- Scoping Review
- Open access
- Published: 14 November 2021

Effectiveness and safety of SARS-CoV-2 vaccine in real-world studies: a systematic review and meta-analysis
- Qiao Liu 1 na1 ,
- Chenyuan Qin 1 , 2 na1 ,
- Min Liu 1 &
- Jue Liu ORCID: orcid.org/0000-0002-1938-9365 1 , 2
Infectious Diseases of Poverty volume 10 , Article number: 132 ( 2021 ) Cite this article
58k Accesses
214 Citations
370 Altmetric
Metrics details
To date, coronavirus disease 2019 (COVID-19) becomes increasingly fierce due to the emergence of variants. Rapid herd immunity through vaccination is needed to block the mutation and prevent the emergence of variants that can completely escape the immune surveillance. We aimed to systematically evaluate the effectiveness and safety of COVID-19 vaccines in the real world and to establish a reliable evidence-based basis for the actual protective effect of the COVID-19 vaccines, especially in the ensuing waves of infections dominated by variants.
We searched PubMed, Embase and Web of Science from inception to July 22, 2021. Observational studies that examined the effectiveness and safety of SARS-CoV-2 vaccines among people vaccinated were included. Random-effects or fixed-effects models were used to estimate the pooled vaccine effectiveness (VE) and incidence rate of adverse events after vaccination, and their 95% confidence intervals ( CI ).
A total of 58 studies (32 studies for vaccine effectiveness and 26 studies for vaccine safety) were included. A single dose of vaccines was 41% (95% CI : 28–54%) effective at preventing SARS-CoV-2 infections, 52% (31–73%) for symptomatic COVID-19, 66% (50–81%) for hospitalization, 45% (42–49%) for Intensive Care Unit (ICU) admissions, and 53% (15–91%) for COVID-19-related death; and two doses were 85% (81–89%) effective at preventing SARS-CoV-2 infections, 97% (97–98%) for symptomatic COVID-19, 93% (89–96%) for hospitalization, 96% (93–98%) for ICU admissions, and 95% (92–98%) effective for COVID-19-related death, respectively. The pooled VE was 85% (80–91%) for the prevention of Alpha variant of SARS-CoV-2 infections, 75% (71–79%) for the Beta variant, 54% (35–74%) for the Gamma variant, and 74% (62–85%) for the Delta variant. The overall pooled incidence rate was 1.5% (1.4–1.6%) for adverse events, 0.4 (0.2–0.5) per 10 000 for severe adverse events, and 0.1 (0.1–0.2) per 10 000 for death after vaccination.
Conclusions
SARS-CoV-2 vaccines have reassuring safety and could effectively reduce the death, severe cases, symptomatic cases, and infections resulting from SARS-CoV-2 across the world. In the context of global pandemic and the continuous emergence of SARS-CoV-2 variants, accelerating vaccination and improving vaccination coverage is still the most important and urgent matter, and it is also the final means to end the pandemic.
Graphical Abstract
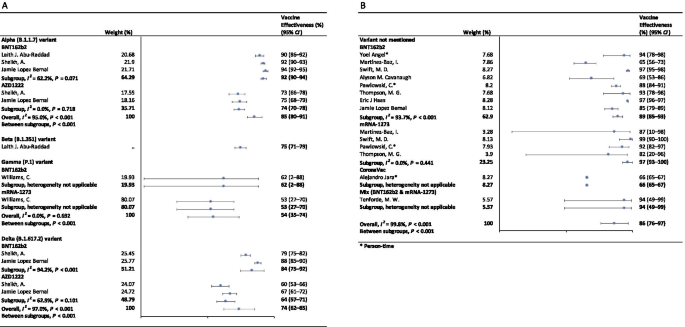
Since its outbreak, coronavirus disease 2019 (COVID-19) has spread rapidly, with a sharp rise in the accumulative number of infections worldwide. As of August 8, 2021, COVID-19 has already killed more than 4.2 million people and more than 203 million people were infected [ 1 ]. Given its alarming-spreading speed and the high cost of completely relying on non-pharmaceutical measures, we urgently need safe and effective vaccines to cover susceptible populations and restore people’s lives into the original [ 2 ].
According to global statistics, as of August 2, 2021, there are 326 candidate vaccines, 103 of which are in clinical trials, and 19 vaccines have been put into normal use, including 8 inactivated vaccines and 5 protein subunit vaccines, 2 RNA vaccines, as well as 4 non-replicating viral vector vaccines [ 3 ]. Our World in Data simultaneously reported that 27.3% of the world population has received at least one dose of a COVID-19 vaccine, and 13.8% is fully vaccinated [ 4 ].
To date, COVID-19 become increasingly fierce due to the emergence of variants [ 5 , 6 , 7 ]. Rapid herd immunity through vaccination is needed to block the mutation and prevent the emergence of variants that can completely escape the immune surveillance [ 6 , 8 ]. Several reviews systematically evaluated the effectiveness and/or safety of the three mainstream vaccines on the market (inactivated virus vaccines, RNA vaccines and viral vector vaccines) based on random clinical trials (RCT) yet [ 9 , 10 , 11 , 12 , 13 ].
In general, RNA vaccines are the most effective, followed by viral vector vaccines and inactivated virus vaccines [ 10 , 11 , 12 , 13 ]. The current safety of COVID-19 vaccines is acceptable for mass vaccination, but long-term monitoring of vaccine safety is needed, especially in older people with underlying conditions [ 9 , 10 , 11 , 12 , 13 ]. Inactivated vaccines had the lowest incidence of adverse events and the safety comparisons between mRNA vaccines and viral vectors were controversial [ 9 , 10 ].
RCTs usually conduct under a very demanding research circumstance, and tend to be highly consistent and limited in terms of population characteristics and experimental conditions. Actually, real-world studies differ significantly from RCTs in terms of study conditions and mass vaccination in real world requires taking into account factors, which are far more complex, such as widely heterogeneous populations, vaccine supply, willingness, medical accessibility, etc. Therefore, the real safety and effectiveness of vaccines turn out to be a major concern of international community. The results of a mass vaccination of CoronaVac in Chile demonstrated a protective effectiveness of 65.9% against the onset of COVID-19 after complete vaccination procedures [ 14 ], while the outcomes of phase 3 trials in Brazil and Turkey were 50.7% and 91.3%, reported on Sinovac’s website [ 14 ]. As for the Delta variant, the British claimed 88% protection after two doses of BNT162b2, compared with 67% for AZD1222 [ 15 ]. What is surprising is that the protection of BNT162b2 against infection in Israel is only 39% [ 16 ]. Several studies reported the effectiveness and safety of the COVID-19 vaccine in the real world recently, but the results remain controversial [ 17 , 18 , 19 , 20 ]. A comprehensive meta-analysis based upon the real-world studies is still in an urgent demand, especially for evaluating the effect of vaccines on variation strains. In the present study, we aimed to systematically evaluate the effectiveness and safety of the COVID-19 vaccine in the real world and to establish a reliable evidence-based basis for the actual protective effect of the COVID-19 vaccines, especially in the ensuing waves of infections dominated by variants.
Search strategy and selection criteria
Our methods were described in detail in our published protocol [PROSPERO (Prospective register of systematic reviews) registration, CRD42021267110]. We searched eligible studies published by 22 July 2021, from three databases including PubMed, Embase and Web of Science by the following search terms: (effectiveness OR safety) AND (COVID-19 OR coronavirus OR SARS-CoV-2) AND (vaccine OR vaccination). We used EndNoteX9.0 (Thomson ResearchSoft, Stanford, USA) to manage records, screen and exclude duplicates. This study was strictly performed according to the Preferred Reporting Items for Systematic Reviews and Meta-Analyses (PRISMA).
We included observational studies that examined the effectiveness and safety of severe acute respiratory syndrome coronavirus 2 (SARS-CoV-2) vaccines among people vaccinated with SARS-CoV-2 vaccines. The following studies were excluded: (1) irrelevant to the subject of the meta-analysis, such as studies that did not use SARS-CoV-2 vaccination as the exposure; (2) insufficient data to calculate the rate for the prevention of COVID-19, the prevention of hospitalization, the prevention of admission to the ICU, the prevention of COVID-19-related death, or adverse events after vaccination; (3) duplicate studies or overlapping participants; (4) RCT studies, reviews, editorials, conference papers, case reports or animal experiments; and (5) studies that did not clarify the identification of COVID-19.
Studies were identified by two investigators (LQ and QCY) independently following the criteria above, while discrepancies reconciled by a third investigator (LJ).
Data extraction and quality assessment
The primary outcome was the effectiveness of SARS-CoV-2 vaccines. The following data were extracted independently by two investigators (LQ and QCY) from the selected studies: (1) basic information of the studies, including first author, publication year and study design; (2) characteristics of the study population, including sample sizes, age groups, setting or locations; (3) kinds of the SARS-CoV-2 vaccines; (4) outcomes for the effectiveness of SARS-CoV-2 vaccines: the number of laboratory-confirmed COVID-19, hospitalization for COVID-19, admission to the ICU for COVID-19, and COVID-19-related death; and (5) outcomes for the safety of SARS-CoV-2 vaccines: the number of adverse events after vaccination.
We evaluated the risk of bias using the Newcastle–Ottawa quality assessment scale for cohort studies and case–control studies [ 21 ]. and assess the methodological quality using the checklist recommended by Agency for Healthcare Research and Quality (AHRQ) [ 22 ]. Cohort studies and case–control studies were classified as having low (≥ 7 stars), moderate (5–6 stars), and high risk of bias (≤ 4 stars) with an overall quality score of 9 stars. For cross-sectional studies, we assigned each item of the AHRQ checklist a score of 1 (answered “yes”) or 0 (answered “no” or “unclear”), and summarized scores across items to generate an overall quality score that ranged from 0 to 11. Low, moderate, and high risk of bias were identified as having a score of 8–11, 4–7 and 0–3, respectively.
Two investigators (LQ and QCY) independently assessed study quality, with disagreements resolved by a third investigator (LJ).
Data synthesis and statistical analysis
We performed a meta-analysis to pool data from included studies and assess the effectiveness and safety of SARS-CoV-2 vaccines by clinical outcomes (rates of the prevention of COVID-19, the prevention of hospitalization, the prevention of admission to the ICU, the prevention of COVID-19-related death, and adverse events after vaccination). Random-effects or fixed-effects models were used to pool the rates and adjusted estimates across studies separately, based on the heterogeneity between estimates ( I 2 ). Fixed-effects models were used if I 2 ≤ 50%, which represented low to moderate heterogeneity and random-effects models were used if I 2 > 50%, representing substantial heterogeneity.
We conducted subgroup analyses to investigate the possible sources of heterogeneity by using vaccine kinds, vaccination status, sample size, and study population as grouping variables. We used the Q test to conduct subgroup comparisons and variables were considered significant between subgroups if the subgroup difference P value was less than 0.05. Publication bias was assessed by funnel plot and Egger’s regression test. We analyzed data using Stata version 16.0 (StataCorp, Texas, USA).
A total of 4844 records were searched from the three databases. 2484 duplicates were excluded. After reading titles and abstracts, we excluded 2264 reviews, RCT studies, duplicates and other studies meeting our exclude criteria. Among the 96 studies under full-text review, 41 studies were excluded (Fig. 1 ). Ultimately, with three grey literatures included, this final meta-analysis comprised 58 eligible studies, including 32 studies [ 14 , 15 , 17 , 18 , 19 , 20 , 23 , 24 , 25 , 26 , 27 , 28 , 29 , 30 , 31 , 32 , 33 , 34 , 35 , 36 , 37 , 38 , 39 , 40 , 41 , 42 , 43 , 44 , 45 , 46 , 47 , 48 ] for vaccine effectiveness and 26 studies [ 49 , 50 , 51 , 52 , 53 , 54 , 55 , 56 , 57 , 58 , 59 , 60 , 61 , 62 , 63 , 64 , 65 , 66 , 67 , 68 , 69 , 70 , 71 , 72 , 73 , 74 ] for vaccine safety. Characteristics of included studies are showed in Additional file 1 : Table S1, Additional file 2 : Table S2. The risk of bias of all studies we included was moderate or low.
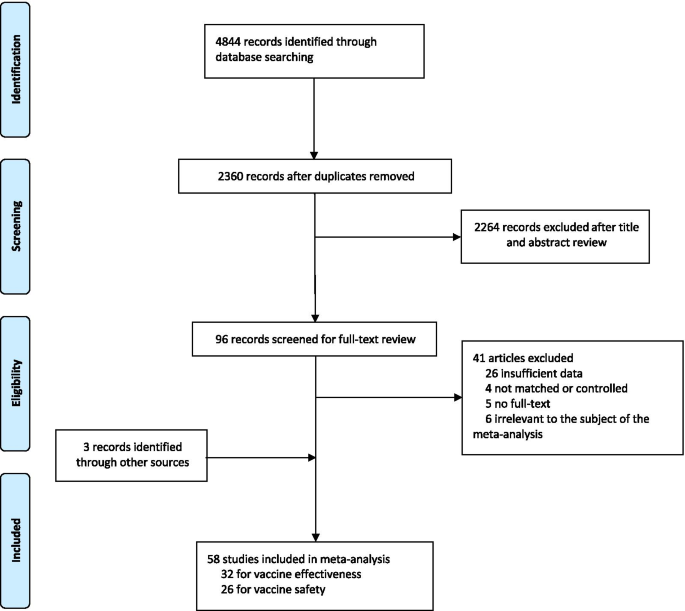
Flowchart of the study selection
Vaccine effectiveness for different clinical outcomes of COVID-19
We separately reported the vaccine effectiveness (VE) by the first and second dose of vaccines, and conducted subgroup analysis by the days after the first or second dose (< 7 days, ≥ 7 days, ≥ 14 days, and ≥ 21 days; studies with no specific days were classified as 1 dose, 2 dose or ≥ 1 dose).
For the first dose of SARS-CoV-2 vaccines, the pooled VE was 41% (95% CI : 28–54%) for the prevention of SARS-CoV-2 infection, 52% (95% CI : 31–73%) for the prevention of symptomatic COVID-19, 66% (95% CI : 50–81%) for the prevention of hospital admissions, 45% (95% CI : 42–49%) for the prevention of ICU admissions, and 53% (95% CI : 15–91%) for the prevention of COVID-19-related death (Table 1 ). The subgroup, ≥ 21 days after the first dose, was found to have the highest VE in each clinical outcome of COVID-19, regardless of ≥ 1 dose group (Table 1 ).
For the second dose of SARS-CoV-2 vaccines, the pooled VE was 85% (95% CI : 81–89%) for the prevention of SARS-CoV-2 infection, 97% (95% CI : 97–98%) for the prevention of symptomatic COVID-19, 93% (95% CI: 89–96%) for the prevention of hospital admissions, 96% (95% CI : 93–98%) for the prevention of ICU admissions, and 95% (95% CI : 92–98%) for the prevention of COVID-19-related death (Table 1 ). VE was 94% (95% CI : 78–98%) in ≥ 21 days after the second dose for the prevention of SARS-CoV-2 infection, higher than other subgroups, regardless of 2 dose group (Table 1 ). For the prevention of symptomatic COVID-19, VE was also relatively higher in 21 days after the second dose (99%, 95% CI : 94–100%). Subgroups showed no statistically significant differences in the prevention of hospital admissions, ICU admissions and COVID-19-related death (subgroup difference P values were 0.991, 0.414, and 0.851, respectively).
Vaccine effectiveness for different variants of SARS-CoV-2 in fully vaccinated people
In the fully vaccinated groups (over 14 days after the second dose), the pooled VE was 85% (95% CI: 80–91%) for the prevention of Alpha variant of SARS-CoV-2 infection, 54% (95% CI : 35–74%) for the Gamma variant, and 74% (95% CI : 62–85%) for the Delta variant. There was only one study [ 23 ] focused on the Beta variant, which showed the VE was 75% (95% CI : 71–79%) for the prevention of the Beta variant of SARS-CoV-2 infection. BNT162b2 vaccine had the highest VE in each variant group; 92% (95% CI : 90–94%) for the Alpha variant, 62% (95% CI : 2–88%) for the Gamma variant, and 84% (95% CI : 75–92%) for the Delta variant (Fig. 2 ).
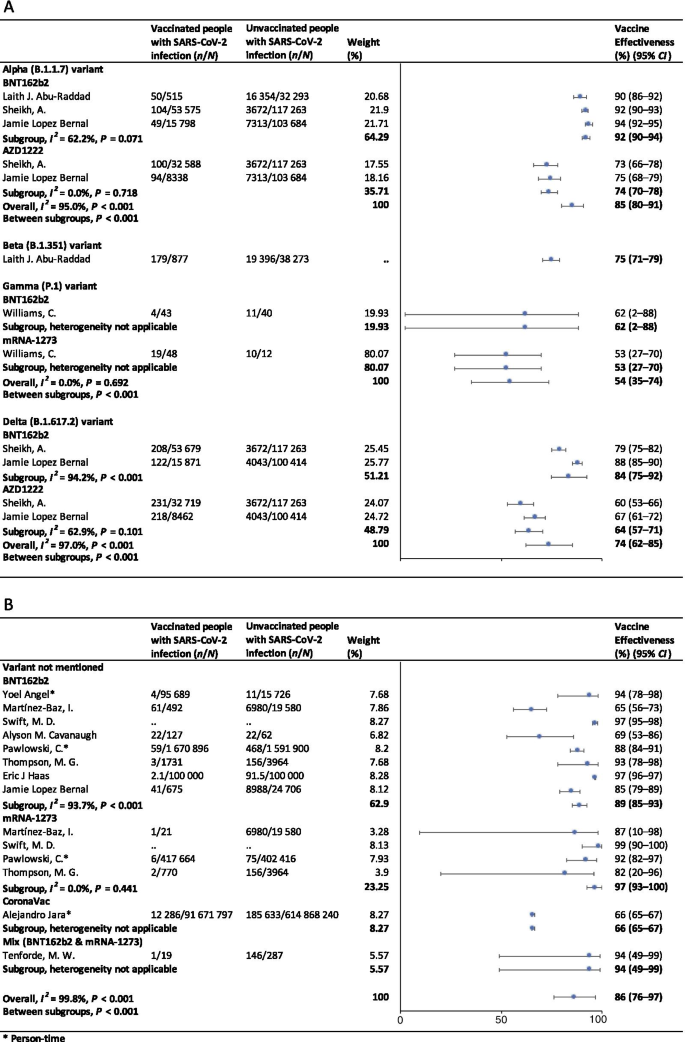
Forest plots for the vaccine effectiveness of SARS-CoV-2 vaccines in fully vaccinated populations. A Vaccine effectiveness against SARS-CoV-2 variants; B Vaccine effectiveness against SARS-CoV-2 with variants not mentioned. SARS-CoV-2 severe acute respiratory syndrome coronavirus 2, COVID-19 coronavirus disease 2019, CI confidence interval
For studies which had not mentioned the variant of SARS-CoV-2, the pooled VE was 86% (95% CI: 76–97%) for the prevention of SARS-CoV-2 infection in fully vaccinated people. mRNA-1273 vaccine had the highest pooled VE (97%, 95% CI: 93–100%, Fig. 2 ).
Safety of SARS-CoV-2 vaccines
As Table 2 showed, the incidence rate of adverse events varied widely among different studies. We conducted subgroup analysis by study population (general population, patients and healthcare workers), vaccine type (BNT162b2, mRNA-1273, CoronaVac, and et al.), and population size (< 1000, 1000–10 000, 10 000–100 000, and > 100 000). The overall pooled incidence rate was 1.5% (95% CI : 1.4–1.6%) for adverse events, 0.4 (95% CI : 0.2–0.5) per 10 000 for severe adverse events, and 0.1 (95% CI : 0.1–0.2) per 10 000 for death after vaccination. Incidence rate of adverse events was higher in healthcare workers (53.2%, 95% CI : 28.4–77.9%), AZD1222 vaccine group (79.6%, 95% CI : 60.8–98.3%), and < 1000 population size group (57.6%, 95% CI : 47.9–67.4%). Incidence rate of sever adverse events was higher in healthcare workers (127.2, 95% CI : 62.7–191.8, per 10 000), Gam-COVID-Vac vaccine group (175.7, 95% CI : 77.2–274.2, per 10 000), and 1000–10 000 population size group (336.6, 95% CI : 41.4–631.8, per 10 000). Incidence rate of death after vaccination was higher in patients (7.6, 95% CI : 0.0–32.2, per 10 000), BNT162b2 vaccine group (29.8, 95% CI : 0.0–71.2, per 10 000), and < 1000 population size group (29.8, 95% CI : 0.0–71.2, per 10 000). Subgroups of general population, vaccine type not mentioned, and > 100 000 population size had the lowest incidence rate of adverse events, severe adverse events, and death after vaccination.
Sensitivity analysis and publication bias
In the sensitivity analyses, VE for SARS-CoV-2 infections, symptomatic COVID-19 and COVID-19-related death got relatively lower when omitting over a single dose group of Maria et al.’s work [ 33 ]; when omitting ≥ 14 days after the first dose group and ≥ 14 days after the second dose group of Alejandro et al.’s work [ 14 ], VE for SARS-CoV-2 infections, hospitalization, ICU admission and COVID-19-related death got relatively higher; and VE for all clinical status of COVID-19 became lower when omitting ≥ 14 days after the second dose group of Eric et al.’s work [ 34 ]. Incidence rate of adverse events and severe adverse events got relatively higher when omitting China CDC’s data [ 74 ]. P values of Egger’s regression test for all the meta-analysis were more than 0.05, indicating that there might not be publication bias.
To our knowledge, this is a comprehensive systematic review and meta-analysis assessing the effectiveness and safety of SARS-CoV-2 vaccines based on real-world studies, reporting pooled VE for different variants of SARS-CoV-2 and incidence rate of adverse events. This meta-analysis comprised a total of 58 studies, including 32 studies for vaccine effectiveness and 26 studies for vaccine safety. We found that a single dose of SARS-CoV-2 vaccines was about 40–60% effective at preventing any clinical status of COVID-19 and that two doses were 85% or more effective. Although vaccines were not as effective against variants of SARS-CoV-2 as original virus, the vaccine effectiveness was still over 50% for fully vaccinated people. Normal adverse events were common, while the incidence of severe adverse events or even death was very low, providing reassurance to health care providers and to vaccine recipients and promote confidence in the safety of COVID-19 vaccines. Our findings strengthen and augment evidence from previous review [ 75 ], which confirmed the effectiveness of the BNT162b2 mRNA vaccine, and additionally reported the safety of SARS-CoV-2 vaccines, giving insight on the future of SARS-CoV-2 vaccine schedules.
Although most vaccines for the prevention of COVID-19 are two-dose vaccines, we found that the pooled VE of a single dose of SARS-CoV-2 vaccines was about 50%. Recent study showed that the T cell and antibody responses induced by a single dose of the BNT162b2 vaccine were comparable to those naturally infected with SARE-CoV-2 within weeks or months after infection [ 76 ]. Our findings could help to develop vaccination strategies under certain circumstances such as countries having a shortage of vaccines. In some countries, in order to administer the first dose to a larger population, the second dose was delayed for up to 12 weeks [ 77 ]. Some countries such as Canada had even decided to delay the second dose for 16 weeks [ 78 ]. However, due to a suboptimum immune response in those receiving only a single dose of a vaccine, such an approach had a chance to give rise to the emergence of variants of SARS-CoV-2 [ 79 ]. There remains a need for large clinical trials to assess the efficacy of a single-dose administration of two-dose vaccines and the risk of increasing the emergence of variants.
Two doses of SARS-CoV-2 vaccines were highly effective at preventing hospitalization, severe cases and deaths resulting from COVID-19, while the VE of different groups of days from the second vaccine dose showed no statistically significant differences. Our findings emphasized the importance of getting fully vaccinated, for the fact that most breakthrough infections were mild or asymptomatic. A recent study showed that the occurrence of breakthrough infections with SARS-CoV-2 in fully vaccinated populations was predictable with neutralizing antibody titers during the peri-infection period [ 80 ]. We also found getting fully vaccinated was at least 50% effective at preventing SARS-CoV-2 variants infections, despite reduced effectiveness compared with original virus; and BNT162b2 vaccine was found to have the highest VE in each variant group. Studies showed that the highly mutated variants were indicative of a form of rapid, multistage evolutionary jumps, which could preferentially occur in the milieu of partial immune control [ 81 , 82 ]. Therefore, immunocompromised patients should be prioritized for anti-COVID-19 immunization to mitigate persistent SARS-CoV-2 infections, during which multimutational SARS-CoV-2 variants could arise [ 83 ].
Recently, many countries, including Israel, the United States, China and the United Kingdom, have introduced a booster of COVID-19 vaccine, namely the third dose [ 84 , 85 , 86 , 87 ]. A study of Israel showed that among people vaccinated with BNT162b2 vaccine over 60 years, the risk of COVID-19 infection and severe illness in the non-booster group was 11.3 times (95% CI: 10.4–12.3) and 19.5 times (95% CI: 12.9–29.5) than the booster group, respectively [ 84 ]. Some studies have found that the third dose of Moderna, Pfizer-BioNTech, Oxford-AstraZeneca and Sinovac produced a spike in infection-blocking neutralizing antibodies when given a few months after the second dose [ 85 , 87 , 88 ]. In addition, the common adverse events associated with the third dose did not differ significantly from the symptoms of the first two doses, ranging from mild to moderate [ 85 ]. The overall incidence rate of local and systemic adverse events was 69% (57/97) and 20% (19/97) after receiving the third dose of BNT162b2 vaccine, respectively [ 88 ]. Results of a phase 3 clinical trial involving 306 people aged 18–55 years showed that adverse events after receiving a third dose of BNT162b2 vaccine (5–8 months after completion of two doses) were similar to those reported after receiving a second dose [ 85 ]. Based on V-safe, local reactions were more frequently after dose 3 (5323/6283; 84.7%) than dose 2 (5249/6283; 83.5%) among people who received 3 doses of Moderna. Systemic reactions were reported less frequently after dose 3 (4963/6283; 79.0%) than dose 2 (5105/6283; 81.3%) [ 86 ]. On August 4, WHO called for a halt to booster shots until at least the end of September to achieve an even distribution of the vaccine [ 89 ]. At this stage, the most important thing we should be thinking about is how to reach a global cover of people at risk with the first or second dose, rather than focusing on the third dose.
Based on real world studies, our results preliminarily showed that complete inoculation of COVID-19 vaccines was still effective against infection of variants, although the VE was generally diminished compared with the original virus. Particularly, the pooled VE was 54% (95% CI : 35–74%) for the Gamma variant, and 74% (95% CI : 62–85%) for the Delta variant. Since the wide spread of COVID-19, a number of variants have drawn extensive attention of international community, including Alpha variant (B.1.1.7), first identified in the United Kingdom; Beta variant (B.1.351) in South Africa; Gamma variant (P.1), initially appeared in Brazil; and the most infectious one to date, Delta variant (B.1.617.2) [ 90 ]. Israel recently reported a breakthrough infection of SARS-CoV-2, dominated by variant B.1.1.7 in a small number of fully vaccinated health care workers, raising concerns about the effectiveness of the original vaccine against those variants [ 80 ]. According to an observational cohort study in Qatar, VE of the BNT162b2 vaccine against the Alpha (B.1.1.7) and Beta (B.1.351) variants was 87% (95% CI : 81.8–90.7%) and 75.0% (95% CI : 70.5–7.9%), respectively [ 23 ]. Based on the National Immunization Management System of England, results from a recent real-world study of all the general population showed that the AZD1222 and BNT162b2 vaccines protected against symptomatic SARS-CoV-2 infection of Alpha variant with 74.5% (95% CI : 68.4–79.4%) and 93.7% (95% CI : 91.6–95.3%) [ 15 ]. In contrast, the VE against the Delta variant was 67.0% (95% CI : 61.3–71.8%) for two doses of AZD1222 vaccine and 88% (95% CI : 85.3–90.1%) for BNT162b2 vaccine [ 15 ].
In terms of adverse events after vaccination, the pooled incidence rate was very low, only 1.5% (95% CI : 1.4–1.6%). However, the prevalence of adverse events reported in large population (population size > 100 000) was much lower than that in small to medium population size. On the one hand, the vaccination population in the small to medium scale studies we included were mostly composed by health care workers, patients with specific diseases or the elderly. And these people are more concerned about their health and more sensitive to changes of themselves. But it remains to be proved whether patients or the elderly are more likely to have adverse events than the general. Mainstream vaccines currently on the market have maintained robust safety in specific populations such as cancer patients, organ transplant recipients, patients with rheumatic and musculoskeletal diseases, pregnant women and the elderly [ 54 , 91 , 92 , 93 , 94 ]. A prospective study by Tal Goshen-lag suggests that the safety of BNT162b2 vaccine in cancer patients is consistent with those previous reports [ 91 ]. In addition, the incidence rate of adverse events reported in the heart–lung transplant population is even lower than that in general population [ 95 ]. On the other hand, large scale studies at the national level are mostly based on national electronic health records or adverse event reporting systems, and it is likely that most mild or moderate symptoms are actually not reported.
Compared with the usual local adverse events (such as pain at the injection site, redness at the injection site, etc.) and normal systemic reactions (such as fatigue, myalgia, etc.), serious and life-threatening adverse events were rare due to our results. A meta-analysis based on RCTs only showed three cases of anaphylactic shock among 58 889 COVID-19 vaccine recipients and one in the placebo group [ 11 ]. The exact mechanisms underlying most of the adverse events are still unclear, accordingly we cannot establish a causal relation between severe adverse events and vaccination directly based on observational studies. In general, varying degrees of adverse events occur after different types of COVID-19 vaccination. Nevertheless, the benefits far outweigh the risks.
Our results showed the effectiveness and safety of different types of vaccines varied greatly. Regardless of SARS-CoV-2 variants, vaccine effectiveness varied from 66% (CoronaVac [ 14 ]) to 97% (mRNA-1273 [ 18 , 20 , 45 , 46 ]). The incidence rate of adverse events varied widely among different types of vaccines, which, however, could be explained by the sample size and population group of participants. BNT162b2, AZD1222, mRNA-1273 and CoronaVac were all found to have high vaccine efficacy and acceptable adverse-event profile in recent published studies [ 96 , 97 , 98 , 99 ]. A meta-analysis, focusing on the potential vaccine candidate which have reached to the phase 3 of clinical development, also found that although many of the vaccines caused more adverse events than the controls, most were mild, transient and manageable [ 100 ]. However, severe adverse events did occur, and there remains the need to implement a unified global surveillance system to monitor the adverse events of COVID-19 vaccines around the world [ 101 ]. A recent study employed a knowledge-based or rational strategy to perform a prioritization matrix of approved COVID-19 vaccines, and led to a scale with JANSSEN (Ad26.COV2.S) in the first place, and AZD1222, BNT162b2, and Sputnik V in second place, followed by BBIBP-CorV, CoronaVac and mRNA-1273 in third place [ 101 ]. Moreover, when deciding the priority of vaccines, the socioeconomic characteristics of each country should also be considered.
Our meta-analysis still has several limitations. First, we may include limited basic data on specific populations, as vaccination is slowly being promoted in populations under the age of 18 or over 60. Second, due to the limitation of the original real-world study, we did not conduct subgroup analysis based on more population characteristics, such as age. When analyzing the efficacy and safety of COVID-19 vaccine, we may have neglected the discussion on the heterogeneity from these sources. Third, most of the original studies only collected adverse events within 7 days after vaccination, which may limit the duration of follow-up for safety analysis.
Based on the real-world studies, SARS-CoV-2 vaccines have reassuring safety and could effectively reduce the death, severe cases, symptomatic cases, and infections resulting from SARS-CoV-2 across the world. In the context of global pandemic and the continuous emergence of SARS-CoV-2 variants, accelerating vaccination and improving vaccination coverage is still the most important and urgent matter, and it is also the final means to end the pandemic.
Availability of data and materials
All data generated or analyzed during this study are included in this published article and its additional information files.
Abbreviations
Coronavirus disease 2019
Severe Acute Respiratory Syndrome Coronavirus 2
Vaccine effectiveness
Confidence intervals
Intensive care unit
Random clinical trials
Preferred reporting items for systematic reviews and meta-analyses
COVID-19 Dashboard by the Center for Systems Science and Engineering (CSSE) at Johns Hopkins University (JHU). 2021. https://coronavirus.jhu.edu/map.html . Accessed 20 Aug 2021.
Barranco R, Rocca G, Molinelli A, Ventura F. Controversies and challenges of mass vaccination against SARS-CoV-2 in Italy: medico-legal perspectives and considerations. Healthcare (Basel). 2021. https://doi.org/10.3390/healthcare9091163 .
Article Google Scholar
COVID-19 vaccine tracker. 2021. https://vac-lshtm.shinyapps.io/ncov_vaccine_landscape/ . Accessed 20 Aug 2021.
Coronavirus (COVID-19) Vaccinations. 2021. https://ourworldindata.org/covid-vaccinations . Accessed 20 Aug 2021.
