, studies project heightened WNV infection rates and new endemic areas under future climate scenarios, particularly at the margin of current transmission zones (e.g., eastern Croatia, northeastern and northwestern Turkey) [ 23 ]. Notably, recent evidence also confirms local transmissions as far north as Germany and the Netherlands, indicating an expansion of risk areas beyond those previously identified [ 10 , 11 ]. In South America, high-risk areas for WNV may shift between 2046–2065 and 2081–2100, becoming more pronounced under high greenhouse gas emission scenarios, potentially altering the current WNV distribution in some countries (e.g. parts of Bolivia, Paraguay, and Brazil) [ 24 ]. In Morocco, the suitable habitat range for Cx. pipiens is projected to expand into new central and southeastern areas by 2050, increasing the risk of WNV transmission [ 151 ].
Current evidence of climate change impacts on WNV
In addition to predictive studies on the future, existing evidence also demonstrates that climatic variability phenomena have already affected the transmission and distribution of WNV in some regions [ 25 , 26 , 144 , 148 ]. In the Powder River Basin of Montana and Wyoming in the US, WNV-related mortality rates in bird populations were significantly higher in 2003, the sixth warmest summer on record, than in 2004 and 2005, the 86th and 41st warmest, respectively [ 25 ]. Although this increase in mortality coincided with higher temperatures, it is crucial to consider that 2003 also marked a period of the virus’s initial introduction into the region. This introduction likely contributed significantly to the observed mortality rates, as populations are often most vulnerable when a pathogen first emerges. In Germany, the extreme heat of the summer of 2018 (the second hottest and most arid summer on record locally) was speculated to be an important reason for the decreased mean extrinsic incubation period values in mosquitoes, leading to rapid viral amplification and increased risk of transmission to vertebrate hosts [ 26 ]. Additionally, the detection of WNV-infected Uranotaenia unguiculata in northern Germany in 2016 presents another case of climate change driving the northward spread of mosquito species and WNV [ 144 ]. In Israel, an intense heat wave and a spike in summer temperatures were observed during WNV outbreaks [ 148 ].
The extent of climate change impacts on WNV transmission depends on local regional conditions, including population immunity levels and vector abundance [ 99 , 101 , 103 ]. In areas where comprehensive vaccination programs for animals susceptible to WNV, such as horses, are in place, alongside robust public health infrastructure and strong vector monitoring and control systems, the impact of WNV may be significantly mitigated or even negligible [ 103 ]. For example, predictions for the island scrub-jay in California showed that vaccinating ≥ 60 individuals during WNV outbreaks could decrease the risk from ≥ 22% to ≤ 5% [ 104 ]. Undoubtedly, strengthening broad-spectrum socioecological resilience through surveillance, preparedness, vector management, and medical capacity building remains paramount for sustainable health amidst climate and global change [ 101 ]. However, these anthropogenic measures require considerable regional coordination and resource mobilization, frequently lacking in disproportionately impacted communities. Therefore, actualizing equitable and adaptive WNV resilience necessitates comprehensively integrating climatological, environmental, veterinary, wildlife, genetic, immunological, and public health data into prediction frameworks and response protocols prioritizing vulnerable populations. International organizations must lead in facilitating such collaborative resilience measures globally.
Adaptation strategies to address climate-driven WNV transmission and spread
Among all 120 reviewed articles, 49 proposed or discussed adaptive strategies against WNV risks in response to climate change. These measures were categorized into six groups based on UNEP criteria and the case of WNV (Fig. 5 ) [ 44 ]: surveillance and monitoring ( n = 19; 38.8%) [ 22 , 23 , 56 , 65 , 69 , 75 , 85 , 92 , 99 , 100 , 101 , 106 , 117 , 120 , 123 , 135 , 148 , 151 , 152 ]; predictive models ( n = 9; 18.4%) [ 49 , 70 , 74 , 81 , 84 , 98 , 103 , 105 , 111 ]; cross-disciplinary/border cooperation ( n = 8; 16.3%) [ 24 , 51 , 80 , 126 , 131 , 133 , 141 , 156 ]; environmental management ( n = 6; 12.2%) [ 25 , 87 , 95 , 104 , 142 , 145 ]; health system preparation ( n = 4; 8.2%) [ 27 , 57 , 102 , 121 ]; and public education ( n = 3; 6.1%) [ 86 , 113 , 118 ]. A brief overview table of identified adaptation strategies is provided (Additional file 2 ), with details accessible on the project website under “Detailed adaptation strategies”.
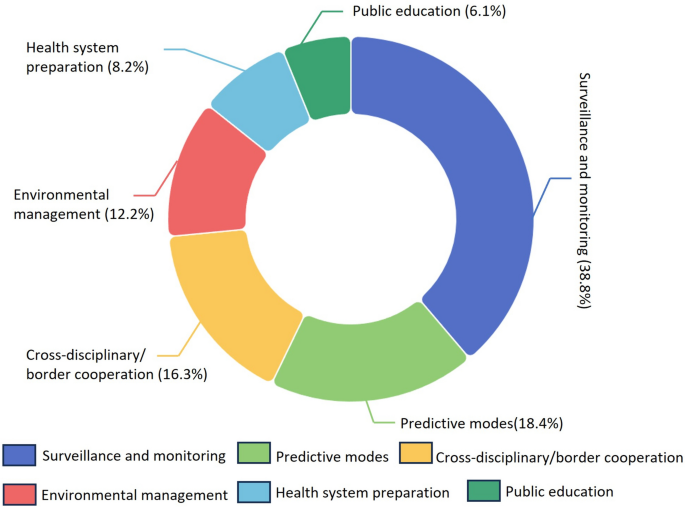
Classification of 49 articles that proposed or discussed adaptive strategies against West Nile virus risks in response to climate change, divided into six categories based on United Nations Environmental Program criteria
Monitoring and surveillance
Most studies reviewed highlight that monitoring and surveillance are the most critical means of preventing and controlling the spread of WNV under climate change scenarios. Specifically, surveillance should concentrate on high-risk populations, vector populations, wildlife and domestic animals, migrating birds, and neglected areas. As Skaff et al. noted, identifying consistencies between highly susceptible communities and local climates approaching critical thermal thresholds can enhance infectious disease prevention efficacy amidst climate change [ 75 ]. Additionally, Semenza et al. recommended fortifying epidemiological monitoring for neuroinvasive diseases potentially indicative of WNV to expand healthcare provider awareness of clinical manifestations and strengthen diagnostic testing capabilities [ 23 ]. They also advised augmenting blood donation screening and transportation safeguards while at the same time accounting for climate change in formulating robust WNV contamination prevention protocols [ 23 ]. Moreover, numerous studies have suggested that a more granular analysis of meteorological and entomological factors could improve comprehension of intricate WNV transmission dynamics [ 31 , 56 , 65 , 101 , 106 , 151 , 152 ]. Concurrently, research and control programs must localize to maximize relevance for regional climate change impacts [ 101 ]. Furthermore, public health agencies and vector control teams should amplify efforts to continuously track distributions to minimize human infection risks [ 151 , 152 ]. Meanwhile, WNV surveillance systems should be strengthened with host monitoring and regular risk assessment, especially for rural livestock, long-distance migratory birds, and wildlife with high mobility [ 117 , 123 ]. Domestic livestock, particularly horses in high-risk areas, should be vaccinated to enhance their immunity and prevent mortality and morbidity [ 85 ]. Routine surveillance should also be conducted in neglected areas (e.g., areas thought not to be transmitted zones and poor areas) [ 22 ]. Based on the results of data analysis from surveillance and monitoring, preventive and control strategies need to be adjusted accordingly to cope with changing infectious diseases.
Predictive models
Beyond intensifying surveillance, advancing predictive models and early warning systems remain vital for honing outbreak preparedness and rapid response. Sophisticated predictive tools enabling localized risk projections and efficient resource allocation can dramatically amplify intervention impact [ 38 ]. Ideally, such systems would synthesize meteorological, biological, genetic, ecological, entomological, and epidemiological data for accurate emergence prediction across scales [ 49 , 70 , 74 , 81 , 84 , 103 , 105 , 111 ]. Developing predictive models by linking laboratory-observed environmental transmission patterns to actual transmission patterns is crucial to accurately predicting the impact of climate change on WNV and other vector-borne pathogens [ 49 ]. Most importantly, next-generation frameworks must address substantial knowledge gaps around viral evolution, vector-host mutations, species migration and adaptation capacity, infection-recovery dynamics, and anthropogenic environmental change impacts on virus shifting dynamics [ 70 , 103 , 111 ]. Advancing models encompassing this intrinsic biocomplexity and policy-environment feedback remains essential to preempt unprecedented post-climate change outbreaks through context-specific preparation and response. International alliances should prioritize pioneering these innovations in prediction science alongside flexible surveillance strengthening for integrated epidemic resilience. Beyond informing ongoing emergence, these efforts will uncover complex ecological interconnectivity in the face of convergence across climate and global changes.
Cross-disciplinary/border cooperation
As climate change accelerates, advanced WNV prevention and control requires integrating “One Health” approaches across human, veterinary, wildlife, and environmental health sectors. Multidisciplinary collaboration enables the holistic elucidation of shifting transmission dynamics for accurate risk prediction, alert activation, and adaptive response [ 126 , 133 ]. Specifically, increased data sharing between public health, vector control, and meteorological agencies, coupled with artificial intelligence integration, can exponentially improve monitoring sensitivity, early warning trigger development, and outbreak interception agility [ 24 , 80 ]. Additionally, transregional information exchange and coordination remain imperative for refining control strategies and resource allocation amidst climate and global change [ 156 ]. The 2018 European WNV emergency exemplified the superiority of integrated “One Health” surveillance, ensuring targeted data-driven countermeasures, bridging counties halted uncontrolled cross-border transmission [ 141 ]. Given the existential threat of vector-borne diseases necessitates all governmental and international institutions prioritizing and operationalizing such interdisciplinary preparedness and response architectures. This obligation will grow increasingly urgent as environments continue transforming unprecedentedly.
Other adaptive strategies
Of all the studies reviewed, there are fewer strategies related to public education, environmental management, and health system preparedness. However, adapting to the growing threat of WNV under climate change will require multifaceted strategies across environmental management, public awareness-raising, and health system preparedness. Effective environmental management to suppress vector populations, including the elimination of mosquito breeding grounds and the establishment of secondary conserved populations for possible vaccination, forms a crucial first line of defense against WNV [ 25 , 104 , 142 ]. However, this must be coupled with sustained public education campaigns to promote protective behaviors among individuals and vigilant surveillance efforts to enable early response [ 86 , 113 , 118 ]. Finally, health systems must enhance their capacity for detecting WNV outbreaks in vectors and hosts, allowing timely intervention measures, as well as boosting clinical diagnosis and treatment capacity [ 102 , 121 ].
Future work
While this review concentrated on the climatic aspects of WNV transmission, it sets the stage for subsequent in-depth analyses of adaptation strategies within the public health domain. Future studies could adopt a One Health approach or leverage the UNEP framework to explore diverse responses to WNV, thereby enriching the dialogue between climate science and public health policy.
