Assessing the Performance of Online Food Delivery (OFD) in India
- Conference paper
- First Online: 05 September 2021
- Cite this conference paper
- Amogh Bhaskara 18 ,
- Siddharth Menon 18 ,
- U. Dinesh Acharya 18 &
- H. C. Shiva Prasad ORCID: orcid.org/0000-0002-1296-8970 19
Part of the book series: Lecture Notes in Networks and Systems ((LNNS,volume 303))
Included in the following conference series:
- International Conference on Information Systems and Management Science
353 Accesses
The purpose of this paper is to analyze the application quality of the online food delivery companies by using a qualitative and exploratory approach through the collection of primary data through a consumer survey and secondary data through a sample of 25 companies operating in the online food delivery sector in India. The analysis has been conducted through the parameters involving the aspects of Content, Usability, Functionality, Trust, Satisfaction and Brand Loyalty. The Data gathered has been analyzed using the SPSS followed by the Structural equation modelling (SEM). The conceptual model for the project has been achieved using the SmartPLS (v.2.3.8). The result shows better usability to the customer has a link to enhance application quality for business cycle and loyal customer trust the OFD system to allow efficiency building and look for profiteering space.
This is a preview of subscription content, log in via an institution to check access.

Access this chapter
Subscribe and save.
- Get 10 units per month
- Download Article/Chapter or Ebook
- 1 Unit = 1 Article or 1 Chapter
- Cancel anytime
- Available as PDF
- Read on any device
- Instant download
- Own it forever
- Available as EPUB and PDF
- Compact, lightweight edition
- Dispatched in 3 to 5 business days
- Free shipping worldwide - see info
Tax calculation will be finalised at checkout
Purchases are for personal use only
Institutional subscriptions
Similar content being viewed by others
Exploring the Customer’s Acceptance Towards Food E-commerce Sites: Evaluation from Service Quality Perspectives
Attributes Affecting to Use Food Ordering App by Young Consumers
An Investigation on Mobile Service Quality of Food Delivery Provider from Customers’ Experiences
Gupta, A.: India hyperlocal market outlook to 2020 - Driven by Surge in Number of Startups and Series of Funding, kenresearch.com, para, 3 March 14 (2016). https://www.kenresearch.com/technology-and-telecom/it-and-ites/india-hyperlocal-market-research-report/7209-105.html . Accessed 28 Jan 2019
India Brand Equity Foundation, Growth of Ecommerce Industry in India-Infographic, October 2018. https://www.ibef.org/industry/ecommerce/infographic . Accessed 28 Jan 2019
AIMS Institute Pvt. Ltd., Online Food Service in India: An Analysis. AIMS, July 2017. https://theaims.ac.in/resources/online-food-service-in-india-an-analysis.html . Accessed 28 Jan 2019
RedSeer Consulting Agency, Food-Tech Market Updates, March 2019. https://redseer.com/newsletters/food-tech-market-updates-june18/ . Accessed 19 Mar 2019
Meenakshi, N., Sinha, A.: Food delivery apps in India: wherein lies the success strategy? Strateg. Dir. 35 (7), 12–15 (2019). https://doi.org/10.1108/SD-10-2018-0197
Article Google Scholar
Anumolu, K., Shiva Prasad, H.C., Gopalkrishna, B., Adarsh, P.K.: A digital marketing: a strategic outreaching process. Intl. J. Manage. IT Eng. 5 (7), 254–260 (2015)
Google Scholar
Vilella, R.M.: Conteúdo, usabilidade e funcionalidade: três dimensões para an avaliação de portais estaduais de governo eletrônico na web, [Dissertação de Mestrado] Escola de Ciência da Informação, Universidade Federal de Minas Gerais, Belo Horizonte, 263f (2003). http://bogliolo.eci.ufmg.br/downloads/VILELLA%20Conteudo%20Usabilidade%20e%20Funcionalidade.pdf . Accessed 14 Feb 2019
Daim, T.U., Basoglu, A.N., Gunay, D., Yildiz, C., Gomez, F.:, Exploring technology acceptance for online food services. Int. J. Buss. Info. Syst. 12 (4), 383–403 (2013). https://www.inderscienceonline.com/doi/pdf/10.1504/IJBIS.2013.053214
Pigatto, G., Guilherme, J., Negreti, A., Machado, M.L.: Have you chosen your request? Analysis of online food delivery companies in Brazil. British Food J. 119 (3), 639–657 (2017)
Thongpapanl, N., Ashraf, A.R.: Enhancing online performance through website content and personalization. J. Comp. Info. Syst. 52 (1), 3–13 (2011)
Statista, Platform-to-Consumer Delivery Statistics, March 2019, Online statistics portal. https://www.statista.com/outlook/376/119/platform-to-consumer-delivery/india . Accessed 25 Mar 2019
Das, J.: Consumer perception towards online food ordering and delivery services: an empirical study. J. Mange. 5 (5), 155–163 (2018). http://www.iaeme.com/JOM/issues.asp?JType=JOM&VType=5&IType=5 . Accessed 31 Jan 2019
Building Brands Online: Interactive Branding: Best Practices in a Direct Response-Driven Media, AdAge Insights (2010)
Kedah, Z., Ismail, Y., Ahasanul, K.M., Ahmed, A., Ahmed, S.: Key success factors of online food ordering services: an empirical study. Malays. Manage. Rev. 50 (2), 19–25 (2015). https://www.researchgate.net/publication/291074636_Key_Success_Factors_of_Online_Food_Ordering_Services_An_Empirical_Study . Accessed 31 Jan 2019
Pallant, J.: SPSS Survival Manual - A Step by Step Guide to Data Analysis Using SPSS for Windows, 3rd edn. Open University Press, Maidenhead (2007)
Srinivasam, S.: Swiggy pilots B2B offering under Swiggy Café at corporate cafeterias, ET Bureau, 3 October (2018). https://www.economictimes.indiatimes.com/articleshow/66048384.cms?utm_source=contentofinterest&utm_medium=text&utm_campaign=cppst . Accessed 21 Apr 2019
Wong, K.K.: Partial least squares structural equation modeling (PLS-SEM) techniques using SmartPLS. Market. Bull. 24 , 1-32 (2013). Technical Note 1. https://www.academia.edu/9210442/Partial_Least_Squares_Structural_Equation_Modeling_PLS-SEM_Techniques_Using_SmartPLS
Hair Jr., J.F., Hult, G.T.M., Ringle, C., Sarstedt, M.: A Primer on Partial Least Squares Structural Equation Modeling (PLS-SEM). Sage Publications, USA (2016)
Bruin, J.: newtest: command to compute new test. UCLA: Statistical Consulting Group (2006). https://stats.idre.ucla.edu/spss
Zaiontz, C.: Real Statistics using excel. http://www.real-statistics.com/reliability/cronbachs-alpha/
Fornell, C., Larcker, D.F.: Structural equation models with unobservable variables and measurement error: algebra and statistics. J. Market. Res. 18 , 382–388 (1981)
Tabachnick, B.G., Fidell, L.S.: Using Multivariate Statistics. HarperCollins, New York (1996)
Rossiter, J.R.: The C-OAR-SE procedure for scale development in marketing. Int. J. Res. Mark. 19 (4), 305–335 (2002)
Vos, K.: Ecommerce delivery trends: what contributes to a positive experience? (2016). https://www.mycustomer.com/selling/ecommerce/ecommerce-delivery-trends-what-contributes-to-a-positiveexperience
Alves, H., Fernandes, C.I., Raposo, M.: Social media marketing: a literature review and implications: implications of social media marketing. Psychol. Mark. 33 (1), 1029–1038 (2016). https://doi.org/10.1002/mar.20936
Krishnan, A.: Accessing the construct and content validity of uncertainty business using Sem approach - an exploratory study of manufacturing firm (2011). https://www.globaljournals.org/GJMBRVolume11/1-Accessing-the-Construct-and-Content-Validity-of-Uncertainty-Business.pdf
Download references
Author information
Authors and affiliations.
Manipal Institute of Technology, Manipal Academy of Higher Education, Manipal, 576104, India
Amogh Bhaskara, Siddharth Menon & U. Dinesh Acharya
Department of Mechanical Engineering, Faculty of Engineering, School of Automobile, Mechanical and Mechatronics, MUJ, Jaipur, 303007, India
H. C. Shiva Prasad
You can also search for this author in PubMed Google Scholar
Corresponding author
Correspondence to H. C. Shiva Prasad .
Editor information
Editors and affiliations.
CIS Dept, University of Malta, Msida, Malta
Department of Computer Science, Central University of Rajasthan, Rajasthan, India
Nishtha Kesswani
Joseph G. Vella
Faculty of ICT, University of Malta, Msida, Malta
Peter A. Xuereb
The Education University of Hong Kong, Hong Kong, Hong Kong
Man Fung Lo
Nueva Ecija University of Science and Technology, Cabanatuan City, Philippines
Rowell Diaz
Computer Engg, CUCRID Bldg, #A301, Covenant University, Ota, Nigeria
Sanjay Misra
Lm Thapar School of Management, Thapar University, Mohali, India
Vipul Gupta
Manipal University Jaipur, Jaipur, India
Princy Randhawa
Rights and permissions
Reprints and permissions
Copyright information
© 2022 The Author(s), under exclusive license to Springer Nature Switzerland AG
About this paper
Cite this paper.
Bhaskara, A., Menon, S., Dinesh Acharya, U., Shiva Prasad, H.C. (2022). Assessing the Performance of Online Food Delivery (OFD) in India. In: Garg, L., et al. Information Systems and Management Science. ISMS 2020. Lecture Notes in Networks and Systems, vol 303. Springer, Cham. https://doi.org/10.1007/978-3-030-86223-7_4
Download citation
DOI : https://doi.org/10.1007/978-3-030-86223-7_4
Published : 05 September 2021
Publisher Name : Springer, Cham
Print ISBN : 978-3-030-86222-0
Online ISBN : 978-3-030-86223-7
eBook Packages : Intelligent Technologies and Robotics Intelligent Technologies and Robotics (R0)
Share this paper
Anyone you share the following link with will be able to read this content:
Sorry, a shareable link is not currently available for this article.
Provided by the Springer Nature SharedIt content-sharing initiative
- Publish with us
Policies and ethics
- Find a journal
- Track your research
Emerging Trends Towards Online Food Delivery Apps in India
10 Pages Posted: 6 May 2021
S. C. B Samuel Anbu Selvan
The American College, Madurai
Susan Anita Andrew
The American College
Date Written: April 30, 2021
In recent times, a growing trend has been noticed in the usage of online food delivery services. With the increased usage of technology, every industry is being re-shaped, to better serve the needs of customers, who are the kings of the market. Businesses which are regularly upgraded in order to meet the evolving expectations of customers are those that can succeed in the long run. It is not the technology behind the services provided, rather it is the experience provided to the consumer using real food technology. With this in the background, it should be noted that even customers who had previously preferred to purchase food through offline means, are now turning to online food delivery Apps to make their purchases. The dining out culture is now being transformed into the eating in culture. And this trend is observed to be prominent among youth (15 – 34), especially in the metropolitan cities where there are constant traffic jams and the heavy population density, which results in even short distance travelling being a highly time - consuming process. The resultant effect is that a greater number of Indian households are being seen turning to online food delivery services as an easier and more convenient alternative. As the usage of food delivery Apps grows in the metropolitan cities in India, a growing trend is also being seen in smaller cities. It has therefore become a necessity for online food delivery service providers to identify the preferences and perceptions of consumers to enable them to meet their expectations. Through this study the historical background, current scenario and possible future developments are analyzed in order to help online food delivery services develop better strategies to improve sales and increase the customer base.
Keywords: Food Delivery, Perception, Consuming Process, Food Technology
Suggested Citation: Suggested Citation
The American College, Madurai ( email )
The American College, Madurai-625002 Madurai, TN Tamilnadu 625002 India 09443531531 (Phone) 625002 (Fax)
Susan Anita Andrew (Contact Author)
The american college ( email ).
Tallakulam MADURAI, TN Tamil Nadu 625002 India +919080944743 (Phone) 0452-2520711 (Fax)
HOME PAGE: http://https://americancollege.edu.in/
Do you have a job opening that you would like to promote on SSRN?
Paper statistics, related ejournals, io: productivity, innovation & technology ejournal.
Subscribe to this fee journal for more curated articles on this topic
Microeconomics: Intertemporal Consumer Choice & Savings eJournal
Ebusiness & ecommerce ejournal, applied computing ejournal, electrical engineering ejournal, industrial & manufacturing engineering ejournal.

An official website of the United States government
The .gov means it’s official. Federal government websites often end in .gov or .mil. Before sharing sensitive information, make sure you’re on a federal government site.
The site is secure. The https:// ensures that you are connecting to the official website and that any information you provide is encrypted and transmitted securely.
- Publications
- Account settings
Preview improvements coming to the PMC website in October 2024. Learn More or Try it out now .
- Advanced Search
- Journal List
- Elsevier - PMC COVID-19 Collection

Online food delivery companies' performance and consumers expectations during Covid-19: An investigation using machine learning approach
Purushottam meena.
a Department of Supply Chain & Information Management, School of Business, College of Charleston, Charleston, SC, 29424, USA
Gopal Kumar
b Department of Operations Management, Indian Institute of Management, Raipur, Chhattisgarh, 492015, India
Online food delivery (OFD) businesses flourished during COVID-19; however, OFD companies experienced different challenges and customers' expectations. This paper uses social media data to explore OFD companies' performance and customers' expectations during the COVID-19 pandemic. The most important topics in developed and developing countries are identified using machine learning. Results show that customers in India are more concerned about social responsibility, while financial aspects are more important in the US. Overall, customers in India are more satisfied with OFD companies during the COVID-19 pandemic than the US customers. We further find that factors such as OFD companies' brand, market size, country, and COVID-19 waves play a crucial role in moderating customer sentiment. The results of the study offer several managerial insights.
1. Introduction
The COVID-19 pandemic has caused a profound and severe impact on the global economy. As the COVID-19 pandemic started, many restaurants worldwide lost billions of dollars, and many even faced business closures ( National Restaurant Association, 2020 ). According to the National Restaurant Association (2020) report, the restaurant industry has already lost approximately $240 billion by 2020. Restaurants that remained in business found it essential to adapt to recent changes in the industry and offer online food delivery (OFD) services for their survival ( Brewer and Sebby, 2021 ). Many restaurants started using third-party food delivery providers' services during the pandemic. It resulted in a significant increase in online OFD businesses worldwide. For example, Uber Eats observed a substantial increase in OFD orders following the mandate of no dine-in service ( Raj et al., 2021 ).
The platform economy in the food sector is not more prominent in terms of labor participation in India ( Sundararajan, 2016 ; Pant and Shende, 2020 ). However, with entries of global OFD leading companies such as Uber Eats along with some Indian companies—Zomato and Swiggy—the OFD industry is proliferating with a 25–30 percent annually ( Boston Consulting Group, 2020 ). The overall platform economy in India is expected to be $30 billion by 2025 ( NASSCOM, 2018 ). In 2019, more than 48 million people used OFD platforms in the US, which is likely to be approximately 70 million in 2024. The OFD revenue in the US is expected to grow steadily at 7.26 percent annually and is estimated to be $41,504 million by 2025 ( Statista, 2021 ). As per the 2022 Statista report, the global OFD service market is expected to reach $223.7 billion in 2025 from $115.07 billion in 2020 (Statista, 2022).
In recent years, the number of laborers who work in the platform economy has increased significantly. According to the NITI Aayog (a policy think tank of the India government), the platform economy employs around 15 million workers, and approximately 0.44 million of them work in the food sector ( Tiwari et al., 2019 ). Food delivery workers are not hired as full-time employees like any other sharing economy sector. These workers are primarily considered interchangeable or “gig workers” by food delivery companies ( Sundararajan, 2016 ).