Kirby T. New variant of SARS-CoV-2 in UK causes surge of COVID-19. Lancet Respir Med. 2021;9(2):e20–1. https://doi.org/10.1016/s2213-2600(21)00005-9 .
Article CAS PubMed PubMed Central Google Scholar
Callaway E. Fast-spreading COVID variant can elude immune responses. Nature. 2021;589(7843):500–1. https://doi.org/10.1038/d41586-021-00121-z .
Article CAS PubMed Google Scholar
Reardon S. How the Delta variant achieves its ultrafast spread. Nature. 2021. https://doi.org/10.1038/d41586-021-01986-w .
Article PubMed Google Scholar
Li R, Liu J, Zhang H. The challenge of emerging SARS-CoV-2 mutants to vaccine development. J Genet Genomics. 2021;48(2):102–6. https://doi.org/10.1016/j.jgg.2021.03.001 .
Article PubMed PubMed Central Google Scholar
Chen M, Yuan Y, Zhou Y, Deng Z, Zhao J, Feng F, Zou H, Sun C. Safety of SARS-CoV-2 vaccines: a systematic review and meta-analysis of randomized controlled trials. Infect Dis Poverty. 2021;10(1):94. https://doi.org/10.1186/s40249-021-00878-5 .
Ling Y, Zhong J, Luo J. Safety and effectiveness of SARS-CoV-2 vaccines: a systematic review and meta-analysis. J Med Virol. 2021. https://doi.org/10.1002/jmv.27203 .
Pormohammad A, Zarei M, Ghorbani S, Mohammadi M, Razizadeh MH, Turner DL, Turner RJ. Efficacy and safety of COVID-19 vaccines: a systematic review and meta-analysis of randomized clinical trials. Vaccines (Basel). 2021. https://doi.org/10.3390/vaccines9050467 .
Sathian B, Asim M, Banerjee I, Roy B, Pizarro AB, Mancha MA, van Teijlingen ER, Kord-Varkaneh H, Mekkodathil AA, Subramanya SH, et al. Development and implementation of a potential coronavirus disease 2019 (COVID-19) vaccine: a systematic review and meta-analysis of vaccine clinical trials. Nepal J Epidemiol. 2021;11(1):959–82. https://doi.org/10.3126/nje.v11i1.36163 .
Yuan P, Ai P, Liu Y, Ai Z, Wang Y, Cao W, Xia X, Zheng JC. Safety, tolerability, and immunogenicity of COVID-19 vaccines: a systematic review and meta-analysis. medRxiv. 2020. https://doi.org/10.1101/2020.11.03.20224998 .
Jara A, Undurraga EA, González C, Paredes F, Fontecilla T, Jara G, Pizarro A, Acevedo J, Leo K, Leon F, et al. Effectiveness of an inactivated SARS-CoV-2 vaccine in Chile. N Engl J Med. 2021. https://doi.org/10.1056/NEJMoa2107715 .
Lopez Bernal J, Andrews N, Gower C, Gallagher E, Simmons R, Thelwall S, Stowe J, Tessier E, Groves N, Dabrera G, et al. Effectiveness of COVID-19 vaccines against the B.1.617.2 (Delta) variant. N Engl J Med. 2021. https://doi.org/10.1056/NEJMoa2108891 .
Israel says Pfizer Covid vaccine is just 39% effective as delta spreads, but still prevents severe illness. 2021. https://www.cnbc.com/2021/07/23/delta-variant-pfizer-covid-vaccine-39percent-effective-in-israel-prevents-severe-illness.html . Accessed 20 Aug 2021.
Zacay G, Shasha D, Bareket R, Kadim I, Hershkowitz Sikron F, Tsamir J, Mossinson D, Heymann AD. BNT162b2 vaccine effectiveness in preventing asymptomatic infection with SARS-CoV-2 virus: a nationwide historical cohort study. Open Forum Infect Dis. 2021;8(6): ofab262. https://doi.org/10.1093/ofid/ofab262 .
Martínez-Baz I, Miqueleiz A, Casado I, Navascués A, Trobajo-Sanmartín C, Burgui C, Guevara M, Ezpeleta C, Castilla J. Effectiveness of COVID-19 vaccines in preventing SARS-CoV-2 infection and hospitalisation, Navarre, Spain, January to April 2021. Eurosurveillance. 2021. https://doi.org/10.2807/1560-7917.Es.2021.26.21.2100438 .
Tenforde MW, Olson SM, Self WH, Talbot HK, Lindsell CJ, Steingrub JS, Shapiro NI, Ginde AA, Douin DJ, Prekker ME, et al. Effectiveness of Pfizer-BioNTech and moderna vaccines against COVID-19 among hospitalized adults aged ≥65 years—United States, January–March 2021. MMWR Morb Mortal Wkly Rep. 2021;70(18):674–9. https://doi.org/10.15585/mmwr.mm7018e1 .
Pawlowski C, Lenehan P, Puranik A, Agarwal V, Venkatakrishnan AJ, Niesen MJM, O’Horo JC, Virk A, Swift MD, Badley AD, et al. FDA-authorized mRNA COVID-19 vaccines are effective per real-world evidence synthesized across a multi-state health system. Med (N Y). 2021. https://doi.org/10.1016/j.medj.2021.06.007 .
Wells G, Shea B, O'Connell D, Peterson J, Welch V, Losos M, Tugwell P. The Newcastle-Ottawa Scale (NOS) for assessing the quality of nonrandomised studies in meta-analyses. http://www.ohri.ca/programs/clinical_epidemiology/oxford.asp . Accessed 20 Aug 2021.
Rostom A, Dubé C, Cranney A, et al. Celiac Disease. Rockville (MD): Agency for Healthcare Research and Quality (US); 2004 Sep. (Evidence Reports/Technology Assessments, No. 104.) Appendix D. Quality Assessment Forms. Available from: https://www.ncbi.nlm.nih.gov/books/NBK35156/ . Accessed 20 Aug 2021
Abu-Raddad LJ, Chemaitelly H, Butt AA. Effectiveness of the BNT162b2 COVID-19 vaccine against the B.1.1.7 and B.1.351 Variants. N Engl J Med. 2021;385(2):187–9. https://doi.org/10.1056/NEJMc2104974 .
Angel Y, Spitzer A, Henig O, Saiag E, Sprecher E, Padova H, Ben-Ami R. Association between vaccination with BNT162b2 and incidence of symptomatic and asymptomatic SARS-CoV-2 infections among health care workers. JAMA. 2021;325(24):2457–65. https://doi.org/10.1001/jama.2021.7152 .
Azamgarhi T, Hodgkinson M, Shah A, Skinner JA, Hauptmannova I, Briggs TWR, Warren S. BNT162b2 vaccine uptake and effectiveness in UK healthcare workers—a single centre cohort study. Nat Commun. 2021;12(1):3698. https://doi.org/10.1038/s41467-021-23927-x .
Bianchi FP, Germinario CA, Migliore G, Vimercati L, Martinelli A, Lobifaro A, Tafuri S, Stefanizzi P. BNT162b2 mRNA COVID-19 vaccine effectiveness in the prevention of SARS-CoV-2 infection: a preliminary report. J Infect Dis. 2021. https://doi.org/10.1093/infdis/jiab262 .
Britton A, Jacobs Slifka KM, Edens C, Nanduri SA, Bart SM, Shang N, Harizaj A, Armstrong J, Xu K, Ehrlich HY, et al. Effectiveness of the Pfizer-BioNTech COVID-19 vaccine among residents of two skilled nursing facilities experiencing COVID-19 outbreaks—Connecticut, December 2020–February 2021. MMWR Morb Mortal Wkly Rep. 2021;70(11):396–401. https://doi.org/10.15585/mmwr.mm7011e3 .
Cavanaugh AM, Fortier S, Lewis P, Arora V, Johnson M, George K, Tobias J, Lunn S, Miller T, Thoroughman D, et al. COVID-19 outbreak associated with a SARS-CoV-2 R1 lineage variant in a skilled nursing facility after vaccination program—Kentucky, March 2021. MMWR Morb Mortal Wkly Rep. 2021;70(17):639–43. https://doi.org/10.15585/mmwr.mm7017e2 .
Chemaitelly H, Yassine HM, Benslimane FM, Al Khatib HA, Tang P, Hasan MR, Malek JA, Coyle P, Ayoub HH, Al Kanaani Z, et al. mRNA-1273 COVID-19 vaccine effectiveness against the B.1.1.7 and B.1.351 variants and severe COVID-19 disease in Qatar. Nat Med. 2021. https://doi.org/10.1038/s41591-021-01446-y .
Chodick G, Tene L, Patalon T, Gazit S, Ben Tov A, Cohen D, Muhsen K. Assessment of effectiveness of 1 dose of BNT162b2 vaccine for SARS-CoV-2 infection 13 to 24 days after immunization. JAMA Netw Open. 2021;4(6): e2115985. https://doi.org/10.1001/jamanetworkopen.2021.15985 .
Chodick G, Tene L, Rotem RS, Patalon T, Gazit S, Ben-Tov A, Weil C, Goldshtein I, Twig G, Cohen D, et al. The effectiveness of the TWO-DOSE BNT162b2 vaccine: analysis of real-world data. Clin Infect Dis. 2021. https://doi.org/10.1093/cid/ciab438 .
Dagan N, Barda N, Kepten E, Miron O, Perchik S, Katz MA, Hernán MA, Lipsitch M, Reis B, Balicer RD. BNT162b2 mRNA COVID-19 vaccine in a nationwide mass vaccination setting. N Engl J Med. 2021;384(15):1412–23. https://doi.org/10.1056/NEJMoa2101765 .
Flacco ME, Soldato G, Acuti Martellucci C, Carota R, Di Luzio R, Caponetti A, Manzoli L. Interim estimates of COVID-19 vaccine effectiveness in a mass vaccination setting: data from an Italian Province. VacCInes (Basel). 2021. https://doi.org/10.3390/vaccines9060628 .
Haas EJ, Angulo FJ, McLaughlin JM, Anis E, Singer SR, Khan F, Brooks N, Smaja M, Mircus G, Pan K, et al. Impact and effectiveness of mRNA BNT162b2 vaccine against SARS-CoV-2 infections and COVID-19 cases, hospitalisations, and deaths following a nationwide vaccination campaign in Israel: an observational study using national surveillance data. Lancet. 2021;397(10287):1819–29. https://doi.org/10.1016/s0140-6736(21)00947-8 .
Hall VJ, Foulkes S, Saei A, Andrews N, Oguti B, Charlett A, Wellington E, Stowe J, Gillson N, Atti A, et al. COVID-19 vaccine coverage in health-care workers in England and effectiveness of BNT162b2 mRNA vaccine against infection (SIREN): a prospective, multicentre, cohort study. Lancet. 2021;397(10286):1725–35. https://doi.org/10.1016/s0140-6736(21)00790-x .
Hyams C, Marlow R, Maseko Z, King J, Ward L, Fox K, Heath R, Tuner A, Friedrich Z, Morrison L, et al. Effectiveness of BNT162b2 and ChAdOx1 nCoV-19 COVID-19 vaccination at preventing hospitalisations in people aged at least 80 years: a test-negative, case-control study. Lancet Infect Dis. 2021. https://doi.org/10.1016/s1473-3099(21)00330-3 .
Khan N, Mahmud N. Effectiveness of SARS-CoV-2 vaccination in a veterans affairs cohort of patients with inflammatory bowel disease with diverse exposure to immunosuppressive medications. Gastroenterology. 2021. https://doi.org/10.1053/j.gastro.2021.05.044 .
Knobel P, Serra C, Grau S, Ibañez R, Diaz P, Ferrández O, Villar R, Lopez AF, Pujolar N, Horcajada JP, et al. COVID-19 mRNA vaccine effectiveness in asymptomatic healthcare workers. Infect Control Hosp Epidemiol. 2021. https://doi.org/10.1017/ice.2021.287 .
Lopez Bernal J, Andrews N, Gower C, Robertson C, Stowe J, Tessier E, Simmons R, Cottrell S, Roberts R, O’Doherty M, et al. Effectiveness of the Pfizer-BioNTech and Oxford-AstraZeneca vaccines on covid-19 related symptoms, hospital admissions, and mortality in older adults in England: test negative case-control study. BMJ. 2021;373: n1088. https://doi.org/10.1136/bmj.n1088 .
Mazagatos C, Monge S, Olmedo C, Vega L, Gallego P, Martín-Merino E, Sierra MJ, Limia A, Larrauri A. Effectiveness of mRNA COVID-19 vaccines in preventing SARS-CoV-2 infections and COVID-19 hospitalisations and deaths in elderly long-term care facility residents, Spain, weeks 53, 2020 to 13 2021. Eurosurveillance. 2021. https://doi.org/10.2807/1560-7917.Es.2021.26.24.2100452 .
Pilishvili T, Fleming-Dutra KE, Farrar JL, Gierke R, Mohr NM, Talan DA, Krishnadasan A, Harland KK, Smithline HA, Hou PC, et al. Interim estimates of vaccine effectiveness of Pfizer-BioNTech and Moderna COVID-19 vaccines among health care personnel—33 US Sites, January–March 2021. MMWR Morb Mortal Wkly Rep. 2021;70(20):753–8. https://doi.org/10.15585/mmwr.mm7020e2 .
Sheikh A, McMenamin J, Taylor B, Robertson C. SARS-CoV-2 Delta VOC in Scotland: demographics, risk of hospital admission, and vaccine effectiveness. Lancet. 2021;397(10293):2461–2. https://doi.org/10.1016/s0140-6736(21)01358-1 .
Shrotri M, Krutikov M, Palmer T, Giddings R, Azmi B, Subbarao S, Fuller C, Irwin-Singer A, Davies D, Tut G, et al. Vaccine effectiveness of the first dose of ChAdOx1 nCoV-19 and BNT162b2 against SARS-CoV-2 infection in residents of long-term care facilities in England (VIVALDI): a prospective cohort study. Lancet Infect Dis. 2021. https://doi.org/10.1016/s1473-3099(21)00289-9 .
Skowronski DM, Setayeshgar S, Zou M, Prystajecky N, Tyson JR, Galanis E, Naus M, Patrick DM, Sbihi H, El Adam S, et al. Single-dose mRNA vaccine effectiveness against SARS-CoV-2, including Alpha and Gamma variants: a test-negative design in adults 70 years and older in British Columbia,Canada. Clin Infect Dis. 2021. https://doi.org/10.1093/cid/ciab616 .
Swift MD, Breeher LE, Tande AJ, Tommaso CP, Hainy CM, Chu H, Murad MH, Berbari EF, Virk A. Effectiveness of mRNA COVID-19 vaccines against SARS-CoV-2 infection in a cohort of healthcare personnel. Clin Infect Dis. 2021. https://doi.org/10.1093/cid/ciab361 .
Thompson MG, Burgess JL, Naleway AL, Tyner H, Yoon SK, Meece J, Olsho LEW, Caban-Martinez AJ, Fowlkes AL, Lutrick K, et al. Prevention and attenuation of COVID-19 with the BNT162b2 and mRNA-1273 Vaccines. N Engl J Med. 2021. https://doi.org/10.1056/NEJMoa2107058 .
Vasileiou E, Simpson CR, Shi T, Kerr S, Agrawal U, Akbari A, Bedston S, Beggs J, Bradley D, Chuter A, et al. Interim findings from first-dose mass COVID-19 vaccination roll-out and COVID-19 hospital admissions in Scotland: a national prospective cohort study. Lancet. 2021;397(10285):1646–57. https://doi.org/10.1016/s0140-6736(21)00677-2 .
Williams C, Al-Bargash D, Macalintal C, Stuart R, Seth A, Latham J, Gitterman L, Fedsin S, Godoy M, Kozak R, et al. COVID-19 outbreak associated with a SARS-CoV-2 P.1 lineage in a long-term care home after implementation of a vaccination program—Ontario, April–May 2021. Clin Infect Dis. 2021. https://doi.org/10.1093/cid/ciab617 .
Alhazmi A, Alamer E, Daws D, Hakami M, Darraj M, Abdelwahab S, Maghfuri A, Algaissi A. Evaluation of side effects associated with COVID-19 vaccines in Saudi Arabia. Vaccines (Basel). 2021. https://doi.org/10.3390/vaccines9060674 .
Andrzejczak-Grządko S, Czudy Z, Donderska M. Side effects after COVID-19 vaccinations among residents of Poland. Eur Rev Med Pharmacol Sci. 2021;25(12):4418–21. https://doi.org/10.26355/eurrev_202106_26153 .
Baldolli A, Michon J, Appia F, Galimard C, Verdon R, Parienti JJ. Tolerance of BNT162b2 mRNA COVI-19 vaccine in patients with a medical history of COVID-19 disease: a case control study. Vaccine. 2021;39(32):4410–3. https://doi.org/10.1016/j.vaccine.2021.06.054 .
Cherian S, Paul A, Ahmed S, Alias B, Manoj M, Santhosh AK, Varghese DR, Krishnan N, Shenoy P. Safety of the ChAdOx1 nCoV-19 and the BBV152 vaccines in 724 patients with rheumatic diseases: a post-vaccination cross-sectional survey. Rheumatol Int. 2021;41(8):1441–5. https://doi.org/10.1007/s00296-021-04917-0 .
Chevallier P, Coste-Burel M, Le Bourgeois A, Peterlin P, Garnier A, Béné MC, Imbert BM, Drumel T, Le Gouill S, Moreau P, et al. Safety and immunogenicity of a first dose of SARS-CoV-2 mRNA vaccine in allogeneic hematopoietic stem-cells recipients. EJHaem. 2021. https://doi.org/10.1002/jha2.242 .
Connolly CM, Ruddy JA, Boyarsky BJ, Avery RK, Werbel WA, Segev DL, Garonzik-Wang J, Paik JJ. Safety of the first dose of mRNA SARS-CoV-2 vaccines in patients with rheumatic and musculoskeletal diseases. Ann Rheum Dis. 2021. https://doi.org/10.1136/annrheumdis-2021-220231 .
Furer V, Eviatar T, Zisman D, Peleg H, Paran D, Levartovsky D, Zisapel M, Elalouf O, Kaufman I, Meidan R, et al. Immunogenicity and safety of the BNT162b2 mRNA COVID-19 vaccine in adult patients with autoimmune inflammatory rheumatic diseases and in the general population: a multicentre study. Ann Rheum Dis. 2021. https://doi.org/10.1136/annrheumdis-2021-220647 .
Gee J, Marquez P, Su J, Calvert GM, Liu R, Myers T, Nair N, Martin S, Clark T, Markowitz L, et al. First month of COVID-19 vaccine safety monitoring—United States, December 14, 2020–January 13, 2021. MMWR Morb Mortal Wkly Rep. 2021;70(8):283–8. https://doi.org/10.15585/mmwr.mm7008e3 .
Hashimoto T, Ozaki A, Bhandari D, Sawano T, Sah R, Tanimoto T. High anaphylaxis rates following vaccination with the Pfizer BNT162b2 mRNA vaccine against COVID-19 in Japanese health care workers; a secondary analysis of initial post-approval safety data. J Travel Med. 2021. https://doi.org/10.1093/jtm/taab090 .
Lv G, Yuan J, Xiong X, Li M. Mortality rate and characteristics of deaths following COVID-19 vaccination. Front Med (Lausanne). 2021;8: 670370. https://doi.org/10.3389/fmed.2021.670370 .
McMurry R, Lenehan P, Awasthi S, Silvert E, Puranik A, Pawlowski C, Venkatakrishnan AJ, Anand P, Agarwal V, O’Horo JC, et al. Real-time analysis of a mass vaccination effort confirms the safety of FDA-authorized mRNA COVID-19 vaccines. Med (N Y). 2021. https://doi.org/10.1016/j.medj.2021.06.006 .
Monin L, Laing AG, Muñoz-Ruiz M, McKenzie DR, Del Molino Del Barrio I, Alaguthurai T, Domingo-Vila C, Hayday TS, Graham C, Seow J, et al. Safety and immunogenicity of one versus two doses of the COVID-19 vaccine BNT162b2 for patients with cancer: interim analysis of a prospective observational study. Lancet Oncol. 2021;22(6):765–78. https://doi.org/10.1016/s1470-2045(21)00213-8 .
Pagotto V, Ferloni A, Mercedes Soriano M, Díaz M, Braguinsky Golde N, González MI, Asprea V, Staneloni MI, Zingoni P, Vidal G, et al. Active monitoring of early safety of Sputnik V vaccine in Buenos Aires, Argentina. MediCIna (B Aires). 2021;81(3):408–14.
Google Scholar
Peled Y, Ram E, Lavee J, Sternik L, Segev A, Wieder-Finesod A, Mandelboim M, Indenbaum V, Levy I, Raanani E, et al. BNT162b2 vaccination in heart transplant recipients: Clinical experience and antibody response. J Heart Lung Transplant. 2021. https://doi.org/10.1016/j.healun.2021.04.003 .
Quiroga B, Sánchez-Álvarez E, Goicoechea M, de Sequera P. COVID-19 vaccination among Spanish nephrologists: acceptance and side effects. J Healthc Qual Res. 2021. https://doi.org/10.1016/j.jhqr.2021.05.002 .
Ram R, Hagin D, Kikozashvilli N, Freund T, Amit O, Bar-On Y, Beyar-Katz O, Shefer G, Moshiashvili MM, Karni C, et al. Safety and immunogenicity of the BNT162b2 mRNA COVID-19 vaccine in patients after allogeneic HCT or CD19-based CART therapy—a single center prospective cohort study. Transplant Cell Ther. 2021. https://doi.org/10.1016/j.jtct.2021.06.024 .
Revon-Riviere G, Ninove L, Min V, Rome A, Coze C, Verschuur A, de Lamballerie X, André N. The BNT162b2 mRNA COVID-19 vaccine in adolescents and young adults with cancer: a monocentric experience. Eur J Cancer. 2021;154:30–4. https://doi.org/10.1016/j.ejca.2021.06.002 .
Riad A, Pokorná A, Mekhemar M, Conrad J, Klugarová J, Koščík M, Klugar M, Attia S. Safety of ChAdOx1 nCoV-19 vaccine: independent evidence from two EU states. Vaccines (Basel). 2021. https://doi.org/10.3390/vaccines9060673 .
Riad A, Sağıroğlu D, Üstün B, Pokorná A, Klugarová J, Attia S, Klugar M. Prevalence and risk factors of CoronaVac Side effects: an independent cross-sectional study among healthcare workers in Turkey. J Clin Med. 2021. https://doi.org/10.3390/jcm10122629 .
Rosman Y, Lavi N, Meir-Shafrir K, Lachover-Roth I, Cohen-Engler A, Mekori YA, Confino-Cohen R. Safety of BNT162b2 mRNA COVID-19 vaccine in patients with mast cell disorders. J Allergy Clin Immunol Pract. 2021. https://doi.org/10.1016/j.jaip.2021.06.032 .
Signorelli C, Odone A, Gianfredi V, Capraro M, Kacerik E, Chiecca G, Scardoni A, Minerva M, Mantecca R, Musarò P, et al. Application of the “immunization islands” model to improve quality, efficiency and safety of a COVID-19 mass vaccination site. Ann Ig. 2021;33(5):499–512. https://doi.org/10.7416/ai.2021.2456 .
Vallée A, Chan-Hew-Wai A, Bonan B, Lesprit P, Parquin F, Catherinot É, Choucair J, Billard D, Amiel-Taieb C, Camps È, et al. Oxford-AstraZeneca COVID-19 vaccine: need of a reasoned and effective vaccine campaign. Public Health. 2021;196:135–7. https://doi.org/10.1016/j.puhe.2021.05.030 .
Wang J, Hou Z, Liu J, Gu Y, Wu Y, Chen Z, Ji J, Diao S, Qiu Y, Zou S, et al. Safety and immunogenicity of COVID-19 vaccination in patients with non-alcoholic fatty liver disease (CHESS2101): a multicenter study. J Hepatol. 2021. https://doi.org/10.1016/j.jhep.2021.04.026 .
Zhang MX, Zhang TT, Shi GF, Cheng FM, Zheng YM, Tung TH, Chen HX. Safety of an inactivated SARS-CoV-2 vaccine among healthcare workers in China. Expert Rev Vaccines. 2021. https://doi.org/10.1080/14760584.2021.1925112 .
Shay DK, Gee J, Su JR, Myers TR, Marquez P, Liu R, Zhang B, Licata C, Clark TA, Shimabukuro TT. Safety monitoring of the Janssen (Johnson & Johnson) COVID-19 Vaccine—United States, March–April 2021. MMWR Morb Mortal Wkly Rep. 2021;70(18):680–4. https://doi.org/10.15585/mmwr.mm7018e2 .
Prevention CCfDCa. Information analysis of COVID-19 vaccine adverse reaction monitoring in China. 2021-5-28. http://www.chinacdc.cn/jkzt/ymyjz/ymyjjz_6758/202105/t20210528_230908.html . Accessed 20 Aug 2021.
Kow CS, Hasan SS. Real-world effectiveness of BNT162b2 mRNA vaccine: a meta-analysis of large observational studies. Inflammopharmacology. 2021;29(4):1075–90. https://doi.org/10.1007/s10787-021-00839-2 .
Angyal A, Longet S, Moore S, Payne RP, Harding A et al. T-Cell and Antibody Responses to First BNT162b2 Vaccine Dose in Previously SARS-CoV-2-Infected and Infection-Naive UK Healthcare Workers: A Multicentre, Prospective, Observational Cohort Study. Available at SSRN: https://ssrn.com/abstract=3820576 or https://doi.org/10.2139/ssrn.3820576 . Accessed 20 Aug 2021.
Pimenta D, Yates C, Pagel C, Gurdasani D. Delaying the second dose of covid-19 vaccines. BMJ. 2021;372: n710. https://doi.org/10.1136/bmj.n710 .
Tauh T, Mozel M, Meyler P, Lee SM. An updated look at the 16-week window between doses of vaccines in BC for COVID-19. BC Med J. 2021;63(3):102–3.
Kadire SR, Wachter RM, Lurie N. Delayed second dose versus standard regimen for COVID-19 vaccination. N Engl J Med. 2021;384(9): e28. https://doi.org/10.1056/NEJMclde2101987 .
Bergwerk M, Gonen T, Lustig Y, Amit S, Lipsitch M, Cohen C, Mandelboim M, Gal Levin E, Rubin C, Indenbaum V, et al. COVID-19 breakthrough infections in vaccinated health care workers. N Engl J Med. 2021. https://doi.org/10.1056/NEJMoa2109072 .
Truong TT, Ryutov A, Pandey U, Yee R, Goldberg L, Bhojwani D, Aguayo-Hiraldo P, Pinsky BA, Pekosz A, Shen L, et al. Persistent SARS-CoV-2 infection and increasing viral variants in children and young adults with impaired humoral immunity. medRxiv. 2021. https://doi.org/10.1101/2021.02.27.21252099 .
Choi B, Choudhary MC, Regan J, Sparks JA, Padera RF, Qiu X, Solomon IH, Kuo HH, Boucau J, Bowman K, et al. Persistence and evolution of SARS-CoV-2 in an Immunocompromised Host. N Engl J Med. 2020;383(23):2291–3. https://doi.org/10.1056/NEJMc2031364 .
Corey L, Beyrer C, Cohen MS, Michael NL, Bedford T, Rolland M. SARS-CoV-2 variants in patients with immunosuppression. N Engl J Med. 2021;385(6):562–6. https://doi.org/10.1056/NEJMsb2104756 .
Bar-On YM, Goldberg Y, Mandel M, Bodenheimer O, Freedman L, Kalkstein N, Mizrahi B, Alroy-Preis S, Ash N, Milo R, et al. Protection of BNT162b2 vaccine booster against Covid-19 in Israel. N Engl J Med. 2021;385(15):1393–400. https://doi.org/10.1056/NEJMoa2114255 .
Hause AM, Baggs J, Gee J, Marquez P, Myers TR, Shimabukuro TT, Shay DK. Safety monitoring of an additional dose of COVID-19 vaccine—United States, August 12–September 19, 2021. MMWR Morb Mortal Wkly Rep. 2021;70(39):1379–84. https://doi.org/10.15585/mmwr.mm7039e4 .
Furlow B. Immunocompromised patients in the USA and UK should receive third dose of COVID-19 vaccine. Lancet Rheumatol. 2021. https://doi.org/10.1016/s2665-9913(21)00313-1 .
Flaxman A, Marchevsky NG, Jenkin D, Aboagye J, Aley PK, Angus B, Belij-Rammerstorfer S, Bibi S, Bittaye M, Cappuccini F, et al. Reactogenicity and immunogenicity after a late second dose or a third dose of ChAdOx1 nCoV-19 in the UK: a substudy of two randomised controlled trials (COV001 and COV002). Lancet. 2021;398(10304):981–90. https://doi.org/10.1016/s0140-6736(21)01699-8 .
Peled Y, Ram E, Lavee J, Segev A, Matezki S, Wieder-Finesod A, Halperin R, Mandelboim M, Indenbaum V, Levy I, et al. Third dose of the BNT162b2 vaccine in heart transplant recipients: immunogenicity and clinical experience. J Heart Lung Transplant. 2021. https://doi.org/10.1016/j.healun.2021.08.010 .
WHO. WHO press conference on coronavirus disease (COVID-19)—4 August 2021. 2021. https://www.who.int/multi-media/details/who-press-conference-on-coronavirus-disease-(covid-19)---4-august-2021 . Accessed 20 Aug 2021.
Cascella M, Rajnik M, Aleem A, Dulebohn SC, Di Napoli R. Features, evaluation, and treatment of coronavirus (COVID-19). In: StatPearls. edn. Treasure Island (FL): StatPearls Publishing Copyright © 2021, StatPearls Publishing LLC.; 2021.
Goshen-Lago T, Waldhorn I, Holland R, Szwarcwort-Cohen M, Reiner-Benaim A, Shachor-Meyouhas Y, Hussein K, Fahoum L, Baruch M, Peer A, et al. Serologic status and toxic effects of the SARS-CoV-2 BNT162b2 vaccine in patients undergoing treatment for cancer. JAMA Oncol. 2021. https://doi.org/10.1001/jamaoncol.2021.2675 .
Ou MT, Boyarsky BJ, Motter JD, Greenberg RS, Teles AT, Ruddy JA, Krach MR, Jain VS, Werbel WA, Avery RK, et al. Safety and reactogenicity of 2 doses of SARS-CoV-2 vaccination in solid organ transplant recipients. Transplantation. 2021. https://doi.org/10.1097/tp.0000000000003780 .
Bookstein Peretz S, Regev N, Novick L, Nachshol M, Goffer E, Ben-David A, Asraf K, Doolman R, Sapir E, Regev Yochay G, et al. Short-term outcome of pregnant women vaccinated by BNT162b2 mRNA COVID-19 vaccine. Ultrasound Obstet Gynecol. 2021. https://doi.org/10.1002/uog.23729 .
Shimabukuro TT, Kim SY, Myers TR, Moro PL, Oduyebo T, Panagiotakopoulos L, Marquez PL, Olson CK, Liu R, Chang KT, et al. Preliminary findings of mRNA COVID-19 vaccine safety in pregnant persons. N Engl J Med. 2021;384(24):2273–82. https://doi.org/10.1056/NEJMoa2104983 .
Peled Y, Ram E, Lavee J, Sternik L, Segev A, Wieder-Finesod A, Mandelboim M, Indenbaum V, Levy I, Raanani E, et al. BNT162b2 vaccination in heart transplant recipients: clinical experience and antibody response. J Heart Lung Transplant. 2021;40(8):759–62. https://doi.org/10.1016/j.healun.2021.04.003 .
Thomas SJ, Moreira ED Jr, Kitchin N, Absalon J, Gurtman A, Lockhart S, Perez JL, Pérez Marc G, Polack FP, Zerbini C, et al. Safety and efficacy of the BNT162b2 mRNA COVID-19 vaccine through 6 months. N Engl J Med. 2021. https://doi.org/10.1056/NEJMoa2110345 .
Falsey AR, Sobieszczyk ME, Hirsch I, Sproule S, Robb ML, Corey L, Neuzil KM, Hahn W, Hunt J, Mulligan MJ, et al. Phase 3 safety and efficacy of AZD1222 (ChAdOx1 nCoV-19) COVID-19 vaccine. N Engl J Med. 2021. https://doi.org/10.1056/NEJMoa2105290 .
El Sahly HM, Baden LR, Essink B, Doblecki-Lewis S, Martin JM, Anderson EJ, Campbell TB, Clark J, Jackson LA, Fichtenbaum CJ, et al. Efficacy of the mRNA-1273 SARS-CoV-2 vaccine at completion of blinded phase. N Engl J Med. 2021. https://doi.org/10.1056/NEJMoa2113017 .
Tanriover MD, Doğanay HL, Akova M, Güner HR, Azap A, Akhan S, Köse Ş, Erdinç F, Akalın EH, Tabak ÖF, et al. Efficacy and safety of an inactivated whole-virion SARS-CoV-2 vaccine (CoronaVac): interim results of a double-blind, randomised, placebo-controlled, phase 3 trial in Turkey. Lancet. 2021;398(10296):213–22. https://doi.org/10.1016/s0140-6736(21)01429-x .
Kumar S, Saurabh MK, Maharshi V. Efficacy and safety of potential vaccine candidates against coronavirus disease 2019: a systematic review. J Adv Pharm Technol Res. 2021;12(3):215–21. https://doi.org/10.4103/japtr.JAPTR_229_20 .
Burgos-Salcedo J. A rational strategy to support approved COVID-19 vaccines prioritization. Hum Vaccin Immunother. 2021;17(10):3474–7. https://doi.org/10.1080/21645515.2021.1922060 .
Download references
Acknowledgements
This study was funded by the National Natural Science Foundation of China (72122001; 71934002) and the National Science and Technology Key Projects on Prevention and Treatment of Major infectious disease of China (2020ZX10001002). The funders had no role in study design, data collection and analysis, decision to publish, or preparation of the paper. No payment was received by any of the co-authors for the preparation of this article.
Author information
Qiao Liu and Chenyuan Qin are joint first authors
Authors and Affiliations
Department of Epidemiology and Biostatistics, School of Public Health, Peking University, Beijing, 100191, China
Qiao Liu, Chenyuan Qin, Min Liu & Jue Liu
Institute for Global Health and Development, Peking University, Beijing, 100871, China
Chenyuan Qin & Jue Liu
You can also search for this author in PubMed Google Scholar
Contributions
LQ and QCY contributed equally as first authors. LJ and LM contributed equally as correspondence authors. LJ and LM conceived and designed the study; LQ, QCY and LJ carried out the literature searches, extracted the data, and assessed the study quality; LQ and QCY performed the statistical analysis and wrote the manuscript; LJ, LM, LQ and QCY revised the manuscript. All authors read and approved the final manuscript.
Corresponding authors
Correspondence to Min Liu or Jue Liu .
Ethics declarations
Ethics approval and consent to participate.
Not applicable.
Consent for publication
Competing interests.
The authors have no conflicts of interest to declare that are relevant to the content of this article.
Supplementary Information
Additional file 1: table s1..
Characteristic of studies included for vaccine effectiveness.
Additional file 2: Table S2.
Characteristic of studies included for vaccine safety.
Rights and permissions
Open Access This article is licensed under a Creative Commons Attribution 4.0 International License, which permits use, sharing, adaptation, distribution and reproduction in any medium or format, as long as you give appropriate credit to the original author(s) and the source, provide a link to the Creative Commons licence, and indicate if changes were made. The images or other third party material in this article are included in the article's Creative Commons licence, unless indicated otherwise in a credit line to the material. If material is not included in the article's Creative Commons licence and your intended use is not permitted by statutory regulation or exceeds the permitted use, you will need to obtain permission directly from the copyright holder. To view a copy of this licence, visit http://creativecommons.org/licenses/by/4.0/ . The Creative Commons Public Domain Dedication waiver ( http://creativecommons.org/publicdomain/zero/1.0/ ) applies to the data made available in this article, unless otherwise stated in a credit line to the data.
Reprints and permissions
About this article
Cite this article.
Liu, Q., Qin, C., Liu, M. et al. Effectiveness and safety of SARS-CoV-2 vaccine in real-world studies: a systematic review and meta-analysis. Infect Dis Poverty 10 , 132 (2021). https://doi.org/10.1186/s40249-021-00915-3
Download citation
Received : 07 September 2021
Accepted : 01 November 2021
Published : 14 November 2021
DOI : https://doi.org/10.1186/s40249-021-00915-3
Share this article
Anyone you share the following link with will be able to read this content:
Sorry, a shareable link is not currently available for this article.
Provided by the Springer Nature SharedIt content-sharing initiative
- Effectiveness
- Meta-analysis
Infectious Diseases of Poverty
ISSN: 2049-9957
- Submission enquiries: Access here and click Contact Us
- General enquiries: [email protected]
Click through the PLOS taxonomy to find articles in your field.
For more information about PLOS Subject Areas, click here .
Loading metrics
Open Access
Peer-reviewed
Research Article
COVID-19 and vaccine hesitancy: A longitudinal study
Roles Conceptualization, Data curation, Formal analysis, Investigation, Methodology, Project administration, Resources, Supervision, Validation, Visualization, Writing – original draft, Writing – review & editing
* E-mail: [email protected]
Affiliation Rady School of Management, University of California San Diego, La Jolla, California, United States of America