Climate change may affect the transmission and distribution of WNV, with the extent of the impact depending on local and regional conditions. Surveillance and monitoring stand out as the most recommended adaptation tactics to address the spread of WNV under climate change scenarios. However, far fewer studies have explicitly focused on adaptation strategies than have investigated the impacts of climate change. Further research on the impacts of climate change and adaptation strategies for vector-borne diseases, as well as more comprehensive evidence synthesis, are needed to inform effective policy responses tailored to local contexts.
Our findings highlight the significant role of climate factors in the transmission dynamics of WNV. However, acknowledging the limitations of our focus, we propose future research to extensively explore adaptation strategies that address these climatic challenges. Such efforts would provide comprehensive insights that are crucial for the development of robust public health policies.
Availability of data and materials
All data generated or analysed during this study are included in this published article and its supplementary information files.
Abbreviations
- West Nile virus
Intergovernmental Panel on Climate Change
United Nations Environmental Program
Kramer LD, Ciota AT, Kilpatrick AM. Introduction, spread, and establishment of West Nile virus in the Americas. J Med Entomol. 2019;56(6):1448–55.
Article PubMed PubMed Central Google Scholar
Bernkopf H, Levine S, Nerson R. Isolation of West Nile virus in Israel. Infect Dis. 1953;93:207–18.
Article CAS Google Scholar
Murgue B, Zeller H, Deubel V. The ecology and epidemiology of West Nile virus in Africa, Europe and Asia. Curr Top Microbiol Immunol. 2002;267:195–221.
CAS PubMed Google Scholar
Johnson N, de FernándezMarco M, Giovannini A, et al. Emerging mosquito-borne threats and the response from European and Eastern Mediterranean countries. Int J Environ Res Public Health. 2018;15(12):2775.
Nash D, Mostashari F, Fine A, Miller J, O’leary D, Murray K, et al. The outbreak of West Nile virus infection in the New York City area in 1999. N Engl J Med. 2001;344:1807–14.
Article CAS PubMed Google Scholar
Petersen LR, Hayes EB. West Nile virus in the Americas. Med Clin North Am. 2008;92:1307–22.
Article PubMed Google Scholar
Lindsey NP, Staples JE, Lehman JA, Fischer M. Surveillance for human West Nile virus disease-United States, 1999–2008. MMWR Surveill Summ. 2010;59:1–17.
PubMed Google Scholar
Haussig JM, Young JJ, Gossner CM, Mezei E, Bella A, Sirbu A, et al. Early start of the West Nile fever transmission season 2018 in Europe. Euro Surveill. 2018;23(32):1800428.
Camp JV, Nowotny N. The knowns and unknowns of West Nile virus in Europe: what did we learn from the 2018 outbreak? Expert Rev Anti-Infect Ther. 2020;18(2):145–54.
Pietsch C, Michalski D, Münch J, Petros S, Bergs S, Trawinski H, et al. Autochthonous West Nile virus infection outbreak in humans, Leipzig, Germany, August to September 2020. Euro Surveill. 2020;25(46):2001786.
Article CAS PubMed PubMed Central Google Scholar
Vlaskamp DRM, Thijsen SFT, Reimerink J, Hilkens P, Bouvy WH, Bantjes SE, et al. First autochthonous human West Nile virus infections in the Netherlands, July to August 2020. Euro Surveill. 2020;25(46):2001904.
Kramer LD, Styer LM, Ebel GD. A global perspective on the epidemiology of West Nile virus. Annu Rev Entomol. 2008;53:61–81.
Kilpatrick AM, Ladeau SL, Marra PP. Ecology of West Nile virus transmission and its impact on birds in the western hemisphere. Auk. 2007;124(4):1121–36.
Article Google Scholar
Gómez A, Kilpatrick AM, Kramer LD, Dupuis AP, Maffei JG, Goetz SJ, et al. Land use and West Nile virus seroprevalence in wild mammals. Emerg Infect Dis. 2008;14(6):962.
David S, Abraham AM. Epidemiological and clinical aspects on West Nile virus, a globally emerging pathogen. Infect Dis. 2016;48:571–86.
Centers for Disease Control. West Nile virus - statistics & maps in 2018. https://www.cdc.gov/westnile/statsmaps/index.html . Accessed 13 June 2023.
Ciota AT. West Nile virus and its vectors. Curr Opin Insect Sci. 2017;22:28–36.
Kilpatrick AM. Globalization, land use, and the invasion of West Nile virus. Science. 2011;334(6054):323–7.
Jia Y, Moudy RM, Dupuis AP II, Ngo KA, Maffei JG, Jerzak GV, et al. Characterization of a small plaque variant of West Nile virus isolated in New York in 2000. Virology. 2007;367:339–47.
Kunkel KE, Novak RJ, Lampman RL, Gu W. Modeling the impact of variable climatic factors on the crossover of Culex restauns and Culex pipiens (Diptera: Culicidae), vectors of West Nile virus in Illinois. Am J Trop Med Hyg. 2006;74:16–173.
Watts N, Amann M, Arnell N, Ayeb-Karlsson S, Beagley J, Belesova K, et al. The 2020 report of The Lancet Countdown on health and climate change: responding to converging crises. Lancet. 2021;397(10269):129–70.
Harrigan RJ, Thomassen HA, Buermann W, Smith T. A continental risk assessment of West Nile virus under climate change. Global Change Biol. 2014;20(8):2417–25.
Semenza JC, Tran A, Espinosa L, Sudre B, Domanovic D, Paz S. Climate change projections of West Nile virus infections in Europe: implications for blood safety practices. Environ Health. 2016;15(1):125–36.
Google Scholar
Lorenz C, de Azevedo TS, Chiaravalloti-Neto F. Impact of climate change on West Nile virus distribution in South America. Trans R Soc Trop Med Hyg. 2022;116(11):1043–53.
Walker BL, Naugle DE, Doherty KE, Cornish TE. West Nile virus and greater sage-grouse: estimating infection rate in a wild bird population. Avian Dis. 2007;51(3):691–6.
Ziegler U, Lühken R, Keller M, Cadar D, van der Grinten E, Michel F, et al. West Nile virus epizootic in Germany, 2018. Antivir Res. 2019;162:39–43.
Keyel AC. Patterns of West Nile virus in the Northeastern United States using negative binomial and mechanistic trait-based models. GeoHealth. 2023;7(4):e2022GH000747.
Keyel AC, Elison Timm O, Backenson PB, Prussing C, Quinones S, McDonough KA, et al. Seasonal temperatures and hydrological conditions improve the prediction of West Nile virus infection rates in Culex mosquitoes and human case counts in New York and Connecticut. Plos One. 2019;14(6): e0217854.
Chersich MF, Wright CY. Climate change adaptation in South Africa: a case study on the role of the health sector. Global Health. 2019;15:1–16.
Bardosh KL, Ryan S, Ebi K, Welburn S, Singer B. Addressing vulnerability, building resilience: community-based adaptation to vector-borne diseases in the context of global change. Infect Dis Pover. 2017;6(1):166.
Cox R, Sanchez J, Revie CW. Multi-criteria decision analysis tools for prioritising emerging or re-emerging infectious diseases associated with climate change in Canada. Plos One. 2013;8(8): e68338.
Hongoh V, Michel P, Gosselin P, Samoura K, Ravel A, Campagna C, et al. Multi-stakeholder decision aid for improved prioritization of the public health impact of climate sensitive infectious diseases. Int J Environ Res Public Health. 2016;13(4):419.
Pachauri RK, Reisinger A. Climate Change 2007: synthesis Report. Contribution of working groups I, II and III to the fourth assessment report of the intergovernmental panel on climate change. Geneva, Switzerland: IPCC; 2007. p. 104.
Pachauri RK, Allen MR, Barros VR, Broome J, Cramer W, Christ R, Climate change, et al. Synthesis Report, Contribution of Working Groups I, II and III to the Fifth Assessment Report of the Intergovernmental Panel on Climate Change. IPCC. 2014;2014:151.
Watts N, Adger WN, Agnolucci P. Health and climate change: policy responses to protect public health. Lancet. 2015;386(10006):1861–914.
Eder M, Cortes F, de SiqueiraFilhaTeixeira N, de Franca Araújo GV, Degroote S, Braga C, et al. Scoping review on vector-borne diseases in urban areas: transmission dynamics, vectorial capacity and co-infection. Infect Dis Poverty. 2018;7(1):1–24.
Orr M, Inoue Y, Seymour R, Dingle G. Impacts of climate change on organized sport: a scoping review. WIREs Clim Change. 2022;13(3): e760.
Kulkarni MA, Duguay C, Ost K. Charting the evidence for climate change impacts on the global spread of malaria and dengue and adaptive responses: a scoping review of reviews. Global Health. 2022;18(1):1–18.
Schultz A, Goertzen L, Rothney J, Wener P, Enns J, Halas G, et al. A scoping approach to systematically review published reviews: adaptations and recommendations. Res Synth Methods. 2018;9(1):116–23.
Tricco AC, Lillie E, Zarin W, O’Brien KK, Colquhoun H, Levac D, et al. PRISMA extension for scoping reviews (PRISMA-ScR): checklist and explanation. Ann Intern Med. 2018;169:467–73.
Sweileh WM. Bibliometric analysis of peer-reviewed literature on climate change and human health with an emphasis on infectious diseases. Glob Health. 2020;16(1):44.
Masson-Delmotte VP, Zhai P, Pirani SL, Connors C, Péan S, Berger N, et al. IPCC. Summary for Policymakers. In: Climate change 2021: The Physical Science Basis. Contribution of Working Group I to the Sixth Assessment Report of the Intergovernmental Panel on Climate Change. Cambridge, UK: Cambridge University Press; 2021.
IPCC. An IPCC Special Report on the impacts of global warming of 1.5°C above preindustrial levels and related global greenhouse gas emission pathways, in the context of strengthening the global response to the threat of climate change, sustainable development, and efforts to eradicate poverty. https://pure.iiasa.ac.at/id/eprint/15716/1/SR15_TS_High_Res.pdf . Accessed 29 Jan 2019.
Feenstra JF, Burton I, Smith JB, Tol RS. Handbook on Methods for Climate Change Impact Assessment and Adaptation Strategies. Amsterdam: Vrije University; 1998.
Isaiah PM, Sólveig Palmeirim M, Steinmann P. Epidemiology of pediatric schistosomiasis in hard-to-reach areas and populations: a scoping review. Infect Dis Poverty. 2023;12(1):37.
Wang H, Guo T, Wang Z, Xiao J, Gao L, Gao X, et al. PreCowKetosis: A Shiny web application for predicting the risk of ketosis in dairy cows using prenatal indicators. Comput Electron Agr. 2023;206: 107697.
Soverow JE, Wellenius GA, Fisman DN, Mittleman MA. Infectious disease in a warming world: how weather influenced West Nile virus in the United States (2001–2005). Environ Health Persp. 2009;117(7):1049–52.
Wang G, Minnis RB, Belant JL, Wax CL. Dry weather induces outbreaks of human West Nile virus infections. BMC Infect Dis. 2010;10:38–38.
Kilpatrick AM, Meola MA, Moudy RM, Kramer LD. Temperature, viral genetics, and the transmission of West Nile virus by Culex pipiens mosquitoes. Plos Pathog. 2008;4(6):e1000092.