During the first wave of COVID-19, most restaurants in the US were forced to suspend dine-in services and were only allowed to operate via takeout, drive-through, or contactless deliveries. As the COVID-19 outbreak started, restaurants demand started plumbing globally and affected the financial performance of the restaurant industry. In US restaurants, customer demand decreased drastically with increasing COVID-19 cases ( Yang et al., 2020 ). However, more and more people started ordering foods online via food delivery platforms like DoorDash, Grubhub, Uber Eats (in the US), Zomato, and Swiggy (in India) ( Jain, 2020 ; Kim et al., 2021 ). Restaurants that provided OFD and curbside pickup services for contactless delivery experienced a lesser effect on financial performance. Most of these OFD services businesses were in operation during the COVID-19 outbreak as they are considered among the essential services. The OFD service platforms were instrumental for restaurants to remain in business during challenging times ( Puram et al., 2021 ). Compared to March 2019, customer spending on food delivery increased significantly (i.e., 70 percent)—during the first COVID-19 wave in March 2020 ( Chen McCain et al., 2021 ).
On the flip side, OFD service providers (i.e., riders or drivers) and consumers faced several challenges during the ongoing pandemic. For example, Indian OFD workers have faced personal and social risks, ranging from loss of income to exposure to COVID-19 and risking their lives ( Lalvani and Seetharaman, 2020 ). The OFD became crucial and popular as millions of people were quarantined and unquarantined required foods ( Chen McCain et al., 2021 ; Kim et al., 2021 ). While delivering food, OFD companies needed to follow strict COVID-19 appropriate behaviors; otherwise, riders and consumers would be exposed to life-threatening health risks.
Overall, during the COVID-19 pandemic, consumers prioritized expectations from OFD companies, and the challenges faced by OFD companies were different from those of the pre-COVID-19 pandemic ( Gavilan et al., 2021 ). The adaptability of food delivery companies to a situation of a global healthcare crisis, where customers' expectations are entirely different ( Gavilan et al., 2021 ; Nguyen and Vu, 2020 ). It may depend on several factors, such as fear ( Balakrishnan, 2020 ; Mehrolia et al., 2021 ; Ahorsu et al., 2020 ; Lo Coco et al., 2021 ), marketing strategies during COVID-19 waves ( Jia et al., 2021 ), brand perception ( Dsouza and Sharma, 2021 ; Prasetyo et al., 2021 ), market size, region (developing or developed countries) of their operations ( Keeble et al., 2020 ; Zanetta et al., 2021 ), public listing ( Bao and Zhu, 2022 ), and COVID-19 waves ( Kohút et al., 2021 ; Mohan et al., 2022 ), etc. For example, OFD companies with better brand perception, bigger market size, and listed in the stock market should do better in a crisis. They may also improve their performance in the second wave better than in the first wave of the COVID-19 pandemic. Therefore, to have a deeper understanding of the delivery operations of OFD companies and consumers' behaviors/expectations during a global healthcare crisis, it is crucial to understand how OFD companies performed, to what extent consumers' expectations were met, and factors that can explain OFD companies' performance or customers' satisfaction during COVID-19 pandemic.
Most of the erstwhile work in the literature on OFD is conducted predominantly using primary data with a limited sample size. To the best of our knowledge, no study exists in the literature that considers social media-based big data relating to OFD companies during the pandemic and uses qualitative (machine learning algorithms) as well as quantitative approaches to investigate issues faced by OFD companies, service providers, and customers during the first two waves of the COVID-19 in the US and Indian market. Specifically, this study investigates how do consumers' sentiments vary on OFD companies' services during COVID-19 across different dimensions of consumer expectations, market characteristics, and companies' characteristics?
The following research objectives (RO) are considered to address the research question:
RO1: To identify the broader issues/topics prominently discussed about OFD companies and service providers during the pandemic.
RO2: To investigate consumers' net sentiment and negative sentiment across identified dimensions, OFD companies, and different countries.
RO3: To test the impact of different market characteristics and OFD companies' characteristics on consumers' sentiments.
To address these research objectives, first, we collected data for four OFD companies (i.e., Uber Eats, Grubhub, Zomato, and Swiggy) from Twitter during the first two waves of the COVID-19 pandemic. Second, the text mining approach is used to identify different topics/issues discussed on Twitter about these OFD companies. Third, for each identified topic, positive and negative sentiments of people are computed. Fourth, different regression models are developed to investigate the relationship between the moderating variables and people's sentiments. Finally, the results of both countries' companies are compared to examine the differences among the topics and customers' sentiments. The results offer several important practical insights which are helpful for OFD companies.
The remainder of the paper is organized as follows. Section 2 discusses the analyses of relevant literature. Section 3 presents the research hypotheses. Data collection, research methodology, and results are provided in Section 4 . Section 5 presents discussion and managerial insights. Finally, section 6 concludes the research and offers the scope for future studies.
2. Literature review
There was not much research conducted on the OFD area before the COVID-19 pandemic. Previous studies have mainly focused on issues such as the OFD platform's performance and customer satisfaction ( Seghezzi and Mangiaracina, 2020 ; Gilitwala and Nag, 2019 ), consumers behavior and attitude ( Pigatto et al., 2017 ; Hwang and Choe, 2019 ; Yeo et al., 2021 ), vehicle routing problems ( Liu et al., 2020 ; Correa et al., 2019 ), adoption and acceptance of technology in OFD apps ( Pigatto et al., 2017 ; Gunden et al., 2020 ; Roh and Park, 2019 ), and riders issues related to wages and health ( Bates et al., 2020 ; Correa et al., 2019 ; Kougiannou and Mendonça, 2021 ). For a detailed discussion on these issues, interested readers can refer to Li et al. (2020) and Seghezzi et al. (2021) , who have provided an excellent review of OFD literature published before the COVID-19 period.
Research on the OFD has witnessed significant growth during the last two years. One of the reasons for this rapid growth was the rapid increase in OFD services demand during the COVID-19 pandemic. For safety reasons, more people started ordering their food from home via OFD apps ( Mehrolia et al., 2021 ; Zanetta et al., 2021 ; Hong et al., 2021 , Hong et al., 2021 ), such as Uber Eats, DoorDash, Zomato, and Swiggy. Several researchers ( Hong et al., 2021 , Hong et al., 2021 ; Gani et al., 2021 ) have studied how these new behaviors of people affect the food delivery business during the COVID-19 outbreak.
As per the world health organization (WHO), more than 6.28 million people have already died because of the COVID-19 pandemic. It has affected people's life emotionally, psychologically, and physically ( Ahorsu et al., 2020 ; Conte et al., 2021; Watson and Popescu, 2021 ). People have used several ways to deal with the stress, anxiety, and loneliness caused by the COVID-19 (Kumarand Shah, 2020; Ahorsu et al., 2020 ; Lo Coco et al., 2021 ). Consumers have extensively utilized different social media platforms to share their opinion and remain updated with the public policies and regulations during the COVID-19 ( Rydell and Kucera, 2021 ; Trivedi and Singh, 2021 ). Several researchers studied how the COVID-19 has changed consumers' behavior, habits, and tastes when buying food online ( Watson and Popescu, 2021 ; Birtus and Lăzăroiu, 2021 ; Rydell and Suler, 2021 ; Smith and Machova, 2021 ). Michalikova et al. (2022) have reviewed the literature on customers' judgment, behavior intentions, and purchase decision dynamics during the COVID-19 pandemic in the food delivery sector.
COVID-19 has considerably impacted the financial strength of restaurant firms; however, restaurant firms that used OFD and other contactless delivery services experienced relatively lesser financial glitches ( Kim et al., 2021 ). Before COVID-19, the primary marketing strategies were based on product imagery, links, and sponsorships. While combatting the COVID-19 pandemic, selling social distancing, appropriating frontline workers, and accelerating digitalization were the selling points ( Jia et al., 2021 ). We classify the research conducted on OFD during the COVID-19 outbreak into three categories—customer satisfaction/experience, intention to use OFD apps, and workers/drivers/riders' conditions during operations of OFD.
The first set of studies ( Kumar and Shah, 2021 ; Mehrolia et al., 2021 ; Prasetyo et al., 2021 : Sharma et al., 2021 ) focused on customer experience and OFD companies' performance. Mehrolia et al. (2020) and Sharma et al. (2021) investigated the characteristics of customers who used and did not use online food delivery services (OFDS) during the first wave of COVID-19 in India. Customers who purchased food via OFD platforms have experienced lesser perceived threats and a high purchase pattern, perceived benefits, and product involvement ( Al Amin et al., 2021 ; Mehrolia et al., 2021 ; Sharma et al., 2021 ; Uzir et al., 2021 ). Studies found that during the COVID-19 pandemic, hedonic motivation ( Prasetyo et al., 2021 ; Sharma et al., 2021 ; Shah et al., 2021 ), food quality, variety, and safety ( Dsouza and Sharma, 2021 ; Shah et al., 2021 ), and mobile app information design/features ( Pal et al., 2021 ) significantly affect customers satisfaction and loyalty. Hedonic motivation is also important for price, information quality, and promotion ( Prasetyo et al., 2021 ; Bao and Zhu, 2022 ; Shah et al., 2021 ; Sharma et al., 2021 ; Ramos, 2021 ; Pal et al., 2021 ). Unlike other studies, Zenetta et al. (2021) found that hedonic motivation was not the most crucial factor for the continuance intention.
India is one of the most populated countries and was severely affected by the COVID-19. Several studies ( Dsouza and Sharma, 2021 ; Mehrolia et al., 2021 ; Pal et al., 2021 ) have been conducted to understand OFD companies and customers' purchase behaviors in the Indian market. Trivedi and Singh (2021) found that Zomato received the most positive and less negative customer sentiments than other competitors, such as Swiggy in India. Dsouza and Sharma (2021) found that the food quality and safety measure of OFDAs positively affects customer satisfaction and loyalty in India. Pal et al. (2021) investigate student satisfaction and loyalty to OFDAs. Their findings suggest that satisfaction is the main predictor of loyalty, whereas mobile app information design has the highest impact on satisfaction and loyalty. Chen McCain et al. (2021) assessed customers' satisfaction with Uber Eats on different dimensions—OFD apps performance, food quality, and service quality during the first wave of COVID-19 in the US. They found that service quality was the most important dimension, followed by OFD app performance and food quality.
The second set of studies focused on the continuous usage intention of OFD apps during the COVID-19 outbreak period in different countries, such as India ( Mehrolia et al., 2021 ), the USA ( Hong et al., 2021 , Hong et al., 2021 ), China ( Zhao and Bacao, 2020 ), Brazil ( Zanetta et al., 2021 ), Mexico ( Ramos, 2021 ), Vietnam ( Tran, 2021 ). These and several other studies have found that performance expectancy ( Mehrolia et al., 2021 ; Zanetta et al., 2021 ; Pal et al., 2021 ; Ramos, 2021 ), habit ( Zanetta et al., 2021 ; Rydell and Kucera, 2021 ), effort expectancy ( Kumar and Shah, 2021 ; Ramos, 2021 ; Pal et al., 2021 ), price saving orientation ( van Doorn, 2020 ; Ramos, 2021 ; Pal et al., 2021 ), perceived usefulness, and employee trust ( Gavilan et al., 2021 ; Chakraborty et al., 2022 ; Trivedi and Singh, 2021 ; Uzir et al., 2021 ) affect consumers intention to use OFD services during the COVID-19 period.
Similarly, delivery, hygiene, subjective norms, attitudes, behavioral control, and social isolation ( Al Amin et al., 2021 ; Gani et al., 2021 ; Tran, 2021 ; Sharma et al., 2021 ; Yeo et al., 2021 ; Hopkins and Potcovaru, 2021 ) positively affect the consumers' continuance intention to use Mobile food delivery apps. Further, studies have also found that OFD apps features, ease of use, convenience, price saving, and food variety ( Pigatto et al., 2017 ; van Doorn, 2020 ; Pal et al., 2021 ; Dirsehan and Cankat, 2021 ; Shah et al., 2021 ; Kumar et al., 2021 ; Bao and Zhu, 2022 ) also affect the continued intention to use the FDA. Gavilan et al. (2021) found that customers preferred innovative solutions by OFD companies during COVID-19. Kumar and Shah (2021) observed that the app aesthetics were responsible for customers' pleasure, significantly affecting the customers' continued usage intent.
The third set of studies ( Huang, 2021 ; Parwez & Ranjan, 2021 ; Puram et al., 2021 ) attempted to understand food delivery drivers' conditions during COVID-19 in China and India. The precarity of work among the food delivery workers had aggravated during COVID-19—and it impacted workers' job loss, health risks, and occupational distress ( Huang, 2021 ; Parwez & Ranjan, 2021 ). Among drivers in China, work insecurity, financial distress, health risks, livelihood crisis, and inflamed racism were also observed ( Huang, 2021 ). Puram et al. (2021) analyzed the challenges faced by last-mile food delivery riders working for different OFD platforms in India during the COVID-19 pandemic. They categorize the riders' challenges under operational, customer-related, organizational, and technological categories.
Apart from the variables discussed above, several other variables moderate or affect customers' satisfaction ( Hu et al., 2009 ), sentiments about the food delivery service ( Oliver, 1977 ; Gavilan et al., 2021 ), and intent to use and reuse OFD services ( Mittal et al., 2001 ; Kim et al., 2021 ; Gani et al., 2021 ). A large number of studies show that a firm's brand image ( Fornell, 1992 ; O'Sullivan and McCallig, 2012 ; Peng et al., 2015 ; Chai and Yat, 2019 ; Hwang and Kim, 2020 ; Prasetyo et al., 2021 ) and market value/size (Daniel et al., 2015; Dai et al., 2021 ) positively affect customers satisfaction in different service industries. The customer's expectations about the OFD service performance may vary between different waves of the COVID-19 ( Sv et al., 2021 ; Mohan et al., 2022 ). The geographic location of customers may affect their expectations and purchase intention about the product and service ( Steyn et al., 2010 ; Ng, 2013 ; Leng et al., 2019 ; Punel et al., 2019 ). Rizou et al. (2020) found similar findings in the food delivery sector as well. Studies have explored the difference in people's sentiments during the first and second waves of the COVID-19 ( Lo Coco et al., 2021 ).
None of the above studies utilize secondary data from social media platforms like Twitter, where customers regularly express their concerns, experience, and advice to OFD companies and their businesses. This data can help companies comprehensively understand customers' expectations and measure their performance during the pandemic. This paper addresses this issue by collecting consumer data on the top two Indian and US OFD companies from Twitter and exploring the issues OFD companies face by employing the text mining approach and regression analyses.
3. Research hypotheses development
In addition to the traditional OFD business market factors, several other variables may affect or moderate customers' sentiments about OFD companies' performance and service during the COVID-19. The variables and related hypotheses are discussed in the following sub-sections.
3.1. The COVID-19 waves and people's sentiments
The COVID-19 pandemic brought havoc to everyone's life and affected people in different ways—financially, emotionally, psychologically, and physically ( Trivedi and Singh, 2021 ; Conte e t al., 2021). At the beginning of the COVID-19 outbreak (i.e., in the first wave), it was difficult for OFD companies and restaurants to run their business and serve customers efficiently and safely. In the first COVID-19 wave, most OFD companies were clueless about the customers' expectations of their delivery services and how to meet them. Due to safety reasons ( Zhao and Bacao, 2020 ), customers with self-protective behavior were hesitant to order food online during the first COVID-19 wave ( Ahorsu et al., 2020 ). The primary reasons for their safety concern were lack of information about hygiene, how and who is preparing food, ingredients used in foods, source of ingredients, and safety measures adopted at restaurants ( Gavilan et al., 2021 ).
However, to address these concerns, soon, OFD companies started adopting government regulations, offered contactless delivery, and implemented several measures for customers' safety ( Nguyen and Vu, 2020 ). During the first COVID-19 wave, OFD companies have learned to meet consumers' expectations in the best way possible. As COVID-19 time passed, OFD service providers started offering more information about safety measures adopted by them to consumers (Keeble et al., 2021). Therefore, in the second wave of COVID-19, companies' performance and consumers' sentiment about their performance should improve. Literature suggests a difference in people's sentiments between the first and second wave of the COVID-19 ( Kohút et al., 2021 ; Sv et al., 2021 ; Mohan et al., 2022 ), which also suggests people's psychological adoption of the new normal situation ( Koppehele-Gossel et al., 2022 ) as well satisfaction with improved services of OFD firms. We believe customers' sentiments on the operations of OFD companies ( Kim et al., 2021 ) should vary from COVID-19 first to the second wave. Therefore, we hypothesized:
COVID-19 waves moderate people's sentiments.