Roles Conceptualization, Data curation, Funding acquisition, Investigation, Methodology, Project administration, Supervision, Validation, Visualization, Writing – original draft, Writing – review & editing
Roles Conceptualization, Funding acquisition, Investigation, Methodology, Project administration, Supervision, Visualization, Writing – original draft, Writing – review & editing
- Ariel Fridman,
- Rachel Gershon,
- Ayelet Gneezy
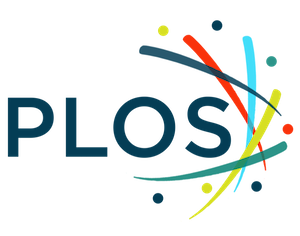
- Published: April 16, 2021
- https://doi.org/10.1371/journal.pone.0250123
- Peer Review
- Reader Comments
How do attitudes toward vaccination change over the course of a public health crisis? We report results from a longitudinal survey of United States residents during six months (March 16 –August 16, 2020) of the COVID-19 pandemic. Contrary to past research suggesting that the increased salience of a disease threat should improve attitudes toward vaccines, we observed a decrease in intentions of getting a COVID-19 vaccine when one becomes available. We further found a decline in general vaccine attitudes and intentions of getting the influenza vaccine. Analyses of heterogeneity indicated that this decline is driven by participants who identify as Republicans, who showed a negative trend in vaccine attitudes and intentions, whereas Democrats remained largely stable. Consistent with research on risk perception and behavior, those with less favorable attitudes toward a COVID-19 vaccination also perceived the virus to be less threatening. We provide suggestive evidence that differential exposure to media channels and social networks could explain the observed asymmetric polarization between self-identified Democrats and Republicans.
Citation: Fridman A, Gershon R, Gneezy A (2021) COVID-19 and vaccine hesitancy: A longitudinal study. PLoS ONE 16(4): e0250123. https://doi.org/10.1371/journal.pone.0250123
Editor: Valerio Capraro, Middlesex University, UNITED KINGDOM
Received: November 12, 2020; Accepted: February 14, 2021; Published: April 16, 2021
Copyright: © 2021 Fridman et al. This is an open access article distributed under the terms of the Creative Commons Attribution License , which permits unrestricted use, distribution, and reproduction in any medium, provided the original author and source are credited.
Data Availability: All data and code are publicly available on the Open Science Framework at https://osf.io/kgvdy/ .
Funding: UC San Diego Global Health Initiative (GHI): awarded to all authors; Project number: 1001288. The funders had no role in study design, data collection and analysis, decision to publish, or preparation of the manuscript. https://medschool.ucsd.edu/som/medicine/divisions/idgph/research/Global-Health/grant-recipients/2019-2020/Pages/Faculty-Postdoc-Travel-and-Research.aspx .
Competing interests: The authors have declared that no competing interests exist.
Introduction
Vaccinations are among the most important public health tools for reducing the spread and harm caused by dangerous diseases [ 1 ]. The World Health Organization estimates that vaccines prevented at least 10 million deaths between 2010–2015 worldwide [ 2 ]. Despite considerable evidence showing vaccines are safe [ 3 , 4 ], there is increasing skepticism toward vaccination [ 5 , 6 ]. Vaccine hesitancy has led to a decline in vaccine uptake and to an increase in the prevalence of vaccine-preventable diseases (VPDs) [ 7 , 8 ]. Ironically, the objection to vaccines is commonly a consequence of their effectiveness—because individuals have lower exposure to VPDs, they are less concerned about contracting them [ 9 ], which consequently leads to greater vaccine hesitancy [ 10 ]. The COVID-19 pandemic has created a new reality where individuals are faced with a previously unknown disease and its effects, providing a unique opportunity to investigate vaccine attitudes during a period of heightened disease salience. The present research reports findings from a longitudinal study conducted during the COVID-19 health crisis, in which we measured changes in attitudes toward a prospective vaccine, as well as shifts in vaccine attitudes in general.
Factors influencing vaccine attitudes and behaviors
Past research has identified a variety of situational and individual-level factors that influence vaccine attitudes and behavior, the most prominent of which are risk perceptions and demographic characteristics.
Assessments of risk are influenced by both cognitive evaluations (i.e., objective features of the situation such as probabilities of outcomes) and affective reactions [ 11 ], as well as by contextual factors (e.g., the information that is most available or salient at the time [ 12 ]). For example, research shows that media coverage plays a significant role in determining the extent to which we take threats seriously [ 13 ]. When individuals perceive heightened risk of a threat, they become more favorable toward interventions that mitigate that threat, including vaccination (for a meta-analysis on the effect of perceived risk on intentions and behaviors, see [ 14 ]). In the case of COVID-19, this would suggest more positive attitudes toward a vaccine and greater likelihood to get vaccinated. Indeed, research suggests that individuals should exhibit a greater interest in vaccinations during a pandemic because disease threat is more salient [ 15 ].
Past efforts to improve vaccine attitudes have had limited success or even backfired; for example, messages refuting claims about the link between vaccines and autism, as well as messages featuring images of children who were sick with VPDs, had negative effects on vaccine attitudes among those who were already hesitant to vaccinate [ 16 ]. In contrast, messaging that increases disease threat salience has shown promise in reducing vaccine hesitancy [ 5 ], and there is evidence suggesting that increased threat salience for a particular disease may also increase intentions to vaccinate for other diseases [ 17 ]. Building on these findings, we expected to find an increase in pro-vaccine attitudes and in individuals’ interest in a COVID-19 vaccine when the perceived threat of the COVID-19 virus increased.
Vaccine attitudes are also influenced by a variety of demographic and ideological factors (for a review, see [ 18 ]). For example, perceptions of vaccine risk differ among individuals of different ethnic backgrounds [ 19 ], and there is extant work demonstrating a positive correlation between socioeconomic status (SES) and vaccine hesitancy [ 20 , 21 ]. Socio-demographic factors are also linked to vaccine-related behaviors: among college students, those whose parents have attained a higher level of education are more likely to get immunized [ 22 ], and researchers have identified age as a predictor for receiving the influenza vaccine [ 23 ].
Political ideology is another well-documented determinant of vaccine-related attitudes and behaviors. Despite a common belief that liberals tend toward anti-vaccination attitudes in the United States, there is strong evidence that this trend is more present among conservatives [ 24 , 25 ]. According to a recent Gallup Poll, Republicans are twice as likely to believe the widely debunked myth that vaccines cause autism [ 26 ]. Recent work has shown that exposure to anti-vaccination tweets by President Trump—the first known U.S. president to publicly express anti-vaccination attitudes—has led to increased concern about vaccines among his supporters [ 27 ]. Based on these findings, and in conjunction with the sentiments expressed by the White House that diminished the significance of the pandemic [ 28 ], we expected to find diverging trends between Democrats and Republicans.
The current research
We collected vaccine-related attitudes of individuals living in the U.S. over a six-month period. Beginning in March 2020, we elicited attitudes from a cohort of the same individuals every month. We began data collection before any COVID-19 lockdown measures were in place (i.e., prior to the nation’s first shelter-in-place order [ 29 ]). Hence, our data spans the early phase of the pandemic, when there were fewer than 2,000 total confirmed cases in the U.S., through the following six months, at which point cumulative cases reached over 5.3 million [ 30 ].
Despite our prediction—that a public health crisis would increase disease threat, consequently increasing pro-vaccine attitudes and interest in vaccination—our data show an overall decrease in favorable attitudes toward vaccines. A closer look at the data revealed that political orientation explains more variance than any other socio-demographic variable. Specifically, participants who identify as Republican showed a decrease in their intention to get the COVID-19 vaccine and the influenza vaccine as well as a general decrease in pro-vaccine attitudes, whereas Democrats’ responses to these measures did not show a significant change during this period.
Our work is the first, to our knowledge, to longitudinally measure individuals’ attitudes toward vaccines. In doing so, our findings advance the understanding of how vaccine attitudes might change during an unprecedented public health crisis, revealing a strong association between political party affiliation and vaccine attitudes.
Participants
We recruited a panel of U.S. residents on Amazon’s Mechanical Turk platform to respond to multiple survey waves. To incentivize completion of all waves, we informed participants their payment would increase for subsequent surveys. Participants were paid 30 cents for wave 1, 40 cents for wave 2, and 60 cents for waves 3 and 4, $1.00 for wave 5, and $1.20 for wave 6. In addition, participants were informed that those who completed the first three waves would enter a $100 raffle. The median survey completion time was 5.5 minutes. The sample size for the first wave was 1,018, and the number of participants ranged from 608–762 on subsequent waves (see S1 Table for attrition details). This project was certified as exempt from IRB review by the University of California, San Diego Human Research Protections Program (Project #191273XX).
Our panel represents the broad and diverse population of the United States. The first wave sample included participants from all 50 states (except Wyoming) and Washington D.C., with an age range of 18 to 82 years old (mean = 38.48, median = 35). Approximately half (53%) identified as male, 46% as female, and.6% as other. The racial makeup in our sample was: 80% White, 9% Asian, 6% Black or African American, 4% multiple racial or ethnic identities, and 1% other. Relative to the U.S. Census (2019) [ 31 ] estimates, our sample over-represents White and Asian individuals, and under-represents Black or African American individuals and other racial groups.
We elicited political affiliation using a 6-point Likert scale, ranging from Strongly Republican to Strongly Democratic. In wave 1, 62% identified as Democrats and 38% identified as Republican, which is consistent with results from the most recent General Social Survey (GSS) [ 32 ]. There was no significant change in mean political identity from wave 1 to waves 2–6 (see S2 Table ). We classified participants as Democrats or Republicans using wave 1 political party affiliation. See S2 Appendix for additional details about the correlation of political party affiliation with age, gender, and SES.
Questions and measures
Our primary measure of interest was participants’ stated intention to get the COVID-19 vaccine when it becomes available. We were also interested in their perceptions of COVID-19 threat, general vaccination attitudes, and intention to get the flu shot. For all measures, except flu shot intentions, we combined multiple items to create a composite measure (see S2 Table for specific questions and construct compositions). Questions designed to measure general vaccination attitudes were adapted from prior work [ 33 ].
Additional measures of interest were participants’ trust in broad institutions (media, local government, and federal government). These trust measures followed different trends from each other, and therefore were not combined. At the end of the survey, participants responded to demographic questions. We retained all questions used in wave 1 throughout all six waves (our survey included additional items not reported in this paper; see S2 and S3 Tables for a complete list of measured items).
Data and analysis plan
Only participants with non-missing and non-duplicated responses were included in the analyses (see S1 Appendix for additional details). For all outcomes of interest, we tested for linear trends over time using a fixed effects regression specification [ 34 ]. All regression results include individual-level fixed effects, and standard errors are clustered at the individual level, to adjust for within-person correlation. We used this approach to control for the impact of omitted or unobserved time-invariant variables. P-values are not adjusted for multiple testing (see [ 35 ]). All analyses were conducted using R (version 4.0.2), and regressions were run using the package “fixest” (version 0.6.0). All materials, data, and additional analyses including robustness checks can be found here: https://osf.io/kgvdy/ .
We report results for three different vaccination-related measures: attitudes toward a COVID-19 vaccine, general vaccination attitudes, and flu shot intentions. All measures showed a decreasing trend (Ps < .001, except flu shot intentions where p = .05) for the 6-month duration of the study, indicating a reduction in pro-vaccination attitudes and intention to get vaccinated (COVID-19 and influenza vaccines). See S4 Table for full results of all regressions.
Heterogeneity in trend by political party
To better understand whether the decline in vaccine attitudes over time was driven by a particular factor, we used a data-driven approach, regressing all demographic characteristics on vaccine attitudes, in separate regressions. These demographics included education, income, SES, race, gender, an item measuring whether participants considered themselves to be a minority, whether the participant has children, and political party. Education, income, and SES were median split; race and gender were dummy coded; and political party affiliation was dichotomized into Democrat or Republican. Among all demographic characteristics, separating time trends by political affiliation (by adding an interaction term) attained the greatest adjusted within-R 2 in explaining vaccination attitude measures. In other words, political party affiliation explains the greatest within-individual variation in vaccine attitudes over time.
An analysis of responses by political affiliation revealed that the observed decreasing trend in all three vaccine measures was mostly driven by participants who identified as Republican (all Ps < .05), whereas Democrats’ responses showed either no significant trend (for COVID-19 vaccination and flu shot intentions: Ps >.67) or a significantly less negative time trend (general vaccination: p < .001). For these regressions, and moving forward, all results included interactions between wave and political party as well as interactions for wave and age, and wave and SES, to control for potentially different time trends associated with these variables. In each regression we also tested whether the strength of political affiliation moderates the observed results, and we reported the result when it did. We also conducted ANOVAs to compare mean responses for the outcomes of interest between Democrats and Republicans, separately for each wave (see S5 Table ).
COVID-19 vaccination attitudes ( Fig 1 , Panel A).
- PPT PowerPoint slide
- PNG larger image
- TIFF original image
Points represent means, and error bars represent 95% confidence intervals. All scale responses range from 1 to 7.
https://doi.org/10.1371/journal.pone.0250123.g001
A two-item construct ( r = .78) was created, with greater values corresponding to more favorable responses.
In wave 1, Democrats ( M = 5.39, SD = 1.55) had more favorable attitudes toward a COVID-19 vaccine than Republicans ( M = 4.57, SD = 1.76; t = -7.38, p < .001, d = -.48, 95% CI = [-.61, -.35]). Among Democrats, there was no significant time trend ( β = .02, SE = .04, p >.67) whereas Republicans’ responses followed a decreasing time trend ( β = -.09, SE = .05, p = .046). These trends were significantly different from each other ( β = -.11, SE = .02, p < .001).
General vaccination attitudes ( Fig 1 , Panel B).
A ten-item construct ( α = .95) was created, with greater values corresponding to a more positive attitude toward vaccination in general.
In wave 1, Democrats ( M = 5.83, SD = 1.15) expressed more favorable general vaccination attitudes than Republicans ( M = 5.17, SD = 1.31; t = -7.91, p < .001, d = -.52, 95% CI = [-.66, -.39]). Although both Democrats and Republicans had a decreasing time trend (Democrats: β = -.04, SE = .02, p = .029; Republicans: β = -.09, SE = .02, p < .001), the trend for Republicans was significantly more negative ( β = -.04, SE = .01, p < .001).
Flu shot intentions ( Fig 1 , Panel C).
We asked participants whether they plan to get the flu shot next year, with greater values indicating greater intentions.
In wave 1, Democrats ( M = 4.84, SD = 2.34) indicated greater intentions to vaccinate against the flu than Republicans ( M = 4.35, SD = 2.39; t = -3.15, p = .002, d = -.21, 95% CI = [-.34, -.08]). Among Democrats, there was no significant time trend ( β = .01, SE = .04, p = .86), suggesting their vaccination intentions remained largely stable. Republicans’ responses, however, revealed a decreasing time trend ( β = -.12, SE = .04, p = .005), and the two trends were significantly different from each other ( β = -.12, SE = .02, p < .001).
Our analyses revealed an interaction with political affiliation strength among Republicans, whereby participants who identified as more strongly Republican had a more negative time trend ( β = -.05, SE = .02, p = .027). This interaction was not significant for Democrats ( β = -.02, SE = .01, p = .19).
Perceived threat of COVID-19 ( Fig 2 ).
https://doi.org/10.1371/journal.pone.0250123.g002
A three-item construct ( α = .82) was created, with greater perceived threat about COVID-19.
In wave 1, Democrats ( M = 4.26, SD = 1.25) expressed greater perceived threat of COVID-19 than Republicans ( M = 3.90, SD = 1.39; t = -4.14, p < .001, d = -.40, 95% CI = [-.27, -.14]). Democrats’ responses showed an increasing time trend ( β = .08, SE = .04, p = .033), indicating they became increasingly concerned about the threat posed by the virus over time. Among Republicans, there was no significant time trend ( β = -.01, SE = .04, p = .83). These trends were significantly different from each other ( β = -.09, SE = .02, p < .001). While our data does not render causal claims, it is possible that the divergence in COVID-19 threat perceptions over time among Republicans and Democrats contributes to the divergence in vaccine attitudes between these groups over time. We revisit this proposition in the General Discussion.
Our analyses revealed an interaction with political affiliation strength among Democrats—participants who identified as more strongly Democrat had a more positive time trend ( β = .03, SE = .01, p = .019), suggesting an increasing threat perception over time. This interaction was not significant for Republicans ( β = .01, SE = .02, p = .61).
Trust in broad institutions.
The measures of trust in media, local government, and federal government were not highly correlated ( α = .66), and were therefore analyzed separately.
Trust in media ( Fig 3 , Panel A) . In wave 1, Democrats ( M = 3.61, SD = 1.66) reported greater trust in the media than Republicans ( M = 2.73, SD = 1.65; t = -8.12, p < .001, d = -.53, 95% CI = [-.66, -.39]). There was no significant time trend for either Democrats ( β = .02, SE = .04, p = .57) or Republicans ( β = -.05, SE = .04, p = .20). However, the trend for Republicans was significantly more negative ( β = -.07, SE = .02, p < .001). The different trends we observe for Democrats and Republicans with respect to trust in the media may explain the divergence in perceived threat and vaccine attitudes between these groups over time (see General discussion ).
https://doi.org/10.1371/journal.pone.0250123.g003
Trust in local government ( Fig 3 , Panel B) . In wave 1, Democrats ( M = 4.07, SD = 1.60) indicated lower trust in local government than Republicans ( M = 4.28, SD = 1.60; t = 2.01, p = .045, d = .13, 95% CI = [.003,.26]). Among Democrats, there was no significant time trend ( β = -.06, SE = .04, p = .18), though among Republicans, there was a decreasing time trend ( β = -.11, SE = .05, p = .015). These trends were significantly different from each other ( β = -.06, SE = .02, p = .004).
Trust in federal government ( Fig 3 , Panel C) . In wave 1, Democrats ( M = 2.96, SD = 1.67) expressed lower trust in the federal government than Republicans ( M = 4.08, SD = 1.60; t = 10.52, p < .001, d = .68, 95% CI = [.55,.82]). Both Democrats and Republicans had decreasing time trends (Democrats: β = -.08, SE = .04, p = .036; Republicans: β = -.10, SE = .04, p = .025). These trends were not significantly different from each other ( β = -.02, SE = .02, p = .37).
To rule out differential attrition, we tested whether the composition of our sample (i.e., age, gender, and political party) changed over time (see S1 Table ). Specifically, we tested whether participants who responded to waves 2–6 were significantly different at baseline (wave 1) from the full sample at baseline. The only significant change detected (Ps < .05) was with respect to participants’ age, though the differences were small—the average age was 38.5 at baseline, and remained between 39.9 and 40.8 at baseline among participants who responded to subsequent waves. We found no other systematic pattern of attrition among our participants.
General discussion
Over the course of six months of the COVID-19 pandemic, beginning with a relatively early phase prior to any U.S. directives to stay home (March 2020) and continuing through a cumulation of over 5 million cases (August 2020), we found a decrease in pro-vaccine attitudes and COVID-19 vaccination intentions, as well as reduced intentions to get the influenza vaccine. These findings are contrary to our prediction that increased salience of COVID-19 would improve attitudes toward vaccines.
Our analyses identify political ideology as the best predictor of the decreasing time trend across our three vaccine-related attitudes and intentions measures. In particular, we found that while Democrats’ responses remained fairly stable over time, Republicans shifted away from their lower initial responses and from Democrats’ responses, leading to increased polarization throughout the six-month period.
Contrary to the polarization observed in our data, social and behavioral scientists have long argued that groups facing threats often come together, demonstrating stronger social cohesion [ 36 ], and more cooperative behaviors [ 37 , 38 ]. Researchers have also found that individuals’ sense of shared identity plays a role in promoting cooperative behavior in response to threat [ 39 – 41 ]. Considering our results in the context of these findings might suggest that our respondents’ sense of shared identity was dominated by their political ideology, as opposed to a broader (e.g. American) identity.
What might be going on?
Although the nature of our data does not render causal claims, it highlights potential explanations. First, we note that participants’ ratings of perceived COVID-19 threat followed a similar diverging pattern by party affiliation to our three vaccine-related measures during the study period. Democrats perceived COVID-19 threat to be greater at the start of the study than Republicans did, and this gap widened significantly as the study progressed. This trend is consistent with previous research showing that vaccine hesitancy is related to perceived risk of a threat; when a VPD threat level is low, individuals are less motivated to take preventative action (i.e., immunize; for a review, see [ 42 ]).
Our data offers one potential explanation for the polarization of threat perception: Republican and Democratic participants in our study reported consuming different sources of information. The most commonly checked news source for Republicans was Fox News (Republicans: 50%, Democrats: 8%; χ 2 = 164.55, p < .001) and for Democrats was CNN (Democrats: 47%, Republicans: 23%, χ 2 = 43.08, p < .001, see S6 Table ). Corroborating this proposition, a Pew Research Center poll conducted in March 2020 found that 56% of respondents whose main news source is Fox News believed that “the news media have greatly exaggerated the risks about the Coronavirus outbreak,” whereas this was only true for 25% of those whose main news source is CNN [ 43 ]. Of note, Facebook and Instagram, were also in the top four most consumed news sources for participants affiliated with either party. Extant work describes these platforms as echo chambers [ 44 , 45 ], which may exacerbate partisan exposure to news and information.
Another trend highlighted by our data shows that similar to vaccine attitudes, Republicans’ trust in the media decreased significantly more during our study than Democrats’, suggesting these patterns might be related. According to Dr. Heidi Larson, an expert on vaccine hesitancy and founder of the Vaccine Confidence Project, misinformation regarding vaccinations is more likely to take root when individuals do not trust the information source [ 46 ]. Future research might further examine the role of trust in the media on vaccine attitudes.
While trust in media or media exposure may be driving COVID-19 threat perceptions and vaccine attitudes, there are many other possible explanatory factors that are not captured by our data or analyses. For example, it is possible that threat perceptions were influenced by how a respondents’ county or state was affected by COVID-19; up until June 2020, COVID-19 cases were more common in Democrat-leaning states [ 47 ], which might have amplified its salience early on and influenced attitudes and behavior. Further, although we included individual-level fixed-effects which control for all time invariant participant characteristics, and controlled for different trends by age and SES, we cannot rule out the possibility that other factors (e.g., educational attainment or population density) may have influenced the observed trends. Finally, as our data collection began after the onset of COVID-19, it is possible that the trend we observe for Republicans represents a return to a pre-pandemic baseline of vaccine-related attitudes.
Contributions
This work advances our understanding of how health-related attitudes evolve over time. Our focus on vaccine-related attitudes and intentions is important because experts agree that having enough people vaccinate against COVID-19 is key to stemming the pandemic [ 48 ]. More broadly, negative attitudes toward vaccination in general, and reduced vaccine uptake, is increasingly a public health concern [ 49 ]. This research provides insight into the trends of such vaccine hesitancy, underlining the importance of risk salience and its roots in ideology and media exposure.
This work also contributes to our understanding of political parties and polarization. Numerous anecdotes and reports have demonstrated a partisan divide in Americans’ response to the COVID-19 pandemic. For example, research found greater negative affective responses to wearing a face covering among politically right (vs. left) leaning individuals [ 50 ]. Here, we show that although these observations are valid, the reality is more nuanced. For example, our analyses reveal that polarization on vaccine measures—both attitudes and intentions—is driven primarily by self-identified Republicans’ gradual movement away from their initial responses whereas Democrats’ responses remained largely stable. This insight has important practical implications: It informs us about the dynamics of individuals’ attitudes, bringing us closer to understanding the underlying factors that influence attitudes and behaviors. Equipped with this knowledge, one could design more effective communications and interventions.
Note on methodology and data availability
The present study contributes to a small but growing literature in the social sciences using longitudinal data [ 51 ]. Using a longitudinal methodology allowed us to track individual-level changes over time. Merely observing a single point in time would allow us to observe across-group differences, but would lack the bigger picture of how polarization between these groups evolved. Another key advantage of panel data is that it allows us to include individual-level fixed effects, which control for the impact of omitted or unobserved time-invariant variables. Finally, panel data allows for more accurate inference of model parameters [ 52 ].
While the focus of this paper is vaccine attitudes, our broad dataset offers a unique opportunity to understand attitudes and behavior over time. Due to the richness of our data, its unique nature, and its timeliness, we believe it is important to make it available to other researchers interested in exploring it and publishing additional findings. The complete dataset is available at https://osf.io/kgvdy/ (see S2 and S3 Tables for all items collected).
Supporting information
S1 appendix. additional information about sample exclusions..
https://doi.org/10.1371/journal.pone.0250123.s001
S2 Appendix. Additional information about political party affiliation.
https://doi.org/10.1371/journal.pone.0250123.s002
S1 Table. Attrition table.
https://doi.org/10.1371/journal.pone.0250123.s003
S2 Table. Summary table of measures and constructs included in the text.
https://doi.org/10.1371/journal.pone.0250123.s004
S3 Table. Summary table of measures excluded from the text.
https://doi.org/10.1371/journal.pone.0250123.s005
S4 Table. Regression results.
https://doi.org/10.1371/journal.pone.0250123.s006
S5 Table. Outcome measures by political party affiliation.
https://doi.org/10.1371/journal.pone.0250123.s007
S6 Table. Summary of news sources.
https://doi.org/10.1371/journal.pone.0250123.s008
- View Article
- Google Scholar
- 2. World Health Organization. The Power of Vaccines: Still not fully utilized. WHO; 2020. https://www.who.int/publications/10-year-review/vaccines/en/ .
- 4. Immunization Safety Review Committee. Immunization safety review: vaccines and autism. National Academies Press; 2004 Sep 30.
- PubMed/NCBI
- 25. Luton R, Hare C. Conservatives are more likely to believe that vaccines cause autism. The Washington Post. 2015 March 1. https://www.washingtonpost.com/news/monkey-cage/wp/2015/03/01/conservatives-are-more-likely-to-believe-that-vaccines-cause-autism/ .
- 26. Reinhart R. Fewer in US continue to see vaccines as important. 2020 Jan 24. https://news.gallup.com/poll/276929/fewer-continue-vaccines-important.aspx .
- 28. Summers, Juana Timeline: How Trump Has Downplayed The Coronavirus Pandemic. NPR. 2020 October 2, 2020. https://www.npr.org/sections/latest-updates-trump-covid-19-results/2020/10/02/919432383/how-trump-has-downplayed-the-coronavirus-pandemic
- 29. Ortiz J, Hauck G. Coronavirus in the US. USA Today. 2020 March 30, 2020. https://www.usatoday.com/story/news/nation/2020/03/30/coronavirus-stay-home-shelter-in-place-orders-by-state/5092413002/ .
- 30. Elflein J. State of Health. World Health Organization. 2020 October 5. https://www.statista.com/statistics/1103185/cumulative-coronavirus-covid19-cases-number-us-by-day/ .
- 31. United States Census Bureau. Quick Facts. 2019. https://www.census.gov/quickfacts/fact/table/US/PST045219 .
- 32. Smith TW, Davern M, Freese J, Morgan S. General Social Surveys, National Science Foundation. 2020. gssdataexplorer.norc.org
- 34. Wooldridge JM. Econometric analysis of cross section and panel data. MIT press; 2010 Oct 1.
- 46. Anderson J. She Hunts Viral Rumors about Real Viruses. The New York Times. 2020 October 13. https://www.nytimes.com/2020/10/13/health/coronavirus-vaccine-hesitancy-larson.html .
- 47. Bump P. Coronavirus has come to Trump country. The Washington Post. 2020 June 17. https://www.washingtonpost.com/politics/2020/06/17/coronavirus-has-come-trump-country/ .
- 48. Quinn M. Fauci warns U.S. "unlikely" to reach Herd Immunity if too Many Refuse Vaccine. CBS News. 2020 June 29. https://www.cbsnews.com/news/fauci-herd-immunity-coronavirus-vaccine/ .
- 49. World Health Organization. Improving vaccination demand and addressing hesitancy. WHO; 2019. [cited 2020 November 3]. https://www.who.int/immunization/programmes_systems/vaccine_hesitancy/en/ .
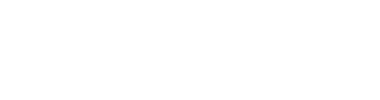
Verify originality of an essay
Get ideas for your paper
Find top study documents
150+ Medical research paper topics for students
Published 26 Jun 2024
Med Research Topics: What Makes a Good One?
Several essential attributes characterize an excellent medical research topic. First and foremost, it should address a significant and relevant issue within the medical field. The topic must have practical implications, contributing to advancing medical knowledge and improving patient care. For example, researching a new treatment for a prevalent disease or understanding the underlying mechanisms of a standard physical and mental health condition can provide valuable insights that can be applied in clinical practice.
Furthermore, a robust medical research topic should be specific and well-defined, allowing for a focused investigation. Vague or overly broad issues can make formulating a straightforward research question and designing a robust study challenging. Interested researchers can delve deeper into the subject matter by narrowing the scope and producing more detailed and meaningful findings. For instance, instead of broadly studying "cancer and treatment options," a more defined topic like "the efficacy of a specific immunotherapy in treating melanoma" would yield more actionable results.
Another critical aspect of an excellent medical research topic is feasibility. Researchers need to consider the availability of resources, such as funding, equipment, and expertise, as well as ethical considerations. The topic should be practical to study within the given constraints and timeframe. The feasibility of interesting topics also encompasses recruiting sufficient participants if human subjects are involved, ensuring that the study can be conducted effectively and ethically.
Lastly, a compelling medical research topic should be innovative and contribute new knowledge. It should challenge existing paradigms, explore uncharted areas, or offer new perspectives on established concepts. Innovation drives progress in medicine, leading to breakthroughs that can revolutionize patient care and improve patient outcomes. By choosing a topic that pushes the boundaries of current knowledge, healthcare researchers can make a lasting impact on the medical community and beyond.
How to Pick a Good Medical Research Paper Topic
Selecting a good medical research paper topic involves carefully considering several key factors. Firstly, choosing a topic that addresses a significant and current issue in the medical field is essential. One such issue is healthcare access, which is crucial in addressing inequities and barriers leading to health disparities and injustices. This ensures that the research will be relevant and contribute valuable knowledge to ongoing discussions and advancements in oral health elsewhere. Reviewing recent literature on medical research topics and identifying gaps in existing research can help pinpoint areas that need further exploration.
Next, the chosen topic should be specific and focused. A narrow scope allows for a more in-depth investigation and produces more detailed and actionable findings. For example, instead of a broad topic like "diabetes management," focusing on "the impact of a specific diet on blood sugar levels in Type 2 diabetes patients" can yield more precise and practical insights.
Feasibility is another crucial aspect to consider. Ensure that the necessary resources, including time, funding, and access to data or study participants, are available to complete the research effectively. Ethical considerations should be addressed, mainly when human subjects are involved. This involves obtaining the appropriate approvals and ensuring the study design protects participants' rights and well-being.
Lastly, the topic should be innovative and advance medical knowledge or practice. Aim to explore new perspectives, challenge existing assumptions, or investigate novel treatments or interventions. By selecting a topic that pushes the boundaries of understanding herbal medicine, researchers can significantly impact trends in the field and contribute to meaningful advancements in medicine.
150 medical research topics for college students
- Impact of lifestyle changes on hypertension management
- Genetic predispositions to heart disease
- Advances in minimally invasive heart surgery
- Role of diet in preventing cardiovascular diseases
- Efficacy of new anticoagulants in stroke prevention
- Long-term effects of statins on heart health
- Emerging treatments for congestive heart failure
- Non-invasive techniques for detecting coronary artery disease
- Impact of mental health on cardiac health
- Role of inflammation in atherosclerosis development
- Immunotherapy for advanced melanoma
- Genetic markers for early cancer detection
- Impact of diet and lifestyle on cancer prognosis
- New targeted therapies for breast cancer
- Role of microRNA in cancer progression
- Advances in radiotherapy for brain tumors
- Psychological support for cancer patients
- Personalized medicine in oncology
- Impact of environmental toxins on cancer incidence
- Survivorship and quality of life post-cancer treatment
- New treatments for Alzheimer's disease
- Role of genetics in multiple sclerosis
- Advances in the understanding of Parkinson's disease
- Impact of sleep disorders on neurological health
- Efficacy of new migraine treatments
- Neuroplasticity in stroke recovery
- Role of gut microbiome in neurodegenerative diseases
- Emerging therapies for epilepsy
- Impact of chronic stress on brain health
- Non-pharmacological interventions for ADHD
- Vaccination and childhood disease prevention
- Impact of screen time on child development
- Pediatric obesity and associated health risks
- Advances in neonatal care
- Genetic disorders in children and early interventions
- Efficacy of behavioral therapies for autism
- Role of nutrition in childhood growth and development
- Preventing and treating pediatric asthma
- Long-term outcomes of premature birth
- Pediatric mental health and early intervention
Endocrinology
- New treatments for Type 1 diabetes
- Impact of thyroid disorders on overall health
- Advances in understanding insulin resistance
- Role of hormones in metabolic syndrome
- Long-term effects of hormone replacement therapy
- Efficacy of new medications for osteoporosis
- Relationship between stress and endocrine disorders
- Impact of endocrine disruptors on health
- Role of diet and exercise in managing diabetes
- Advances in adrenal gland disorder treatments
Infectious Diseases
- Impact of antibiotic resistance on public health
- New vaccines for emerging infectious diseases
- Role of climate change in disease spread
- Advances in HIV treatment and prevention
- Efficacy of antiviral therapies for hepatitis C
- Impact of global travel on infectious disease transmission
- Role of the microbiome in infection prevention
- Emerging zoonotic diseases
- Efficacy of new tuberculosis treatments
- Strategies for preventing hospital-acquired infections
- Advances in the treatment of depression
- Impact of social media on mental health
- Role of genetics in psychiatric disorders
- Efficacy of cognitive-behavioral therapy for anxiety
- Long-term effects of antipsychotic medications
- Role of Lifestyle changes in managing bipolar disorder
- Impact of trauma on mental health
- Emerging treatments for PTSD
- Role of neurobiology in addiction
- Efficacy of mindfulness-based therapies
- Insomnia effect on patients with mental health conditions
Public Health
- Impact of public health policies on smoking rates
- Role of community programs in obesity prevention
- Strategies for reducing health disparities
- Impact of urbanization on public health
- Efficacy of health education programs
- Role of public health in disaster preparedness
- Advances in global health initiatives
- Impact of socioeconomic status on health outcomes
- Role of vaccination in public health
- Efficacy of public health interventions for substance abuse
- Impact of public health regulations on social behavior and health outcomes amid COVID-19
Dermatology
- Advances in the treatment of psoriasis
- Role of diet in managing acne
- Efficacy of new therapies for eczema
- Impact of environmental factors on skin health
- Role of genetics in skin disorders
- Advances in melanoma detection
- Impact of skincare products on skin health
- Role of microbiome in skin diseases
- Efficacy of laser treatments for skin conditions
- Strategies for preventing skin cancer
Gastroenterology
- Impact of diet on gut health
- Advances in the treatment of inflammatory bowel disease
- Role of probiotics in digestive health
- Efficacy of new therapies for irritable bowel syndrome
- Effect of gut microbiome on overall health
- Role of genetics in gastrointestinal disorders
- Advances in colorectal cancer screening
- Efficacy of dietary interventions for celiac disease
- Impact of chronic stress on digestive health
- Strategies for managing liver diseases
- Impact of electronic health records on gastroenterology research
Pulmonology
- Advances in asthma management
- Role of genetics in lung diseases
- Impact of air pollution on respiratory health
- Efficacy of new treatments for COPD
- Role of Lifestyle changes in managing Sleep Apnea
- Advances in lung cancer treatment
- Effect of Smoking Cessation Programs
- Efficacy of pulmonary rehabilitation
- Role of diet in respiratory health
- Strategies for managing chronic bronchitis
- Advances in kidney transplant techniques
- Role of diet in managing kidney disease
- Impact of hypertension on kidney health
- Efficacy of new treatments for chronic kidney disease
- Role of genetics in nephrological disorders
- Advances in dialysis technology
- Impact of diabetes on kidney health
- Efficacy of lifestyle interventions for kidney stones
- Role of hydration in preventing kidney diseases
- Strategies for early detection of kidney disorders
Orthopedics
- Advances in joint replacement surgery
- Role of physical therapy in managing osteoarthritis
- Efficacy of new treatments for osteoporosis
- Impact of sports on musculoskeletal health
- Role of genetics in orthopedic disorders
- Advances in minimally invasive orthopedic surgery
- Efficacy of regenerative therapies for bone injuries
- Role of nutrition in bone health
- Strategies for preventing sports injuries
- Impact of aging on musculoskeletal health
Ophthalmology
- Advances in cataract surgery techniques
- Role of genetics in eye diseases
- Impact of screen time on vision health
- Efficacy of new treatments for glaucoma
- Role of diet in maintaining eye health
- Advances in retinal disease management
- Efficacy of laser eye surgery
- Strategies for preventing macular degeneration
- Role of lifestyle changes in managing dry eye syndrome
- Impact of environmental factors on eye health
Rheumatology
- Advances in the treatment of rheumatoid arthritis
- Role of genetics in autoimmune disorders
- Efficacy of new therapies for lupus
- Impact of diet on inflammatory conditions
- Role of physical activity in managing arthritis
- Advances in understanding fibromyalgia
- Efficacy of biologic drugs in rheumatology
- Impact of chronic inflammation on overall health
- Strategies for managing gout
- Role of complementary therapies in rheumatic diseases
Selecting a Medical Research Paper Topic
Choosing a good medical research paper topic is a critical first step in the research process, influencing the direction and impact of the study. A well-chosen topic should address a significant and current issue within the medical field, ensuring relevance and the potential to contribute valuable insights to ongoing medical discussions and advancements. By focusing research projects on areas of primary care that need further exploration, researchers can make meaningful contributions to the body of medical knowledge.
A specific and well-defined topic allows for a focused investigation, leading to detailed and actionable findings for future medical students and others. Narrowing the scope helps researchers delve deeper into the subject matter, enhancing the quality and precision of the research. This approach benefits the study and ensures that the results are practical and applicable in real-world medical scenarios. Additionally, the feasibility of the topic, considering available resources and ethical considerations, is crucial for completing the research.
Innovative research topics that push the boundaries of current understanding are essential for driving progress in medicine. Researchers can significantly impact the field by exploring new perspectives, challenging existing assumptions, and investigating novel treatments or interventions for chronic diseases. Such groundbreaking research has the potential to revolutionize patient care and improve health outcomes on a broader scale.
If you're embarking on your medical research journey, start by identifying a topic that not only fascinates you but also meets the criteria of significance, specificity, feasibility, and innovation. Dive into current literature, consult with experts, and consider the practical implications of your research. By choosing a compelling topic, you'll set the stage for a successful and impactful study that can contribute to advancing medical science and improving patient care. Don't hesitate to seek guidance and support from your peers and mentors throughout this process, as collaboration and feedback are invaluable in refining your research focus.
Was this helpful?
Thanks for your feedback.