LaDeau SL, Calder CA, Doran PJ, Marra PP. West Nile virus impacts in American crow populations are associated with human land use and climate. Ecol Res. 2011;26:909–16.
Liu H, Weng Q. Environmental factors and risk areas of West Nile Virus in southern California, 2007–2009. Environ Model Assess. 2012;17:441–52.
Walsh MG. The role of hydrogeography and climate in the landscape epidemiology of West Nile virus in New York State from 2000 to 2010. Plos One. 2012;7(2): e30620.
Landesman WJ, Allan BF, Langerhans RB, Knight TM, Chase JM. Inter-annual associations between precipitation and human incidence of West Nile virus in the United States. Vector-Borne Zoonot. 2007;7(3):337–43.
Wimberly MC, Hildreth MB, Boyte SP, Lindquist E, Kightlinger L. Ecological niche of the 2003 West Nile virus epidemic in the northern Great Plains of the United States. Plos One. 2008;3(12): e3744.
Deichmeister JM, Telang A. Abundance of West Nile virus mosquito vectors in relation to climate and landscape variables. J Vector Ecol. 2011;36(1):75–85.
DeGroote JP, Sugumaran R, Brend SM, Tucker BJ, Bartholomay LC. Landscape, demographic, entomological, and climatic associations with human disease incidence of West Nile virus in the state of Iowa, USA. Int J Health Geogr. 2008;7(1):1–16.
Shaman J, Harding K, Campbell SR. Meteorological and hydrological influences on the spatial and temporal prevalence of West Nile virus in Culex mosquitoes, Suffolk County. New York J Med Entomol. 2011;48(4):867–75.
Winters AM, Eisen RJ, Lozano-Fuentes S, Moore CG, Pape WJ, Eisen L. Predictive spatial models for risk of West Nile virus exposure in eastern and western Colorado. Am J Trop Med Hyg. 2008;79(4):581.
Chuang TW, Wimberly MC. Remote sensing of climatic anomalies and West Nile virus incidence in the northern Great Plains of the United States. Plos One. 2012;7(10): e46882.
Hartley DM, Barker CM, Le Menach A, Niu T, Gaff HD, Reisen WK. Effects of temperature on emergence and seasonality of West Nile virus in California. Am J Trop Med Hyg. 2012;86(5):884.
Ruiz MO, Chaves LF, Hamer GL, Sun T, Brown WM, Walker ED, et al. Local impact of temperature and precipitation on West Nile virus infection in Culex species mosquitoes in northeast Illinois, USA. Parasites Vector. 2010;3(1):1–16.
Shaman J, Day JF, Komar N. Hydrologic conditions describe West Nile virus risk in Colorado. Int J Environ Res Public He. 2010;7(2):494–508.
Epp TY, Waldner C, Berke O. Predictive risk mapping of West Nile virus (WNV) infection in Saskatchewan horses. Can J Vet Res. 2011;75(3):161–70.
PubMed PubMed Central Google Scholar
Roth D, Henry B, Mak S, Fraser M, Taylor M, Li M, et al. West Nile virus range expansion into British Columbia. Emerg Infect Dis. 2010;16(8):1251.
Platonov AE, Tolpin VA, Gridneva KA, Titkov AV, Platonova OV, Kolyasnikova NM, et al. The incidence of West Nile disease in Russia in relation to climatic and environmental factors. Int J Environ Res Public Health. 2014;11(2):1211–32.
Paz S, Albersheim I. Influence of warming tendency on Culex pipiens population abundance and on the probability of West Nile fever outbreaks (Israeli case study:2001–2005). EcoHealth. 2008;5:40–8.
Uejio CK, Kemp A, Comrie AC. Climatic controls on West Nile virus and Sindbis virus transmission and outbreaks in South Africa. Vector-Borne Zoonot. 2012;12(2):117–25.
Chaves LF, Hamer GL, Walker ED, Brown WM, Ruiz MO, Kitron UD. Climatic variability and landscape heterogeneity impact urban mosquito diversity and vector abundance and infection. Ecosphere. 2011;2(6):1–21.
Hongoh V, Berrang-Ford L, Scott ME, Lindsay LR. Expanding geographical distribution of the mosquito, Culex pipiens , in Canada under climate change. Appl Geogr. 2012;33:53–62.
Gale P, Brouwer A, Ramnial V, Kelly L, Kosmider R, Fooks AR, et al. Assessing the impact of climate change on vector-borne viruses in the EU through the elicitation of expert opinion. Epidemiol Infect. 2010;138(2):214–25.
Paz S, Malkinson D, Green MS, Tsioni G, Papa A, Danis K, et al. Permissive summer temperatures of the 2010 European West Nile fever upsurge. Plos One. 2013;8(2): e56398.
Johnson BJ, Sukhdeo MVK. Drought-induced amplification of local and regional West Nile virus infection rates in New Jersey. J Med Entomol. 2013;50(1):195–204.
Ukawuba I, Shaman J. Association of spring-summer hydrology and meteorology with human West Nile virus infection in West Texas, USA, 2002–2016. Parasites Vector. 2018;11:1–15.
Little E, Campbell SR, Shaman J. Development and validation of a climate-based ensemble prediction model for West Nile virus infection rates in Culex mosquitoes, Suffolk County New York. Parasites Vector. 2016;9(1):1–13.
Skaff NK, Cheng Q, Clemesha RES, Collender PA, Gershunov A, Head JR, et al. Thermal thresholds heighten sensitivity of West Nile virus transmission to changing temperatures in coastal California. Proc Biol Sci. 2020;287(1932):20201065.
Shocket MS, Verwillow AB, Numazu MG, Slamani H, Cohen JM, El Moustaid F, et al. Transmission of West Nile and five other temperate mosquito-borne viruses peaks at temperatures between 23 C and 26 C. Elife. 2020;9: e58511.
Crowder DW, Dykstra EA, Brauner JM, Duffy A, Reed C, Martin E, et al. West Nile virus prevalence across landscapes is mediated by local effects of agriculture on vector and host communities. Plos One. 2013;8(1): e55006.
Smith KH, Tyre AJ, Hamik J, Hayes MJ, Zhou Y, Dai L. Using climate to explain and predict West Nile Virus risk in Nebraska. GeoHealth. 2020;4(9):e2020GH000244.
Tokarz RE, Smith RC. Crossover dynamics of Culex (Diptera: Culicidae) vector populations determine WNV transmission intensity. J Med Entomol. 2020;57(1):289–96.
Lockaby G, Noori N, Morse W, Zipperer W, Kalin L, Governo R, et al. Climatic, ecological, and socioeconomic factors associated with West Nile virus incidence in Atlanta, Georgia, USA. J Vector Ecol. 2016;41(2):232–43.
Uelmen JA, Brokopp C, Patz J. A 15 year evaluation of West Nile Virus in Wisconsin: effects on wildlife and human health. Int J Environ Res Public Health. 2020;17(5):1767.
Hahn MB, Monaghan AJ, Hayden MH, Eisen RJ, Delorey MJ, Lindsey NP, et al. Meteorological conditions associated with increased incidence of West Nile virus disease in the United States, 2004–2012. Am J Trop Med Hyg. 2015;92(5):1013.
Wimberly MC, Lamsal A, Giacomo P, Chuang TW. Regional variation of climatic influences on West Nile virus outbreaks in the United States. Am J Trop Med Hyg. 2014;91(4):677.
Fay RL, Ngo KA, Kuo L, Willsey GG, Kramer LD, Ciota AT. Experimental evolution of West Nile virus at higher temperatures facilitates broad adaptation and increased genetic diversity. Viruses. 2021;13(10):1889.
Humphreys JM, Pelzel-McCluskey AM, Cohnstaedt LW, McGregor BL, Hanley KA, Hudson AR, et al. Integrating spatiotemporal epidemiology, eco-phylogenetics, and distributional ecology to assess West Nile disease risk in horses. Viruses. 2021;13(9):1811.
Hernandez E, Torres R, Joyce AL. Environmental and sociological factors associated with the incidence of West Nile virus cases in the Northern San Joaquin Valley of California, 2011–2015. Vector-Borne Zoonot. 2019;19(11):851–8.
Myer MH, Campbell SR, Johnston JM. Spatiotemporal modeling of ecological and sociological predictors of West Nile virus in Suffolk County, NY, mosquitoes. Ecosphere. 2017;8(6): e01854.
Myer MH, Johnston JM. Spatiotemporal Bayesian modeling of West Nile virus: Identifying risk of infection in mosquitoes with local-scale predictors. Sci Total Environ. 2019;650:2818–29.
Kala AK, Tiwari C, Mikler AR, Atkinson SF. A comparison of least squares regression and geographically weighted regression modeling of West Nile virus risk based on environmental parameters. Peer J. 2017;5: e3070.
Day JF, Shaman J. Using hydrologic conditions to forecast the risk of focal and epidemic arboviral transmission in peninsular Florida. J Med Entomol. 2014;45(3):458–65.
Peper ST, Dawson DE, Dacko N, Athanasiou K, Hunter J, Loko F, et al. Predictive modeling for West Nile virus and mosquito surveillance in Lubbock Texas. J Am Mosquito Contr. 2018;34(1):18–24.
Poh KC, Chaves LF, Reyna-Nava M, Roberts CM, Fredregill C, Bueno R Jr, et al. The influence of weather and weather variability on mosquito abundance and infection with West Nile virus in Harris County, Texas, USA. Sci Total Environ. 2019;675:260–72.
Shand L, Brown WM, Chaves LF, Goldberg TL, Hamer GL, Haramis L, et al. Predicting West Nile virus infection risk from the synergistic effects of rainfall and temperature. J Med Entomol. 2016;53(4):935–44.
Mori H, Wu J, Ibaraki M, Schwartz FW. Key factors influencing the incidence of West Nile virus in Burleigh County, North Dakota. Int J Environ Res Public Health. 2018;15(9):1928.
Ward MJ, Sorek-Hamer M, Henke JA, Little E, Patel A, Shaman J, et al. A spatially resolved and environmentally informed forecast model of West Nile virus in Coachella Valley, California. GeoHealth. 2023;7(12):e2023GH000855.
Gorris ME, Randerson JT, Coffield SR, Treseder KK, Zender CS, Xu C, Manore CA. Assessing the influence of climate on the spatial pattern of West Nile virus incidence in the United States. Environ Health Perspect. 2023;131(4):047016.
Huang X, Athrey GN, Kaufman PE, Fredregill C, Slotman MA. Effective population size of Culex quinquefasciatus under insecticide-based vector management and following Hurricane Harvey in Harris County Texas. Front Genet. 2023;14:1297271.
Holcomb KM, Mathis S, Staples JE, Fischer M, Barker CM, Beard CB, et al. Evaluation of an open forecasting challenge to assess skill of West Nile virus neuroinvasive disease prediction. Parasite Vector. 2023;16(1):11.
Paull SH, Horton DE, Ashfaq M, Rastogi D, Kramer LD, Diffenbaugh NS, et al. Drought and immunity determine the intensity of West Nile virus epidemics and climate change impacts. P Roy Soc B-Biol Sci. 1848;2017(284):20162078.