3.2. Market size and public listing impact on people's sentiments
From the expectancy theory perspective ( Oliver, 1977 ), customers will have specific expectations from a firm based on their previous experiences, influencing their behaviors to achieve specific goals. Before the COVID-19 outbreak, OFD companies with a bigger market size and listed publicly may have provided better customer experiences. Customers would be expecting similar services during the COVID-19 period as well. Some OFD companies (e.g., Zomato and Groubhub) are publicly listed and have a better brand value than others ( Savitri et al., 2020 ; Yao et al., 2021 ). OFD companies with more resources can invest liberally to bring innovation to delivery operations to handle the COVID-19 healthcare crisis and market them to the consumers. Different innovative OFD options are discussed in the literature ( Keeble et al., 2020 ; Richardson, 2020 ; Shah et al., 2021 ; Sharma et al., 2021 ; Bao and Zhu, 2022 ), which helps in reducing customers' fear of safety concerns and increases their experiential value ( Gavilan et al., 2021 ) during COVID-19. Such companies can be more successful in conveying their safety-related measures to consumers.
Numerous studies ( O'Sullivan and McCallig, 2012 ; Peng et al., 2015 ) have investigated how a firm's value affects customer satisfaction. Bolton et al. (2004) and Luo et al. (2010) found that customer satisfaction significantly affects the firm's value in the stock market and vice-versa. It is established in the literature that high firm value not only helps increase customer satisfaction ( Hu et al., 2009 ) but also enhances its brand image ( Prasetyo et al., 2021 ), purchase and repurchase intention ( Bao and Zhu, 2022 ), customer retention ( Mittal et al., 2001 ), and reduces complaints ( Fornell, 1992 ). Dai et al. (2021) studied the impact of the COVID-19 outbreak on small and medium-sized enterprises (SMEs) across different industries during the first and second waves. They found that during the second wave, companies were more prepared based on their learning from the first wave. Daniel et al. (2005) find a higher correlation between a firm's market values and customers' confidence in the firm. Based on the above studies, one can conclude that if a firm has a higher market size or value and is listed publicly, it leads to high customer confidence or sentiments in the firm.
The market size of OFD companies moderates people's sentiments .
Public listing of OFD companies moderates people's sentiments.
3.3. The brand image and people's sentiments
The brand image of a food company is crucial for customers to use their OFD service ( Prasetyo et al., 2021 ). In the restaurant industry, brand image is defined as “emotions, ideas or attitudes that customers associate with full-service dining restaurants” ( Jin et al., 2012 ). It is established in the literature that customers prefer to purchase products or services from well-known brands as they offer high-quality food ( Aaker and Equity, 1991 ). During the COVID-19 outbreak, several restaurants have increased their presence on OFD platforms to increase brand awareness ( Chai and Yat, 2019 ; Hwang and Kim, 2020 ). In general, a better brand image helps increasing consumer trust, purchase intention ( Erdem and Swait, 2004 ), and customer satisfaction with a better service and quality ( Baek et al., 2010 ). Aureliano-Silva et al. (2022) argued that brand love and service recovery are important for purchase intention and brand trust in food delivery platforms. Studies show that brand love is instrumental to customer satisfaction and intentions for future purchases ( Han et al., 2011 ; Erkmen and Hancer, 2019 ; Bao and Zhu, 2022 ).
Ibrahim et al. (2017) found a positive relationship between consumer sentiments on Twitter and the company's brand image. A positive brand image creates a good reputation for the company in customers' minds for a long time, and it increases customer loyalty toward a high brand image company ( Pitta and Katsanis, 1995 ). Customers heavily rely on a company's brand image when they are concerned about uncertain product or service quality ( Berry, 2000 ; Erdem and Swait, 2004 ), food safety, and quality under uncertain times like the COVID-19 outbreak ( Kim et al., 2021 ). A company's brand image positively affects customer loyalty and is a significant predictor of customer satisfaction in the restaurant ( Hwang and Kim, 2020 ) and other industries ( Jin et al., 2012 ; Ryu et al., 2012 ; Faullant et al., 2008 ). The high brand image of a food delivery firm positively affects the customer's behavioral intention to use OFD service ( Hwang and Choe, 2019 ; Gani et al., 2021 ). Therefore, we hypothesized:s.
The brand image of OFD companies moderates people's sentiments.

3.4. Operating regions and people's sentiments
The customers' expectations about the same products and services may vary between different regions based on different factors such as behaviors, loyalty, attitudes, and cultural influence ( Steyn et al., 2010 ). Punel et al. (2019) found that customers' experiences and expectations about the same airline service vary based on customers' geographical region. The authors find a difference in the Noth American and Asian passengers' expectations of the ticket price and in-flight service. Some studies show that cultural regions moderate online purchase intention ( Ng, 2013 ). Leng et al. (2019) observed differences in the US and Japanese people's choices and expectations about food products and services.
Twitter is a popular social media platform where people freely express their feelings. People's reactions on Twitter to the COVID-19 varied in different regions. For example, the US people's sentiment score was more negative than the UK people toward the COVD-19 ( Zou et al., 2020 ). Similarly, the fear and awareness of the COVID-19 safety measures vary from country to country Rizou et al. (2020) ; Lo Coco et al. (2021) . Consumers' expectations and sentiments about the OFD service providers may also vary according to their country ( Keeble et al., 2020 ), as consumers in developed countries would be more educated and aware and, hence, have more expectations. In India, the OFD services business is growing exponentially. However, it is still new compared to the US market. Thus, OFD companies may focus more on consumer-centric service delivery in developed countries than in developing countries. Variation in the above factors should change customer sentiment. Therefore, we hypothesize that:
Operating regions (i.e., countries) of OFD companies moderate people's sentiments .
4. Research methodology and results
We have collected consumer data for four popular OFD companies from India (e.g., Zomato and Swiggy) and from the US (e.g., Uber Eats and Grubhub). The consumer-level data were extracted from Twitter —a social networking site—on which billions of users express their ideas and experiences. Each post by a user is known as a “tweet”. The customer-level data were collected between February 01, 2020 to November 30, 2021 covering the first two COVID-19 waves.
As shown in Fig. 1 , in India, the first COVID-19 wave is considered from March 01, 2020 to February 28, 2021 and the second wave is considered from March 01, 2021 to November 30, 2021. For the US, the first wave is considered from February 01, 2020 to June 30, 2021 and the second wave is considered from July 01, 2021 to November 30, 2021. The second wave in India brought more negative impacts as the number of new daily cases and fatality rate were significantly higher than the first wave. The second wave inflicted more fear on people's minds as the number of people hospitalized, and the load on medical infrastructure were significantly higher than the first wave of COVID-19.

COVID-19 cases and deaths in India and US.
Fig. 2 summarizes the research methodology adopted in this paper. Primarily, a four steps method was utilized for: (i) data collection, (ii) topic extraction, (iii) sentiment analysis, and (iv) moderation variable analysis. We used the company name, their Twitter handle, and related hashtags as keywords to extract the data. We extracted a total sample of 11,134 for Zomato, 14,355 for Swiggy, 15,583 for Uber Eats, and 2030 for Grubhub. The data were preprocessed by employing language identification, cleaning, tokenization, lemmatization, and removing stopwords. We kept data in English for further analysis, leading to a useful sample of 9447 for Zomato, 13,160 for Swiggy, 12,536 for Uber Eats, and 1951 for Grubhub. The remaining three steps are described in subsections 4.1, 4.2, and 4.3.

The proposed research methodology framework.
4.1. Text analytics and topic extraction
We employed the latent Dirichlet allocation (LDA) tool ( Blei et al., 2003 ) to identify hidden topics discussed in the large volume of unstructured data, as it does not assume any structure of grammar properties. The model was trained to identify the number of topics in unstructured documents. The extant literature ( Nikolenko et al., 2017 ; Lee, 2022) suggests a coherence score as a more reliable measure to discover the most prominent number of topics in a large volume of documents. Fig. 3 shows the variation of coherence score over topics 2 to 40 for each OFD company.

Coherence score of each OFD company.
We found the highest coherence score for each OFD company when the number of topics extracted was 13 for Zomato, 6 for Swiggy, 2 for Uber Eats, and 11 for Grubhub. We believe that there must be more than two topics related to Uber Eats discussed by customers, so we preferred to extract topics that correspond to the next highest coherence score. It results in 7 topics for Uber Eats. Topics and their associated 10 most important keywords of Zomato, Swiggy, Uber Eats, and Grubhub are presented in Table 1 , Table 2 , Table 3 , Table 4 , respectively.
Keywords, topics, and dimensions of Zomato and their statistics.
Dimension | Topics | Keywords | Positive | Negative | ||||
---|---|---|---|---|---|---|---|---|
mean | std | max | mean | std | max | |||
Social responsibility | Help in providing food to needy during lockdown | daili, support, wager, donat, help, feed, provid, coronaviru, food, lockdown | 0.14 | 0.119 | 0.505 | 0.076 | 0.091 | 0.651 |
Impact of staff/workers' salary on food delivery business | employe, busi, impact, worker, deliveri, food, staff, swiggi, uber, salari | 0.067 | 0.084 | 0.469 | 0.079 | 0.092 | 0.388 | |
Riders' help in delivering donated food and groceries | help, deliveri, work, groceri, delhi, donat, food, rider, launch, deliv | 0.135 | 0.122 | 0.574 | 0.057 | 0.081 | 0.411 | |
Delivery operations | Responsiveness of food delivery person | food, order, time, come, corona, foodi, think, action, carri, know | 0.104 | 0.104 | 0.535 | 0.082 | 0.088 | 0.478 |
Pizza and food delivery during home quarantine | deliveri, food, pizza, quarantin, isol, home, swiggi, posit, test, thank | 0.117 | 0.117 | 0.672 | 0.061 | 0.068 | 0.351 | |
Customized food orders by following corona appropriate protocols | deliveri, corona, order, food, viru, custom, time, contactless, mask, deliv | 0.108 | 0.101 | 0.571 | 0.087 | 0.096 | 0.573 | |
Perceived responsibility of consumers | Stay home and order food from restaurants during quarantine time | order, food, swiggi, restaur, quarantin, corona, stay, time, deliv, stayhom | 0.109 | 0.105 | 0.545 | 0.06 | 0.089 | 0.504 |
Fight corona and save people's life by having food products delivered at home | corona, life, swiggi, quarantin, avoid, deliveri, indiafightscorona, soon, peopl, product | 0.104 | 0.115 | 0.577 | 0.066 | 0.101 | 0.583 | |
Use food delivery services to stop spread of corona virus among people | food, corona, peopl, order, deliveri, stop, deliv, servic, spread, time | 0.099 | 0.094 | 0.474 | 0.096 | 0.099 | 0.623 | |
Financial impact of COVID-19 | Loss of income in food delivery business nationally | food, hous, lose, nation, delivers, medicin, incom, leav, coronaviruspandem, live | 0.037 | 0.078 | 0.415 | 0.078 | 0.063 | 0.302 |
Consumers' purchase behaviors | Preferences of gold (highly) rated restaurants membership during corona outbreak | corona, serv, thing, restaur, outbreak, gold, food, face, membership, lockdown | 0.065 | 0.116 | 0.623 | 0.105 | 0.12 | 0.487 |
Perceived solutions | Indian food delivery startup business help fighting corona | swiggi, indian, corona, compani, startup, amazon, fund, fight, busi, flipkart | 0.112 | 0.101 | 0.624 | 0.067 | 0.087 | 0.505 |
Online food delivery order to stop corona virus spread | deliveri, swiggi, lockdown, coronaviru, home, corona, deliv, food, onlin, spread | 0.076 | 0.088 | 0.412 | 0.047 | 0.073 | 0.636 |
Keywords, topics, and dimensions of Swiggy and their statistics.
Positive | Negative | |||||||
---|---|---|---|---|---|---|---|---|
Dimension | Topics | Keys | Mean | Max | Std. | Mean | Max | Std. |
Perceived consumers' responsibility | Stay home and have food delivered from restaurants during lockdown | deliveri, home, food, corona, stay, restaur, work, lockdown, time, amazon | 0.108 | 0.594 | 0.109 | 0.058 | 0.503 | 0.08 |
Online food delivery may help in reducing the corona virus spread among people | deliveri, corona, food, viru, order, deliv, spread, peopl, onlin, like | 0.110 | 0.6 | 0.098 | 0.065 | 0.536 | 0.08 | |
Social responsibility | Help hungry (needy) people by a delivery chain through food delivery during corona | deliveri, corona, help, peopl, partner, fund, work, need, relief, hunger | 0.128 | 0.67 | 0.111 | 0.071 | 0.512 | 0.081 |
Negative impact of COVID-19 on OFD business | Lockdown prevented/affected food delivery operations | lockdown, quarantin, order, oper, coronaviru, food, prevent, current, poor, foodi | 0.106 | 0.778 | 0.117 | 0.035 | 0.375 | 0.06 |
Negative impacts of corona virus on online food delivery business | employe, food, coronaviru, busi, deliveri, lockdown, uber, startup, impact, Indian | 0.066 | 0.623 | 0.095 | 0.051 | 0.506 | 0.089 | |
Delivery operations | More customized food orders (delivery time, service, refund) from restaurants during corona | order, custom, food, time, deliv, restaur, servic, corona, deliveri, refund | 0.102 | 0.674 | 0.103 | 0.103 | 0.586 | 0.097 |
Keywords, topics, and dimensions of Ubereats and their statistics.
Positive | Negative | |||||||
---|---|---|---|---|---|---|---|---|
Dimension | Topics | Keys | Mean | Std. | Max | Mean | Std. | Max |
Importance of Coupon/promocode on food delivery order | Promocode accounts for more food orders during coronavirus | order, coronaviru, code, discount, quarantin, ubereatspromocod, promocod, account, ubereatsuk, coronavirusuk | 0.032 | 0.078 | 0.457 | 0.005 | 0.043 | 0.701 |
Problems with discount coupons in food delivery order during quarantine | order, quarantin, spend, code, account, problem, discount, ubereatspromocodecod, vhoplook, vhopif | 0.03 | 0.073 | 0.496 | 0.095 | 0.054 | 0.369 | |
Financial impact of COVID-19 | In quarantine, people think about working to get money | quarantin, peopl, netflix, week, money, think, work, isol, month, watch | 0.093 | 0.101 | 0.808 | 0.081 | 0.096 | 0.603 |
Delivery operations | Drivers and restaurants' food delivery time and service | deliveri, food, driver, restaur, order, time, deliv, peopl, servic, leav | 0.086 | 0.086 | 0.579 | 0.072 | 0.082 | 0.484 |
Free delivery service | Restaurants help local people by offering free delivery services during lockdown | deliveri, restaur, busi, help, support, local, servic, offer, free, lockdown | 0.108 | 0.102 | 0.532 | 0.055 | 0.081 | 0.519 |
People want free home delivery during quarantine/coronavirus | corona, viru, quarantin, peopl, deliv, want, free, home, thank, stay | 0.123 | 0.122 | 0.647 | 0.072 | 0.095 | 0.47 | |
Perceived consumers' responsibility | Need for home food delivery to stay in isolation during the self-quarantine period | quarantin, self, isol, food, home, like, deliveri, deliv, stay, need | 0.104 | 0.103 | 0.612 | 0.084 | 0.095 | 0.613 |
Keywords, topics, and dimensions of Grubhub and their statistics.