Written by David Kidwell
David is one of those experienced content creators from the United Kingdom who has a high interest in social issues, culture, and entrepreneurship. He always says that reading, blogging, and staying aware of what happens in the world is what makes a person responsible. He likes to learn and share what he knows by making things inspiring and creative enough even for those students who dislike reading.
Related Blog Posts
100 qualitative research topics to impress your teacher.
Qualitative research is a method of inquiry employed in various academic disciplines, traditionally in the social sciences, but also in market rese...
Join our 150K of happy users
- Get original papers written according to your instructions
- Save time for what matters most
- Health Tech
- Health Insurance
- Medical Devices
- Gene Therapy
- Neuroscience
- H5N1 Bird Flu
- Health Disparities
- Infectious Disease
- Mental Health
- Cardiovascular Disease
- Chronic Disease
- Alzheimer's
- Coercive Care
- The Obesity Revolution
- The War on Recovery
- Adam Feuerstein
- Matthew Herper
- Jennifer Adaeze Okwerekwu
- Ed Silverman
- CRISPR Tracker
- Breakthrough Device Tracker
- Generative AI Tracker
- Obesity Drug Tracker
- 2024 STAT Summit
- Wunderkinds Nomination
- STAT Madness
- STAT Brand Studio
Don't miss out
Subscribe to STAT+ today, for the best life sciences journalism in the industry
Journal retracts study tied to Micronoma’s effort to create cancer blood test