Keyel AC, Raghavendra A, Ciota AT, Elison TO. West Nile virus is predicted to be more geographically widespread in New York State and Connecticut under future climate change. Global Change Biol. 2021;27(21):5430–45.
Morin CW, Comrie AC. Regional and seasonal response of a West Nile virus vector to climate change. Proc Natl Acad Sci U S A. 2013;110(39):15620–5.
Filippelli GM, Freeman JL, Gibson J, Jay S, Moreno-Madriñán MJ, Ogashawara I, et al. Climate change impacts on human health at an actionable scale: a state-level assessment of Indiana, USA. Clim Change. 2020;163(4):1985–2004.
Brown HE, Young A, Lega J, Andreadis TG, Schurich J, Comrie A. Projection of climate change influences on US West Nile virus vectors. Earth Interact. 2015;19(18):1–18.
Bakker VJ, Sillett TS, Boyce WM, Doak DF, Vickers TW, Reisen WK, et al. Translocation with targeted vaccination is the most effective strategy to protect an island endemic bird threatened by West Nile virus. Divers Distrib. 2020;26(9):1104–15.
Chen CC, Epp T, Jenkins E, Waldner C, Curry PS, Soos C, et al. Modeling monthly variation of Culex tarsalis (Diptera: Culicidae) abundance and West Nile Virus infection rate in the Canadian Prairies. Int J Environ Res Public Health. 2013;10(7):3033–51.
Mallya S, Sander B, Roy-Gagnon MH, Taljaard M, Jolly A, Kulkarn MA. Factors associated with human West Nile virus infection in Ontario: a generalized linear mixed modelling approach. BMC Infect Dis. 2018;18(1):1–9.
Temple SD, Manore CA, Kaufeld KA. Bayesian time-varying occupancy model for West Nile virus in Ontario Canada. Stoch Environ Res Risk Assess. 2022;36(8):2337–52.
Talbot B, Kulkarni MA, Rioux-Rousseau M, Siebels K, Kotchi SO, Ogden NH, et al. Ecological niche and positive clusters of two West Nile virus vector in Ontario Canada. EcoHealth. 2023;20(3):249–62.
Albrecht L, Kaufeld KA. Investigating the impact of environmental factors on West Nile virus human case prediction in Ontario Canada. Front Public Health. 2023;11:1100543.
Baril C, Pilling BG, Mikkelsen MJ, Sparrow JM, Duncan CAM, Koloski CW, et al. The influence of weather on the population dynamics of common mosquito vector species in the Canadian Prairies. Parasite Vector. 2023;16(1):153.
Chen CC, Jenkins E, Epp T, Waldner C, Curry PS, Soos C. Climate change and West Nile virus in a highly endemic region of North America. Int J Environ Res Public Health. 2013;10(7):3052–71.
Otten A, Fazil A, Chemeris A, Breadner P, Ng V. Prioritization of vector-borne diseases in Canada under current climate and projected climate change. Microbial Risk Anal. 2020;14:100089.
Hongoh V, Campagna C, Panic M, Samuel O, Gosselin P, Waaub JP, et al. Assessing interventions to manage West Nile virus using multi-criteria decision analysis with risk scenarios. Plos One. 2016;11(8): e0160651.
Tam BY, Tsuji LJS. West Nile virus in American crows ( Corvus brachyrhynchos ) in Canada: projecting the influence of climate change. GeoJournal. 2016;81:89–101.
Tam BY, Martin I, Tsuji LJS. Geospatial analysis between the environment and past incidences of West Nile virus in bird specimens in Ontario Canada. GeoJournal. 2014;79:805–17.
Rakotoarinia MR, Seidou O, Lapen DR, Leighton PA, Ogden NH, Ludwig A. Future land-use change predictions using Dyna-Clue to support mosquito-borne disease risk assessment. Environ Monit Assess. 2023;195(7):815.
Di Pol G, Crotta M, Taylor RA. Modelling the temperature suitability for the risk of West Nile Virus establishment in European Culex pipiens populations. Transbound Emerg Dis. 2022;69(5):1787–99.
Coroian M, Petrić M, Pistol A, Sirbu A, Domșa C, Mihalca AD. Human West Nile Meningo-Encephalitis in a highly endemic country: a complex epidemiological analysis on biotic and abiotic risk factors. Int J Environ Res Public Health. 2020;17(21):8250.
Stilianakis NI, Syrris V, Petroliagkis T, Pärt P, Gewehr S, Kalaitzopoulou S, et al. Identification of climatic factors affecting the epidemiology of human West Nile virus infections in northern Greece. Plos One. 2016;11(9): e0161510.
Vogels CB, Hartemink N, Koenraadt CJM. Modelling West Nile virus transmission risk in Europe: effect of temperature and mosquito biotypes on the basic reproduction number. Sci Rep. 2017;7(1):5022.
Fros JJ, Geertsema C, Vogels CB, Roosjen PP, Failloux AB, Vlak JM, et al. West Nile virus: high transmission rate in north-western European mosquitoes indicates its epidemic potential and warrants increased surveillance. Plos Neglect Trop Dis. 2015;9(7):e0003956.
Tran A, Sudre B, Paz S, Rossi M, Desbrosse A, Chevalier V, et al. Environmental predictors of West Nile fever risk in Europe. Int J Health Geogr. 2014;13:1–11.
Radojicic S, Zivulj A, Petrovic T, Nisavic J, Milicevic V, Sipetic-Grujicic S, et al. Spatiotemporal analysis of West Nile virus epidemic in South Banat District, Serbia, 2017–2019. Animals. 2021;11(10):2951.
Platonov AE, Fedorova MV, Karan LS, Shopenskaya TA, Platonova OV, Zhuravlev VI. Epidemiology of West Nile infection in Volgograd, Russia, in relation to climate change and mosquito (Diptera: Culicidae) bionomics. Parasitol Res. 2008;1(103):45–53.
Moirano G, Gasparrini A, Acquaotta F, Fratianni S, Merletti F, Maule M, et al. West Nile virus infection in Northern Italy: Case-crossover study on the short-term effect of climatic parameters. Environ Res. 2018;167:544–9.
Marcantonio M, Rizzoli A, Metz M, Rosà R, Marini G, Chadwick E, et al. Identifying the environmental conditions favouring West Nile virus outbreaks in Europe. Plos One. 2015;10(3): e0121158.
Mihailović DT, Petrić D, Petrović T, Hrnjaković-Cvjetković I, Djurdjevic V, Nikolić-Đorić E, et al. Assessment of climate change impact on the malaria vector Anopheles hyrcanus, West Nile disease, and incidence of melanoma in the Vojvodina Province (Serbia) using data from a regional climate model. Plos One. 2020;15(1): e0227679.
Trájer AJ, Bede-Fazekas Á, Bobvos J, Páldy A. Seasonality and geographical occurrence of West Nile fever and distribution of Asian tiger mosquito. Q J Hung Meteorol Se. 2014;118(1):19–40.
Townroe S, Callaghan A. British container breeding mosquitoes: the impact of urbanisation and climate change on community composition and phenology. Plos One. 2014;9(4): e95325.
Paz S. West Nile Virus Eruptions in Summer 2010–What Is the Possible Linkage with Climate Change? Netherlands: National Security and Human Health Implications of Climate Change. Springer; 2012. p. 253–60.
Mavrakis A, Papavasileiou C, Alexakis D, Papakitsos EC, Salvati L. Meteorological patterns and the evolution of West Nile virus in an environmentally stressed Mediterranean area. Environ Monit Assess. 2021;193:1–11.
Vlasova NV, Masyagutova LM, Abdrakhmanova ER, Rafikova LA, Chudnovets GM. A conceptual scheme of a predictive-analytical model for describing incidence of west nile fever based on weather and climate estimation (exemplified by the Volgograd region). Health Risk Anal. 2022;4:124.
Farooq Z, Rocklöv J, Wallin J, Abiri N, Sewe MO, Sjödin H, et al. Artificial intelligence to predict West Nile virus outbreaks with ecoclimatic drivers. Lancet Reg Health Eu. 2022;17:100370.
Marini G, Pugliese A, Wint W, Alexander NS, Rizzoli A, Rosà R. Modelling the West Nile virus force of infection in the European human population. One Health. 2022;15: 100462.
Vukmir NR, Bojanić J, Mijović B, Roganović T, Aćimović J. Did intensive floods influence higher incidence rate of the West Nile virus in the population exposed to flooding in the Republic of Srpska in 2014. Arch Vet Med. 2019;12(1):21–32.
Krol L, Blom R, Dellar M, van der Beek JG, Stroo ACJ, van Bodegom PM, et al. Interactive effects of climate, land use and soil type on Culex pipiens/torrentium abundance. One Health. 2023;17: 100589.
Magallanes S, Llorente F, Ruiz-López MJ, de la PuenteMartinez - J, Soriguer R, Calderon J, et al. Long-term serological surveillance for West Nile and Usutu virus in horses in south-West Spain. One Health. 2023;17:100578.
Niczyporuk JS, Kozdrun W, Czujkowska A, Blanchard Y, Helle M, Dheilly NM, et al. West Nile virus lineage 2 in free-living Corvus cornix birds in Poland. Trop Med Infect Dis. 2023;8(8):417.
Angelou A, Gewehr S, Mourelatos S, Kioutsioukis I. Early warning impact of temperature and rainfall anomalies onto West Nile virus human cases. Environ Sci Proc. 2023;26(1):93.
Watts MJ, iMonteys VS, Mortyn PG, Kotsila P. The rise of West Nile virus in Southern and Southeastern Europe: A spatial–temporal analysis investigating the combined effects of climate, land use and economic changes. One Health. 2021;13:100315.
Lourenço J, Barros SC, Zé-Zé L, Damineli DSC, Giovanetti M, Osório HC, et al. West Nile virus transmission potential in Portugal. Comms Biol. 2022;5(1):6.
Ewing DA, Purse BV, Cobbold CA, White SM. A novel approach for predicting risk of vector-borne disease establishment in marginal temperate environments under climate change: West Nile virus in the UK. J R Soc Interface. 2021;18(178):20210049.
Trájer AJ. Meteorological conditions associated with West Nile fever incidences in mediterranean and continental climates in Europe. Idojaras. 2017;121:303–28.
Tippelt L, Walther D, Kampen H. The thermophilic mosquito species Uranotaenia unguiculata Edwards, 1913 (Diptera: Culicidae) moves north in Germany. Parasitol Res. 2017;116:3437–40.
Farooq Z, Sjödin H, Semenza JC, Tozan Y, Sewe MO, Wallin J, Rocklöv J. European projections of West Nile virus transmission under climate change scenarios. One Health. 2023;16: 100509.
Aharonson-Raz K, Lichter-Peled A, Tal S, Gelman B, Cohen D, Klement E, et al. Spatial and temporal distribution of West Nile virus in horses in Israel (1997–2013)-From endemic to epidemics. Plos One. 2014;9(11):e113149.
Ahmadnejad F, Otarod V, Fathnia A, Ahmadabadi A, Fallah MH, Zavareh A, et al. Impact of climate and environmental factors on West Nile virus circulation in Iran. J Arthropod Borne Dis. 2016;10(3):315.