Positive | Negative | |||||||
---|---|---|---|---|---|---|---|---|
Dimension | Topics | Keys | mean | std | max | mean | std | max |
People's supports in adapting to pickup/takeout service | Avail takeout and pickup service for food and alcohol | deliveri, coronaviru, food, takeout, pickup, chicagoindustri, alcohol, avail, dinneridea, luckysandwich | 0.068 | 0.103 | 0.512 | 0.037 | 0.101 | 0.730 |
Restaurants and drivers need local support to provide service | restaur, food, deliveri, support, servic, order, driver, local, need, like | 0.130 | 0.112 | 0.552 | 0.075 | 0.088 | 0.519 | |
Food delivery operations | Longer delivery time caused order cancellation | order, driver, quarantin, food, time, know, like, deliveri, cancel, deliv | 0.091 | 0.089 | 0.355 | 0.083 | 0.091 | 0.463 |
People like food order delivered on time from restaurants and drivers | deliveri, quarantin, time, deliv, restaur, order, driver, food, like, busi | 0.106 | 0.094 | 0.409 | 0.072 | 0.08 | 0.454 | |
People want home food delivery from restaurants and drivers | food, deliveri, quarantin, want, order, peopl, driver, like, restaur, home | 0.116 | 0.106 | 0.458 | 0.078 | 0.094 | 0.574 | |
Free delivery service | Restaurant food delivery offers needed to save people | restaur, corona, deliveri, quarantin, save, peopl, money, deliv, need, offer | 0.101 | 0.115 | 0.532 | 0.094 | 0.115 | 0.5 |
People like free food delivery offers during corona spread | deliveri, food, corona, deliv, peopl, like, quarantin, spread, free, offer | 0.143 | 0.122 | 0.55 | 0.054 | 0.072 | 0.279 | |
Appreciation for drivers/restaurants for delivery service | Thank gift card to food delivery drivers for risking their life during corona | deliveri, quarantin, driver, risk, gift, compani, order, card, thank, meal | 0.124 | 0.115 | 0.496 | 0.084 | 0.093 | 0.351 |
Thanking drivers for working, wearing masks, and delivery service | peopl, deliveri, mask, food, thank, come, servic, work, like, wear | 0.123 | 0.11 | 0.527 | 0.091 | 0.101 | 0.474 | |
Delivery drivers working during the COVID-19 outbreak help greatly people and restaurant business | quarantin, work, driver, order, busi, help, peopl, start, great, restaur | 0.119 | 0.12 | 0.633 | 0.068 | 0.087 | 0.522 | |
Need to thank restaurants and drivers for offering free food delivery | order, deliveri, quarantin, food, driver, free, thank, place, need, restaur | 0.132 | 0.116 | 0.548 | 0.084 | 0.1 | 0.513 |
Topics extraction results are analyzed in three steps: (i) an expert team reviewed keywords of each topic and gave an appropriate name, as shown in Table 1 , Table 2 , Table 3 , Table 4 , (ii) each topic was then reviewed and higher dimensions which were a collection of topics were formed (see Table 1 , Table 2 , Table 3 , Table 4 ), keeping literature in mind, and (iii) based on percent tweets related to the dimensions (see Table 5 ), most prominent dimensions of each food delivery company and regions were identified and discussed in Section 5 . To label topics, the keywords of each topic were discussed and reviewed carefully by experts from academics. Further, we observed that a set of topics represents a higher-level dimension. Thus, we identified sets of topics representing higher-level dimensions. These dimensions are also shown in Table 1 , Table 2 , Table 3 , Table 4 .
Performance of delivery companies across different dimensions.
positive | negative | |||||||||
---|---|---|---|---|---|---|---|---|---|---|
Dimension | count | Percent | mean | std | min | max | mean | std | min | max |
S. responsibility | 1803 | 19.08% | 0.110 | 0.113 | 0 | 0.574 | 0.071 | 0.089 | 0 | 0.651 |
Delivery operations | 2367 | 25.06% | 0.110 | 0.107 | 0 | 0.672 | 0.078 | 0.088 | 0 | 0.573 |
P. C. responsibility | 2579 | 27.30% | 0.104 | 0.101 | 0 | 0.577 | 0.078 | 0.097 | 0 | 0.623 |
Financial impact | 119 | 1.26% | 0.037 | 0.078 | 0 | 0.415 | 0.078 | 0.063 | 0 | 0.302 |
Consumers PB | 515 | 5.45% | 0.065 | 0.116 | 0 | 0.623 | 0.105 | 0.12 | 0 | 0.487 |
P. solutions | 2064 | 21.85% | 0.089 | 0.095 | 0 | 0.624 | 0.054 | 0.079 | 0 | 0.636 |
S. responsibility | 1796 | 13.65% | 0.128 | 0.111 | 0 | 0.67 | 0.071 | 0.081 | 0 | 0.512 |
Delivery operations | 2785 | 21.16% | 0.102 | 0.103 | 0 | 0.674 | 0.103 | 0.097 | 0 | 0.586 |
P. -ve impact | 2963 | 22.52% | 0.078 | 0.104 | 0 | 0.778 | 0.046 | 0.082 | 0 | 0.506 |
P. C. responsibility | 5616 | 42.67% | 0.109 | 0.103 | 0 | 0.6 | 0.062 | 0.08 | 0 | 0.536 |
Promocode and food delivery | 1790 | 14.28% | 0.031 | 0.075 | 0 | 0.496 | 0.052 | 0.066 | 0 | 0.701 |
Delivery operations | 3817 | 30.45% | 0.086 | 0.086 | 0 | 0.579 | 0.072 | 0.082 | 0 | 0.484 |
Financial impact | 2058 | 16.42% | 0.093 | 0.101 | 0 | 0.808 | 0.081 | 0.096 | 0 | 0.603 |
Fee delivery | 2579 | 20.57% | 0.115 | 0.112 | 0 | 0.647 | 0.063 | 0.088 | 0 | 0.519 |
P. C. responsibility | 2292 | 18.28% | 0.104 | 0.103 | 0 | 0.612 | 0.084 | 0.095 | 0 | 0.613 |
Adapting to pickup service | 391 | 20.04% | 0.110 | 0.113 | 0 | 0.552 | 0.063 | 0.094 | 0 | 0.73 |
Delivery operations | 640 | 32.8% | 0.103 | 0.096 | 0 | 0.458 | 0.078 | 0.088 | 0 | 0.574 |
Free delivery | 282 | 14.45% | 0.126 | 0.121 | 0 | 0.55 | 0.071 | 0.094 | 0 | 0.500 |
Appreciation for drivers/restaurants | 638 | 32.7% | 0.124 | 0.115 | 0 | 0.633 | 0.081 | 0.095 | 0 | 0.522 |
Further, we also extracted topics based on the COVID-19 waves to check if there is any difference in topics. The results show that in India, people discussed more regarding help and support for drivers and restaurants, vaccination requests, customized orders, and food quality in the second COVID-19 wave. In the first wave, we observed more discussion about consumers' perceived responsibility, perceived solutions, and companies' social responsibility. On the other hand, US people discussed more about coupons and promocode during the first COVID-19 wave. Whereas in the second wave, people emphasize more about vaccination, money spending, and delivery operations in the US market.
4.2. Sentiment analysis
Sentiment analysis is a procedure to quantify customers' experiences or emotions based on the subjective text data expressed by the customers (Lee, 2022). The sentiment was labeled based on the python package, Gensim/VADER. We preferred the VADER-based model as they provide a parsimonious rule -or lexicon-based model specially designed for social media text sentiment analysis. Social media text, especially tweets data, is characterized as short sentences/descriptions with emoji, copious use of question marks and exclamation marks, and repetitive words. This technique of unsupervised sentiment computation does not require features and is widely used in the literature ( Ibrahim and Wang, 2019 ; Lee, 2022; Mehta et al., 2021 ).
Customer sentiment may include positive, negative, or neutral (Lee, 2022; Liu et al., 2021), depending upon VADER based compound score that varies from −1 to +1. If the compound score was ≤ −0.05, between −0.05 and +0.05, and ≥ +0.05, the sentiment was labeled as negative, neutral, and positive (Lee, 2022). Lexicon-based sentiment computation approach matches words with a dictionary of words. First, we assessed the sentiment score of each tweet. Subsequently, we assessed topic-level and dimension-level sentiments using dictionaries or a pre-defined list of words approach. The topic- and dimension-level sentiment was determined by averaging the tweet- and topic-level sentiments, respectively. Dimension-level positive and negative sentiments of delivery companies are shown in Table 5 . Based on the mean score of positive and negative sentiment, the results are further discussed in section 5 .
4.3. Moderating variables
To test hypotheses, we considered two types of sentiment: net sentiment and negative sentiment, as response variables. Five important variables—COVID-19 wave, brand, market size, country, and listed, may influence customers' both types of sentiment/satisfaction. We employ the ordinal least square (OLS) regressions to estimate response variables, net sentiment, and negative sentiment. The relationship between the response variable and explanatory variables takes the form of equations (1) , (2) .
The COVID-19 wave, country, and “listed” are binary variables. The COVID-19 wave has two labels —the first and the second wave. The country has two labels—India and US. Similarly, listed has labels yes and no, where yes implies a company is listed in the stock market, and no for otherwise. Zomato and Grubhub are publicly listed OFD companies, while Uber Eats and Swiggy are not. The brand and market size are categorical variables, where the brand has four labels—Zomato, Swiggy, Uber Eats, and Grubhub. The market size has three labels: small, medium, and large. With a nearly $80.53 billion market cap, Uber Eats is considered a large-market size company. Zomato, Swiggy, and Grubhub, with a market cap of nearly $13.82 billion, $5 billion, and $10.55 billion, are considered medium, small, and medium market sizes, respectively.
The ordinary least squares (OLS) regression results are presented in Table 6 . The results show that there is a significant relationship between net sentiment and explanatory variable, the COVID waves (p < 0.05), brand (p < 0.05), market size (p < 0.05), country (p < 0.05), and listed (p < 0.10). Thus, the hypotheses H1 , H2 , H3 , H4 , and H5 are supported for the net sentiment. For the negative sentiment, we found that all the explanatory variables are significant (p < 0.05) except the country (p > 0.10). Therefore, hypotheses H1 , H2 , H3 , and H4 are supported for the negative sentiment; hypothesis H5 is rejected.
OLS regression results for hypotheses.
Hypotheses | Response variable | Explanatory variable | F-statistic | Prob (F-statistic) | Conclusion |
---|---|---|---|---|---|
Net sentiment (Positive – Negative) | COVID waves | 6.225 | 0.0126 | Supported | |
Brand | 35.33 | 0.00 | Supported | ||
Market size | 47.17 | 0.00 | Supported | ||
Country | 52.12 | 0.00 | Supported | ||
Listed | 3.37 | 0.0663 | Supported | ||
Negative | COVID waves | 29.10 | 0.00 | Supported | |
Brand | 6.623 | 0.00018 | Supported | ||
Market size | 9.525 | 0.00 | Supported | ||
Country | 1.853 | 0.173 | Not Supported | ||
Listed | 13.20 | 0.00 | Supported |
To identify specific pairs of groups that differ from each other, we conducted the Post-Hoc test of multiple comparisons of means—Tukey Honestly Significant Difference (Tukey HSD). The results are shown in Table 7 and discussed in the Discussion section.
Multiple comparison test results.
Response variable | Explanatory variable | Group1 | Group2 | Mean difference | p-adj | Lower bound | Upper bound | Reject Hypothesis |
---|---|---|---|---|---|---|---|---|
Net sentiment (positive – negative) | COVID waves | First | Second | 0.005 | 0.0126 | 0.0011 | 0.009 | True |
Brand | Grubhub | Swiggy | −0.0048 | 0.5232 | −0.014 | 0.0043 | False | |
Grubhub | Ubereats | −0.0223 | 0.001 | −0.0315 | −0.0132 | True | ||
Grubhub | Zomato | −0.0125 | 0.0036 | −0.0219 | −0.0031 | True | ||
Swiggy | Ubereats | −0.0175 | 0.001 | −0.0222 | −0.0128 | True | ||
Swiggy | Zomato | −0.0076 | 0.001 | −0.0127 | −0.0025 | True | ||
Ubereats | Zomato | 0.0099 | 0.001 | 0.0047 | 0.015 | True | ||
Market size | High | Low | 0.0175 | 0.001 | 0.0132 | 0.0218 | True | |
High | Medium | 0.012 | 0.001 | 0.0075 | 0.0165 | True | ||
Low | Medium | −0.0055 | 0.0098 | −0.0099 | −0.0011 | True | ||
Country | India | USA | −0.0113 | 0.001 | −0.0144 | −0.0082 | True | |
Listed | No | Yes | 0.003 | 0.0663 | −0.0002 | 0.0063 | False | |
Negative | COVID waves | First | Second | 0.0065 | 0.001 | 0.0041 | 0.0089 | True |
Brand | Grubhub | Swiggy | −0.0066 | 0.0118 | −0.0121 | −0.0011 | True | |
Grubhub | Ubereats | −0.0039 | 0.268 | −0.0094 | 0.0016 | False | ||
Grubhub | Zomato | −0.002 | 0.7771 | −0.0076 | 0.0037 | False | ||
Swiggy | Ubereats | 0.0027 | 0.0737 | −0.0002 | 0.0055 | False | ||
Swiggy | Zomato | 0.0046 | 0.001 | 0.0015 | 0.0076 | True | ||
Ubereats | Zomato | 0.0019 | 0.3891 | −0.0012 | 0.005 | False | ||
Market size | High | Low | −0.0027 | 0.0413 | −0.0052 | −0.0001 | True | |
High | Medium | 0.0022 | 0.1212 | −0.0004 | 0.0049 | False | ||
Low | Medium | 0.0049 | 0.001 | 0.0023 | 0.0076 | True | ||
Country | India | USA | 0.0013 | 0.1734 | −0.0006 | 0.0031 | False | |
Listed | No | Yes | 0.0036 | 0.001 | 0.0017 | 0.0056 | True |
5. Discussions and managerial implications
The topic modeling results (from Table 1 , Table 2 , Table 3 , Table 4 ) convey the prominence of 13 topics for Zomato, 6 topics for Swiggy, 7 for Uber Eats, and 11 for Grubhub. These topics are further used to form higher-level dimensions that resulted—6 dimensions for Zomato, 4 dimensions for Swiggy, 5 dimensions for Uber Eats, and 4 dimensions for Grubhub. We find a wide range of topics (and dimensions) relating to OFD companies were expressed and discussed during the COVID-19 pandemic. Delivery operations, social responsibility, perceived consumers responsibility, the financial impact of COVID-19 on consumers, consumers perceived behaviors, perceived solutions, perceived negative impact of OFD, promocode and food delivery, free delivery, adapting to pickup/takeout service, and appreciation for drivers/restaurants are dimensions that emerged from our analysis.
As count or percent contribution is shown in Table 5 , overall, perceived consumer responsibility, delivery operations, social responsibility, perceived negative impact, free delivery, etc., are the most prominent dimensions related to OFD companies across emerging and developed countries. These findings differ from the previous literature ( Chen McCain et al., 2021 ; Dsouza and Sharma, 2021 ; Shah et al., 2021 ; Trivedi and Singh, 2021 ) that observed food quality and safety measures, socialization, and marketing are the main themes for OFD companies during COVID-19. Our topics seem more enlightening and COVID-19 pandemic-oriented rather than topics related to the usual expectations from OFD companies. Reasons for the difference may be due to the fact that this study is more comprehensive in terms of the number of companies included, the sample size considered, and the time period during which the sample was collected.
Table 5 further conveys that the mean score of the positive sentiment of most dimensions is more than the mean score of a negative sentiment of most dimensions of all OFD companies. Exceptions to this are financial impact and consumers' perceived behaviors of Zomato; delivery operations of Swiggy; promocode and food delivery of Uber Eats. These findings are different from the findings of Chen McCain et al. (2021) in the sense that they found that negative sentiment is more than positive sentiment.
Most of the identified topics for Indian firms Zomato and Swiggy belong to delivery operations, social responsibility, and perceived consumer responsibility dimensions (see Table 1 ). However, most of the topics of Uber Eats operating in the US belong to promocode/coupon on food delivery orders and free delivery. In contrast, for Grubhub, most topics belong to the appreciation of drivers/restaurants for delivery service and food delivery operations. Interestingly, a closer look at the topics and dimensions of Zomato and Swiggy conveys that people in India are more concerned about society, such as the social responsibility of OFD companies and the responsibility of consumers to society in such a hard time. People are more inclined toward spreading awareness and highlighting the responsibility of both consumers and delivery companies. As expected for OFD companies, we also found people discussing delivery operations dimension concerning to responsiveness of OFD companies and customized orders by following COVID-19 appropriate behaviors. Results in Table 5 show that perceived customer responsibility, delivery operations, and social responsibility are the three most prominent dimensions in the Indian market. On the other hand, delivery operations, fee delivery, and perceived customer responsibility are the three most prominent dimensions in the US.