By Angus Chen June 26, 2024

N ature retracted a high-profile paper that served as part of the scientific groundwork for Micronoma, a San Diego-based startup, on Wednesday.
“Some of the findings of the article are affected and the corresponding conclusions are no longer supported,” Nature said in the retraction.
advertisement
The retraction comes after a team of researchers published work on the preprint site bioRxiv critiquing the Nature paper last August, describing what they called “fatal errors” in the paper’s analyses. STAT covered the criticism shortly after the team’s manuscript went live on bioRxiv. This same work was later published in the peer-reviewed journal mBio .
STAT+ Exclusive Story
Already have an account? Log in

This article is exclusive to STAT+ subscribers
Unlock this article — plus in-depth analysis, newsletters, premium events, and networking platform access..
Totals $468 per year
for 3 months, then $39/month
Then $39/month
Savings start at 25%!
Annually per user
$300 Annually per user
Get unlimited access to award-winning journalism and exclusive events.
About the Author Reprints
Cancer Reporter
Angus Chen covers all issues broadly related to cancer including drugs, policy, science, and equity. He joined STAT in 2021 after covering health and science at NPR and NPR affiliate stations. His work has been recognized by national Edward R. Murrow awards, the June L. Biedler prize for cancer journalism, and more.
biotechnology
diagnostics
STAT encourages you to share your voice. We welcome your commentary, criticism, and expertise on our subscriber-only platform, STAT+ Connect
To submit a correction request, please visit our Contact Us page .

Recommended

Recommended Stories

CDC advisory panel opts for a go-slow approach on expanding usage of RSV vaccines

FDA issues long-awaited draft guidance for enrolling more people of color in clinical trials

STAT Plus: A primer on HELIOS-B, an enormous stock-moving event for Alnylam Pharmaceuticals


STAT Plus: Top FDA official Peter Marks overruled staff, review team to approve Sarepta gene therapy

STAT Plus: What the Sarepta decision means for Duchenne patients, the company, and the FDA
- Fact sheets
- Facts in pictures
- Publications
- Questions and answers
- Tools and toolkits
- HIV and AIDS
- Hypertension
- Mental disorders
- Top 10 causes of death
- All countries
- Eastern Mediterranean
- South-East Asia
- Western Pacific
- Data by country
- Country presence
- Country strengthening
- Country cooperation strategies
- News releases
- Feature stories
- Press conferences
- Commentaries
- Photo library
- Afghanistan
- Cholera
- Coronavirus disease (COVID-19)
- Greater Horn of Africa
- Israel and occupied Palestinian territory
- Disease Outbreak News
- Situation reports
- Weekly Epidemiological Record
- Surveillance
- Health emergency appeal
- International Health Regulations
- Independent Oversight and Advisory Committee
- Classifications
- Data collections
- Global Health Estimates
- Mortality Database
- Sustainable Development Goals
- Health Inequality Monitor
- Global Progress
- Data collection tools
- Global Health Observatory
- Insights and visualizations
- COVID excess deaths
- World Health Statistics
- Partnerships
- Committees and advisory groups
- Collaborating centres
- Technical teams
- Organizational structure
- Initiatives
- General Programme of Work
- WHO Academy
- Investment in WHO
- WHO Foundation
- External audit
- Financial statements
- Internal audit and investigations
- Programme Budget
- Results reports
- Governing bodies
- World Health Assembly
- Executive Board
- Member States Portal
2024 Preferred Product Characteristics (PPC) for next-generation influenza vaccines – public consultation
Deadline for submission: 31 july 2024.
Influenza remains a significant global health concern a century after the 1918 pandemic, causing substantial morbidity and mortality every year, especially among vulnerable populations such as older adults, children, pregnant women, and those with underlying health conditions. The threat of pandemic influenza persists, including the ongoing risk of introduction of new zoonotic influenza viruses.
World Health Organization (WHO) guidance underscores the importance of influenza vaccination as a critical tool against both seasonal and pandemic influenza. The Global Influenza Strategy 2019–2030 emphasizes the role of seasonal influenza vaccination in reducing associated morbidity and mortality while strengthening preparedness for pandemic response. WHO also promotes a life-course approach to vaccination through the Immunization Agenda 2030; influenza vaccines are a key example of vaccines that should be available throughout the course of life.
Although current seasonal influenza vaccines are safe and reduce influenza-related illness, their effectiveness varies, particularly among older adults, and their protection is of limited duration, necessitating annual administration. Acknowledging these limitations, the Global Influenza Strategy prioritizes the development of improved, novel influenza vaccines with better breadth of and/or longer-laster protection.
To guide the development of next-generation influenza vaccines, WHO published Preferred Product Characteristics (PPCs) in 2017, aiming to encourage innovation and address global public health needs. While developed for global use, it is highly desirable that next-generation influenza vaccines would also be particularly suitable and available for use in low- and middle-income countries (LMICs). The PPCs provide early guidance for vaccine development, considering factors such as safety, efficacy, programmatic suitability, and access.
A revised version of the PPCs has been issued, reflecting advancements in influenza vaccine research, updated WHO guidance, and lessons from the COVID-19 pandemic. These PPCs focus on developing safe, more efficacious influenza vaccines that offer greater breadth and prolonged protection beyond a single year and are suitable for programmatic use in LMICs.
WHO is seeking feedback on the PPC from experts in the industry, product developers, the scientific community, national infection control programme personnel and clinicians currently involved in the management and control of influenza.
Details of the TPP may be found in the linked document:
- “ 2024 PPC FluDraft For Public Comment ”
Proposed revisions arising from the public consultation will be considered by the PPC working group before it is finalized.
If you have any comments, please submit them to [email protected] using the Comment Form
- “ Comment Form FluVx ”
Deadline for submission of comments: 31 July 2024

An official website of the United States government
The .gov means it’s official. Federal government websites often end in .gov or .mil. Before sharing sensitive information, make sure you’re on a federal government site.
The site is secure. The https:// ensures that you are connecting to the official website and that any information you provide is encrypted and transmitted securely.
- Publications
- Account settings
Preview improvements coming to the PMC website in October 2024. Learn More or Try it out now .
- Advanced Search
- Journal List
- Proc Natl Acad Sci U S A
- v.114(16); 2017 Apr 18

Simply put: Vaccination saves lives
Walter a. orenstein.
a Department of Medicine, Emory Vaccine Center, Emory University School of Medicine, Atlanta, GA, 30322;
b Department of Microbiology & Immunology, Emory Vaccine Center, Emory University School of Medicine, Atlanta, GA, 30322
Author contributions: W.A.O. and R.A. wrote the paper.
Few measures in public health can compare with the impact of vaccines. Vaccinations have reduced disease, disability, and death from a variety of infectious diseases. For example, in the United States, children are recommended to be vaccinated against 16 diseases ( 1 ). Table 1 highlights the impact in the United States of immunization against nine vaccine-preventable diseases, including smallpox and a complication of one of those diseases, congenital rubella syndrome, showing representative annual numbers of cases in the 20th century compared with 2016 reported cases ( 2 , 3 ). All of the diseases have been reduced by more than 90% and many have either been eliminated or reductions of 99% or more have been achieved. A recent analysis of vaccines to protect against 13 diseases estimated that for a single birth cohort nearly 20 million cases of diseases were prevented, including over 40,000 deaths ( 4 ). In addition to saving the lives of our children, vaccination has resulted in net economic benefits to society amounting to almost $69 billion in the United States alone. A recent economic analysis of 10 vaccines for 94 low- and middle-income countries estimated that an investment of $34 billion for the immunization programs resulted in savings of $586 billion in reducing costs of illness and $1.53 trillion when broader economic benefits were included ( 5 ). The only human disease ever eradicated, smallpox, was eradicated using a vaccine, and a second, polio, is near eradication, also using vaccines ( 6 , 7 ).
Comparison of 20th century annual morbidity and current estimates vaccine-preventable diseases
Disease | 20th Century annual morbidity ( ) | 2016 Reported cases ( ) | Percent decrease (%) |
Smallpox | 29,005 | 0 | 100 |
Diphtheria | 21,053 | 0 | 100 |
Measles | 530,217 | 69 | >99 |
Mumps | 162,344 | 5,311 | 97 |
Pertussis | 200,752 | 15,737 | 92 |
Polio (paralytic) | 16,316 | 0 | 100 |
Rubella | 47,745 | 5 | >99 |
Congenital rubella syndrome | 152 | 1 | 99 |
Tetanus | 580 | 33 | 94 |
20,000 | 22 | >99 |
Vaccines not only provide individual protection for those persons who are vaccinated, they can also provide community protection by reducing the spread of disease within a population ( Fig. 1 ). Person-to-person infection is spread when a transmitting case comes in contact with a susceptible person. If the transmitting case only comes in contact with immune individuals, then the infection does not spread beyond the index case and is rapidly controlled within the population. Interestingly, this chain of human-to-human transmission can be interrupted, even if there is not 100% immunity, because transmitting cases do not have infinite contacts; this is referred to as “herd immunity” or “community protection,” and is an important benefit of vaccination.

( A ) A highly susceptible population in which a transmitting case is likely to come in contact with a susceptible person leading to a chain of person-to-person transmission. ( B ) A highly immune population in which a transmitting case is unlikely to come in contact with a susceptible person, thereby breaking the chain of transmission and achieving indirect protection of remaining susceptibles because they are not exposed.
Mathematical modelers can estimate on average how many persons the typical transmitting case is capable of infecting if all of the contacts were susceptible (i.e., a population of 100% susceptibility). This number is known as R 0 , or the basic reproductive number. The immunity threshold needed within the population for terminating transmission can be calculated in percent as ( R 0 − 1)/ R 0 × 100 and is a guide to setting immunity levels and vaccination coverage targets for various diseases ( 8 ). For example, measles is one of the most contagious of vaccine-preventable diseases, with an estimated immunity threshold of 92–94%. In contrast, the protection threshold for rubella is estimated at 83–85%. Thus, eliminating rubella transmission is easier than measles, and when there are gaps in immunization coverage leading to accumulation of susceptibles, measles is often the first vaccine-preventable disease identified. Because of community protection induced by vaccines, persons who cannot be vaccinated (e.g., have contraindications or are younger than the age for whom vaccines are recommended), as well as persons who fail to make an adequate immune response to the vaccine (although most vaccines are highly effective, they are not 100% effective), can be protected indirectly because they are not exposed ( Fig. 1 ). Thus, for most vaccines, achieving high levels of coverage is important not only for individual protection but in preventing disease in vulnerable populations that cannot be directly protected by vaccination. This provides the rationale for interventions to achieve high population immunity, such as removing barriers that may prevent access to vaccines (e.g., providing recommended vaccines without cost), as well as mandates for immunization requirements for attending school ( 9 ). There are many reasons why vaccinations may not be received as recommended. One extreme is outright opposition to vaccines. Probably even more common may be that making the effort to receive vaccines (e.g., making the healthcare visits at the appropriate time so vaccines can be administered) may be a low priority compared with other issues, so in the absence of having a mandate for vaccination, other things take priority. Thus, appropriate mandates could help in making vaccination a priority for all ( 10 ).
It’s often said that vaccines save lives, but this is not strictly true; it is vaccination that saves lives. A vaccine that remains in the vial is 0% effective even if it is the best vaccine in the world. Thus, it is imperative that we all work together to assure that a high level of coverage is obtained among populations for whom vaccines are recommended. In some sense, vaccines have become victims of their own success. Diseases that once induced fear and sparked desire for vaccines are now rare, and there is a false and dangerous sense of complacency among the public.
In addition, in recent years, growing numbers of persons have become hesitant about vaccines, fearing side effects and not appreciative of the enormous health and economic benefits that vaccines provide. A CDC report on 159 measles cases reported between January 4 and April 2, 2015, showed that 68 United States residents with measles were unvaccinated, and of these 29 (43%) cited philosophical or religious objections to vaccination ( 11 ). A 2014 national web-based poll of parents in the United States estimated that 90.8% (89.3–92.1%) reported accepting or planning to accept all recommended noninfluenza childhood vaccines, 5.6% (4.6–6.9%) reported intentionally delaying one or more, and 3.6% (2.8–4.5%) reported refusing one or more vaccines ( 12 ). A national survey of pediatricians in the United States reported that the proportion of pediatricians reporting parental vaccine refusals increased from 74.5% in 2006 to 87.0% in 2013 ( 13 ). A 67-country survey on the state of vaccine confidence reported an average of 5.8% of respondents globally were skeptical about the importance of vaccines, with that proportion rising to more than 15% in some countries ( 14 ). One of the major concerns in recent years has been the allegations that vaccines can cause autism. There are three major theories advanced on the role of vaccines in causing autism: ( i ) measles, mumps, rubella vaccine (MMR); ( ii ) thimerosal, an ethyl mercury containing preservative in many vaccines in the United States in the past, now mostly out of vaccines recommended for children; and ( iii ) too many vaccines ( 15 ). There have been multiple well-conducted studies and independent reviews of those studies by the Institute of Medicine (now the National Academy of Medicine) that do not support a role for vaccines in causing autism ( 16 ). Independent evaluation of the safety of the immunization schedule has found it to be extremely safe ( 17 ). However, translating the science into information capable of influencing vaccine skeptics has been difficult.
The National Vaccine Advisory Committee (NVAC) in the United States issued a report in 2015, with 23 recommendations to assure high levels of vaccine confidence ( 18 ). The recommendations have five focus areas: ( i ) measuring and tracking vaccine confidence, ( ii ) communication and community strategies to increase vaccine confidence, ( iii ) healthcare provider strategies to increase vaccine confidence, ( iv ) policy strategies to increase vaccine confidence, and ( v ) continued support and monitoring of the state of vaccine confidence. Critical to assuring confidence is evidence-based research to evaluate which interventions are most effective. The NVAC recommended that a repository of evidence-based best practices for informing, educating, and communicating with parents and others in ways that foster or increase vaccine confidence be created. And while we have focused on children, vaccine preventable diseases exact a substantial health burden in adults and immunization coverage rates for most recommended vaccines are substantially lower for adults than those achieved for recommended vaccines in children. Thus, there is need not only in enhancing immunization rates in children but also in adults.
In summary, vaccines are some of the most effective and also cost-effective prevention tools we have. But vaccines that are not administered to persons for whom they are recommended are not useful. It is incumbent upon all of us who work in the healthcare setting, as well as community leaders, to stress to our friends and colleagues the importance of vaccination both for the individual vaccinated as well as for the communities in which the individuals live. Also critically important, there remains an urgent need for greater emphasis on research to develop vaccines for global diseases for which vaccines either do not exist or need improvement.
Acknowledgments
The authors thank Dianne Miller, Ali Ellebedy, and Sandra Roush for their assistance in preparation of the manuscript.
See Perspective on page 4055 .
share this!
June 24, 2024
This article has been reviewed according to Science X's editorial process and policies . Editors have highlighted the following attributes while ensuring the content's credibility:
fact-checked
peer-reviewed publication
trusted source
New research uncovers hidden phenomena in ultra-clean quantum materials
by Forschungsverbund Berlin e.V. (FVB)
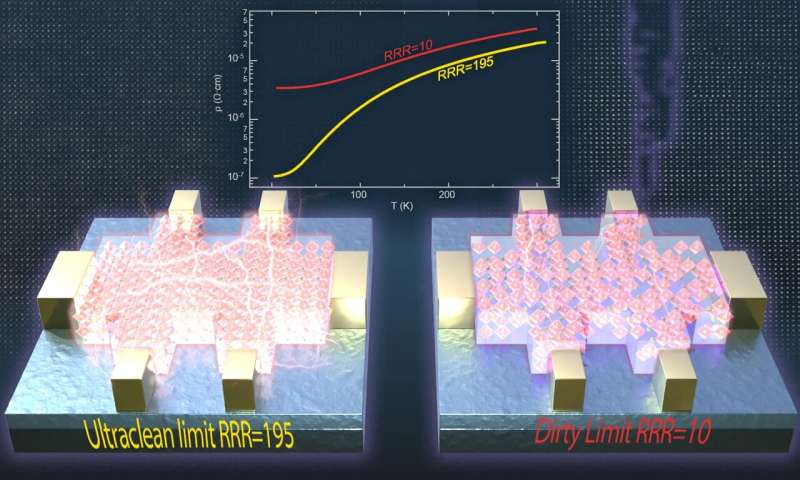
In a paper published today in Nature Communications , researchers unveiled previously unobserved phenomena in an ultra-clean sample of the correlated metal SrVO 3 . The study offers experimental insights that challenge the prevailing theoretical models of these unusual metals.
The international research team—from the Paul Drude Institute of Solid State Electronics (PDI), Germany; Oak Ridge National Laboratory (ORNL); Pennsylvania State University; University of Pittsburgh; the Pittsburgh Quantum Institute; and University of Minnesota—believes their findings will prompt a re-evaluation of current theories on electron correlation effects, shedding light on the origins of valuable phenomena in these systems, including magnetic properties , high-temperature superconductivity , and the unique characteristics of highly unusual transparent metals.
The perovskite oxide material SrVO 3 is classified as a Fermi liquid—a state describing a system of interacting electrons in a metal at sufficiently low temperatures.
In conventional metals, electrons that conduct electricity move independently, commonly referred to as a Fermi gas. In contrast, Fermi liquids feature significant mutual interactions between electrons, meaning the motion of one electron strongly influences the others. This collective behavior can lead to unique electronic properties with profound technological applications, providing insights into the interactions between electrons in correlated metals.
SrVO 3 serves as an ideal model system for studying electron correlation phenomena due to its crystalline and electronic simplicity. This simplicity is crucial for understanding complex phenomena such as magnetic order or superconductivity, which can complicate theoretical and experimental studies.
Another crucial factor in understanding experimental results that guide theoretical models for electron correlation effects is the presence or absence of defects in the material itself. Dr. Roman Engel-Herbert, study lead and Director of PDI in Berlin, said, "If you want to get to the bottom of one of the best-kept secrets in condensed matter physics, then you must study it in its purest form; in the absence of any extrinsic disturbance. High-quality materials that are virtually defect-free are essential. You need to synthesize ultra-clean materials."
Achieving a defect-free sample of SrVO 3 has been a seemingly insurmountable challenge until now. By employing an innovative thin film growth technique that combines the advantages of molecular beam epitaxy and chemical vapor deposition , the team achieved an unprecedented level of material purity.
Dr. Matt Brahlek, first author of the study, quantifies the improvement: "A simple measure of material purity is the ratio of how easily electricity flows at room temperature compared to low temperature, called the residual resistivity ratio, RRR value. If the metal contains many defects, RRR values are low, typically around 2–5.
"We have been able to synthesize SrVO 3 films with RRR nearly 100 times larger, 200, opening the door to study the true properties of the correlated metal SrVO 3 . In particular, the high material quality allowed accessing special regime at high magnetic fields for the first time, where surprises were found."
The interdisciplinary team of scientists was surprised to discover a series of peculiar transport phenomena that were in sharp contrast to the transport properties measured previously on highly defective samples. Their findings challenge the long-standing scientific consensus regarding SrVO 3 as a simple Fermi liquid.
Engel-Herbert explains, "This situation was very exciting but also puzzling. While we reproduced previously reported transport behavior of SrVO 3 in our highly defective samples, identical measurements in ultraclean samples with high RRR values differed."
Results from defective samples allowed a straight-forward interpretation of the results that matched theoretical expectation. These results were used as experimental evidence that the theoretical understanding correctly captured the electron correlation effects in SrVO 3 . However, the team found that measurements on the ultraclean samples could not be explained so easily.
Brahlek added, "An observation that stands out is the expectation that the number of electrons that carry electricity in a metal is independent of temperature and magnetic field. This is of course true, but the interpretation of the measured quantity is not a direct measure of the carrier concentration.
"Rather, this quantity is mixed up with other aspects of the material properties, such as how defects and temperature impact the flow of electricity. We had to delve deeper into the physics to understand what we saw. That is what makes it so important and exciting."
The researchers believe their discovery can serve as a basis to refine theoretical models and prompt a re-examination of established views and interpretations of materials exhibiting a sizeable electron correlation.
Engel-Herbert says, "Our job as experimental physicists is to push beyond the boundaries of the current understanding of nature. This is where discoveries can be made, where we advance science. As condensed matter physicists, it is key to keep perfecting our object of study by challenging ourselves to push the limits of perfecting materials.
"This can potentially give new insights into the true behavior of this class of materials and enables a comprehensive explanation of the phenomena measured and observed. It takes an interdisciplinary team of experts to do this.
"While the job is not yet completed, our results are an opportunity for the community to recalibrate their theories; re-examining materials we believed were well-understood and re-evaluate their potential for applications."
Journal information: Nature Communications
Provided by Forschungsverbund Berlin e.V. (FVB)
Explore further
Feedback to editors