Salama M, Amitai Z, Lustig Y, Mor Z, Weiberger M, Chowers M, et al. Outbreak of West Nile virus disease in Israel (2015): A retrospective analysis of notified cases. Travel Med Infect Dis. 2018;28:41–5.
Calistri P, Ippoliti C, Candeloro L, Benjelloun A, El Harrak M, Bouchra B, et al. Analysis of climatic and environmental variables associated with the occurrence of West Nile virus in Morocco. Prev Vet Med. 2013;110(3–4):549–53.
Velu RM, Kwenda G, Bosomprah S, Chisola MN, Simunyandi M, Chisenga CC, et al. Ecological niche modeling of Aedes and Culex mosquitoes: a risk map for Chikungunya and West Nile viruses in Zambia. Viruses. 2023;15(9):1900.
Outammassine A, Zouhair S, Loqman S. Rift Valley fever and West Nile virus vectors in Morocco: Current situation and future anticipated scenarios. Transbound Emerg Dis. 2022;69(3):1466–78.
Figueroa DP, Scott S, González CR, Bizama G, Flores Mara R, Bustamante R, et al. Estimating the climate change consequences on the potential distribution of Culex pipiens L. 1758, to assess the risk of West Nile virus establishment in Chile. Gatana. 2020;84(1):46–53.
Huang B, Prow NA, van den Hurk AF, Allcock RJ, Moore PR, Doggett SL, et al. Archival isolates confirm a single topotype of West Nile virus in Australia. Plos Negl Trop Dis. 2016;10(12):e0005159.
Anyamba A, Small JL, Britch SC, Tucker CJ, Pak EW, Reynolds CA, et al. Recent weather extremes and impacts on agricultural production and vector-borne disease outbreak patterns. Plos One. 2014;9(3): e92538.
Samy AM, Elaagip AH, Kenawy MA, Ayres CF, Peterson AT, Soliman DE. Climate change influences on the global potential distribution of the mosquito Culex quinquefasciatus , vector of West Nile virus and lymphatic filariasis. Plos One. 2016;11(10): e0163863.
Negev M, Paz S, Clermont A, Pri-Or NG, Shalom U, Yeger T, et al. Impacts of climate change on vector borne diseases in the Mediterranean Basin—implications for preparedness and adaptation policy. Int J Environ Res Public Health. 2015;12(6):6745–70.
CDC. Historic Data for WNV, 1999–2022. https://www.cdc.gov/westnile/statsmaps/historic-data.html . Accessed 11 Oct 2023.
Soto RA, Hughes ML, Staples JE, Lindsey NP. West Nile virus and other domestic nationally notifiable arboviral diseases-United States, 2020. MMWR Morb Mortal Wkly Rep. 2022;71(18):628.
Zheng H, Drebot MA, Coulthart MB. West Nile virus in Canada: ever-changing, but here to stay Canada. Commun Dis Rep. 2014;40(10):173–7.
Public Health Agency of Canada. West Nile virus and other mosquito-borne disease national surveillance report. https://www.canada.ca/en/public-health/services/diseases/west-nile-virus/surveillance-west-nile-virus.html . Accessed 11 March 2024.
Young JJ, Haussig JM, Aberle SW, Pervanidou D, Riccardo F, Sekulić N, et al. Epidemiology of human West Nile virus infections in the European Union and European Union enlargement countries, 2010 to 2018. Eurosurveillance. 2021;26(19):2001095.
CDC. FAQ: general questions about West Nile virus. 2015. http://www.cdc.gov/westnile/faq/genQuestions.html . Accessed 13 Jun 2023.
Komar N. West Nile virus: epidemiology and ecology in North America. Adv Virus Res. 2003;61:185–234.
George TL, Harrigan RJ, LaManna JA, DeSante DF, Saracco JF, Smith TB. Persistent impacts of West Nile virus on North American bird populations. Proc Natl Acad Sci U S A. 2015;112(46):14290–4.
Hamer GL, Walker ED, Brawn JD, Loss SR, Ruiz MO, Goldberg TL, et al. Rapid amplification of West Nile virus: the role of hatch-year birds. Vector Borne Zoonotic Dis. 2008;8(1):57–68.
Reisen WK. Effect of temperature on Culex tarsalis (Diptera: Culicidae) from the Coachella and San Joaquin valleys of California. J Med Entomol. 1995;32:636–45.
Cotton PA. Avian migration phenology and global climate change. Proc Natl Acad Sci U S A. 2003;100:12219–22.
Caillouët KA, Riggan AE, Bulluck LP, Carlson JC, Sabo RT. Nesting bird “host funnel” increases mosquito-bird contact rate. J Med Entomol. 2013;50(2):462–6.
Shaman J, Day JF, Stieglitz M. Drought induced amplification and epidemic transmission of West Nile virus in southern Florida. J Med Entomol. 2005;42:134–41.
Paz S. Climate change impacts on West Nile virus transmission in a global context. Philos Trans R Soc Lond B Biol Sci. 2015;370(1665):20130561.
Download references
Acknowledgements
Not applicable.
This work was supported by the National Natural Science Foundation of China (No.31802217).
Author information
Authors and affiliations.
Department of Veterinary Surgery, Northeast Agricultural University, Harbin, 150030, Heilongjiang, People’s Republic of China
Hao-Ran Wang, Tao Liu, Xiang Gao, Hong-Bin Wang & Jian-Hua Xiao
Heilongjiang Key Laboratory for Laboratory Animals and Comparative Medicine, College of Veterinary Medicine, Northeast Agricultural University, Harbin, 150030, Heilongjiang, People’s Republic of China
You can also search for this author in PubMed Google Scholar
Contributions
HRW participated in the design, study selection, data extraction and analysis, and write-up of this study. TL, XG, and HBW participated in the design of this study and revised the manuscript. JHX participated in the revision of the manuscript. All authors approved the final version.
Corresponding author
Correspondence to Jian-Hua Xiao .
Ethics declarations
Ethics approval and consent to participate, consent for publication, competing interests.
The authors declare that they have no competing interests.
Supplementary Information
Supplementary material 1., supplementary material 2., rights and permissions.
Open Access This article is licensed under a Creative Commons Attribution 4.0 International License, which permits use, sharing, adaptation, distribution and reproduction in any medium or format, as long as you give appropriate credit to the original author(s) and the source, provide a link to the Creative Commons licence, and indicate if changes were made. The images or other third party material in this article are included in the article's Creative Commons licence, unless indicated otherwise in a credit line to the material. If material is not included in the article's Creative Commons licence and your intended use is not permitted by statutory regulation or exceeds the permitted use, you will need to obtain permission directly from the copyright holder. To view a copy of this licence, visit http://creativecommons.org/licenses/by/4.0/ . The Creative Commons Public Domain Dedication waiver ( http://creativecommons.org/publicdomain/zero/1.0/ ) applies to the data made available in this article, unless otherwise stated in a credit line to the data.
Reprints and permissions
About this article
Cite this article.
Wang, HR., Liu, T., Gao, X. et al. Impact of climate change on the global circulation of West Nile virus and adaptation responses: a scoping review. Infect Dis Poverty 13 , 38 (2024). https://doi.org/10.1186/s40249-024-01207-2
Download citation
Received : 03 January 2024
Accepted : 17 May 2024
Published : 24 May 2024
DOI : https://doi.org/10.1186/s40249-024-01207-2
Share this article
Anyone you share the following link with will be able to read this content:
Sorry, a shareable link is not currently available for this article.
Provided by the Springer Nature SharedIt content-sharing initiative
- Climate change
- Vector-borne pathogens
Infectious Diseases of Poverty
ISSN: 2049-9957
- Submission enquiries: Access here and click Contact Us
- General enquiries: [email protected]
Mapping Payment and Pricing Schemes for Health Innovation: Protocol of a Scoping Literature Review
- Open access
- Published: 21 May 2024
Cite this article
You have full access to this open access article
- Vittoria Ardito ORCID: orcid.org/0000-0003-2034-716X 1 ,
- Ludovico Cavallaro ORCID: orcid.org/0009-0002-3426-2570 1 ,
- Michael Drummond ORCID: orcid.org/0000-0002-6126-0944 1 , 2 &
- Oriana Ciani ORCID: orcid.org/0000-0002-3607-0508 1
280 Accesses
1 Altmetric
Explore all metrics
Introduction
Innovative pricing and payment/reimbursement schemes have been proposed as one part of the solution to the problem of patient access to new health technologies or to the uncertainty about their long-term effectiveness. As part of a Horizon Europe research project on health innovation next generation pricing and payment models (HI-PRIX), this protocol illustrates the conceptual and methodological steps related to a scoping review aiming at investigating nature and scope of pricing and payment/reimbursement schemes applied to, or proposed for, existing or new health technologies.
A scoping review of literature will be performed according to the PRISMA guidelines for scoping reviews (PRISMA-ScR) guidelines. The search will be conducted in three scientific databases (i.e., PubMed, Web of Science, and Scopus), over a 2010–2023 timeframe. The search strategy is structured around two blocks of keywords, namely “pricing and payment/reimbursement schemes,” and “innovativeness” (of the scheme type or scheme use). A simplified search will be replicated in the gray literature. Studies illustrating pricing and payment/reimbursement schemes with a sufficient level of details to explain their characteristics and functioning will be deemed eligible to be considered for data synthesis. Pricing and payment/reimbursement schemes will be classified according to several criteria, such as their purpose, nature, governance, data collection needs, and foreseen distribution of risk. The results will populate a publicly available online tool, the Pay-for-Innovation Observatory.
The findings of this review have the potential to offer a comprehensive toolkit with a variety of pricing and payment schemes to policymakers and manufacturers facing reimbursement and access decisions.
Similar content being viewed by others
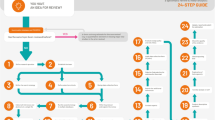
A 24-step guide on how to design, conduct, and successfully publish a systematic review and meta-analysis in medical research
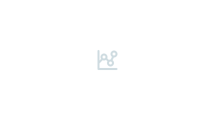
Preferred reporting items for systematic review and meta-analysis protocols (PRISMA-P) 2015 statement
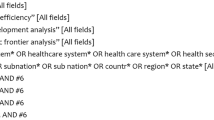
Analysing the Efficiency of Health Systems: A Systematic Review of the Literature
Avoid common mistakes on your manuscript.
1 Introduction
1.1 background.
Medical innovation is advancing rapidly, but it is often characterized by clinical and economic uncertainty at the time of entry to the health care system. For medicinal products, clinical uncertainty is linked to the fact that often pivotal studies used for marketing approval do not follow the “gold standard” [i.e., blinded, two-arm, phase III randomized controlled trials (RCTs)] [ 1 ], or rely on surrogate endpoints as predictors of clinical effectiveness [ 2 ]. Regulatory agencies such as the European Medicines Agency (EMA) and the Federal Drug Administration (FDA) are, therefore, granting marketing authorization on the basis of incomplete or limited evidence, sometimes with the commitment for the manufacturer to conduct postapproval clinical studies [ 2 , 3 , 4 , 5 ]. For medical devices (MDs), the quantity, type, and quality of evidence required for their approval has been traditionally considered to be weaker than for drugs [ 6 , 7 ]. RCTs can often be not viable for MDs, characterized by unique features such as the incremental innovation or the learning curve associated with reiterated use. Similar considerations arise for drug–device combinations, including software-incorporating devices and digital medical devices, for which the incremental improvements of the device/software components can rapidly make past RCT results outdated. This scenario is often coupled with extremely high prices of certain health innovations, often resulting in large upfront payments, that occur before accrual of any clinical benefits and generate large budgetary impacts associated with reimbursement or coverage [ 8 ]. Considered together, this situation is posing significant challenges to authorities, payers, providers, and, ultimately, patients.