Table 8 shows our identified dimensions and compares them with dimensions discussed in the literature. We observe that most of the existing studies ( Kim et al., 2021 ; Pal et al., 2021 ) deal with the operational aspect of delivery, while a few studies ( Li et al., 2020 ; Yeo et al., 2021 ) highlight the sustainability aspect of OFD companies, discount and promotion ( Kim et al., 2021 ), and concerned for drivers ( Huang, 2021 ; Ramos, 2021 ). However, we find perceived customer responsibility (contributing 28.27 percent) is the most important dimension, followed by delivery operations (contributing 25.9 percent) during the COVID-19 (see Table 5 ). Interestingly, compared to the existing literature, this study finds perceived consumers' responsibility, COVID-19 impacts on delivery business, benefits of competition, free food delivery service, and pickup/takeout services as unique concerns expressed and discussed by customers during the COVID-19 pandemic. During the COVID-19 pandemic, customers in India want OFD companies to be more socially sensitive, while US customers are concerned about discounts, coupons, free food delivery, etc.
Identified dimensions in this study and comparison with dimensions in literature.
Companies | Identified dimensions | Related dimension in literature |
---|---|---|
Zomato, Swiggy | Social responsibility | OFDA Sustainability ( ) |
Social influence ( ; ; ; Zahao & Bacoa, 2020) | ||
Zomato, Swiggy, UberEats, GrubHub | Delivery operations | Delivery Operations ( ) |
Delivery Performance ( ) | ||
System Operational Transparency ( ) | ||
Delivery quality ( ) | ||
Order and delivery ( ) | ||
Food delivery hygiene (Tran, 201) | ||
Zomato, Swiggy, UberEats | Perceived customers' responsibility | |
Zomato, Swiggy, UberEats | Financial impact of COVID-19 | |
Zomato | Benefits of competition | |
UberEats | Importance of Coupon/promocode on food delivery order | Discounts on delivery ( ) |
Price and Promotion ( ) | ||
UberEats, Grubhub | Free food delivery service | |
Grubhub | Pick up/takeout service | |
Grubhub | Appreciation or support for drivers service | Drivers' safety ( ; ) |
Drivers work-life ( ) | ||
Solidarity with riders (Tassinari & Maccarrone, 2020) |
We further observed that the net sentiment (i.e., positive-negative ) differs across COVID-19 waves, brands, market sizes, countries, and listed (see Table 6 , Table 7 ). Net sentiment during the second COVID-19 wave is significantly higher than that of the first COVID-19 wave. However, we also found that negative sentiment in the second wave (0.0761) was higher than in the first wave (0.0696) and significantly different (p < 0.05). It conveys that even though negative sentiment increased in the second wave, OFD companies have used their learning and experiences from the first wave and therefore could improve net sentiment during the second wave of COVID-19. Our findings are different from the literature ( Hong et al., 2021 , Hong et al., 2021 ) that finds no moderation effect of COVID-19 on the relationship between explanatory variables and intention to use OFD apps. We find that COVID-19 waves significantly influence customer sentiment, which aligns with the literature ( Birtus and Lăzăroiu, 2021 ; Mehrolia et al., 2021 ; Watson and Popescu, 2021 ), that observed that COVID-19 reshaped customers behaviors, attitude, and expectations.
Analyzing net sentiment across brands, we find that except for Grubhub and Swiggy (p > 0.05), all other pairs of brands are significantly different based on the net sentiment. Grubhub's mean net sentiment (0.0398) is significantly higher (p < 0.05) than Uber Eats (0.0175) and Zomato (0.0274). It shows that Grubhub performed better than other OFD companies. Swiggy received the second-highest mean net sentiment score (0.035), which is significantly higher (p < 0.05) than the net sentiment of Uber Eats and Zomato (see Table 7 ). Finally, Zomato's net sentiment is significantly higher (p < 0.05) than Uber Eats. Overall, Grubhub received the highest net sentiment, which is significantly higher than all other OFD companies. In India, Swiggy performed better than Zomato, whereas Grubhub did better than Uber Eats in the US. Grubhub also received the highest negative sentiment (0.07489), followed by Zomato (0.0729), Uber Eats (0.0710), and Swiggy (0.0683).
Table 7 further conveys that Grubhub's and Zomato's negative sentiments are significantly (p < 0.05) higher than Swiggy's negative sentiments. The negative sentiment of the remaining pair of companies does not differ significantly. Our findings indicate that brands play an important role in driving customer sentiment. This finding is aligned with previous literature ( Gani et al., 2021 ) that observed that restaurant reputation influences OFD apps' usefulness during the COVID-19 pandemic. These findings are different from the literature ( Prasetyo et al., 2021 ) that discovers restaurant credibility does not impact the intention to use OFD apps. It also differs from Trivedi and Singh (2021) , who observed more positive and less negative sentiment for Zomato than Swiggy.
Observing the importance of market size, we found that OFD companies with low, medium, and large market sizes received a mean net sentiment score of 0.0350, 0.0295, and 0.0175, respectively. Further, Table 7 clearly shows that low and medium market size companies have significantly higher (p < 0.05) mean net sentiment scores than large market size companies. This interesting finding conveys that low or medium market size companies can do better and receive better customer appreciation if they perform better during a crisis or on special customer demands.
We further observed that the medium market size company received the highest negative sentiment (0.0732), followed by large (0.071) and small market share (0.068). The negative sentiment of medium and large companies is significantly (p < 0.05) higher than those with small market sizes. It conveys that smaller companies may satisfy customers more in odd times than bigger companies. It may be because smaller companies might be delivering more customer-friendly services as they may be determined to increase their market share by satisfying customers. Challenging times like the COVID-19 pandemic can be used as an opportunity to create more customer-based value. Our findings are different from the literature ( De Mendonca and Zhou, 2019 ) that says larger companies can generate higher profit and customer satisfaction. Our results are more contextual and complex than the literature that says companies with higher market value may positively influence customers' perception ( Hu et al., 2009 ) and repurchase intention ( Bao and Zhu, 2022 ).
Analyzing results at the country level, we noticed that the mean net customer sentiment scores in India and the US are 0.0318 and 0.0205, respectively. Table 7 further confirms that India's net sentiment score is significantly higher (p < 0.05) than the US. As the US is a developed country with excellent infrastructure and customer-based operations are more prevalent—one may expect better customer satisfaction and customer experience from OFD companies in the US. However, interestingly, we find customers are more satisfied with OFD companies in India than in the US. These findings are in line with previous studies ( Charm et al., 2020 ; Steyn et al., 2010 ) that found customer sentiments vary greatly across different countries. Consumers in India, China, and Indonesia display more optimism than in the US (and the rest of the world) ( Charm et al., 2020 ). As far as negative sentiment is concerned, we find no significant difference (p > 0.10). OFD companies in India and US attract similar negative sentiments. It may be because the challenges faced by the companies are almost the same, and the reasons for customers to get discontent are identical, especially in terms of sanitization and COVID-19 appropriate behaviors.
Further, we found mean net sentiment score of the publicly listed companies (0.0295) is significantly higher (p < 0.10) than those of not listed companies (0.0264). Listed companies may have better perceptions and brand names that may positively influence customers' net sentiment. It is also possible that listed companies may adopt a more stakeholder-oriented approach ( Hickman, 2019 ), bringing better customer experiences. These findings align with the literature ( De Mendonca and Zhou, 2019 ; Hickman, 2019 ), highlighting that customers may be more loyal to publicly traded companies as they are more likely to be responsible to the environment and society. The negative sentiment of listed OFD companies (0.07325) also differs significantly (p < 0.05) from unlisted companies (0.06964). Noticeably, the negative sentiment of listed companies is higher than that of unlisted companies. It is possibly because customers have more expectations from listed companies ( Hickman, 2019 ) during the COVID-19 pandemic. Service delivery below the expectation may generate more negative sentiment. Our observations in terms of negative sentiment are different from the literature ( Hickman, 2019 ).
6. Conclusions and future scopes
This study brings a deeper understanding of customers' experience of OFD companies operating in the COVID-19 pandemic, a worse health crisis globally. During the pandemic, thousands of people were quarantined, and the remaining people could not go out to have food in restaurants because of strict lockdowns. During this time, OFD companies delivered food to people who had different expectations from the companies than expectations during the normal time. Especially, sanitization, COVID-19 appropriate behaviors, and social responsibility were among the most important expectations of customers. The central theme of this paper is to explore how OFD companies performed during the COVID-19 pandemic and whether they performed up to the expectations. It also analyzed whether OFD companies' performance differs across emerging and developed countries and different COVID-19 waves. We collected consumer data from Twitter, conducted a qualitative and quantitative analysis to understand several intricacies of OFD companies' operations during the COVID-19 pandemic, and compared our findings with existing literature. This study throws several exciting findings.
The most talked-about topics were social responsibility, delivery operations, perceived consumer responsibility, financial impacts of COVID-19, etc. People in India and the US think differently regarding OFD service during such a hard time. People in India are more concerned about the responsibility of OFD and consumers towards society, but in the US, people discuss more about discounts, coupons, free food delivery, etc. Therefore, managers of OFD companies in India and the US should prioritize their services accordingly. Our findings convey to managers of OFD companies that customer expectations during a crisis are not usual expectations (such as prompt service, good taste, etc.); they expect something beyond. Therefore, managers must understand expectations first and deliver experience accordingly. A few previous studies demonstrated more generic expectations. Theoretically, we observe that dimensions of customer expectations during the COVID-19 crisis differ from normal times.
We further offer important theoretical contributions that the net sentiment of people differs across different COVID-19 waves, brands, market size, countries, and publicly listed (or unlisted) companies. OFD companies improved net sentiment in the second wave of COVID-19, perhaps by using their learnings from the first wave. Overall, Grubhub received the highest net sentiment as well as the negative sentiment. Hence, to minimize negative sentiment, managers of Grubhub should align their service operations as per customer expectations. In India, Swiggy did better than Zomato. Managers of OFD companies should see the events like COVID-19 as opportunities where OFD companies with smaller market sizes also can do better than medium and large-market size companies. Customers are more satisfied in India than in the US; however, negative sentiment is almost equal in the two countries. The publicly listed OFD companies generate higher net sentiment as well as negative sentiment than those of unlisted companies. Hence, managers of listed OFD companies should control negative sentiment as per the performance on different topics identified. Interactions of COVID-19 waves, brands, market size, countries, and publicly listed (or unlisted) companies with net and negative sentiment of customers of OFD companies and its intricacies are interesting theoretical implications of this research.
This paper compares and contrasts OFD companies' consumer service and operations across emerging and developed countries and during the first and second waves of the COVID-19. By studying OFD during the COVID-19 pandemic, this study significantly and uniquely contributes to the existing literature ( Huang, 2021 ; Kim et al., 2021 ; Yeo et al., 2021 ) on retailers and consumers. We identified new topics of importance during a hard time of the COVID-19 pandemic, which are entirely different from topics discussed before the COVID-19 outbreak. We further identified different variables which are significantly influencing customers' sentiment.
Like any other study, this paper also has some limitations. We mainly studied OFD companies operating in India and US by collecting customers' data from Twitter. Future studies can extend the scope of this study further. In addition to the US and Indian markets, it would be interesting to study people's sentiments and identify concerning dimensions about OFD companies' performance in the European and South American markets. Further, the inclusion of financial variables, such as revenue and profit for OFD companies' delivery performance, would be another exciting study. Nonetheless, this study documents the service and operations performance of OFD companies during the COVID-19 health crisis on a large scale which can be used for a future contingency plan. Finally, another promising problem for future studies is investigating how people's sentiment affects the OFD companies' performance in the stock market during the COVID-19 period.