Early childhood problems linked to persistent school absenteeism
7 hours ago

Researchers find genetic stability in a long-term Panamanian hybrid zone of manakins
10 hours ago

Detective work enables Perseverance Mars rover team to revive SHERLOC instrument

NASA's Juno probe gets a close-up look at lava lakes on Jupiter's moon Io

Simple new process stores carbon dioxide in concrete without compromising strength

Surprising phosphate finding in NASA's OSIRIS-REx asteroid sample
11 hours ago

First case of Down syndrome in Neanderthals documented in new study

Understanding quantum states: New research shows importance of precise topography in solid neon qubits

New study reveals comet airburst evidence from 12,800 years ago

Time-compression in electron microscopy: Terahertz light controls and characterizes electrons in space and time
12 hours ago
Relevant PhysicsForums posts
Bulk and slab electronic structure differences.
18 hours ago
Gap Equations Plotting Error in Python: Need Help Debugging
Jun 20, 2024
How Do You Solve Harper's Equation in Quantum Mechanics?
Jun 3, 2024
Latest explanation for "stability of high multiplicity states"
May 28, 2024
How to do Convergent-Close Coupling (CCC)
May 24, 2024
Anyone knows the slow light in EIT?
May 22, 2024
More from Atomic and Condensed Matter
Related Stories

How a transparent conductor responds to strain
Aug 4, 2023

Researchers show an old law still holds for quirky quantum materials
Nov 30, 2023

Quantum materials: A new state of matter with chiral properties
Feb 7, 2024

Thanks to trapped electrons, a material expected to be a conducting metal remains an insulator
Jul 14, 2023

Scientists identify mechanism that explains the characteristic properties of 'strange metals'
Aug 17, 2023

A new material for light-matter interactions
Jun 26, 2020
Recommended for you

A high-temperature superconductor with zero resistance that exhibits strange metal behavior
Jun 25, 2024

Foregoing quantum chaos to achieve high-fidelity quantum state transfer

Researchers discover new flat electronic bands, paving way for advanced quantum materials