On top of this, access to health innovations can be further challenged by operational complexities raised by the distinctive features of certain technologies. These characteristics include new modes of administration (e.g., single-administration therapies), high treatment personalization, the need for a highly-skilled workforce, sophistication of the logistics of treatment delivery (e.g., transportation of lab-treated specimens), and difficulties in scaling up the manufacturing capacity due to the above. Consider, for example, Advanced Therapy Medicinal Products (ATMPs), often cited as paradigmatic examples of technologies that may combine all those challenges together [ 9 , 10 ].
In this context, new pricing and payment/reimbursement models have been proposed as practical solutions to ensure timely patient access to promising innovations, while simultaneously addressing coverage problems. Innovative payment models (IPM) are agreements between manufacturers, governmental bodies, and payers defined to act as a bridge to access, reward research and Advanced therapy medicinal products: Overview.development (R&D) efforts adequately, and balance the financial sustainability of healthcare systems [ 11 ]. New payment models have been termed differently and might be referred to as risk-sharing agreements (RSA) [ 12 ], managed entry agreements (MEAs) [ 13 ], or innovative contracting [ 14 ]. They might have a wide variety of formulations, with outcome-based and/or financial-based components or with payments split over time (e.g., instalments or annuities). For instance, a taxonomy developed by Carlson and colleagues categorized performance-based reimbursement schemes in terms of timing, execution, and health outcomes, distinguishing between outcome-based versus nonoutcome-based schemes [ 15 ]. In addition, Towse et al. distinguished between the agreements that specified how evidence would be translated into revisions of price, revenues, and/or use, and those that instead specified an evidence review point where renegotiation would take place [ 12 ]. Other frameworks have focused on coverage options more generally, distinguishing between schemes with objectives of evidence generation from those of price reduction [ 16 ], on different types of performance-based RSAs [ 17 ], or on the key reasons for using MEAs [ 18 , 19 ]. More recently, Horrow and Kesselheim developed a taxonomy of possible payment arrangements for gene therapies, that include, among others, installments, subscriptions, expenditure caps, and others [ 8 ].
For clarity, we specify that this work will be focused on health technologies at large, including medicinal products, MDs, and drug–device combinations, and that these will be referred to interchangeably as “health technologies” or “health innovations.” We also specify in this context the distinction between pricing and payment/reimbursement schemes. “Pricing schemes” refer to any approach or methodology to calculate, measure, or quantify a fair price for health technologies. An example is rate of return pricing, namely a scheme in which a prespecified rate of return is ensured to manufacturers, after covering the costs of developing and marketing the product [ 20 ]. On the other hand, “payment/reimbursement schemes” or arrangements refer to formulation of any aspect that has to be defined to govern the payment of health innovations, including, but not limited to, the types and number of stakeholders involved, the moment in which the payment occurs, the split of payments over time, or the linkage to an outcome component. Examples here include the subscription model, that delinks reimbursements from volumes of sales, offering manufacturers a fixed monetary amount [ 21 ], or the conditional treatment continuation agreement, where coverage is continued only for patients who achieve a prespecified response to treatment [ 13 , 22 , 23 ]. While the two approaches might capture the same value from different perspectives (i.e., manufacturers and payers), this is not always the case.
1.2 Objectives
Given the contemporary challenges experienced by healthcare systems globally in ensuring access to the latest available health technologies, the objective of this study is to perform an extensive mapping of the pricing and payment/reimbursement schemes that are currently used, or have been proposed, to allow for timely and widespread use of potentially innovative health technologies. Specifically, a scoping literature review will be conducted to respond to the following three research objectives:
To generate a comprehensive and updated catalogue of innovative pricing and payment/reimbursement schemes for health technologies;
To develop a conceptual framework that characterizes any pricing and payment/reimbursement schemes for health technologies, ultimately contributing to cluster them through a newly defined taxonomy;
To investigate which pricing and payment/reimbursement schemes are better suited to address a given coverage or reimbursement challenge, by accounting for the distinctive features of different technology classes, therapeutic areas, settings and healthcare systems, and ultimately clarifying which scheme best serves a given policy objective.
2.1 Protocol and Registration
This protocol was developed based on the PRISMA protocol guidelines and written in accordance with the PRISMA-P statement [ 24 , 25 ]. The protocol has been registered in the International Prospective Register of Systematic Reviews (PROSPERO; registration number: CRD42023444824). The review will be conducted according to the updated methodological guidance and the PRISMA guidelines for scoping reviews (PRISMA-ScR) [ 26 , 27 ]. Scoping reviews are a type of knowledge synthesis that follow a systematic approach to map relevant concepts, theories, sources, and knowledge gaps in a given area by extensively identifying, reviewing, and synthetizing the evidence available in literature [ 28 ].
2.2 Intervention
This scoping review will be focused both on pricing and payment/reimbursement schemes for health technologies as described above. Such schemes will be investigated across several dimensions relevant to their application, including technology classes, therapeutic areas, setting of care, healthcare systems, and geographies. These dimensions are described in more detail below.
2.3 Setting
Any pricing and payment/reimbursement schemes/strategy/arrangements that are used or that have been proposed for health technologies delivered either in-hospital or outpatient settings will be included. Within this perimeter, the focus is on technologies for which a pricing arrangement has to be established and negotiated with a manufacturer (i.e., external innovation). Conversely, innovations in services originated directly by health care providers (e.g., hospital-based innovation or innovation embedded in healthcare service delivery processes) will not be considered (i.e., internal innovation).
2.4 Timeframe
The timeframe of the current study will extend from 2010 onwards. Our search started in 2010 to build on the previously conducted study by Carlson et al. published in 2010, knowing that at the same time several countries started experimenting new schemes [ 15 ]. The literature search was performed in the first quarter of 2024 and will be updated in April 2024.
2.5 Eligibility Criteria
Studies illustrating pricing and payment/reimbursement schemes of health technologies with a level of detail that is sufficient to explain their functioning across different health technologies will be deemed eligible to be included in this review. Theoretical schemes (i.e., schemes that have only been proposed) and implemented schemes (i.e., schemes that have practical applications) will be equally considered in the analyses. Pricing and payment/reimbursement schemes will not be excluded based on their perceived innovativeness, as not only the scheme per se could be innovative but also the application or use in a given context. Furthermore, no exclusions will be made based on the country of implementation of the schemes, nor on the type of study design. For this reason, editorials, commentaries, and perspectives will be included when a given scheme is proposed and discussed. Search records will be extracted with no exclusions on the publication language, but the language expertise of the research team (e.g., English or Italian) will guide the study selection.
2.6 Information Sources
Literature searches will be conducted through different sources, and both scientific and gray literature will be considered.
Scientific publications will be searched in three databases, namely PubMed (Medline), Web of Science, and Scopus. In addition, the reference list of the studies included and of the reviews identified will be scanned to ensure that no relevant important work has been missed. In case relevant papers are not retrieved by our search, it will be replicated in top-tier journals in the area of pharmaceutical policy (i.e., Journal of Pharmaceutical Policy and Practice; Expert Review of Medical Devices; Expert Review of Pharmacoeconomics and Outcome Research; Value in Health, European Journal of Health Economics; PharmacoEconomics; PharmacoEconomics—Open; Health Economics; Applied Health Economics; Health Policy; Health Affairs; Applied Health Services Research and Policy; Cost-effectiveness and Resource Allocation).
As for the gray literature, reports, white papers, and websites of a range of relevant institutions will be searched. Key institutions include, but are not limited to, international organizations, industry-oriented organizations, HTA agencies, patient associations, and consulting and research companies, such as European Commission (EC), Organization for Economic Cooperation and Development (OECD), European Federation of Pharmaceutical Industries and Associations (EFPIA), International HTA Database, Pharmaceutical Pricing and Reimbursement Information (PPRI), European Patient Forum (EPF), European Patients Academy for Therapeutic Innovation (EUPATI), and others. As a subsequent step, the list of schemes identified will be circulated to the relevant individuals in HTA bodies and other relevant institutions mentioned above, in case they are aware of any that have not been identified.
2.7 Search Strategy
The structure of the search strategy is developed around two main concepts: (1) “pricing and payment/reimbursement schemes” and (2) “innovativeness” (of the scheme type or scheme use). Particularly, it is built using combinations of the following terms: performance-based, value-based, evidence-based, risk-sharing, reimbursement, rebate, pricing, contract, scheme, guarantee, and health system. To restrict the number of retrieved records, database-specific addendums are used to filter the two main search blocks, namely Mesh Terms in PubMed (Medline), Web of Science categories in Web of Science, and index terms in Scopus. The complete search for each database is presented in Table 1 .
2.8 Study Records
2.8.1 study selection.
The records retrieved through the database search will be imported in RefWorks, a tool for reference management that is used to detect and remove duplicated studies. The final list of records will be exported into a structured Microsoft Excel spreadsheet, where they will be screened based on title and abstract, and assessed against eligibility criteria. Two members of the research team (V.A. and L.C.) will assess the first 200 records based on title and abstract, and the interrater agreement will be measured using kappa statistics [ 29 ]. The remaining papers will be first screened based on title and abstract and then read full/text by two researchers. Disagreement over final inclusions will be solved by an arbitrator (O.C.). The entire research team will read all the studies eventually included in the analysis.
2.8.2 Data Collection Process
Data collection will be performed by two independent researchers (V.A. and L.C.). Data will be extracted using an ad hoc Microsoft spreadsheet, developed by the research team after preliminarily reading a pool of seminal papers. To ensure consistency across reviewers, the extraction sheet will be tested by each reviewer and possibly recalibrated before starting the data collection process. Information on the pricing and payment/reimbursement schemes will be collected, as specified in the following section.
2.8.3 Data Extraction
Data items will be collected at the individual scheme level, although different studies may contribute to the definition of a single scheme. Data items to be extracted may include general information on the scheme, and information on one or more examples of implementation, if available, as indicated in the following Table 2 .
2.8.4 Data Synthesis
The study findings will be synthetized using narrative synthesis. Descriptive statistics on the pricing and payment/reimbursement schemes identified through this review will be provided, according to the most relevant dimensions of the data collection. Given the exploratory nature of this scoping review and the variety of the types of studies (expected to be predominantly studies with qualitative designs), a quantitative synthesis of the results will not be performed. Furthermore, given the foreseen high variety of the studies, a risk of bias assessment will not be performed. In parallel, the catalogue of pricing and payment/reimbursement schemes mapped through the review will be made accessible online to the scientific community in the form of a freely available repository called the Pay-for-Innovation Observatory, that different stakeholders could use for a variety of purposes. This broad availability of the findings of the review will also facilitate constructive comments and feedback.