- Aaker D.A., Equity M.B. vol. 28. 1991. pp. 35–37. (Capitalizing on the Value of a Brand Name). New York 1. [ Google Scholar ]
- Ahorsu D.K., Lin C.Y., Imani V., Saffari M., Griffiths M.D., Pakpour A.H. The fear of COVID-19 scale: development and initial validation. Int. J. Ment. Health Addiction. 2020:1–9. [ PMC free article ] [ PubMed ] [ Google Scholar ]
- Al Amin M.A., Arefin M.S., Alam M.R., Ahammad T., Hoque M.R. Using mobile food delivery applications during COVID-19 pandemic: an extended model of planned behavior. J. Food Prod. Market. 2021; 27 (2):105–126. [ Google Scholar ]
- Aureliano-Silva L., Spers E.E., Lodhi R.N., Pattanayak M. Who loves to forgive? The mediator mechanism of service recovery between brand love, brand trust and purchase intention in the context of food-delivery apps. Br. Food J. 2022 doi: 10.1108/BFJ-07-2021-0819. [ CrossRef ] [ Google Scholar ]
- Baek T.H., Kim J., Yu J.H. The differential roles of brand credibility and brand prestige in consumer brand choice. Psychol. Market. 2010; 27 (7):662–678. [ Google Scholar ]
- Balakrishnan V. Delhi food delivery boy tests positive for COVID-19: should you be ordering food from outside? This is what doctors feel. Indiana. 2020 https://timesofindia.indiatimes.com/life-style/health-fitness/diet/delhi-food-delivery-boy-tests-positive-for-COVID-19-should-you-be-ordering-food-from-outside-this-is-what-doctors-feel/articleshow/75180601.cms from. [ Google Scholar ]
- Bao Z., Zhu Y. Why customers have the intention to reuse food delivery apps: evidence from China." British Food Journal (2021) Br. Food J. 2022; 124 (1):179–196. [ Google Scholar ]
- Bates S., Reeve B., Trevena H. A narrative review of online food delivery in Australia: challenges and opportunities for public health nutrition policy. Publ. Health Nutr. 2020 doi: 10.1017/S1368980020000701. [ PMC free article ] [ PubMed ] [ CrossRef ] [ Google Scholar ]
- Berry L.L. Cultivating service brand equity. J. Acad. Market. Sci. 2000; 28 (1):128–137. [ Google Scholar ]
- Birtus M., Lăzăroiu G. The neurobehavioral economics of the COVID-19 pandemic: consumer cognition, perception, sentiment, choice, and decision-making. Anal. Metaphys. 2021; 20 :89–101. [ Google Scholar ]
- Blei D.M., Ng A.Y., Jordan M.I. Latent dirichlet allocation. J. Mach. Learn. Res. 2003; 3 :993–1022. [ Google Scholar ]
- Bolton R.N., Lemon K.N., Verhoef P.C. The theoretical underpinnings of customer asset management: a framework and propositions for future research. J. Acad. Market. Sci. 2004; 32 (3):271–292. [ Google Scholar ]
- Boston Consulting Group India's online food delivery industry to touch $8-bn mark by 2022: Report. Business Standard. 2020 https://www.business-standard.com/article/current-affairs/india-s-online-food-delivery-industry-to-touch-8-bn-mark-by-2022-report-120012800822_1.html Accessed from. [ Google Scholar ]
- Brewer P., Sebby A.G. The effect of online restaurant menus on consumers' purchase intentions during the COVID-19 pandemic. Int. J. Hospit. Manag. 2021; 94 [ PMC free article ] [ PubMed ] [ Google Scholar ]
- Chai L.T., Yat D.N.C. Online food delivery services: making food delivery the new normal. Journal of Marketing advances and Practices. 2019; 1 (1):62–77. [ Google Scholar ]
- Chakraborty D., Kayal G., Mehta P., Nunkoo R., Rana N.P. Consumers' usage of food delivery app: a theory of consumption values. J. Hospit. Market. Manag. 2022:1–19. [ Google Scholar ]
- Charm T., Grimmelt A., Kim H., Robinson K., Lu N., Ortega M. Consumer sentiment is diverging across countries. McKinsey. 2020 https://www.mckinsey.com/business-functions/growth-marketing-and-sales/our-insights/a-global-view-of-how-consumer-behavior-is-changing-amid-covid-19 available at. [ Google Scholar ]
- Chen McCain S.L., Lolli J., Liu E., Lin L.C. An analysis of a third-party food delivery app during the COVID-19 pandemic. Br. Food J. 2021 doi: 10.1108/BFJ-03-2021-0332. [ CrossRef ] [ Google Scholar ]
- Cheng C.C., Chang Y.Y., Chen C.T. Construction of a service quality scale for the online food delivery industry. Int. J. Hospit. Manag. 2021; 95 [ Google Scholar ]
- Correa J.C., Garzon W., Brooker P., Sakarkar G., Carranzaa S.A., Yunado L., Rincon A. Evaluation of collaborative consumption of food delivery services through web mining techniques. J. Retailing Consum. Serv. 2019; 46 :45–50. [ Google Scholar ]
- Dai R., Feng H., Hu J., Jin Q., Li H., Wang R., Wang R., Xu L., Zhang X. The impact of COVID-19 on small and medium-sized enterprises (SMEs): evidence from two-wave phone surveys in China. China Econ. Rev. 2021; 67 [ PMC free article ] [ PubMed ] [ Google Scholar ]
- Daniel K.D., Hirshleifer D.A., Subrahmanyam A. 2005. Investor psychology and tests of factor pricing models. Available at SSRN 854024. [ Google Scholar ]
- De Mendonca T.R., Zhou Y. Environmental performance, customer satisfaction, and profitability: a study among large U.S. Companies, Sustain. Multidiscip. Digit. Publish. Inst. 2019; 11 (19):5418. [ Google Scholar ]
- Dirsehan T., Cankat E. Role of mobile food-ordering applications in developing restaurants' brand satisfaction and loyalty in the pandemic period. J. Retailing Consum. Serv. 2021; 62 [ Google Scholar ]
- Dsouza D., Sharma D. Online food delivery portals during COVID-19 times: an analysis of changing consumer behavior and expectations. Int. J. Innovat. Sci. 2021; 13 (2):218–232. [ Google Scholar ]
- Erdem T., Swait J. Brand credibility, brand consideration, and choice. J. Consum. Res. 2004; 31 (1):191–198. [ Google Scholar ]
- Erkmen E., Hancer M. Building brand relationship for restaurants: an examination of other customers, brand image, trust, and restaurant attributes. Int. J. Contemp. Hospit. Manag. 2019; 31 (3):1469–1487. [ Google Scholar ]
- Faullant R., Matzler K., Füller J. The impact of satisfaction and image on loyalty: the case of Alpine ski resorts. Manag. Serv. Qual. 2008; 18 (2):163–178. [ Google Scholar ]
- Fornell C. A national customer satisfaction barometer: the Swedish experience, J. Market. 1992; 56 :6–22. [ Google Scholar ]
- Gani M.O., Faroque A.R., Muzareba A.M., Amin S., Rahman M. An integrated model to decipher online food delivery app adoption behavior in the COVID-19 pandemic. J. Foodserv. Bus. Res. 2021:1–41. [ Google Scholar ]
- Gavilan D., Balderas-Cejudo A., Fernández-Lores S., Martinez-Navarro G. Innovation in online food delivery: learnings from COVID-19. Int. J. Gastronomy Food Sci. 2021 [ PMC free article ] [ PubMed ] [ Google Scholar ]
- Gilitwala B., Nag A.K. A study of factors influencing repurchase intention of online food delivery in Bangkok, Thailand. Int. J. Emerg. Technol. 2019; 10 (3):193–201. [ Google Scholar ]
- Gunden N., Morosan C., DeFranco A. Consumers' intentions to use online food delivery systems in the USA. Int. J. Contemp. Hospit. Manag. 2020; 32 (3):1325–1345. [ Google Scholar ]
- Han H., Kim W., Hyun S.S. Switching intention model development: role of service performances, customer satisfaction, and switching barriers in the hotel industry. Int. J. Hospit. Manag. 2011; 30 (3):619–629. [ Google Scholar ]
- Hickman L.E. Information asymmetry in CSR reporting: publicly-traded versus privately-held firms, Sustainability Accounting. Manag. Policy J. 2019; 11 (1):207–232. [ Google Scholar ]
- Hong C., Choi H., Choi E.K., Joung H.W. Factors affecting customer intention to use online food delivery services before and during the COVID-19 pandemic. J. Hospit. Tourism Manag. 2021; 48 :509–518. [ Google Scholar ]
- Hong C., Choi H.H., Choi E.-K.C., Joung H.-W.D. Factors affecting customer intention to use online food delivery services before and during the COVID-19 pandemic. J. Hospit. Tourism Manag. 2021; 48 :509–518. [ Google Scholar ]
- Hopkins E., Potcovaru A.M. Consumer attitudes, values, needs. Anal. Metaphys. 2021; 20 :202–215. [ Google Scholar ]
- Hu H.H., Kandampully J., Juwaheer T.D. Relationships and impacts of service quality, perceived value, customer satisfaction, and image: an empirical study. Serv. Ind. J. 2009; 29 (2):111–125. [ Google Scholar ]
- Huang H. Riders on the storm: amplified platform precarity and the impact of COVID-19 on online food-delivery drivers in China. J. Contemp. China. 2021:1–15. [ Google Scholar ]
- Hwang J., Choe J.Y. Exploring perceived risk in building successful drone food delivery services. Int. J. Contemp. Hospit. Manag. 2019; 31 (8):3249–3269. [ Google Scholar ]
- Hwang J., Kim H. The effects of expected benefits on image, desire, and behavioral intentions in the field of drone food delivery services after the outbreak of COVID-19. Sustainability. 2020; 13 (1):117. [ Google Scholar ]
- Ibrahim N.F., Wang X. A text analytics approach for online retailing service improvement: evidence from Twitter, Decis. Support Syst. 2019; 121 :37–50. [ Google Scholar ]
- Ibrahim N.F., Wang X., Bourne H. Exploring the effect of user engagement in online brand communities: evidence from Twitter. Comput. Hum. Behav. 2017; 72 :321–338. [ Google Scholar ]
- Jain D. Effect of COVID-19 on restaurant industry–how to cope with changing demand. Eff. COVID-19 Restaur. Ind. How Cope Chang. Demand. 2020 doi: 10.2139/ssrn.3577764. April 16, 2020. [ CrossRef ] [ Google Scholar ]
- Jia S.S., Raeside R., Redfern J., Gibson A.A., Singleton A., Partridge S.R. #SupportLocal: how online food delivery services leveraged the COVID-19 pandemic to promote food and beverages on Instagram. Publ. Health Nutr. 2021; 24 (15):4812–4822. [ PMC free article ] [ PubMed ] [ Google Scholar ]
- Jin N., Lee S., Huffman L. Impact of restaurant experience on brand image and customer loyalty: moderating role of dining motivation. J. Trav. Tourism Market. 2012; 29 :532–551. [ Google Scholar ]
- Keeble M., Adams J., Sacks G., Vanderlee L., White C.M., Hammond D. Use of online food delivery services to order food prepared away-from-home and associated sociodemographic characteristics: a cross-sectional, multi-country analysis. Int. J. Environ. Res. Publ. Health. 2020; 17 (14):5190. [ PMC free article ] [ PubMed ] [ Google Scholar ]
- Kim J., Kim J., Wang Y. Uncertainty risks and strategic reaction of restaurant firms amid COVID-19: evidence from China. Int. J. Hospit. Manag. 2021; 92 [ PMC free article ] [ PubMed ] [ Google Scholar ]
- Kohút M., Kohútová V., Halama P. Big Five predictors of pandemic-related behavior and emotions in the first and second COVID-19 pandemic wave in Slovakia. Pers. Indiv. Differ. 2021; 180 [ PMC free article ] [ PubMed ] [ Google Scholar ]
- Koppehele-Gossel J., Weinmann L.M., Klimke A., Windmann S., Voss U. Adapting to a major crisis: sleep and mental health during two lockdowns. J. Sleep Res. 2022:e13565. [ PMC free article ] [ PubMed ] [ Google Scholar ]
- Kougiannou N.K., Mendonça P. Breaking the managerial silencing of worker voice in platform capitalism: the rise of a food courier network. Br. J. Manag. 2021; 32 (3):744–759. [ Google Scholar ]
- Kumar S., Shah A. Revisiting food delivery apps during COVID-19 pandemic? Investigating the role of emotions. J. Retailing Consum. Serv. 2021; 62 [ Google Scholar ]
- Kumar S., Jain A., Hsieh J.K. Impact of apps aesthetics on revisit intentions of food delivery apps: the mediating role of pleasure and arousal. J. Retailing Consum. Serv. 2021; 63 [ Google Scholar ]
- Lalvani S., Seetharaman B. The personal and social risks that India's food delivery workers are taking during COVID-19. Wire. 2020 https://thewire.in/business/COVID-19-food-delivery-workers from. [ Google Scholar ]
- Leng X., Ochi M., Sakaki T., Mori J., Sakata I. A cross-lingual analysis on culinary perceptions to understand the cross-cultural difference. AAAI Spring Symp. Interpretable AI Well-being. 2019 [ Google Scholar ]
- Li C., Mirosa M., Bremer P. Review of online food delivery platforms and their impacts on sustainability. Sustainability. 2020; 12 (14):5528. [ Google Scholar ]
- Liu S., Jiang H., Chen S., Ye J., He R., Sun Z. Integrating Dijkstra's algorithm into deep inverse reinforcement learning for food delivery route planning. Transport. Res. Part E. 2020; 142 [ Google Scholar ]
- Lo Coco G., Gentile A., Bosnar K., Milovanović I., Bianco A., Drid P., Pišot S. A cross-country examination on the fear of COVID-19 and the sense of loneliness during the first wave of COVID-19 outbreak. International. J. Environ. Res. Publ. Health. 2021; 18 (5):2586. [ PMC free article ] [ PubMed ] [ Google Scholar ]
- Luo X., Homburg C., Wieseke J. Customer satisfaction, analyst stock recommendations, and firm value. J. Market. Res. 2010; 47 (6):1041–1058. [ Google Scholar ]
- Mehrolia S., Alagarsamy S., Solaikutty V.M. Customers response to online food delivery services during COVID-19 outbreak using binary logistic regression. Int. J. Consum. Stud. 2021; 45 :396–408. [ PMC free article ] [ PubMed ] [ Google Scholar ]
- Mehta M.P., Kumar G., Ramkumar M. Customer expectations in the hotel industry during the COVID-19 pandemic: a global perspective using sentiment analysis. Tour. Recreat. Res. 2021:1–18. [ Google Scholar ]
- Michalikova K.F., Blazek R., Rydell L. Delivery apps use during the COVID-19 pandemic: consumer satisfaction judgments, behavioral intentions, and purchase decisions. Econ. Manag. Financ. Mark. 2022; 17 (1):70–82. [ Google Scholar ]
- Mittal V., Anderson E., Sayrak A., Kamakura W. Satisfaction, repurchase intent, and repurchase behavior: investigating the moderating effect of customer characteristics. J. Market. Res. 2001; 38 :131–142. [ Google Scholar ]
- Mohan S., Solanki A.K., Taluja H.K., Singh A. Predicting the impact of the third wave of COVID-19 in India using hybrid statistical machine learning models: a time series forecasting and sentiment analysis approach. Comput. Biol. Med. 2022; 144 [ PMC free article ] [ PubMed ] [ Google Scholar ]
- NASSCOM Catalyzing the ecosystem for a trillion-dollar digital economy. Hyderabad: Natl. Assoc. Software Serv. Co. 2018 https://nasscom.in/sites/default/files/NASSCOM-annual-guidance-fy-2018.pdf from. [ Google Scholar ]
- National Restaurant Association Coronavirus information and resource. 2020. https://restaurant.org/Covid19 Accessed from.
- Ng C.S.P. Intention to purchase on social commerce websites across cultures: a cross-regional study. Inf. Manag. 2013; 50 (8):609–620. [ Google Scholar ]
- Nguyen T.H., Vu D.C. Food delivery service during social distancing: proactively preventing or potentially spreading COVID-19? Disaster Med. Public Health Prep. 2020; 14 (3):e9–e10. [ PMC free article ] [ PubMed ] [ Google Scholar ]
- Nikolenko S.I., Koltcov S., Koltsova O. Topic modelling for qualitative studies. J. Inf. Sci. 2017; 43 (1):88–102. [ Google Scholar ]
- Oliver R.L. Effect of expectation and disconfirmation on postexposure product evaluations: an alternative interpretation. J. Appl. Psychol. 1977; 62 (4):480. [ Google Scholar ]
- O'Sullivan D., McCallig J. Customer satisfaction, earnings and firm value. Eur. J. Market. 2012; 46 (6):827–843. [ Google Scholar ]
- Pal D., Funilkul S., Eamsinvattana W., Siyal S. Using online food delivery applications during the COVID-19 lockdown period: what drives University Students' satisfaction and loyalty. J. Foodserv. Bus. Res. 2021:1–45. [ Google Scholar ]
- Pant B., Shende U. The Impact of COVID-19 on the sharing economy in India. 2020. [ CrossRef ]
- Parwez S., Ranjan R. The platform economy and the precarisation of food delivery work in the COVID-19 pandemic: evidence from India. Work Organisa. Lab. Glob. 2021; 15 (1):11–30. [ Google Scholar ]
- Peng C.L., Lai K.L., Chen M.L., Wei A.P. Investor sentiment, customer satisfaction and stock returns. Eur. J. Market. 2015; 49 (5/6):827–850. [ Google Scholar ]
- Pigatto G., Machado J.G.d.C.F., Negreti A.d.S., Machado L.M. Have you chosen your request? Analysis of online food delivery companies in Brazil. Br. Food J. 2017; 119 (3):639–657. [ Google Scholar ]
- Pitta D.A., Katsanis L.P. Understanding brand equity for successful brand extension. J. Consum. Market. 1995; 12 (4):51–64. [ Google Scholar ]
- Prasetyo Y.T., Tanto H., Mariyanto M., Hanjaya C., Young M.N., Persada S.F., Miraja B.A., Redi A.A.N.P. Factors affecting customer satisfaction and loyalty in online food delivery service during the COVID-19 pandemic: its relation with open innovation. J. Open Innovat.: Technol. Market Complex. 2021; 7 (1):76. [ Google Scholar ]
- Punel A., Hassan L.A.H., Ermagun A. Variations in airline passenger expectation of service quality across the globe. Tourism Manag. 2019; 75 :491–508. [ Google Scholar ]
- Puram P., Gurumurthy A., Narmetta M., Mor R.S. Last-mile challenges in on-demand food delivery during COVID-19: understanding the riders' perspective using a grounded theory approach. Int. J. Logist. Manag. 2021 doi: 10.1108/IJLM-01-2021-0024. [ CrossRef ] [ Google Scholar ]
- Raj M., Sundararajan A., You C. Evidence from Uber Eats NYU Stern School of Business, 2021; 2021. COVID-19 and Digital Resilience. [ CrossRef ] [ Google Scholar ]
- Ramos K. Factors influencing customers' continuance usage intention of food delivery apps during COVID-19 quarantine in Mexico. Br. Food J. 2021; 124 (3):833–852. [ Google Scholar ]
- Research and Markets Global online food delivery services market report 2021: COVID-19 growth. Impacts Change 2030. 2021 https://www.marketresearch.com/Business-Research-Company-v4006/Online-Food-Delivery-Services-Global-14484234/ Accessed from. [ Google Scholar ]
- Richardson L. Platforms, markets, and contingent calculation: the flexible arrangement of the delivered meal. Antipode. 2020; 52 (3):619–636. [ Google Scholar ]
- Rizou M., Galanakis I.M., Aldawoud T.M.S., Galanakis C.M. Safety of foods, food supply chain and environment within the COVID-19 pandemic. Trends Food Sci. Technol. 2020; 102 :293–299. [ PMC free article ] [ PubMed ] [ Google Scholar ]
- Roh M., Park K. Adoption of O2O food delivery services in South Korea: the moderating role of moral obligation in meal preparation. Int. J. Inf. Manag. 2019; 47 :262–273. [ Google Scholar ]
- Rydell L., Kucera J. Cognitive attitudes, behavioral choices, and purchasing habits during the COVID-19 pandemic. J. Self Govern. Manag. Econ. 2021; 9 (4):35–47. [ Google Scholar ]
- Rydell L., Suler P. Underlying values that motivate behavioral intentions and purchase decisions: lessons from the COVID-19 pandemic. Anal. Metaphys. 2021; 20 :116–129. [ Google Scholar ]
- Ryu K., Lee H.R., Kim W.G. The influence of the quality of the physical environment, food, and service on restaurant image, customer perceived value, customer satisfaction, and behavioral intentions. Int. J. Contemp. Hospit. Manag. 2012; 24 (2):200–223. [ Google Scholar ]
- Savitri P.D., Krisnatuti D., Hannan S. The effect of innovation and marketing mix toward brand image and usage decision in online food delivery services industry. Asia Pac. Manag. Bus. Appl. 2020; 9 (2):99–110. [ Google Scholar ]
- Seghezzi A., Mangiaracina R. On-demand food delivery: investigating the economic performances. Int. J. Retail Distrib. Manag. 2020; 49 (4):531–549. [ Google Scholar ]
- Seghezzi A., Winkenbach M., Mangiaracina R. On-demand food delivery: a systematic literature review. Int. J. Logist. Manag. 2021; 32 (4):1334–1355. [ Google Scholar ]
- Shah A.M., Yan X., Qayyum A. Adoption of mobile food ordering apps for O2O food delivery services during the COVID-19 outbreak. Br. Food J. 2021 doi: 10.1108/BFJ-09-2020-0781. [ CrossRef ] [ Google Scholar ]
- Sharma R., Dhir A., Talwar S., Kaur P. Over-ordering and food waste: the use of food delivery apps during a pandemic. Int. J. Hospit. Manag. 2021; 96 [ Google Scholar ]
- Smith A., Machova V. Consumer tastes, sentiments, attitudes, and behaviors related to COVID-19. Anal. Metaphys. 2021; 20 :145–158. [ Google Scholar ]
- Statista Online food delivery: highlights. 2021. https://www.statista.com/outlook/dmo/eservices/online-food-delivery/united-states from.