Quantum annealer improves understanding of quantum many-body systems

Quantum effects forbid the formation of black holes from high concentrations of intense light, say physicists
Jun 24, 2024
Let us know if there is a problem with our content
Use this form if you have come across a typo, inaccuracy or would like to send an edit request for the content on this page. For general inquiries, please use our contact form . For general feedback, use the public comments section below (please adhere to guidelines ).
Please select the most appropriate category to facilitate processing of your request
Thank you for taking time to provide your feedback to the editors.
Your feedback is important to us. However, we do not guarantee individual replies due to the high volume of messages.
E-mail the story
Your email address is used only to let the recipient know who sent the email. Neither your address nor the recipient's address will be used for any other purpose. The information you enter will appear in your e-mail message and is not retained by Phys.org in any form.
Newsletter sign up
Get weekly and/or daily updates delivered to your inbox. You can unsubscribe at any time and we'll never share your details to third parties.
More information Privacy policy
Donate and enjoy an ad-free experience
We keep our content available to everyone. Consider supporting Science X's mission by getting a premium account.
E-mail newsletter
Thank you for visiting nature.com. You are using a browser version with limited support for CSS. To obtain the best experience, we recommend you use a more up to date browser (or turn off compatibility mode in Internet Explorer). In the meantime, to ensure continued support, we are displaying the site without styles and JavaScript.
- View all journals
- Explore content
- About the journal
- Publish with us
- Sign up for alerts
- Open access
- Published: 19 June 2024
Detecting hallucinations in large language models using semantic entropy
- Sebastian Farquhar ORCID: orcid.org/0000-0002-9185-6415 1 na1 ,
- Jannik Kossen 1 na1 ,
- Lorenz Kuhn 1 na1 &
- Yarin Gal ORCID: orcid.org/0000-0002-2733-2078 1
Nature volume 630 , pages 625–630 ( 2024 ) Cite this article
57k Accesses
1456 Altmetric
Metrics details
- Computer science
- Information technology
Large language model (LLM) systems, such as ChatGPT 1 or Gemini 2 , can show impressive reasoning and question-answering capabilities but often ‘hallucinate’ false outputs and unsubstantiated answers 3 , 4 . Answering unreliably or without the necessary information prevents adoption in diverse fields, with problems including fabrication of legal precedents 5 or untrue facts in news articles 6 and even posing a risk to human life in medical domains such as radiology 7 . Encouraging truthfulness through supervision or reinforcement has been only partially successful 8 . Researchers need a general method for detecting hallucinations in LLMs that works even with new and unseen questions to which humans might not know the answer. Here we develop new methods grounded in statistics, proposing entropy-based uncertainty estimators for LLMs to detect a subset of hallucinations—confabulations—which are arbitrary and incorrect generations. Our method addresses the fact that one idea can be expressed in many ways by computing uncertainty at the level of meaning rather than specific sequences of words. Our method works across datasets and tasks without a priori knowledge of the task, requires no task-specific data and robustly generalizes to new tasks not seen before. By detecting when a prompt is likely to produce a confabulation, our method helps users understand when they must take extra care with LLMs and opens up new possibilities for using LLMs that are otherwise prevented by their unreliability.
Similar content being viewed by others
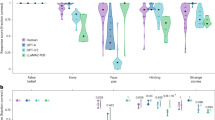
Testing theory of mind in large language models and humans
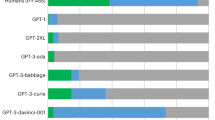
Human-like intuitive behavior and reasoning biases emerged in large language models but disappeared in ChatGPT
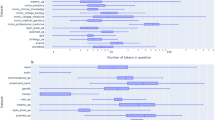
ThoughtSource: A central hub for large language model reasoning data
‘Hallucinations’ are a critical problem 9 for natural language generation systems using large language models (LLMs), such as ChatGPT 1 or Gemini 2 , because users cannot trust that any given output is correct.
Hallucinations are often defined as LLMs generating “content that is nonsensical or unfaithful to the provided source content” 9 , 10 , 11 but they have come to include a vast array of failures of faithfulness and factuality. We focus on a subset of hallucinations which we call ‘confabulations’ 12 for which LLMs fluently make claims that are both wrong and arbitrary—by which we mean that the answer is sensitive to irrelevant details such as random seed. For example, when asked a medical question “What is the target of Sotorasib?” an LLM confabulates by sometimes answering KRASG12 ‘C’ (correct) and other times KRASG12 ‘D’ (incorrect) despite identical instructions. We distinguish this from cases in which a similar ‘symptom’ is caused by the following different mechanisms: when LLMs are consistently wrong as a result of being trained on erroneous data such as common misconceptions 13 ; when the LLM ‘lies’ in pursuit of a reward 14 ; or systematic failures of reasoning or generalization. We believe that combining these distinct mechanisms in the broad category hallucination is unhelpful. Our method makes progress on a portion of the problem of providing scalable oversight 15 by detecting confabulations that people might otherwise find plausible. However, it does not guarantee factuality because it does not help when LLM outputs are systematically bad. Nevertheless, we significantly improve question-answering accuracy for state-of-the-art LLMs, revealing that confabulations are a great source of error at present.
We show how to detect confabulations by developing a quantitative measure of when an input is likely to cause an LLM to generate arbitrary and ungrounded answers. Detecting confabulations allows systems built on LLMs to avoid answering questions likely to cause confabulations, to make users aware of the unreliability of answers to a question or to supplement the LLM with more grounded search or retrieval. This is essential for the critical emerging field of free-form generation in which naive approaches, suited to closed vocabulary and multiple choice, fail. Past work on uncertainty for LLMs has focused on simpler settings, such as classifiers 16 , 17 and regressors 18 , 19 , whereas the most exciting applications of LLMs relate to free-form generations.
The term hallucination in the context of machine learning originally comes from filling in ungrounded details, either as a deliberate strategy 20 or as a reliability problem 4 . The appropriateness of the metaphor has been questioned as promoting undue anthropomorphism 21 . Although we agree that metaphor must be used carefully with LLMs 22 , the widespread adoption of the term hallucination reflects the fact that it points to an important phenomenon. This work represents a step towards making that phenomenon more precise.
To detect confabulations, we use probabilistic tools to define and then measure the ‘semantic’ entropy of the generations of an LLM—an entropy that is computed over meanings of sentences. High entropy corresponds to high uncertainty 23 , 24 , 25 —so semantic entropy is one way to estimate semantic uncertainties. Semantic uncertainty, the broader category of measures we introduce, could be operationalized with other measures of uncertainty, such as mutual information, instead. Entropy in free-form generation is normally hard to measure because answers might mean the same thing (be semantically equivalent) despite being expressed differently (being syntactically or lexically distinct). This causes naive estimates of entropy or other lexical variation scores 26 to be misleadingly high when the same correct answer might be written in many ways without changing its meaning.
By contrast, our semantic entropy moves towards estimating the entropy of the distribution of meanings of free-form answers to questions, insofar as that is possible, rather than the distribution over the ‘tokens’ (words or word-pieces) which LLMs natively represent. This can be seen as a kind of semantic consistency check 27 for random seed variation. An overview of our approach is provided in Fig. 1 and a worked example in Supplementary Table 1 .
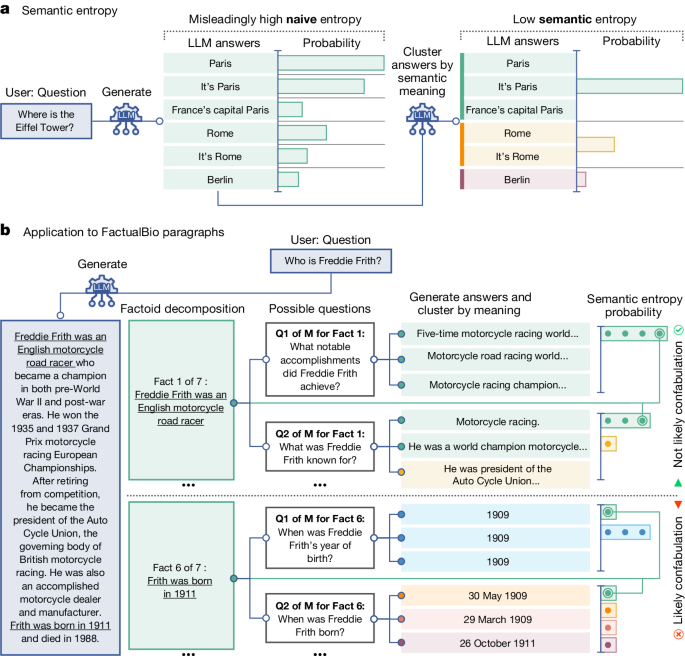
a , Naive entropy-based uncertainty measures variation in the exact answers, treating ‘Paris’, ‘It’s Paris’ and ‘France’s capital Paris’ as different. But this is unsuitable for language tasks for which sometimes different answers mean the same things. Our semantic entropy clusters answers which share meanings before computing the entropy. A low semantic entropy shows that the LLM is confident about the meaning. b , Semantic entropy can also detect confabulations in longer passages. We automatically decompose a long generated answer into factoids. For each factoid, an LLM generates questions to which that factoid might have been the answer. The original LLM then samples M possible answers to these questions. Finally, we compute the semantic entropy over the answers to each specific question, including the original factoid. Confabulations are indicated by high average semantic entropy for questions associated with that factoid. Here, semantic entropy classifies Fact 1 as probably not a confabulation because generations often mean the same thing, despite very different wordings, which a naive entropy would have missed.
Intuitively, our method works by sampling several possible answers to each question and clustering them algorithmically into answers that have similar meanings, which we determine on the basis of whether answers in the same cluster entail each other bidirectionally 28 . That is, if sentence A entails that sentence B is true and vice versa, then we consider them to be in the same semantic cluster. We measure entailment using both general-purpose LLMs and natural language inference (NLI) tools developed specifically for detecting entailment for which we show direct evaluations in Supplementary Tables 2 and 3 and Supplementary Fig. 1 . Textual entailment has previously been shown to correlate with faithfulness 10 in the context of factual consistency 29 as well as being used to measure factuality in abstractive summarization 30 , especially when applied at the right granularity 31 .
Semantic entropy detects confabulations in free-form text generation across a range of language models and domains, without previous domain knowledge. Our evaluations cover question answering in trivia knowledge (TriviaQA 32 ), general knowledge (SQuAD 1.1; ref. 33 ), life sciences (BioASQ 34 ) and open-domain natural questions (NQ-Open 35 ) derived from actual queries to Google Search 36 . In addition, semantic entropy detects confabulations in mathematical word problems (SVAMP 37 ) and in a biography-generation dataset, FactualBio, accompanying this paper.
Our results for TriviaQA, SQuAD, BioASQ, NQ-Open and SVAMP are all evaluated context-free and involve sentence-length answers (96 ± 70 characters, mean ± s.d.) and use LLaMA 2 Chat (7B, 13B and 70B parameters) 38 , Falcon Instruct (7B and 40B) 39 and Mistral Instruct (7B) 40 . In the Supplementary Information , we further consider short-phrase-length answers. Results for FactualBio (442 ± 122 characters) use GPT-4 (ref. 1 ). At the time of writing, GPT-4 (ref. 1 ) did not expose output probabilities 41 or hidden states, although it does now. As a result, we propose a discrete approximation of our estimator for semantic entropy which allows us to run experiments without access to output probabilities, which we use for all GPT-4 results in this paper and which performs similarly well.
Our confabulation detection with semantic entropy is more robust to user inputs from previously unseen domains than methods which aim to ‘learn’ how to detect confabulations from a set of example demonstrations. Our method is unsupervised, meaning that we do not need labelled examples of confabulations. By contrast, supervised methods detect confabulations by learning patterns behind examples of confabulations, assuming that future questions preserve these patterns. But this assumption is often untrue in new situations or with confabulations that human overseers are unable to identify (compare Fig. 17 of ref. 24 ). As a strong supervised baseline, we compare to an embedding regression method inspired by ref. 24 which trains a logistic regression classifier to predict whether the model correctly answered a question on the basis of the final ‘embedding’ (hidden state) of the LLM. We also use the P (True) method 24 which looks at the probability with which an LLM predicts that the next token is ‘True’ when few-shot prompted to compare a main answer with ‘brainstormed’ alternatives.
Confabulations contribute substantially to incorrect answers given by language models. We show that semantic entropy can be used to predict many incorrect model answers and to improve question-answering accuracy by refusing to answer those questions the model is uncertain about. Corresponding to these two uses, we evaluate two main metrics. First, the widely used area under the receiver operating characteristic (AUROC) curve for the binary event that a given answer is incorrect. This measure captures both precision and recall and ranges from 0 to 1, with 1 representing a perfect classifier and 0.5 representing an un-informative classifier. We also show a new measure, the area under the ‘rejection accuracy’ curve (AURAC). This studies the case in which the confabulation detection score is used to refuse to answer the questions judged most likely to cause confabulations. Rejection accuracy is the accuracy of the answers of the model on the remaining questions and the area under this curve is a summary statistic over many thresholds (representative threshold accuracies are provided in Supplementary Material ). The AURAC captures the accuracy improvement which users would experience if semantic entropy was used to filter out questions causing the highest entropy.
Detecting confabulations in QA and math
In Fig. 2 , we show that both semantic entropy and its discrete approximation outperform our best baselines for sentence-length generations. These results are averaged across datasets and provide the actual scores on the held-out evaluation dataset. We report the raw average score across held-out evaluation datasets without standard error because the distributional characteristics are more a property of the models and datasets selected than the method. Consistency of relative results across different datasets is a stronger indicator of variation in this case.
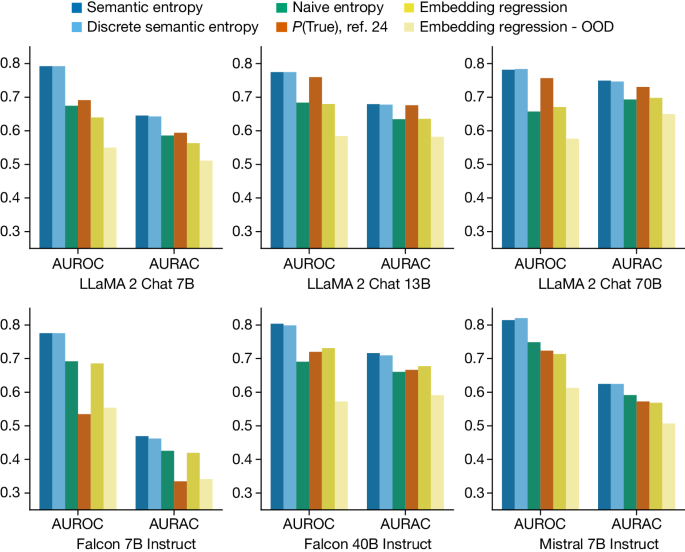
Semantic entropy outperforms leading baselines and naive entropy. AUROC (scored on the y -axes) measures how well methods predict LLM mistakes, which correlate with confabulations. AURAC (likewise scored on the y -axes) measures the performance improvement of a system that refuses to answer questions which are judged likely to cause confabulations. Results are an average over five datasets, with individual metrics provided in the Supplementary Information .
Semantic entropy greatly outperforms the naive estimation of uncertainty using entropy: computing the entropy of the length-normalized joint probability of the token sequences. Naive entropy estimation ignores the fact that token probabilities also express the uncertainty of the model over phrasings that do not change the meaning of an output.
Our methods also outperform the supervised embedding regression method both in- and out-of-distribution. In pale-yellow bars we show that embedding regression performance deteriorates when its training data do not match the deployment distribution—which mirrors the common real-world case in which there is a distribution shift between training and deployment 42 —the plotted value is the average metric for embedding regression trained on one of the four ‘off-distribution’ datasets for that evaluation. This is critical because reliable uncertainty is most important when the data distribution shifts. Semantic entropy also outperforms P (True) which is supervised ‘in-context’; that is, it is adapted to the deployment task with a few training examples provided in the LLM prompt itself. The discrete variant of semantic entropy performs similarly to our standard estimator, despite not requiring exact output probabilities.
Averaged across the 30 combinations of tasks and models we study, semantic entropy achieves the best AUROC value of 0.790 whereas naive entropy (0.691), P (True) (0.698) and the embedding regression baseline (0.687) lag behind it. Semantic entropy performs well consistently, with stable performance (between 0.78 and 0.81 AUROC) across the different model families (LLaMA, Falcon and Mistral) and scales (from 7B to 70B parameters) which we study (we report summary statistics for each dataset and model as before). Although semantic entropy outperforms the baselines across all model sizes, P (True) seems to improve with model size, suggesting that it might become more competitive for very capable honest models in settings that the model understands well (which are, however, not the most important cases to have good uncertainty). We use ten generations to compute entropy, selected using analysis in Supplementary Fig. 2 . Further results for short-phrase generations are described in Supplementary Figs. 7 – 10 .
The results in Fig. 2 offer a lower bound on the effectiveness of semantic entropy at detecting confabulations. These evaluations determine whether semantic entropy and baseline methods can detect when the answers of the model are incorrect (which we validate against human correctness evaluations in Supplementary Table 4 ). In addition to errors from confabulations (arbitrary incorrectness), this also includes other types of mistakes for which semantic entropy is not suited, such as consistent errors learned from the training data. The fact that methods such as embedding regression are able to spot other kinds of errors, not just confabulations, but still are outperformed by semantic entropy, suggests that confabulations are a principal category of errors for actual generations.
Examples of questions and answers from TriviaQA, SQuAD and BioASQ, for LLaMA 2 Chat 70B, are shown in Table 1 . These illustrate how only semantic entropy detects when the meaning is constant but the form varies (the first row of the table) whereas semantic entropy and naive entropy both correctly predict the presence of confabulations when the form and meaning vary together (second row) and predict the absence of confabulations when the form and meaning are both constant across several resampled generations (third row). In the final row, we give an example in which semantic entropy is erroneously high as a result of overly sensitive semantic clustering relative to the reference answer. Our clustering method distinguishes the answers which provide a precise date from those which only provide a year. For some contexts that would have been correct but in this context the distinction between the specific day and the year is probably irrelevant. This highlights the importance of context and judgement in clustering, especially in subtle cases, as well as the shortcomings of evaluating against fixed reference answers which do not capture the open-ended flexibility of conversational deployments of LLMs.
Detecting confabulations in biographies
Semantic entropy is most natural for sentences that express a single proposition but the idea of semantic equivalence is trickier to apply to longer passages which express many propositions which might only agree partially 43 . Nevertheless, we can use semantic entropy to detect confabulations in longer generations, such as entire paragraphs of text. To show this, we develop a dataset of biographical generations from GPT-4 (v.0613) for 21 individuals notable enough to have their own Wikipedia page but without extensive online biographies. From each biography generated by GPT-4, we automatically extract propositional factual claims about the individual (150 factual claims in total), which we manually label as true or false.
Applying semantic entropy to this problem is challenging. Naively, one might simply regenerate each sentence (conditioned on the text so far) and then compute semantic entropy over these regenerations. However, the resampled sentences often target different aspects of the biography: for example, one time describing family and the next time profession. This is analogous to the original problem semantic entropy was designed to resolve: the model is uncertain about the right ordering of facts, not about the facts themselves. To address this, we break down the entire paragraph into factual claims and reconstruct questions which might have been answered by those claims. Only then do we apply semantic entropy (Fig. 1 ) by generating three new answers to each question (selected with analysis in Supplementary Figs. 3 and 4 ) and computing the semantic entropy over those generations plus the original factual claim. We aggregate these by averaging the semantic entropy over all the questions to get an uncertainty score for each proposition, which we use to detect confabulations. Unaggregated results are shown in Supplementary Figs. 5 and 6 .
As GPT-4 did not allow access to the probability of the generation at the time of writing, we use a discrete variant of semantic entropy which makes the further approximation that we can infer a discrete empirical distribution over semantic meaning clusters from only the generations ( Methods ). This allows us to compute semantic entropy using only the black-box outputs of an LLM. However, we were unable to compute the naive entropy baseline, the standard semantic entropy estimator or the embedding regression baseline for GPT-4 without output probabilities and embeddings.
In Fig. 3 we show that the discrete variant of semantic entropy effectively detects confabulations on this dataset. Its AUROC and AURAC are higher than either a simple ‘self-check’ baseline—which just asks the LLM whether the factoid is likely to be true—or a variant of P (True) which has been adapted to work for the paragraph-length setting. Discrete semantic entropy has better rejection accuracy performance until 20% of the questions have been rejected at which point P (True) has a narrow edge. This indicates that the questions predicted to cause confabulations are indeed more likely to be wrong.
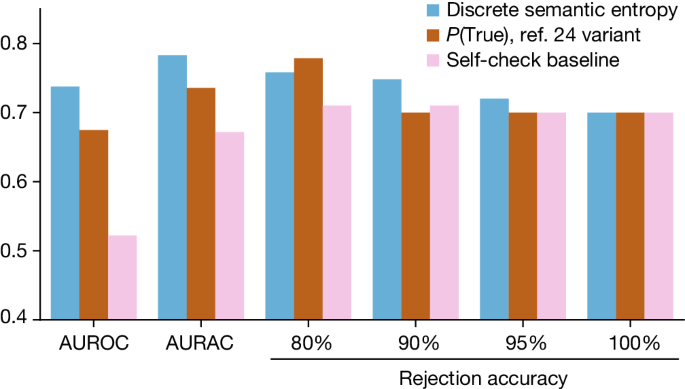
The discrete variant of our semantic entropy estimator outperforms baselines both when measured by AUROC and AURAC metrics (scored on the y -axis). The AUROC and AURAC are substantially higher than for both baselines. At above 80% of questions being answered, semantic entropy has the highest accuracy. Only when the top 20% of answers judged most likely to be confabulations are rejected does the answer accuracy on the remainder for the P (True) baseline exceed semantic entropy.
Our probabilistic approach, accounting for semantic equivalence, detects an important class of hallucinations: those that are caused by a lack of LLM knowledge. These are a substantial portion of the failures at present and will continue even as models grow in capabilities because situations and cases that humans cannot reliably supervise will persist. Confabulations are a particularly noteworthy failure mode for question answering but appear in other domains too. Semantic entropy needs no previous domain knowledge and we expect that algorithmic adaptations to other problems will allow similar advances in, for example, abstractive summarization. In addition, extensions to alternative input variations such as rephrasing or counterfactual scenarios would allow a similar method to act as a form of cross-examination 44 for scalable oversight through debate 45 .
The success of semantic entropy at detecting errors suggests that LLMs are even better at “knowing what they don’t know” than was argued by ref. 24 —they just don’t know they know what they don’t know. Our method explicitly does not directly address situations in which LLMs are confidently wrong because they have been trained with objectives that systematically produce dangerous behaviour, cause systematic reasoning errors or are systematically misleading the user. We believe that these represent different underlying mechanisms—despite similar ‘symptoms’—and need to be handled separately.
One exciting aspect of our approach is the way it makes use of classical probabilistic machine learning methods and adapts them to the unique properties of modern LLMs and free-form language generation. We hope to inspire a fruitful exchange of well-studied methods and emerging new problems by highlighting the importance of meaning when addressing language-based machine learning problems.
Semantic entropy as a strategy for overcoming confabulation builds on probabilistic tools for uncertainty estimation. It can be applied directly to any LLM or similar foundation model without requiring any modifications to the architecture. Our ‘discrete’ variant of semantic uncertainty can be applied even when the predicted probabilities for the generations are not available, for example, because access to the internals of the model is limited.
In this section we introduce background on probabilistic methods and uncertainty in machine learning, discuss how it applies to language models and then discuss our contribution, semantic entropy, in detail.
Uncertainty and machine learning
We aim to detect confabulations in LLMs, using the principle that the model will be uncertain about generations for which its output is going to be arbitrary.
One measure of uncertainty is the predictive entropy of the output distribution, which measures the information one has about the output given the input 25 . The predictive entropy (PE) for an input sentence x is the conditional entropy ( H ) of the output random variable Y with realization y given x ,
A low predictive entropy indicates an output distribution which is heavily concentrated whereas a high predictive entropy indicates that many possible outputs are similarly likely.
Aleatoric and epistemic uncertainty
We do not distinguish between aleatoric and epistemic uncertainty in our analysis. Researchers sometimes separate aleatoric uncertainty (uncertainty in the underlying data distribution) from epistemic uncertainty (caused by having only limited information) 46 . Further advances in uncertainty estimation which separate these kinds of uncertainty would enhance the potential for our semantic uncertainty approach by allowing extensions beyond entropy.
Joint probabilities of sequences of tokens
Generative LLMs produce strings of text by selecting tokens in sequence. Each token is a wordpiece that often represents three or four characters (though especially common sequences and important words such as numbers typically get their own token). To compute entropies, we need access to the probabilities the LLM assigns to the generated sequence of tokens. The probability of the entire sequence, s , conditioned on the context, x , is the product of the conditional probabilities of new tokens given past tokens, whose resulting log-probability is \(\log P({\bf{s}}| {\boldsymbol{x}})={\sum }_{i}\log P({s}_{i}| {{\bf{s}}}_{ < i},{\boldsymbol{x}})\) , where s i is the i th output token and s < i denotes the set of previous tokens.
Length normalization
When comparing the log-probabilities of generated sequences, we use ‘length normalization’, that is, we use an arithmetic mean log-probability, \(\frac{1}{N}{\sum }_{i}^{N}\log P({s}_{i}| {{\bf{s}}}_{ < i},{\boldsymbol{x}})\) , instead of the sum. In expectation, longer sequences have lower joint likelihoods because of the conditional independence of the token probabilities 47 . The joint likelihood of a sequence of length N shrinks exponentially in N . Its negative log-probability therefore grows linearly in N , so longer sentences tend to contribute more to entropy. We therefore interpret length-normalizing the log-probabilities when estimating the entropy as asserting that the expected uncertainty of generations is independent of sentence length. Length normalization has some empirical success 48 , including in our own preliminary experiments, but little theoretical justification in the literature.
Principles of semantic uncertainty
If we naively calculate the predictive entropy directly from the probabilities of the generated sequence of tokens, we conflate the uncertainty of the model over the meaning of its answer with the uncertainty over the exact tokens used to express that meaning. For example, even if the model is confident in the meaning of a generation, there are still usually many different ways for phrasing that generation without changing its meaning. For the purposes of detecting confabulations, the uncertainty of the LLM over meanings is more important than the uncertainty over the exact tokens used to express those meanings.
Our semantic uncertainty method therefore seeks to estimate only the uncertainty the LLM has over the meaning of its generation, not the choice of words. To do this, we introduce an algorithm that clusters model generations by meaning and subsequently calculates semantic uncertainty. At a high level this involves three steps:
Generation: sample output sequences of tokens from the predictive distribution of a LLM given a context x .
Clustering: cluster sequences by their meaning using our clustering algorithm based on bidirectional entailment.
Entropy estimation: estimate semantic entropy by summing probabilities of sequences that share a meaning following equation ( 2 ) and compute their entropy.
Generating a set of answers from the model
Given some context x as input to the LLM, we sample M sequences, { s (1) , …, s ( M ) } and record their token probabilities, { P ( s (1) ∣ x ), …, P ( s ( M ) ∣ x )}. We sample all our generations from a single model, varying only the random seed used for sampling from the token probabilities. We do not observe the method to be particularly sensitive to details of the sampling scheme. In our implementation, we sample at temperature 1 using nucleus sampling ( P = 0.9) (ref. 49 ) and top- K sampling ( K = 50) (ref. 50 ). We also sample a single generation at low temperature (0.1) as an estimate of the ‘best generation’ of the model to the context, which we use to assess the accuracy of the model. (A lower sampling temperature increases the probability of sampling the most likely tokens).
Clustering by semantic equivalence
To estimate semantic entropy we need to cluster generated outputs from the model into groups of outputs that mean the same thing as each other.
This can be described using ‘semantic equivalence’ which is the relation that holds between two sentences when they mean the same thing. We can formalize semantic equivalence mathematically. Let the space of tokens in a language be \({\mathcal{T}}\) . The space of all possible sequences of tokens of length N is then \({{\mathcal{S}}}_{N}\equiv {{\mathcal{T}}}^{N}\) . Note that N can be made arbitrarily large to accommodate whatever size of sentence one can imagine and one of the tokens can be a ‘padding’ token which occurs with certainty for each token after the end-of-sequence token. For some sentence \({\bf{s}}\in {{\mathcal{S}}}_{N}\) , composed of a sequence of tokens, \({s}_{i}\in {\mathcal{T}}\) , there is an associated meaning. Theories of meaning are contested 51 . However, for specific models and deployment contexts many considerations can be set aside. Care should be taken comparing very different models and contexts.
Let us introduce a semantic equivalence relation, E ( ⋅ , ⋅ ), which holds for any two sentences that mean the same thing—we will operationalize this presently. Recall that an equivalence relation is any reflexive, symmetric and transitive relation and that any equivalence relation on a set corresponds to a set of equivalence classes. Each semantic equivalence class captures outputs that can be considered to express the same meaning. That is, for the space of semantic equivalence classes \({\mathcal{C}}\) the sentences in the set \(c\in {\mathcal{C}}\) can be regarded in many settings as expressing a similar meaning such that \(\forall {\bf{s}},{{\bf{s}}}^{{\prime} }\in c:E({\bf{s}},{{\bf{s}}}^{{\prime} })\) . So we can build up these classes of semantically equivalent sentences by checking if new sentences share a meaning with any sentences we have already clustered and, if so, adding them into that class.
We operationalize E ( ⋅ , ⋅ ) using the idea of bidirectional entailment, which has a long history in linguistics 52 and natural language processing 28 , 53 , 54 . A sequence, s , means the same thing as a second sequence, s ′, only if the sequences entail (that is, logically imply) each other. For example, ‘The capital of France is Paris’ entails ‘Paris is the capital of France’ and vice versa because they mean the same thing. (See later for a discussion of soft equivalence and cases in which bidirectional entailment does not guarantee equivalent meanings).
Importantly, we require that the sequences mean the same thing with respect to the context—key meaning is sometimes contained in the context. For example, ‘Paris’ does not entail ‘The capital of France is Paris’ because ‘Paris’ is not a declarative sentence without context. But in the context of the question ‘What is the capital of France?’, the one-word answer does entail the longer answer.
Detecting entailment has been the object of study of a great deal of research in NLI 55 . We rely on language models to predict entailment, such as DeBERTa-Large-MNLI 56 , which has been trained to predict entailment, or general-purpose LLMs such as GPT-3.5 (ref. 57 ), which can predict entailment given suitable prompts.
We then cluster sentences according to whether they bidirectionally entail each other using the algorithm presented in Extended Data Fig. 1 . Note that, to check if a sequence should be added to an existing cluster, it is sufficient to check if the sequence bidirectionally entails any of the existing sequences in that cluster (we arbitrarily pick the first one), given the transitivity of semantic equivalence. If a sequence does not share meaning with any existing cluster, we assign it its own cluster.
Computing the semantic entropy
Having determined the classes of generated sequences that mean the same thing, we can estimate the likelihood that a sequence generated by the LLM belongs to a given class by computing the sum of the probabilities of all the possible sequences of tokens which can be considered to express the same meaning as
Formally, this treats the output as a random variable whose event-space is the space of all possible meaning-classes, C , a sub- σ -algebra of the standard event-space S . We can then estimate the semantic entropy (SE) as the entropy over the meaning-distribution,
There is a complication which prevents direct computation: we do not have access to every possible meaning-class c . Instead, we can only sample c from the sequence-generating distribution induced by the model. To handle this, we estimate the expectation in equation ( 3 ) using a Rao–Blackwellized Monte Carlo integration over the semantic equivalence classes C ,
where \(P({C}_{i}| {\boldsymbol{x}})=\frac{P({c}_{i}| {\boldsymbol{x}})}{{\sum }_{c}P(c| {\boldsymbol{x}})}\) estimates a categorical distribution over the cluster meanings, that is, ∑ i P ( C i ∣ x ) = 1. Without this normalization step cluster ‘probabilities’ could exceed one because of length normalization, resulting in degeneracies. Equation ( 5 ) is the estimator giving our main method that we refer to as semantic entropy throughout the text.
For scenarios in which the sequence probabilities are not available, we propose a variant of semantic entropy which we call ‘discrete’ semantic entropy. Discrete semantic entropy approximates P ( C i ∣ x ) directly from the number of generations in each cluster, disregarding the token probabilities. That is, we approximate P ( C i ∣ x ) as \({\sum }_{1}^{M}\frac{{I}_{c={C}_{i}}}{M}\) , the proportion of all the sampled answers which belong to that cluster. Effectively, this just assumes that each output that was actually generated was equally probable—estimating the underlying distribution as the categorical empirical distribution. In the limit of M the estimator converges to equation ( 5 ) by the law of large numbers. We find that discrete semantic entropy results in similar performance empirically.
We provide a worked example of the computation of semantic entropy in Supplementary Note 1 .
Semantic entropy is designed to detect confabulations, that is, model outputs with arbitrary meaning. In our experiments, we use semantic uncertainty to predict model accuracy, demonstrating that confabulations make up a notable fraction of model mistakes. We further show that semantic uncertainty can be used to improve model accuracy by refusing to answer questions when semantic uncertainty is high. Last, semantic uncertainty can be used to give users a way to know when model generations are probably unreliable.
We use the datasets BioASQ 34 , SQuAD 33 , TriviaQA 32 , SVAMP 37 and NQ-Open 35 . BioASQ is a life-sciences question-answering dataset based on the annual challenge of the same name. The specific dataset we use is based on the QA dataset from Task B of the 2023 BioASQ challenge (11B). SQuAD is a reading comprehension dataset whose context passages are drawn from Wikipedia and for which the answers to questions can be found in these passages. We use SQuAD 1.1 which excludes the unanswerable questions added in v.2.0 that are deliberately constructed to induce mistakes so they do not in practice cause confabulations to occur. TriviaQA is a trivia question-answering dataset. SVAMP is a word-problem maths dataset containing elementary-school mathematical reasoning tasks. NQ-Open is a dataset of realistic questions aggregated from Google Search which have been chosen to be answerable without reference to a source text. For each dataset, we use 400 train examples and 400 test examples randomly sampled from the original larger dataset. Note that only some of the methods require training, for example semantic entropy does not use the training data. If the datasets themselves are already split into train and test (or validation) samples, we sample our examples from within the corresponding split.
All these datasets are free-form, rather than multiple choice, because this better captures the opportunities created by LLMs to produce free-form sentences as answers. We refer to this default scenario as our ‘sentence-length’ experiments. In Supplementary Note 7 , we also present results for confabulation detection in a ‘short-phrase’ scenario, in which we constrain model answers on these datasets to be as concise as possible.
To make the problems more difficult and induce confabulations, we do not provide the context passages for any of the datasets. When the context passages are provided, the accuracy rate is too high for these datasets for the latest generations of models to meaningfully study confabulations.
For sentence-length generations we use: Falcon 39 Instruct (7B and 40B), LLaMA 2 Chat 38 (7B, 13B and 70B) and Mistral 40 Instruct (7B).
In addition to reporting results for semantic entropy, discrete semantic entropy and naive entropy, we consider two strong baselines.
Embedding regression is a supervised baseline inspired by the P (IK) method 24 . In that paper, the authors fine-tune their proprietary LLM on a dataset of questions to predict whether the model would have been correct. This requires access to a dataset of ground-truth answers to the questions. Rather than fine-tuning the entire LLM in this way, we simply take the final hidden units and train a logistic regression classifier to make the same prediction. By contrast to their method, this is much simpler because it does not require fine-tuning the entire language model, as well as being more reproducible because the solution to the logistic regression optimization problem is not as seed-dependent as the fine-tuning procedure. As expected, this supervised approach performs well in-distribution but fails when the distribution of questions is different from that on which the classifier is trained.
The second baseline we consider is the P (True) method 24 , in which the model first samples M answers (identically to our semantic entropy approach) and then is prompted with the list of all answers generated followed by the highest probability answer and a question whether this answer is “(a) True” or “(b) False”. The confidence score is then taken to be the probability with which the LLM responds with ‘a’ to the multiple-choice question. The performance of this method is boosted with a few-shot prompt, in which up to 20 examples from the training set are randomly chosen, filled in as above, but then provided with the actual ground truth of whether the proposed answer was true or false. In this way, the method can be considered as supervised ‘in-context’ because it makes use of some ground-truth training labels but can be used without retraining the model. Because of context-size constraints, this method cannot fit a full 20 few-shot examples in the context when input questions are long or large numbers of generations are used. As a result, we sometimes have to reduce the number of few-shot examples to suit the context size and we note this in the Supplementary Material .
Entailment estimator
Any NLI classification system could be used for our bidirectional entailment clustering algorithm. We consider two different kinds of entailment detector.
One option is to use an instruction-tuned LLM such as LLaMA 2, GPT-3.5 (Turbo 1106) or GPT-4 to predict entailment between generations. We use the following prompt:
We are evaluating answers to the question {question} Here are two possible answers: Possible Answer 1: {text1} Possible Answer 2: {text2} Does Possible Answer 1 semantically entail Possible Answer 2? Respond with entailment, contradiction, or neutral.
Alternatively, we consider using a language model trained for entailment prediction, specifically the DeBERTa-large model 56 fine-tuned on the NLI dataset MNLI 58 . This builds on past work towards paraphrase identification based on embedding similarity 59 , 60 and BERT-style models 61 , 62 . We template more simply, checking if DeBERTa predicts entailment between the concatenation of the question and one answer and the concatenation of the question and another answer. Note that DeBERTa-large is a relatively lightweight model with only 1.5B parameters which is much less powerful than most of the LLMs under study.
In Supplementary Note 2 , we carefully evaluate the benefits and drawbacks of these methods for entailment prediction. We settle on using GPT-3.5 with the above prompt, as its entailment predictions agree well with human raters and lead to good confabulation detection performance.
In Supplementary Note 3 , we provide a discussion of the computational cost and choosing the number of generations for reliable clustering.
Prompting templates
We use a simple generation template for all sentence-length answer datasets:
Answer the following question in a single brief but complete sentence. Question: {question} Answer:
Metrics and accuracy measurements
We use three main metrics to evaluate our method: AUROC, rejection accuracy and AURAC. Each of these is grounded in an automated factuality estimation measurement relative to the reference answers provided by the datasets that we use.
AUROC, rejection accuracy and AURAC
First, we use the AUROC curve, which measures the reliability of a classifier accounting for both precision and recall. The AUROC can be interpreted as the probability that a randomly chosen correct answer has been assigned a higher confidence score than a randomly chosen incorrect answer. For a perfect classifier, this is 1.
Second, we compute the ‘rejection accuracy at X %’, which is the question-answering accuracy of the model on the most-confident X % of the inputs as identified by the respective uncertainty method. If an uncertainty method works well, predictions on the confident subset should be more accurate than predictions on the excluded subset and the rejection accuracy should increase as we reject more inputs.
To summarize this statistic we compute the AURAC—the total area enclosed by the accuracies at all cut-off percentages X %. This should increase towards 1 as given uncertainty method becomes more accurate and better at detecting likely-inaccurate responses but it is more sensitive to the overall accuracy of the model than the AUROC metric.
In Supplementary Note 5 , we provide the unaggregated rejection accuracies for sentence-length generations.
Assessing accuracy
For the short-phrase-length generation setting presented in Supplementary Note 7 , we simply assess the accuracy of the generations by checking if the F1 score of the commonly used SQuAD metric exceeds 0.5. There are limitations to such simple scoring rules 63 but this method is widely used in practice and its error is comparatively small on these standard datasets.
For our default scenario, the longer sentence-length generations, this measure fails, as the overlap between the short reference answer and our long model answer is invariably too small. For sentence-length generations, we therefore automatically determine whether an answer to the question is correct or incorrect by using GPT-4 to compare the given answer to the reference answer. We use the template:
We are assessing the quality of answers to the following question: {question} The expected answer is: {reference answer} The proposed answer is: {predicted answer} Within the context of the question, does the proposed answer mean the same as the expected answer? Respond only with yes or no.
We make a small modification for datasets with several reference answers: line two becomes “The following are expected answers to this question:” and the final line asks “does the proposed answer mean the same as any of the expected answers?”.
In Supplementary Note 6 , we check the quality of our automated ground-truth evaluations against human judgement by hand. We find that GPT-4 gives the best results for determining model accuracy and thus use it in all our sentence-length experiments.
In this section we describe the application of semantic entropy to confabulation detection in longer model generations, specifically paragraph-length biographies.
We introduce a biography-generation dataset—FactualBio—available alongside this paper. FactualBio is a collection of biographies of individuals who are notable enough to have Wikipedia pages but not notable enough to have large amounts of detailed coverage, generated by GPT-4 (v.0613). To generate the dataset, we randomly sampled 21 individuals from the WikiBio dataset 64 . For each biography, we generated a list of factual claims contained in each biography using GPT-4, with 150 total factual claims (the total number is only coincidentally a round number). For each of these factual claims, we manually determined whether the claim was correct or incorrect. Out of 150 claims, 45 were incorrect. As before, we apply confabulation detection to detect incorrect model predictions, even though there may be model errors which are not confabulations.
Prompting and generation
Given a paragraph-length piece of LLM-generated text, we apply the following sequence of steps:
Automatically decompose the paragraph into specific factual claims using an LLM (not necessarily the same as the original).
For each factual claim, use an LLM to automatically construct Q questions which might have produced that claim.
For each question, prompt the original LLM to generate M answers.
For each question, compute the semantic entropy of the answers, including the original factual claim.
Average the semantic entropies over the questions to arrive at a score for the original factual claim.
We pursue this slightly indirect way of generating answers because we find that simply resampling each sentence creates variation unrelated to the uncertainty of the model about the factual claim, such as differences in paragraph structure.
We decompose the paragraph into factual claims using the following prompt:
Please list the specific factual propositions included in the answer above. Be complete and do not leave any factual claims out. Provide each claim as a separate sentence in a separate bullet point.
We found that we agreed with the decompositions in all cases in the dataset.
We then generate six questions for each of the facts from the decomposition. We generate these questions by prompting the model twice with the following:
Following this text: {text so far} You see the sentence: {proposition} Generate a list of three questions, that might have generated the sentence in the context of the preceding original text, as well as their answers. Please do not use specific facts that appear in the follow-up sentence when formulating the question. Make the questions and answers diverse. Avoid yes-no questions. The answers should not be a full sentence and as short as possible, e.g. only a name, place, or thing. Use the format “1. {question} – {answer}”.
These questions are not necessarily well-targeted and the difficulty of this step is the main source of errors in the procedure. We generate three questions with each prompt, as this encourages diversity of the questions, each question targeting a different aspect of the fact. However, we observed that the generated questions will sometimes miss obvious aspects of the fact. Executing the above prompt twice (for a total of six questions) can improve coverage. We also ask for brief answers because the current version of GPT-4 tends to give long, convoluted and highly hedged answers unless explicitly told not to.
Then, for each question, we generate three new answers using the following prompt:
We are writing an answer to the question “{user question}”. So far we have written: {text so far} The next sentence should be the answer to the following question: {question} Please answer this question. Do not answer in a full sentence. Answer with as few words as possible, e.g. only a name, place, or thing.
We then compute the semantic entropy over these answers plus the original factual claim. Including the original fact ensures that the estimator remains grounded in the original claim and helps detect situations in which the question has been interpreted completely differently from the original context. We make a small modification to handle the fact that GPT-4 generations often include refusals to answer questions. These refusals were not something we commonly observe in our experiments with LLaMA 2, Falcon or Mistral models. If more than half of the answers include one of the strings ‘not available’, ‘not provided’, ‘unknown’ or ‘unclear’ then we treat the semantic uncertainty as maximal.
We then average the semantic entropies for each question corresponding to the factual claim to get an entropy for this factual claim.
Despite the extra assumptions and complexity, we find that this method greatly outperforms the baselines.
To compute semantic entailment between the original claim and regenerated answers, we rely on the DeBERTa entailment prediction model as we find empirically that DeBERTa predictions result in higher train-set AUROC than other methods. Because DeBERTa has slightly lower recall than GPT-3.5/4, we use a modified set-up for which we say the answers mean the same as each other if at least one of them entails the other and neither is seen to contradict the other—a kind of ‘non-defeating’ bidirectional entailment check rather than true bidirectional entailment. The good performance of DeBERTa in this scenario is not surprising as both factual claims and regenerated answers are relatively short. We refer to Supplementary Notes 2 and 3 for ablations and experiments regarding our choice of entailment estimator for paragraph-length generations.
We implement two baselines. First, we implement a variant of the P (True) method, which is adapted to the new setting. For each factoid, we generate a question with answers in the same way as for semantic entropy. We then use the following prompt:
Question: {question} Here are some brainstormed ideas: {list of regenerated answers} Possible answer: {original answer} Is the possible answer true? Respond with “yes” or “no”.
As we cannot access the probabilities GPT-4 assigns to predicting ‘yes’ and ‘no’ as the next token, we approximate this using Monte Carlo samples. Concretely, we execute the above prompt ten times (at temperature 1) and then take the fraction of answers which was ‘yes’ as our unbiased Monte Carlo estimate of the token probability GPT-4 assigns to ‘yes’.
As a second, simpler, baseline we check if the model thinks the answer is true. We simply ask:
Following this text: {text so far} You see this statement: {proposition} Is it likely that the statement is true? Respond with ‘yes’ or ‘no’.
It is interesting that this method ought to perform very well if we think that the model has good ‘self-knowledge’ (that is, if “models mostly know what they don’t know” 24 ) but in fact semantic entropy is much better at detecting confabulations.
Data availability
The data used for the short-phrase and sentence-length generations are publicly available and the released code details how to access it. We release a public version of the FactualBio dataset as part of the code base for reproducing the paragraph-length experiments.
Code availability
We release all code used to produce the main experiments. The code for short-phrase and sentence-length experiments can be found at github.com/jlko/semantic_uncertainty and https://doi.org/10.5281/zenodo.10964366 (ref. 65 ). The code for paragraph-length experiments can be found at github.com/jlko/long_hallucinations and https://doi.org/10.5281/zenodo.10964366 (ref. 65 ).
GPT-4 technical report. Preprint at https://arxiv.org/abs/2303.08774 (2023).
Gemini: a family of highly capable multimodal models. Preprint at https://arxiv.org/abs/2312.11805 (2023).
Xiao, Y. & Wang, W. Y. On hallucination and predictive uncertainty in conditional language generation. In Proc. 16th Conference of the European Chapter of the Association for Computational Linguistics 2734–2744 (Association for Computational Linguistics, 2021).
Rohrbach, A., Hendricks, L. A., Burns, K., Darrell, T. & Saenko, K. Object hallucination in image captioning. In Proc. 2018 Conference on Empirical Methods in Natural Language Processing (eds Riloff, E., Chiang, D., Hockenmaier, J. & Tsujii, J.) 4035–4045 (Association for Computational Linguistics, 2018).
Weiser, B. Lawyer who used ChatGPT faces penalty for made up citations. The New York Times (8 Jun 2023).
Opdahl, A. L. et al. Trustworthy journalism through AI. Data Knowl. Eng . 146 , 102182 (2023).
Shen, Y. et al. ChatGPT and other large language models are double-edged swords. Radiology 307 , e230163 (2023).
Article PubMed Google Scholar
Schulman, J. Reinforcement learning from human feedback: progress and challenges. Presented at the Berkeley EECS Colloquium. YouTube www.youtube.com/watch?v=hhiLw5Q_UFg (2023).
Ji, Z. et al. Survey of hallucination in natural language generation. ACM Comput. Surv. 55 , 248 (2023).
Maynez, J., Narayan, S., Bohnet, B. & McDonald, R. On faithfulness and factuality in abstractive summarization. In Proc. 58th Annual Meeting of the Association for Computational Linguistics (eds Jurafsky, D., Chai, J., Schluter, N. & Tetreault, J.) 1906–1919 (Association for Computational Linguistics, 2020).
Filippova, K. Controlled hallucinations: learning to generate faithfully from noisy data. In Findings of the Association for Computational Linguistics: EMNLP 2020 (eds Webber, B., Cohn, T., He, Y. & Liu, Y.) 864–870 (Association for Computational Linguistics, 2020).
Berrios, G. Confabulations: a conceptual history. J. Hist. Neurosci. 7 , 225–241 (1998).
Article CAS PubMed Google Scholar
Lin, S., Hilton, J. & Evans, O. Teaching models to express their uncertainty in words. Transact. Mach. Learn. Res. (2022).
Evans, O. et al. Truthful AI: developing and governing AI that does not lie. Preprint at https://arxiv.org/abs/2110.06674 (2021).
Amodei, D. et al. Concrete problems in AI safety. Preprint at https://arxiv.org/abs/1606.06565 (2016).
Jiang, Z., Araki, J., Ding, H. & Neubig, G. How can we know when language models know? On the calibration of language models for question answering. Transact. Assoc. Comput. Linguist. 9 , 962–977 (2021).
Article Google Scholar
Desai, S. & Durrett, G. Calibration of pre-trained transformers. In Proc. 2020 Conference on Empirical Methods in Natural Language Processing (EMNLP) (eds Webber, B., Cohn, T., He, Y. & Liu, Y.) 295–302 (Association for Computational Linguistics, 2020).
Glushkova, T., Zerva, C., Rei, R. & Martins, A. F. Uncertainty-aware machine translation evaluation. In Findings of the Association for Computational Linguistics: EMNLP 2021 (eds Moens, M-F., Huang, X., Specia, L. & Yih, S.) 3920–3938 (Association for Computational Linguistics, 2021).
Wang, Y., Beck, D., Baldwin, T. & Verspoor, K. Uncertainty estimation and reduction of pre-trained models for text regression. Transact. Assoc. Comput. Linguist. 10 , 680–696 (2022).
Baker, S. & Kanade, T. Hallucinating faces. In Proc. Fourth IEEE International Conference on Automatic Face and Gesture Recognition . 83–88 (IEEE, Catalogue no PR00580, 2002).
Eliot, L. AI ethics lucidly questioning this whole hallucinating AI popularized trend that has got to stop. Forbes Magazine (24 August 2022).
Shanahan, M. Talking about large language models. Commun. Assoc. Comp. Machinery 67 , 68–79 (2024).
MacKay, D. J. C. Information-based objective functions for active data selection. Neural Comput. 4 , 590–604 (1992).
Kadavath, S. et al. Language models (mostly) know what they know. Preprint at https://arxiv.org/abs/2207.05221 (2022).
Lindley, D. V. On a measure of the information provided by an experiment. Ann. Math. Stat. 27 , 986–1005 (1956).
Article MathSciNet Google Scholar
Xiao, T. Z., Gomez, A. N. & Gal, Y. Wat zei je? Detecting out-of-distribution translations with variational transformers. In Workshop on Bayesian Deep Learning at the Conference on Neural Information Processing Systems (NeurIPS, Vancouver, 2019).
Christiano, P., Cotra, A. & Xu, M. Eliciting Latent Knowledge (Alignment Research Center, 2021); https://docs.google.com/document/d/1WwsnJQstPq91_Yh-Ch2XRL8H_EpsnjrC1dwZXR37PC8/edit .
Negri, M., Bentivogli, L., Mehdad, Y., Giampiccolo, D. & Marchetti, A. Divide and conquer: crowdsourcing the creation of cross-lingual textual entailment corpora. In Proc. 2011 Conference on Empirical Methods in Natural Language Processing 670–679 (Association for Computational Linguistics, 2011).
Honovich, O. et al. TRUE: Re-evaluating factual consistency evaluation. In Proc. Second DialDoc Workshop on Document-grounded Dialogue and Conversational Question Answering 161–175 (Association for Computational Linguistics, 2022).
Falke, T., Ribeiro, L. F. R., Utama, P. A., Dagan, I. & Gurevych, I. Ranking generated summaries by correctness: an interesting but challenging application for natural language inference. In Proc. 57th Annual Meeting of the Association for Computational Linguistics 2214–2220 (Association for Computational Linguistics, 2019).
Laban, P., Schnabel, T., Bennett, P. N. & Hearst, M. A. SummaC: re-visiting NLI-based models for inconsistency detection in summarization. Trans. Assoc. Comput. Linguist. 10 , 163–177 (2022).
Joshi, M., Choi, E., Weld, D. S. & Zettlemoyer, L. TriviaQA: a large scale distantly supervised challenge dataset for reading comprehension. In Proc. 55th Annual Meeting of the Association for Computational Linguistics 1601–1611 (Association for Computational Linguistics. 2017).
Rajpurkar, P., Zhang, J., Lopyrev, K. & Liang, P. SQuAD: 100,000+ questions for machine compression of text. In Proc. 2016 Conference on Empirical Methods in Natural Language Processing (eds Su, J., Duh, K. & Carreras, X.) 2383–2392 (Association for Computational Linguistics, 2016).
Tsatsaronis, G. et al. An overview of the BIOASQ large-scale biomedical semantic indexing and question answering competition. BMC Bioinformatics 16 , 138 (2015).
Article PubMed PubMed Central Google Scholar
Lee, K., Chang, M.-W. & Toutanova, K. Latent retrieval for weakly supervised open domain question answering. In Proc. 57th Annual Meeting of the Association for Computational Linguistics 6086–6096 (Association for Computational Linguistics, 2019).
Kwiatkowski, T. et al. Natural questions: a benchmark for question answering research. Transact. Assoc. Comput. Linguist. 7 , 452–466 (2019).
Patel, A., Bhattamishra, S. & Goyal, N. Are NLP models really able to solve simple math word problems? In Proc. 2021 Conference of the North American Chapter of the Association for Computational Linguistics: Human Language Technologies (eds Toutanova, K. et al.) 2080–2094 (Assoc. Comp. Linguistics, 2021).
Touvron, H. et al. Llama 2: open foundation and fine-tuned chat models. Preprint at https://arxiv.org/abs/2307.09288 (2023).
Penedo, G. et al. The RefinedWeb dataset for Falcon LLM: outperforming curated corpora with web data, and web data only. In Proc. 36th Conference on Neural Information Processing Systems (eds Oh, A. et al.) 79155–79172 (Curran Associates, 2023)
Jiang, A. Q. et al. Mistral 7B. Preprint at https://arxiv.org/abs/2310.06825 (2023).
Manakul, P., Liusie, A. & Gales, M. J. F. SelfCheckGPT: Zero-Resource Black-Box hallucination detection for generative large language models. In Findings of the Association for Computational Linguistics: EMNLP 2023 (eds Bouamor, H., Pino, J. & Bali, K.) 9004–9017 (Assoc. Comp. Linguistics, 2023).
Mukhoti, J., Kirsch, A., van Amersfoort, J., Torr, P. H. & Gal, Y. Deep deterministic uncertainty: a new simple baseline. In IEEE/CVF Conference on Computer Vision and Pattern Recognition 24384–24394 (Computer Vision Foundation, 2023).
Schuster, T., Chen, S., Buthpitiya, S., Fabrikant, A. & Metzler, D. Stretching sentence-pair NLI models to reason over long documents and clusters. In Findings of the Association for Computational Linguistics: EMNLP 2022 (eds Goldberg, Y. et al.) 394–412 (Association for Computational Linguistics, 2022).
Barnes, B. & Christiano, P. Progress on AI Safety via Debate. AI Alignment Forum www.alignmentforum.org/posts/Br4xDbYu4Frwrb64a/writeup-progress-on-ai-safety-via-debate-1 (2020).
Irving, G., Christiano, P. & Amodei, D. AI safety via debate. Preprint at https://arxiv.org/abs/1805.00899 (2018).
Der Kiureghian, A. & Ditlevsen, O. Aleatory or epistemic? Does it matter? Struct. Saf. 31 , 105–112 (2009).
Malinin, A. & Gales, M. Uncertainty estimation in autoregressive structured prediction. In Proceedings of the International Conference on Learning Representations https://openreview.net/forum?id=jN5y-zb5Q7m (2021).
Murray, K. & Chiang, D. Correcting length bias in neural machine translation. In Proc. Third Conference on Machine Translation (eds Bojar, O. et al.) 212–223 (Assoc. Comp. Linguistics, 2018).
Holtzman, A., Buys, J., Du, L., Forbes, M. & Choi, Y. The curious case of neural text degeneration. In Proceedings of the International Conference on Learning Representations https://openreview.net/forum?id=rygGQyrFvH (2020).
Fan, A., Lewis, M. & Dauphin, Y. Hierarchical neural story generation. In Proc. 56th Annual Meeting of the Association for Computational Linguistics (eds Gurevych, I. & Miyao, Y.) 889–898 (Association for Computational Linguistics, 2018).
Speaks, J. in The Stanford Encyclopedia of Philosophy (ed. Zalta, E. N.) (Metaphysics Research Lab, Stanford Univ., 2021).
Culicover, P. W. Paraphrase generation and information retrieval from stored text. Mech. Transl. Comput. Linguist. 11 , 78–88 (1968).
Google Scholar
Padó, S., Cer, D., Galley, M., Jurafsky, D. & Manning, C. D. Measuring machine translation quality as semantic equivalence: a metric based on entailment features. Mach. Transl. 23 , 181–193 (2009).
Androutsopoulos, I. & Malakasiotis, P. A survey of paraphrasing and textual entailment methods. J. Artif. Intell. Res. 38 , 135–187 (2010).
MacCartney, B. Natural Language Inference (Stanford Univ., 2009).
He, P., Liu, X., Gao, J. & Chen, W. Deberta: decoding-enhanced BERT with disentangled attention. In International Conference on Learning Representations https://openreview.net/forum?id=XPZIaotutsD (2021).
Brown, T. et al. Language models are few-shot learners. Adv. Neural Inf. Process. Syst. 33 , 1877–1901 (2020).
Williams, A., Nangia, N. & Bowman, S. R. A broad-coverage challenge corpus for sentence understanding through inference. In Proc. 2018 Conference of the North American Chapter of the Association for Computational Linguistics: Human Language Technologies (eds Walker, M. et al.) 1112–1122 (Assoc. Comp. Linguistics, 2018).
Yu, L., Hermann, K. M., Blunsom, P. & Pulman, S. Deep learning for answer sentence selection. Preprint at https://arxiv.org/abs/1412.1632 (2014).
Socher, R., Huang, E., Pennin, J., Manning, C. D. & Ng, A. Dynamic pooling and unfolding recursive autoencoders for paraphrase detection. In Proceedings of the 24th Conference on Neural Information Processing Systems (eds Shawe-Taylor, J. et al.) (2011)
He, R., Ravula, A., Kanagal, B. & Ainslie, J. Realformer: Transformer likes residual attention. In Findings of the Association for Computational Linguistics: ACL-IJCNLP 2021 (eds Zhong, C., et al.) 929–943 (Assoc. Comp. Linguistics, 2021).
Tay, Y. et al. Charformer: fast character transformers via gradient-based subword tokenization. In Proceedings of the International Conference on Learning Representations https://openreview.net/forum?id=JtBRnrlOEFN (2022).
Kane, H., Kocyigit, Y., Abdalla, A., Ajanoh, P. & Coulibali, M. Towards neural similarity evaluators. In Workshop on Document Intelligence at the 32nd conference on Neural Information Processing (2019).
Lebret, R., Grangier, D. & Auli, M. Neural text generation from structured data with application to the biography domain. In Proc. 2016 Conference on Empirical Methods in Natural Language Processing (eds Su, J. et al.) 1203–1213 (Association for Computational Linguistics, 2016).
Kossen, J., jlko/semantic_uncertainty: Initial release v.1.0.0. Zenodo https://doi.org/10.5281/zenodo.10964366 (2024).
Download references
Acknowledgements
We thank G. Irving, K. Perlin, J. Richens, L. Rimell and M. Turpin for their comments or discussion related to this work. We thank K. Handa for his help with the human evaluation of our automated accuracy assessment. We thank F. Bickford Smith and L. Melo for their code review. Y.G. is supported by a Turing AI Fellowship funded by the UK government’s Office for AI, through UK Research and Innovation (grant reference EP/V030302/1), and delivered by the Alan Turing Institute.
Author information
These authors contributed equally: Sebastian Farquhar, Jannik Kossen, Lorenz Kuhn
Authors and Affiliations
OATML, Department of Computer Science, University of Oxford, Oxford, UK
Sebastian Farquhar, Jannik Kossen, Lorenz Kuhn & Yarin Gal
You can also search for this author in PubMed Google Scholar
Contributions
S.F. led the work from conception to completion and proposed using bidirectional entailment to cluster generations as a way of computing entropy in LLMs. He wrote the main text, most of the Methods and Supplementary Information and prepared most of the figures. J.K. improved the mathematical formalization of semantic entropy; led the extension of semantic entropy to sentence- and paragraph-length generations; wrote the code for, and carried out, all the experiments and evaluations; wrote much of the Methods and Supplementary Information and prepared drafts of many figures; and gave critical feedback on the main text. L.K. developed the initial mathematical formalization of semantic entropy; wrote code for, and carried out, the initial experiments around semantic entropy and its variants which demonstrated the promise of the idea and helped narrow down possible research avenues to explore; and gave critical feedback on the main text. Y.G. ideated the project, proposing the idea to differentiate semantic and syntactic diversity as a tool for detecting hallucinations, provided high-level guidance on the research and gave critical feedback on the main text; he runs the research laboratory in which the work was carried out.
Corresponding author
Correspondence to Sebastian Farquhar .
Ethics declarations
Competing interests.
S.F. is currently employed by Google DeepMind and L.K. by OpenAI. For both, this paper was written under their University of Oxford affiliation. The remaining authors declare no competing interests.
Peer review
Peer review information.
Nature thanks Mirella Lapata and the other, anonymous, reviewer(s) for their contribution to the peer review of this work.
Additional information
Publisher’s note Springer Nature remains neutral with regard to jurisdictional claims in published maps and institutional affiliations.
Extended data figures and tables
Extended data fig. 1 algorithm outline for bidirectional entailment clustering..
Given a set of outputs in response to a context, the bidirectional entailment answer returns a set of sets of outputs which have been classified as sharing a meaning.
Supplementary information
Supplementary information.
Supplementary Notes 1–7, Figs. 1–10, Tables 1–4 and references. Includes, worked example for semantic entropy calculation, discussion of limitations and computational cost of entailment clustering, ablation of entailment prediction and clustering methods, discussion of automated accuracy assessment, unaggregated results for sentence-length generations and further results for short-phrase generations.
Rights and permissions
Open Access This article is licensed under a Creative Commons Attribution 4.0 International License, which permits use, sharing, adaptation, distribution and reproduction in any medium or format, as long as you give appropriate credit to the original author(s) and the source, provide a link to the Creative Commons licence, and indicate if changes were made. The images or other third party material in this article are included in the article’s Creative Commons licence, unless indicated otherwise in a credit line to the material. If material is not included in the article’s Creative Commons licence and your intended use is not permitted by statutory regulation or exceeds the permitted use, you will need to obtain permission directly from the copyright holder. To view a copy of this licence, visit http://creativecommons.org/licenses/by/4.0/ .
Reprints and permissions
About this article
Cite this article.
Farquhar, S., Kossen, J., Kuhn, L. et al. Detecting hallucinations in large language models using semantic entropy. Nature 630 , 625–630 (2024). https://doi.org/10.1038/s41586-024-07421-0
Download citation
Received : 17 July 2023
Accepted : 12 April 2024
Published : 19 June 2024
Issue Date : 20 June 2024
DOI : https://doi.org/10.1038/s41586-024-07421-0
Share this article
Anyone you share the following link with will be able to read this content:
Sorry, a shareable link is not currently available for this article.
Provided by the Springer Nature SharedIt content-sharing initiative
By submitting a comment you agree to abide by our Terms and Community Guidelines . If you find something abusive or that does not comply with our terms or guidelines please flag it as inappropriate.
Quick links
- Explore articles by subject
- Guide to authors
- Editorial policies
Sign up for the Nature Briefing: AI and Robotics newsletter — what matters in AI and robotics research, free to your inbox weekly.