2.9 Machine Learning-Powered Updates of the Scoping Review
Considering the rapidly evolving landscape of health innovations and the ensuing pricing and payment challenges, our work will be periodically updated with ASReview ( https://asreview.nl/ ), an open-source machine learning (ML) software that allows to streamline the screening process for titles and abstracts within systematic reviews. In addition to the primary search, the ML-based software will be employed to perform periodic updates of the scoping review. ASReview utilizes an active researcher-in-the-loop ML algorithm, employing text mining to rank articles in terms of their likelihood for inclusion. This approach involves prior human input from the research team to guide the ML screening process and decision. ASReview offers various classifier models to determine the relevance of included articles. In a simulation study using six comprehensive systematic review datasets covering diverse topics, it was observed that the naive Bayes (NB) and term frequency-inverse document frequency (TF-IDF) models outperformed other settings [ 30 ]. The NB classifier estimates an article’s relevance probability based on TF-IDF measurements, which gauge the uniqueness of specific words within an article relative to their frequency across all articles [ 31 ]. Consequently, the combination of NB and TF-IDF has been selected for use in our work.
The software will be trained using at least one relevant and one irrelevant article to establish a foundational knowledge base, with the expectation that performance will be enhanced as prior knowledge increases.
ASReview will conduct an initial ranking of all unlabeled articles, sorting them based on descending probabilities of relevance. The top-ranked article will undergo assessment of its title and abstract against the predetermined eligibility criteria, thereby determining its relevance. Following this assessment, the ML tool will assimilate the acquired knowledge and recalibrate the article rankings, with the subsequent highest-ranked article being presented for evaluation against the eligibility criteria. This iterative interplay between the ML tool’s ranking and the reviewers’ decision making continues until reaching a data-driven stopping criterion previously defined by the research team, i.e., the sampling criterion (which entails screening a set proportion of the highest-ranked articles) and the heuristic criterion (which prompts screening cessation upon encountering n consecutive predefined irrelevant articles).
3 Discussion
This scoping review of literature aims at investigating innovative pricing and payment/reimbursement schemes for health technologies, as well as at exploring innovative ways of using established schemes (e.g., price–volume agreements). This work will be conducted as part of the larger Horizon Europe research project Health Innovation Next Generation Payment and Pricing Model (HI-PRIX; grant agreement number 101095593), which aims at fostering access to health innovations by promoting the adoption of new pricing and payment models, in an effort to balance sustainability of health innovation with sustainability of healthcare systems. The findings of this review will be made freely accessible to the scientific community that includes governmental bodies, payers, HTA agencies, and policy makers, through an online tool, which will be termed the Pay-for-Innovation Observatory. Other databases already exist, such as the Performance Based Risk Sharing Database, proprietary of the University of Washington [ 32 ], or the repository on medical devices produced as part of the EU’s Horizon 2020 research project Pushing the Boundaries of Cost and Outcome Analysis of Medical Technologies (COMED). Our Pay-for-Innovation Observatory will build on these prior examples, expanding on the dimensions investigated (e.g., classes of health technologies covered) and making the database openly accessible.
Previously published taxonomies have classified pricing or payment/reimbursement schemes with a siloed approach, typically focusing separately on clusters of schemes, such as performance-based risk sharing agreements RSAs only [ 17 ], MEAs only [ 18 , 19 ], or coverage with evidence development (CED) schemes only. Furthermore, prior taxonomies have been predominantly developed using the lens of the public authorities or payers [ 15 , 16 , 23 ], as these mostly categorize the available coverage options as opposed to the strategies available to manufacturers to price health technologies. Lastly, these previous frameworks were published mostly in the early 2010s (i.e., the majority before 2014) and might fail at accounting for some of the innovative contracting schemes that have been designed to address the distinctive features of new health technologies, such gene therapies, that have now become available.
All in all, this work will inform on the different schemes available to promote access to potentially innovative, new or expensive health technologies in the area of medicinal products, medical devices, and drug–device combinations.
Data Availability
Data sharing is not applicable to this article as no datasets were generated or analyzed during the current study.
Code Availability
Not applicable.
Zhang AD, Puthumana J, Downing NS, Shah ND, Krumholz HM, Ross JS. Assessment of clinical trials supporting US food and drug administration approval of novel therapeutic agents, 1995–2017. JAMA Netw Open. 2020;3(4): e203284. https://doi.org/10.1001/jamanetworkopen.2020.3284 .
Article PubMed PubMed Central Google Scholar
Schuster Bruce C, Brhlikova P, Heath J, McGettigan P. The use of validated and nonvalidated surrogate endpoints in two European Medicines Agency expedited approval pathways: A cross-sectional study of products authorised 2011–2018. PLOS Med. 2019;16(9): e1002873. https://doi.org/10.1371/journal.pmed.1002873 .
Vokinger KN, Kesselheim AS, Glaus CEG, Hwang TJ. Therapeutic value of drugs granted accelerated approval or conditional marketing authorization in the US and Europe From 2007 to 2021. JAMA Health Forum. 2022;3(8): e222685. https://doi.org/10.1001/jamahealthforum.2022.2685 .
Gyawali B, Kesselheim AS, Ross JS. the accelerated approval program for cancer drugs — finding the right balance. N Engl J Med. 2023;389(11):968–71. https://doi.org/10.1056/NEJMp2306872 .
Article PubMed Google Scholar
Pease AM, Krumholz HM, Downing NS, Aminawung JA, Shah ND, Ross JS. Postapproval studies of drugs initially approved by the FDA on the basis of limited evidence: systematic review. BMJ. 2017;357: j1680. https://doi.org/10.1136/bmj.j1680 .
Drummond M, Griffin A, Tarricone R. Economic evaluation for devices and drugs—same or different? Value Health. 2009;12(4):402–4. https://doi.org/10.1111/j.1524-4733.2008.00476_1.x .
Tarricone R, et al. Lifecycle evidence requirements for high-risk implantable medical devices: a European perspective. Expert Rev Med Devices. 2020;17(10):993–1006. https://doi.org/10.1080/17434440.2020.1825074 .
Article CAS PubMed Google Scholar
Horrow C, Kesselheim AS. Confronting high costs and clinical uncertainty: innovative payment models for gene therapies: study examines costs, clinical uncertainties, and payment models for gene therapies. Health Aff (Millwood). 2023;42(11):1532–40. https://doi.org/10.1377/hlthaff.2023.00527 .
Advanced therapy medicinal products: Overview. European Medicines Agency. [Online]. https://www.ema.europa.eu/en/human-regulatory/overview/advanced-therapy-medicinal-products-overview . Accessed 10 Apr 2024.
Drummond M, et al. How are health technology assessment bodies responding to the assessment challenges posed by cell and gene therapy? BMC Health Serv Res. 2023;23(1):484. https://doi.org/10.1186/s12913-023-09494-5 .
European Commission. Directorate General for Health and Food Safety. and Expert Panel on effective ways of investing in Health (EXPH)., Opinion on innovative payment models for high-cost innovative-medicines. LU: Publications Office, 2018. Accessed: Jun. 08, 2023. [Online]. https://doi.org/10.2875/049700 .
Towse A, Garrison LP. Canʼt get no satisfaction? Will pay for performance help?: toward an economic framework for understanding performance-based risk-sharing agreements for innovative medical Products. Pharmacoeconomics. 2010;28(2):93–102. https://doi.org/10.2165/11314080-000000000-00000 .
Performance-based managed entry agreements for new medicines in OECD countries and EU member states: How they work and possible improvements going forward. OECD Health Working Papers 115. 2019. https://doi.org/10.1787/6e5e4c0f-en .
‘INNOVATIVE CONTRACTING FOR ATMPS IN EUROPE: Recent learnings from the manufacturer experience’. Alliance for Regenerative Medicine-Dolon, Aug. 2023. [Online]. https://dolon.com/wp-content/uploads/2023/08/Innovative-contracting-for-ATMPs-in-Europe-1.pdf?x23572 . Accessed 10 Apr 2024.
Carlson JJ, Sullivan SD, Garrison LP, Neumann PJ, Veenstra DL. Linking payment to health outcomes: a taxonomy and examination of performance-based reimbursement schemes between healthcare payers and manufacturers. Health Policy Amst Neth. 2010;96(3):179–90. https://doi.org/10.1016/j.healthpol.2010.02.005 .
Article Google Scholar
Walker S, Sculpher M, Claxton K, Palmer S. Coverage with evidence development, only in research, risk sharing, or patient access scheme? A framework for coverage decisions. Value Health. 2012;15(3):570–9. https://doi.org/10.1016/j.jval.2011.12.013 .
Garrison LP, et al. Performance-based risk-sharing arrangements—good practices for design, implementation, and evaluation: report of the ISPOR Good Practices for Performance-Based Risk-Sharing Arrangements Task Force. Value Health. 2013;16(5):703–19. https://doi.org/10.1016/j.jval.2013.04.011 .
Ferrario A, Kanavos P. Managed entry agreements for pharmaceuticals: The European experience. EMiNet, Brussels, 2013. [Online]. Available: http://eprints.lse.ac.uk/50513/1/__Libfile_repository_Content_Ferrario . Accessed 10 Apr 2024.
Ferrario A, Kanavos P. Dealing with uncertainty and high prices of new medicines: a comparative analysis of the use of managed entry agreements in Belgium, England, the Netherlands and Sweden. Soc Sci Med. 2015;124:39–47. https://doi.org/10.1016/j.socscimed.2014.11.003 .
Drummond M, Towse A. Is rate of return pricing a useful approach when value-based pricing is not appropriate? Eur J Health Econ. 2019;20(7):945–8. https://doi.org/10.1007/s10198-019-01032-7 .
Leonard C, et al. Can the UK “Netflix” Payment Model Boost the Antibacterial Pipeline? Appl Health Econ Health Policy. 2023;21(3):365–72. https://doi.org/10.1007/s40258-022-00786-1 .
Carlson JJ, Gries KS, Yeung K, Sullivan SD, Garrison LP. Current status and trends in performance-based risk-sharing arrangements between healthcare payers and medical product manufacturers. Appl Health Econ Health Policy. 2014;12(3):231–8. https://doi.org/10.1007/s40258-014-0093-x .
Launois R, Navarrete LF, Ethgen O, Le Moine J-G, Gatsinga R. Health economic value of an innovation: delimiting the scope and framework of future market entry agreements. J Mark Access Health Policy. 2014;2(1):24988. https://doi.org/10.3402/jmahp.v2.24988 .
Shamseer L, et al. Preferred reporting items for systematic review and meta-analysis protocols (PRISMA-P) 2015: elaboration and explanation. BMJ. 2015;349(jan021):g7647–g7647. https://doi.org/10.1136/bmj.g7647 .
PRISMA-P Group, et al. ‘Preferred reporting items for systematic review and meta-analysis protocols (PRISMA-P) 2015 statement. Syst Rev. 2015;4(1):1. https://doi.org/10.1186/2046-4053-4-1 .
Article PubMed Central Google Scholar
Peters MDJ, et al. Updated methodological guidance for the conduct of scoping reviews. JBI Evid Synth. 2020;18(10):2119–26. https://doi.org/10.11124/JBIES-20-00167 .