- Steyn P., Pitt L., Strasheim A., Boshoff C., Abratt R. A cross-cultural study of the perceived benefits of a retailer loyalty scheme in Asia. J. Retailing Consum. Serv. 2010; 17 (5):355–373. [ Google Scholar ]
- Sundararajan A. MIT Press; Cambridge: 2016. The Sharing Economy: the End of Employment and the Rise of Crowd-Based Capitalism. [ Google Scholar ]
- Sv P., Ittamalla R., Balakrishnan J. Analyzing general public's perception on posttraumatic stress disorder and COVID-19: a machine learning study. J. Loss Trauma. 2021:1–3. [ Google Scholar ]
- Tiwari S., Ram S.G., Roy S. 'What is it like to work in a gig economy job'. Indiana. 2019 https://timesofindia.indiatimes.com/india/what-it-is-like-to-work-in-agig-economy-job/articleshow/69371217.cms Available from. [ Google Scholar ]
- Tran V.D. Using mobile food delivery applications during the COVID-19 pandemic: applying the theory of planned behavior to examine continuance behavior. Sustainability. 2021; 13 :1206. [ Google Scholar ]
- Trivedi S.K., Singh A. Twitter sentiment analysis of app based online food delivery companies, Global Knowledge. Memory Commun. 2021; 70 (8/9):891–910. [ Google Scholar ]
- Uzir M.U.H., Al Halbusi H., Thurasamy R., Hock R.L.T., Aljaberi M.A., Hasan N., Hamid M. The effects of service quality, perceived value and trust in home delivery service personnel on customer satisfaction: evidence from a developing country. J. Retailing Consum. Serv. 2021; 63 [ Google Scholar ]
- van Doorn N. At what price? Labour politics and calculative power struggles in on-demand food delivery, Work Organisation. Lab. Glob. 2020; 14 (1):136–149. [ Google Scholar ]
- Watson R., Popescu G.H. Will the COVID-19 pandemic lead to long-term consumer perceptions, behavioral intentions, and acquisition decisions? Econ. Manag. Financ. Mark. 2021; 16 (4):70–83. [ Google Scholar ]
- Yang Y., Liu H., Chen X. COVID-19 and restaurant demand: early effects of the pandemic and stay-at-home orders. Int. J. Contemp. Hospit. Manag. 2020; 32 (12):3809–3834. [ Google Scholar ]
- Yao Q., Zeng S., Sheng S., Gong S. Green innovation and brand equity: moderating effects of industrial institutions. Asia Pac. J. Manag. 2021; 38 (2):573–602. [ Google Scholar ]
- Yeo S.F., Tan C.L., Teo S.L., Tan K.H. The role of food apps servitisation on repurchase intention: a study of FoodPanda. Int. J. Prod. Econ. 2021; 234 [ Google Scholar ]
- Zanetta L.D., Hakim M.P., Gastaldi G.B., Seabra L.M.J., Rolim P.M., Nascimento L.G.P., Medeiros C.O., da Cunha D.T. The use of food delivery apps during the COVID-19 pandemic in Brazil: the role of solidarity, perceived risk, and regional aspects. Food Res. Int. 2021; 149 [ PMC free article ] [ PubMed ] [ Google Scholar ]
- Zhao Y., Bacao F. What factors determining customer continuingly using food delivery apps during 2019 novel coronavirus pandemic period? Int. J. Hospit. Manag. 2020; 91 [ PMC free article ] [ PubMed ] [ Google Scholar ]
- Zou C., Wang X., Xie Z., Li D. 2020. Public reactions towards the covid-19 pandemic on Twitter in the United Kingdom and the United States. [ CrossRef ] [ Google Scholar ]
Academia.edu no longer supports Internet Explorer.
To browse Academia.edu and the wider internet faster and more securely, please take a few seconds to upgrade your browser .
Enter the email address you signed up with and we'll email you a reset link.
- We're Hiring!
- Help Center
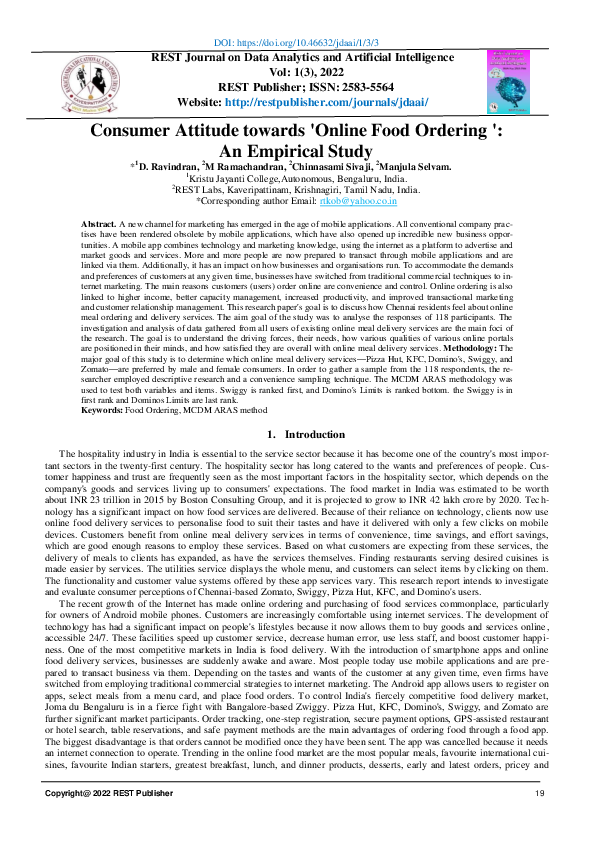
Consumer Attitude towards 'Online Food Ordering ': An Empirical Study

2022, REST Journal on Data Analytics and Artificial Intelligence
A new channel for marketing has emerged in the age of mobile applications. All conventional company practises have been rendered obsolete by mobile applications, which have also opened up incredible new business opportunities. A mobile app combines technology and marketing knowledge, using the internet as a platform to advertise and market goods and services. More and more people are now prepared to transact through mobile applications and are linked via them. Additionally, it has an impact on how businesses and organisations run. To accommodate the demands and preferences of customers at any given time, businesses have switched from traditional commercial techniques to internet marketing. The main reasons customers (users) order online are convenience and control. Online ordering is also linked to higher income, better capacity management, increased productivity, and improved transactional marketing and customer relationship management. This research paper's goal is to discuss how Chennai residents feel about online meal ordering and delivery services. The aim goal of the study was to analyse the responses of 118 participants. The investigation and analysis of data gathered from all users of existing online meal delivery services are the main foci of the research. The goal is to understand the driving forces, their needs, how various qualities of various online portals are positioned in their minds, and how satisfied they are overall with online meal delivery services. Methodology: The major goal of this study is to determine which online meal delivery services-Pizza Hut, KFC, Domino's, Swiggy, and Zomato-are preferred by male and female consumers. In order to gather a sample from the 118 respondents, the researcher employed descriptive research and a convenience sampling technique. The MCDM ARAS methodology was used to test both variables and items. Swiggy is ranked first, and Domino's Limits is ranked bottom. the Swiggy is in first rank and Dominos Limits are last rank.
Related Papers
International Journal of Management, Technology, and Social Sciences
Sureshramana Mayya
Purpose: In a country like India, the advent of technology has magnified e- businesses. A person or a consumer who is hungry or craving food or lazy to cook or may not have time to go out and eat may now have food ordered online by getting a quick door delivery. Consumers continue to eat out, but they find ordering food online immensely convenient because it banishes the need to visit restaurants physically. The fundamental goal of the current study was to assess the consumer preferences and perceptions of online food ordering amenities. Objectives: To probe customers' insights on online food delivery amenities and to recognize the several components that influence the end-user decisions. Another objective is to avail oneself of online food delivery amenities. Consumer’s preferred online food delivery amenities portal was investigated. This study also intended to determine the elements that impact the consumer's decision to order food online. Due to the analysis of this stud...
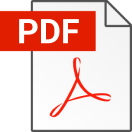
Advances in economics, business and management research
mohit totuka
Prabandhan: Indian Journal of Management
ramesh bagla
SK International Journal of Multidisciplinary Research Hub
SK Publisher
The era of mobile applications has opened up a new path for modern marketing. The mobile application has opened up a world of new opportunities in business and rendered all of the old methods of doing business obsolete. The Internet is used as a medium for advertising and selling services and goods in mobile applications, which combine marketing expertise with technology. Today, more and more people are connecting with one another through mobile applications, and they are prepared to trade using them. Additionally, it has an impact on how businesses and organizations operate. Online marketing has replaced traditional business strategies for meeting the needs and preferences of customers at any time. The purpose of this research paper is to discuss how consumers in Gurugram perceive online food ordering and delivery services. The objective is to ascertain their perceptions, requirements, positioning of various online portal attributes in their minds, and overall satisfaction with online food delivery services. In order to accomplish the goal, data have been gathered from various Gurugram area restaurants have been visited to learn about their perspectives. Using a positioning study, four parameters are taken into consideration for the analysis.
Journal of Information System and Technology Management
Norshita Nayan
International Journal of Travel, Hospitality and Events
Ramon Hurdawaty
This research aims to determine consumer preference factors when purchasing Jakarta's online food delivery services. Research methods: This study uses a comparative quantitative method. Data was obtained by distributing online questionnaires via Google form to 100 users of Online Food Delivery Services (Grab-Food et al.) in Jakarta. In this study, the sample was determined by purposive sampling. Researchers conducted a oneway ANOVA test to see if there were differences in respondents' preferences. The preference factors used are transaction, price, and promotion. Results and discussion: Research has been conducted to understand consumer preferences in popular food delivery services such as Go-Food, GrabFood, and ShopeeFood. Understanding these preferences is critical for companies to tailor their services and offerings to meet their customers' needs and expectations better. The research results show differences in consumer preferences when choosing online food delivery services. Implication: By leveraging this research, all stakeholders can contribute to a more competitive, user-friendly, and innovative food delivery landscape. Go-Food, Grab-Food, and Shopee-Food can continue to innovate, develop transaction systems so that users are more comfortable making transactions when using the Food Delivery Service application, provide prices according to the quality provided, and take a more innovative and creative promotional approach so that many users can know the promotion.
IAEME PUBLICATION
IAEME Publication
Owing to the hard-pressed way of life these days, online food applications have emerged as a trend. Everyone prefers to order food online, rather than cooking at home or eating home-cooked meals, in the search of flavor and variety. Advancing technology has been playing a significant role in the establishment and progression of online cellular food ordering applications of which HungerStation, Walem, Talabat, Daeemdelivery, Shadda, Careem Now, and Jahez are the most recurrent and frequently used applications by the customers in Saudi Arabia. In this paper, various factors are explored which influence the customers in the use of online food ordering applications by using Exploratory Factor Analysis (EFA). Four factors, namely Right Contents & Packaging, Offers & Discounts, Timely Delivery, and User-Friendly, are identified and then, based on these factors' preferences of customers towards the top four online food ordering apps of Saudi Arabia are evaluated by Evaluation Based on Distance from Average Solution (EDAS) technique.
Shodh Sarita
Prof. Gaurav Gawade
Online food ordering and delivery may be a new sort of business model within the current era of e-commerce which results in the startups of several online business. Online food ordering and delivery is vastly successful because it bridges the gap between restaurants and consumers.. An application for food ordering and delivery was fundamentally evolved to supply an entire order and delivery solution to the metropolitan foodies. The aim of this research is to review the factors influencing the buyer perception and buying decision and to review the extent of consumer satisfaction towards online food delivery service. Since the rising of the Web, vendors have endeavoured to bring to the table their things to individuals who program the Internet. It has been noticed that there is a change from one individual to another with respect to consumer's perception on online food ordering and also the reformed perception is seen restricted to a limited degree along with the accessibility of the acceptable network and the openness to online food services.
AMIR MANSHOOR
Online food ordering and delivery services are the platforms that provide a system and service to the consumer to order and buy food products from foodservice or restaurant operators. The service is an up-and-coming trend among Millennials and the trend has ballooned with the introduction of movement control order (MCO) due to the pandemic COVID-19. The consumer’s demand towards online food ordering and delivery service has increased markedly due to the prohibition of dine-in at restaurant premises. This study focuses on highlighting the factors that influence consumer’s intention to use online food ordering and delivery services. The survey questionnaires were distributed among 384 respondents that represent the customers of restaurants in Shah Alam, Selangor. Data analyses were conducted using SPSS and multiple regression analysis. Findings revealed that usefulness, ease of use, and consumer’s enjoyment are the factors that influence consumer’s intention to use online food orderin...
Loading Preview
Sorry, preview is currently unavailable. You can download the paper by clicking the button above.
RELATED PAPERS
Proceedings of the 5th Sriwijaya Economics, Accounting, and Business Conference (SEABC 2019)
Aslamia Rosa
Ijaems Journal
Asian Journal of Management
akanksha john
International Journal of Case Studies in Business, IT, and Education
Kavitha Pakkala
Srinivas Publication
Our Heritage Journal
Dr. Mukul Burghate
International Journal of Agricultural and Environmental Information Systems
Eugenia Papaioannou
Journal of Entrepreneurship and Business
Umairah Rosli
Journal of Pharmaceutical Negative Results
Smita Kavatekar
Global Journal for Management and Administrative Sciences
Affifa Sardar
Edutourism Journal Of Tourism Research
Said Keliwar
International Journal of Scientific and Research Publications (IJSRP)
Vreha Surya
Journal of Industrial and Information Technology in Agriculture
Arita Dewi Nugrahini
Advances in Mathematics: Scientific Journal
Dr. arpit sidhu
International Journal of Recent Technology and Engineering (IJRTE)
Merry Borgohain
D Gayathiry
raghavendra hugr
lamesgen wubishet
Malaysian Journal of Social Sciences and Humanities (MJSSH)
wan rusni wan ismail
Journal of Marketing Management and Consumer Behavior
DR. ROZITA NAINA MOHAMED , Journal of Marketing Management and Consumer Behavior
TVISHA BHANOT
RELATED TOPICS
- We're Hiring!