IMAGES
COMMENTS
Introduction. The coronavirus disease 2019 (COVID-19) pandemic caused by the severe acute respiratory syndrome coronavirus 2 (SARS-CoV-2) has resulted in over 192 million cases and 4.1 million deaths as of July 22, 2021. 1 This pandemic has brought along a massive burden in morbidity and mortality in the healthcare systems. Despite the implementation of stringent public health measures, there ...
Important milestones in vaccine research are the development of recombinant viral-vector vaccines, virus-like particle vaccines, conjugated polysaccharide- or protein-based vaccines, and toxoid vaccines. ... hence allowing rapid, scalable, and cost-effective production. For example, a 5 L bioreactor can produce a million doses of mRNA vaccine ...
A two-dose regimen of BNT162b2 (30 μg per dose, given 21 days apart) was found to be safe and 95% effective against Covid-19. The vaccine met both primary efficacy end points, with more than a 99 ...
The development of the Merck Ebola vaccine is an example. ... research, and response. Vaccine 39, 85-120 (2021). Article PubMed PubMed Central Google Scholar ...
Vaccination is a powerful method of disease prevention that is relevant to people of all ages and in all countries, as the Covid-19 pandemic illustrates. Vaccination can improve people's chances ...
The effectiveness of partial vaccination, estimated in this study at 78% with the BNT162b2 vaccine and at 89% with the mRNA-1273 vaccine, was higher than the estimates from the respective phase 3 ...
For example, in one of the papers in this Collection, Goodswen et al. 7 present a state-of-the-art methodology for high-throughput in silico vaccine discovery against protozoan parasites ...
Our analyses indicate that vaccine effectiveness generally decreases over time against SARS-CoV-2 infections, hospitalisations, and mortality. The baseline vaccine effectiveness levels for the omicron variant were notably lower than for other variants. Therefore, other preventive measures (eg, face-mask wearing and physical distancing) might be necessary to manage the pandemic in the long term.
Vaccine effectiveness for different clinical outcomes of COVID-19. We separately reported the vaccine effectiveness (VE) by the first and second dose of vaccines, and conducted subgroup analysis by the days after the first or second dose (< 7 days, ≥ 7 days, ≥ 14 days, and ≥ 21 days; studies with no specific days were classified as 1 dose, 2 dose or ≥ 1 dose).
The antigenic component of non-live vaccines can be killed whole organisms (for example, whole-cell pertussis vaccine and inactivated polio vaccine), purified proteins from the organism (for ...
In the 1960's at the Walter Reed Army Institute of Research, vaccines were developed using capsular polysaccharides (Gold and Artenstein, 1971; Artenstein, 1975), of encapsulated organisms including meningococci and later pneumococci ... for example VZV or pertussis, a milder disease course may follow (Andre et al., 2008; Bonanni et al., 2015).
Background Despite reliable evidence-based research supporting the COVID-19 vaccines, population-wide confidence and trust remain limited. We sought to expand prior knowledge about COVID-19 vaccine perceptions, while determining which population groups are at greatest risk for not getting a vaccine. Methods Study participants in the U.S. (79% female, median age group 46-60 years) were ...
Childhood vaccination has dramatically reduced morbidity, mortality, and disability caused by vaccine-preventable diseases, with ∼21 million hospitalizations, 732 000 deaths, and 322 million cases of disease averted in the United States between 1994 and 2013. 1 Among diseases targeted by vaccines recommended before 1980, 3—polio, measles, and rubella—have achieved elimination status as ...
The Coronavirus Efficacy (COVE) phase 3 trial was launched in late July 2020 to assess the safety and efficacy of the mRNA-1273 vaccine in preventing SARS-CoV-2 infection. An independent data and ...
The COVID-19 pandemic has created a new reality where individuals are faced with a previously unknown disease and its effects, providing a unique opportunity to investigate vaccine attitudes during a period of heightened disease salience. The present research reports findings from a longitudinal study conducted during the COVID-19 health crisis ...
For example, other research has suggested that preexisting vaccine hesitancy inspires misinformation consumption rather than vice versa , whereas the few lab studies testing for a causal relationship between vaccine misinformation and behavioral intentions have shown conflicting evidence (30, 31). Thus, whether and to what extent misinformation ...
Abstract. Vaccine hesitancy (VH) is considered a top-10 global health threat. The concept of VH has been described and applied inconsistently. This systematic review aims to clarify VH by ...
of vaccines despite availability" (Macdonald, 2015, p. 34). After a thorough literature review, evidence reveals that there is a gap between perceived vaccine importance and perceived vaccine safety in developed nations as many survey respondents believe in the efficacy and importance of vaccines but lack confidence in the safety of vaccines.
Vaccines 22 December 2020 Background paper on Covid-19 disease and vaccines Prepared by the Strategic Advisory Group of Experts (SAGE) on Immunization Working Group on ... Serological testing of a representative random sample of the population to detect evidence of exposure to a pathogen is an important method to estimate the true number of
The vaccine has entered phase II clinical trials, where it shall be evaluated in a large sample of the population (Anon, 2020f). 2.3. mRNA Vaccine. ... Moderna and the Vaccine Research Centre are co-developing an mRNA based vaccine candidate, wherein the mRNA is encapsulated in the lipid nanoparticles while Codagenix in collaboration with the ...
Discover the best examples of medical and healthcare research topics at EduBirdie. Explore innovative and significant topics that can inspire your next research paper. ... New vaccines for emerging infectious diseases ... Choosing a good medical research paper topic is a critical first step in the research process, influencing the direction and ...
N ature retracted a high-profile paper that served as part of the scientific groundwork for Micronoma, a San Diego-based startup, on Wednesday. "Some of the findings of the article are affected ...
As mass vaccination campaigns against coronavirus disease 2019 (Covid-19) commence worldwide, vaccine effectiveness needs to be assessed for a range of outcomes across diverse populations in a nonc...
WHO also promotes a life-course approach to vaccination through the Immunization Agenda 2030; influenza vaccines are a key example of vaccines that should be available throughout the course of life.Although current seasonal influenza vaccines are safe and reduce influenza-related illness, their effectiveness varies, particularly among older ...
A recent economic analysis of 10 vaccines for 94 low- and middle-income countries estimated that an investment of $34 billion for the immunization programs resulted in savings of $586 billion in reducing costs of illness and $1.53 trillion when broader economic benefits were included ( 5 ). The only human disease ever eradicated, smallpox, was ...
Overall, the PHE study showed vaccine effectiveness after two doses of the BNT162b2 vaccine of 94% against the alpha variant and 88% against the delta variant. The corresponding percentages with ...
Research article Full text access Obtaining the optimal shortest path between two points on a quasi-developable Bézier-type surface using the Geodesic-based Q-learning algorithm Vahide Bulut, Aytug Onan, Betul Senyayla
In a paper published today in Nature Communications, researchers unveiled previously unobserved phenomena in an ultra-clean sample of the correlated metal SrVO3. The study offers experimental ...
a, Naive entropy-based uncertainty measures variation in the exact answers, treating 'Paris', 'It's Paris' and 'France's capital Paris' as different.But this is unsuitable for ...