Tricco AC, et al. PRISMA Extension for Scoping Reviews (PRISMA-ScR): Checklist and Explanation. Ann Intern Med. 2018;169(7):467–73. https://doi.org/10.7326/M18-0850 .
Arksey H, O’Malley L. Scoping studies: towards a methodological framework. Int J Soc Res Methodol. 2005;8(1):19–32. https://doi.org/10.1080/1364557032000119616 .
Viera AJ, Garrett JM. Understanding interobserver agreement: the kappa statistic. Fam Med. 2005;37(5):360–3.
PubMed Google Scholar
Ferdinands G, et al. Active learning for screening prioritization in systematic reviews—a simulation study. Open Sci Framework. 2020. https://doi.org/10.31219/osf.io/w6qbg .
Havrlant L, Kreinovich V. A simple probabilistic explanation of term frequency-inverse document frequency (tf-idf) heuristic (and variations motivated by this explanation). Int J Gen Syst. 2017;46(1):27–36. https://doi.org/10.1080/03081079.2017.1291635 .
Performance Based Risk Sharing Database. University of Washington. [Online]. Available: https://sop.washington.edu/department-of-pharmacy/research/performance-based-risk-sharing-database/ . Accessed 8 Jan 2024
Download references
Author information
Authors and affiliations.
Center for Research on Health and Social Care Management, SDA Bocconi School of Management, Via Sarfatti, 10, 20136, Milan, MI, Italy
Vittoria Ardito, Ludovico Cavallaro, Michael Drummond & Oriana Ciani
Centre for Health Economics, University of York, York, UK
Michael Drummond
You can also search for this author in PubMed Google Scholar
Corresponding author
Correspondence to Vittoria Ardito .
Ethics declarations
This project has received funding from the European Union’s Horizon Europe research and innovation program under Grant agreement number 101095593.
Conflict of Interest
V.A., L.C., M.D., and O.C. declare that they have no conflict of interest.
Authors’ Contribution
V.A. and O.C. contributed to the study conception. V.A. drafted the first version of the manuscript. V.A. and L.C. designed the preliminary search strategy and performed the initial screening of the records. All authors (V.A., L.C., M.D., and O.C.) contributed to developing the data extraction form and revised and approved the final version of this manuscript.
Ethics Approval
Consent to participate, consent for publication (from patients/participants), rights and permissions.
Open Access This article is licensed under a Creative Commons Attribution-NonCommercial 4.0 International License, which permits any non-commercial use, sharing, adaptation, distribution and reproduction in any medium or format, as long as you give appropriate credit to the original author(s) and the source, provide a link to the Creative Commons licence, and indicate if changes were made. The images or other third party material in this article are included in the article's Creative Commons licence, unless indicated otherwise in a credit line to the material. If material is not included in the article's Creative Commons licence and your intended use is not permitted by statutory regulation or exceeds the permitted use, you will need to obtain permission directly from the copyright holder. To view a copy of this licence, visit http://creativecommons.org/licenses/by-nc/4.0/ .
Reprints and permissions
About this article
Ardito, V., Cavallaro, L., Drummond, M. et al. Mapping Payment and Pricing Schemes for Health Innovation: Protocol of a Scoping Literature Review. PharmacoEconomics Open (2024). https://doi.org/10.1007/s41669-024-00496-5
Download citation
Accepted : 06 May 2024
Published : 21 May 2024
DOI : https://doi.org/10.1007/s41669-024-00496-5
Share this article
Anyone you share the following link with will be able to read this content:
Sorry, a shareable link is not currently available for this article.
Provided by the Springer Nature SharedIt content-sharing initiative
- Find a journal
- Publish with us
- Track your research

IMAGES
VIDEO
COMMENTS
However, a review of the literature shows that for the first time the definition of Data Science appears in the book Concise Survey of Computer Methods [20], where the author defines it as "Data ...
Data science, and the related field of big data, is an emerging discipline involving the analysis of data to solve problems and develop insights. This rapidly growing domain promises many benefits to both consumers and businesses. However, the use of big data analytics can also introduce many ethical concerns, stemming from, for example, the possible loss of privacy or the harming of a sub ...
The objective of this paper is to assess and synthesize the published literature related to the application of data analytics, big data, data mining and machine learning to healthcare engineering systems.,A systematic literature review (SLR) was conducted to obtain the most relevant papers related to the research study from three different ...
The digital world has a wealth of data, such as internet of things (IoT) data, business data, health data, mobile data, urban data, security data, and many more, in the current age of the Fourth Industrial Revolution (Industry 4.0 or 4IR). Extracting knowledge or useful insights from these data can be used for smart decision-making in various applications domains. In the area of data science ...
This paper presents a literature review of Data Science for Industry 4.0, and how Open Design approaches compare to existing alternatives in industry and engineering. 2. Research Methodology The used method to extract information about the study subject was the Systematic Literature Review (SLR) which is a process that enables researchers to ...
A non-systematic review of all data science, big data in healthcare-related English language literature published in the last decade (2010-2020) was conducted in November 2020 using MEDLINE, Scopus, EMBASE, and Google Scholar. Our search strategy involved creating a search string based on a combination of keywords.
After running the literature review, the first conclusion points out the existence of a tendency of few articles published where the work profile and the career profile of a Data Scientist is established. ... The ambiguity of data science team roles and the need for a data science workforce framework, pp. 2355-2361. IEEE (2017). http ...
In this paper, we examine the literature review practices of data scientists. Data science represents a field seeing an exponential rise in papers, and increasingly drawing on and being applied in numerous diverse disciplines. Recent efforts have seen the development of several tools intended to help data scientists cope with a deluge of ...
How Data Scientists Review the Scholarly Literature. Sheshera Mysore, Mahmood Jasim, Haoru Song, Sarah Akbar, Andre Kenneth Chase Randall, Narges Mahyar. Keeping up with the research literature plays an important role in the workflow of scientists - allowing them to understand a field, formulate the problems they focus on, and develop the ...
How Data Scientists Review the Scholarly Literature CHIIR '23, March 19-23, 2023, Austin, TX, USA. aids users in making information literate decisions. Smith and Rieh [117] advocate for greater use of this knowledge context instead of their reduction and search engines "getting out of the way" of users.
This article is a practical guide to conducting data analysis in general literature reviews. The general literature review is a synthesis and analysis of published research on a relevant clinical issue, and is a common format for academic theses at the bachelor's and master's levels in nursing, physiotherapy, occupational therapy, public health and other related fields.
Data science, and the related field of big data, is an emerging discipline involving the analysis of data to solve problems and develop insights. ... What literature review is not: Diversity, boundaries and recommendations. European Journal of Information Systems, 23(3), 241-255. Google Scholar Cross Ref; Saltz, J., Dewar, N., & Heckman, R ...
Examples of literature reviews. Step 1 - Search for relevant literature. Step 2 - Evaluate and select sources. Step 3 - Identify themes, debates, and gaps. Step 4 - Outline your literature review's structure. Step 5 - Write your literature review.
A systematic literature review of data science, data analytics and machine learning applied to healthcare engineering systems. Management Decision. 2020. Shah P, Kendall F, Khozin S, Goosen R, Hu J, Laramie J, et al. Artificial intelligence and machine learning in clinical development: a translational perspective. ...
As mentioned previously, there are a number of existing guidelines for literature reviews. Depending on the methodology needed to achieve the purpose of the review, all types can be helpful and appropriate to reach a specific goal (for examples, please see Table 1).These approaches can be qualitative, quantitative, or have a mixed design depending on the phase of the review.
The best proposals are timely and clearly explain why readers should pay attention to the proposed topic. It is not enough for a review to be a summary of the latest growth in the literature: the ...
The development of data science curricula has gained attention in academia and industry. Yet, less is known about the pedagogical practices and tools employed in data science education. Through a systematic literature review, we summarize prior pedagogical practices and tools used in data science initiatives at the higher education level. Following the Technological Pedagogical Content ...
Find the right journal for your literature review using actual data; Discover literature review examples and templates; We'll also provide an overview of all the products helpful for your next narrative review, including the Web of Science, EndNote™ and Journal Citation Reports™. 1. Don't miss a paper: tips for a thorough topic search
This article presented a systematic literature review focused on Industry 4.0, data science, and time series. This work investigated the usage of data science methods and software tools in several industrial segments, taking into account the implementation of time series and the data quality employed by the authors.
Recommended literature for those looking to get started in deep…. Read more…. 71. Read writing about Literature Review in Towards Data Science. Your home for data science. A Medium publication sharing concepts, ideas and codes.
Computer Science > Databases. arXiv:2405.16033 (cs) ... View a PDF of the paper titled Wrangling Data Issues to be Wrangled: Literature Review, Taxonomy, and Industry Case Study, by Qiaolin Qin and 2 other authors. View PDF Abstract: Data quality is vital for user experience in products reliant on data. As solutions for data quality problems ...
Statistics provides the foundation for extracting meaningful insights from data. Understanding these key concepts will empower you to analyze data effectively, build robust models, and make informed decisions in the field of data science. 1. Correlation. Correlation quantifies the relationship between two variables.
Introduction to TensorFlow for Artificial Intelligence, Machine Learning, and Deep Learning. Course. Learn Data Science or improve your skills online today. Choose from a wide range of Data Science courses offered from top universities and industry leaders. Our Data Science courses are perfect for individuals or for corporate Data Science ...
There is dearth of studies on comprehensive 'Data Science' adoption as an umbrella constituting all of its components. The study conducts a "Systematic Literature Review (SLR)" on enablers and barriers affecting the implementation and success of DSS in enterprises. The SLR comprised of 113 published articles during the period 1998 and 2021.
Scholars have studied the construction of smart home care services since the 1980s, and there are some related studies at this stage. According to the literature search results of WOS of "smart home" and "elderly care" related topics shown in Figure 1, it can be found that the main research directions are sensors, ambient-assisted living (AAL), and health care.
This review synthesizes qualitative literature on chemotherapy adherence within the context of patients' experiences. Data were collected from Medline, Web of Science, CINAHL, PsychINFO, Embase, Scopus, and the Cochrane Library, systematically searched from 2006 to 2023.
sible goals of a literature review, such as summarizing prior research, examining contributions of past research or clarify - ing and/or integrating views created via previous research. As ethics within data science is such a new domain, our aim is to integrate views previously articulated, thus providing
Protocol and registration. We used a scoping review methodology to select studies for inclusion in this synthesis. Our review followed an established protocol, guided by the PRISMA Scoping Review Extension (PRISMA-SCR) and published scoping review methodology [36,37,38].It was registered with the OSF Registries (https://osf.io/9j2as) on December 25, 2023, to ensure transparency [39, 40].
Abstract. In the information era, enormous amounts of data have become available on hand to decision makers. Big data refers to datasets that are not only big, but also high in variety and velocity, which makes them difficult to handle using traditional tools and techniques. Due to the rapid growth of such data, solutions need to be studied and ...
A scoping review of literature will be performed according to the PRISMA guidelines for scoping reviews (PRISMA-ScR) guidelines. The search will be conducted in three scientific databases (i.e., PubMed, Web of Science, and Scopus), over a 2010-2023 timeframe.