- Help Center
- Find new research papers in:
- Health Sciences
- Earth Sciences
- Cognitive Science
- Mathematics
- Computer Science
- Academia ©2024
- [email protected]
- ISSN[Online] : 2643-9875 || ISSN[Print] : 2643-9840
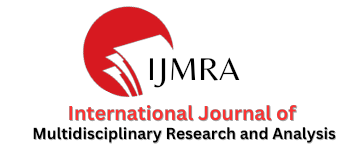
- call for paper
- Board Members
- AUTHOR'S GUIDLINES
- MODE OF PAYMENT
- Manuscript Templete
- Copyright form
- PUBLICATION ETHICS
- REVIEWER POLICY
- REVIEWER GUIDELINES
- PLAGIARISM POLICIES
- WITHDRAWAL POLICIES
VOLUME 04 ISSUE 03 MARCH 2021
Online food delivery: study with special reference to food aggregators, 1 dr. manish kumar srivastava, 2 mr. atul kumar srivastava, 1 associate professor, department of commerce, deen dayal upadhyaya gorakhpur university, gorakhpur., 2 research scholar, department of business administration, deen dayal upadhyaya gorakhpur university, gorakhpur..
Technology has emerged as one of the factors which are affecting every business throughout the world. It has changed business practices throughout the world. The service industry is hugely affected by the use of technology. The Food and Restaurant Industry is also started making efficient use of technology. In recent times, consumers have shown an inclination towards ordering foods from hotels and restaurants and having them at the convenience of their homes. Due to this online food delivery has emerged as a fast-growing services sector. In this paper, an attempt has been made by the authors to identify the preferred food aggregators operating in the Indian online food delivery market and to find the major reasons behind ordering food through these food aggregators
online food delivery, food aggregators, online marketing
1) Rathore S. Suryadev, Chaudhary Mahik (2018). “Consumer's Perception on Online Food Ordering”. International journal of Management and Business Studies, Volume 8, Issue 4, Oct-Dec 2018, ISSN 2230-9519 (Online) and ISSN: 2231-2463 (Print) 2) Panse Chetan, Rastogi Shailesh (2019), “Understanding Consumer Behaviour Towards Utilization of Online Food Delivery Platforms”Journal of theoretical and applied information technology., Volume 97, No.16, ISSN: 1992-8645, E-ISSN: 1817- 3195 3) Sethu H.S., Saini Bhavya (2016). “Customer Perception and Satisfaction on Ordering Food via Internet”.Proceedings of the Seventh Asia-Pacific Conference on Global Business, Economics, Finance and Social Sciences (AP16Malaysia Conference) ISBN: 978-1-943579-81-5 Kuala Lumpur, Malaysia. 15-17, July 2016. Paper ID: KL631 4) Das Jyotishman (2018). “Consumer Perception Towards ‘Online Food Ordering and Delivery Services’: An Empirical Study”. Journal of Management (JOM), Volume 5, Issue 5, September-October 2018, ISSN Print: 2347-3940 and ISSN Online: 2347-3959, pp. 155–163 5) Hong, L., Li, Y. N., & Wang, S. H. (2016). Improvement of Online Food Delivery Service Based on Consumers’ Negative Comments. Canadian Social Science, 12 (5), 84-88. Available from: http://www.cscanada.net/index.php/css/article/view/8464 DOI: http://dx.doi.org/10.3968/8464
Our Services and Policies
- Submission Policy
- Frequency of Publication
- Open Access
- Call For Paper
Authors should prepare their manuscripts according to the instructions given in the authors' guidelines. Manuscripts which do not conform to the format and style of the Journal may be returned to the authors for revision or rejected.
The Journal reserves the right to make any further formal changes and language corrections necessary in a manuscript accepted for publication so that it conforms to the formatting requirements of the Journal.
International Journal of Multidisciplinary Research and Analysis will publish 12 monthly online issues per year,IJMRA publishes articles as soon as the final copy-edited version is approved. IJMRA publishes articles and review papers of all subjects area.
Open access is a mechanism by which research outputs are distributed online, Hybrid open access journals, contain a mixture of open access articles and closed access articles.
International Journal of Multidisciplinary Research and Analysis initiate a call for research paper for Volume 07 Issue 07 (July 2024) .
PUBLICATION DATES: 1) Last Date of Submission : 26 July 2024 . 2) Article published within a week. 3) Submit Article : [email protected] or Online
Why with us
The editors reserve the right to reject papers without sending them out for review..
Authors should prepare their manuscripts according to the instructions given in the authors' guidelines. Manuscripts which do not conform to the format and style of the Journal may be returned to the authors for revision or rejected. The Journal reserves the right to make any further formal changes and language corrections necessary in a manuscript accepted for publication so that it conforms to the formatting requirements of the Journal.
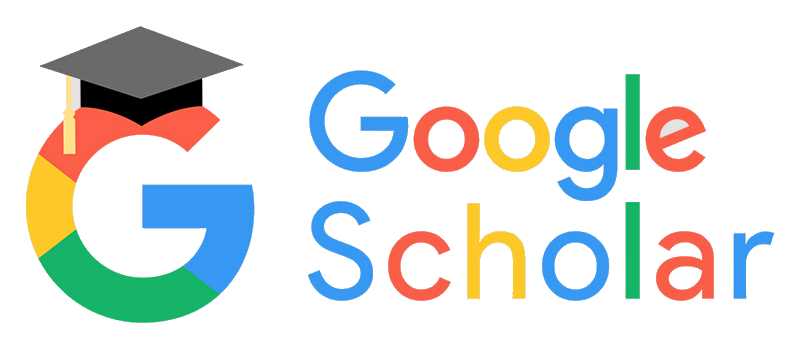

The Economic Times daily newspaper is available online now.
Food services market in india to reach rs 10 lakh crore by 2030: report.
The food services market in India is set to reach Rs 10 lakh crore by 2030, with an estimated customer base of up to 45 crore, driven by an 18 per cent CAGR in online food delivery. Currently valued at Rs 5.5 lakh crore, the market is expected to grow at 10-12 per cent annually, with online delivery contributing 20 per cent by 2030, fueled by expanding customer base, digitisation, and regional diversity.
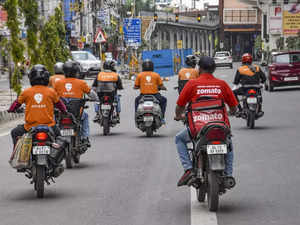
Food delivery market size to cross Rs 2 lakh crore by 2030: Bain-Swiggy report
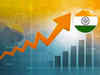
Indian economy poised for potentially stable high growth phase, says RBI's monetary policy panel member
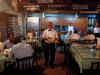
Zomato launches platform to offer hiring, regulatory services to restaurants
Read More News on
(Catch all the Business News , Breaking News , Budget 2024 Events and Latest News Updates on The Economic Times .)
Subscribe to The Economic Times Prime and read the ET ePaper online.
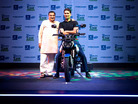
Bajaj launches word’s first CNG bike. Here’s what can make or break it.
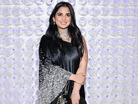
How Isha Ambani’s Shein ambition is set to disrupt fast fashion in India
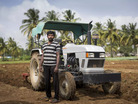
MFs pour into Mahindra Finance after this move by Modi
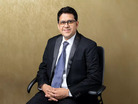
Why the IPO success party of India’s white oil major didn’t last too long
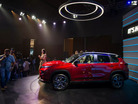
Seven checks to help you buy the car that suits your fuel needs
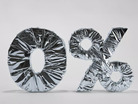
Sebi and the burning question of cost of trading for retail investors

Find this comment offensive?
Choose your reason below and click on the Report button. This will alert our moderators to take action
Reason for reporting:
Your Reason has been Reported to the admin.
To post this comment you must
Log In/Connect with:
Fill in your details:
Will be displayed
Will not be displayed
Share this Comment:
Stories you might be interested in
Development co-operation
The OECD designs international standards and guidelines for development co-operation, based on best practices, and monitors their implementation by its members. It works closely with member and partner countries, and other stakeholders (such as the United Nations and other multilateral entities) to help them implement their development commitments. It also invites developing country governments to take an active part in policy dialogue.
- Development Co-operation Report
- Official development assistance (ODA)

Select a language
Key messages, charting development co-operation trends and challenges.
The OECD keeps track of key trends and challenges for development co-operation providers and offers practical guidance. It draws from the knowledge and experience of Development Assistance Committee (DAC) members and partners, as well as from independent expertise, with the ultimate goal of advancing reforms in the sector, and achieving impact. Using data, evidence, and peer learning, this work is captured in publications and online tools that are made publicly available.
Making development co-operation more effective and impactful
The OECD works with governments, civil society organisations, multilateral organisations, and others to improve the quality of development co-operation. Through peer reviews and evaluations, it periodically assesses aid programmes and co-operation policies, and offers recommendations to improve their efficiency. The OECD also brings together multiple stakeholders to share good and innovative practices and discuss progress.
Strengthening development co-operation evaluation practices and systems
The OECD helps development co-operation providers evaluate their actions both to better learn from experience and to improve transparency and accountability. Innovative approaches, such as using smart and big data, digital technology and remote sensing, help gather evidence and inform policy decisions. With in-depth analysis and guidance, the Organisation helps providers manage for results by building multi-stakeholder partnerships and adapting to changing contexts and crisis situations.
Civil society engagement in development co-operation
National and international civil society organisations (CSOs) are key partners in monitoring development co-operation policies and programmes. Development co-operation can also be channelled to or through CSOs:
Aid is characterized as going to CSOs when it is in the form of core contributions and contributions to programmes, with the funds programmed by the CSOs.
Aid is characterized as going through CSOs when funds are channeled through these organisations to implement donor-initiated projects. This is also known as earmarked funding.
Development co-operation TIPs - Tools, Insights, Practices
TIPs is a searchable peer learning platform that offers insights into making policies, systems and partnerships more effective.

Related data
Related publications.

Related policy issues
- Development co-operation evaluation and effectiveness
- Development co-operation in practice
- Development co-operation peer reviews and learning
- Innovation in development co-operation

IMAGES
VIDEO
COMMENTS
K. Senthamarai Kannan ([email protected]) is currently working as a senior professor and head, Department of Statistics, Manonmaniam Sundaranar University, Abishekapatti, Tirunelveli, Tamil Nadu, India.He has more than 25 years of teaching and research experience. He has published more than 100 research papers in international and national reputed journals.
turing industry, now it refers to its application in food ordering and delivery. market. The pace (double-digit CAGR) with which India's online food delivery. market is growing, food supply ...
Currently Indian Online food market is$350billion.Food technology in broad area, online food. delivery apps are just part of it. This conceptual study will give more insight about emerging ...
Email; [email protected]. Abstract. E-commerce industry in India is witnessing a high record in online food & restaurant service. companies, which is going to reach $350 billion by 2020 year ...
The statistical reports by RedSeer research and consulting group, the food-tech market of India indicates that the increasing order frequency by the consumers is the critical leveraging factor in the food-tech sector's growth in the past few years . The current top market players, Swiggy, and Zomato are recording approximately 30-35 million ...
As the usage of food delivery Apps grows in the metropolitan cities in India, a growing trend is also being seen in smaller cities. It has therefore become a necessity for online food delivery service providers to identify the preferences and perceptions of consumers to enable them to meet their expectations.
The article titled "Online Food Ordering Trend is Hot and on a Roll" discusses the rapid growth of online food ordering in India. It highlights the emergence of several food tech companies on the internet, driven by an expanding customer base and increasing demand for food. The Indian market is often regarded as a
The number of increased downloads of food ordering app is an indication of both popularity of these apps and helplessness of the present age customer. ... BIT, Mesra, Ranchi, assisted Govt Sponsored Project in Department of Scientific and Industrial Research, Ministry of HRD, Govt. of India. He was awarded PhD in management from BIT, Mesra. He ...
The origins of the online food industry go back to 1994 where the first online food order received was a pizza from Pizza Hut in 1994. And the first online food ordering service, was Worldwide Waiter (now known as Waiter.com), was founded in 1995. With a population of over 1.2 billion, India is undeniably one of the biggest
This research paper is aimed to investigate consumers‟ views about the services they receive from different portals. This paper will ... Analysis of Online Food Ordering Applications in India . IJSRD - International Journal for Scientific Research & Development| Vol. 7, Issue 07, 2019 | ISSN (online): 2321-0613 ...
Locale of the study: This initiative is confined to an online industry for food ordering in India. India has over 1.3 billion people and 80 percent and 37 percent smartphone coverage and internet penetration. This means that the online food ordering industry will benefit a tremendous sum, difference
According to the "Online Food Delivery (OFD) Services Global Market Report 2020-2030," the OFD market is projected to grow from $107.44 billion in 2019 to $154.34 billion in 2023 (Businesswire ...
However, more and more people started ordering foods online via food delivery platforms like DoorDash, Grubhub, Uber Eats (in the US), Zomato, and Swiggy (in India) (Jain, 2020; Kim et al., 2021). Restaurants that provided OFD and curbside pickup services for contactless delivery experienced a lesser effect on financial performance.
In this connection, the perception of the customers about Online Food Ordering and about the services of the Online Food Ordering companies gains significance. This has inspired the researcher to undertake the present study to investigate the Customers' views about the services they receive from the different Online Food Ordering portals.
View Online Food Delivery Research Papers on Academia.edu for free. ... The online food ordering system is one of the latest services. With this method, food is ordered online and delivered to the customer. ... The case traces the origin of online food delivery services in India and highlights the challenges to be faced by restaurateurs because ...
Mayurkumar Patel (2015) "Online food order system for restaurants", is a paper mainly proposes how to developan online food ordering system for both the customers and restaurants. The main aim is to highlight how this system will allow the hotels and restaurants to increase the scope of business by reducing the labour cost involved.
INTERNATIONAL JOURNAL OF INTERNATIONAL INSTITUTE OF MANAGEMENT STUDIES / VOL 2/ ISSUE 1/ JAN/ 2023. Page | 105. The table shows that majorly 90% and 83.8% respondents who order food online and who having family income up to 2Lakh and 5.1L - 7.5L want to pay by google pay and spend more than 200rs. 5.5 Cross tabulation of Food order on each ...
This research paper's goal is to discuss how Chennai residents feel about online meal ordering and delivery services. The aim goal of the study was to analyse the responses of 118 participants. ... online food ordering apps are gaining popularity in India. Online food ordering has become more popular among customers and business owners alike in ...
rise at a steady rate of 15% over the last year and i s projected to grow at the same rate in t he coming. years. The proliferation of online food ordering in the Indian market is largely due to t ...
In this paper, an attempt has been made by the authors to identify the preferred food aggregators operating in the Indian online food delivery market and to find the major reasons behind ordering food through these food aggregators. online food delivery, food aggregators, online marketing. 1) Rathore S. Suryadev, Chaudhary Mahik (2018).
a School of Computer Science Engineering, Lovely Professional University, Phagwara, Punjab, India b School of Mechanical Engineering, Lovely Professional University, Phagwara, Punjab, India Abstract. The net Food Ordering System delineated during this paper has been designed to fill a particular niche within the market by providing
An Analysis of Online Food Ordering Applications in India: Zomato and Swiggy Anupriya Saxena (Amity University, ABS, Lucknow, Uttar Pradesh, India) ... research papers, blogs, magazines, news paper.
The food services market in India is set to reach Rs 10 lakh crore by 2030, with an estimated customer base of up to 45 crore, driven by an 18 per cent CAGR in online food delivery. Currently valued at Rs 5.5 lakh crore, the market is expected to grow at 10-12 per cent annually, with online delivery contributing 20 per cent by 2030, fueled by expanding customer base, digitisation, and regional ...
The OECD designs international standards and guidelines for development co-operation, based on best practices, and monitors their implementation by its members. It works closely with member and partner countries, and other stakeholders (such as the United Nations and other multilateral entities) to help them implement their development commitments. It also invites developing country ...
287 respondents who participated in both surveys. According to the data, the most common frequency of. ordering food online is less than three times a month, with 37.63% of respondents selecting ...