
An official website of the United States government
Here’s how you know
Official websites use .gov A .gov website belongs to an official government organization in the United States.
Secure .gov websites use HTTPS A lock ( A locked padlock ) or https:// means you’ve safely connected to the .gov website. Share sensitive information only on official, secure websites.
- Heart-Healthy Living
- High Blood Pressure
- Sickle Cell Disease
- Sleep Apnea
- Information & Resources on COVID-19
- The Heart Truth®
- Learn More Breathe Better®
- Blood Diseases and Disorders Education Program
- Publications and Resources
- Blood Disorders and Blood Safety
- Sleep Science and Sleep Disorders
- Lung Diseases
- Health Disparities and Inequities
- Heart and Vascular Diseases
- Precision Medicine Activities
- Obesity, Nutrition, and Physical Activity
- Population and Epidemiology Studies
- Women’s Health
- Research Topics
- Clinical Trials
- All Science A-Z
- Grants and Training Home
- Policies and Guidelines
- Funding Opportunities and Contacts
- Training and Career Development
- Email Alerts
- NHLBI in the Press
- Research Features
- Past Events
- Upcoming Events
- Mission and Strategic Vision
- Divisions, Offices and Centers
- Advisory Committees
- Budget and Legislative Information
- Jobs and Working at the NHLBI
- Contact and FAQs
- NIH Sleep Research Plan
- < Back To Research Topics

Coronary Heart Disease Research
Language switcher.
For almost 75 years, the NHLBI has been at the forefront of improving the nation’s health and reducing the burden of heart and vascular diseases . Heart disease, including coronary heart disease, remains the leading cause of death in the United States. However, the rate of heart disease deaths has declined by 70% over the past 50 years, thanks in part to NHLBI-funded research. Many current studies funded by the NHLBI focus on discovering genetic associations and finding new ways to prevent and treat the onset of coronary heart disease and associated medical conditions.

NHLBI research that really made a difference
The NHLBI supports a wide range of long-term studies to understand the risk factors of coronary heart disease. These ongoing studies, among others, have led to many discoveries that have increased our understanding of the causes of cardiovascular disease among different populations, helping to shape evidence-based clinical practice guidelines.
- Risk factors that can be changed: The NHLBI Framingham Heart Study (FHS) revealed that cardiovascular disease is caused by modifiable risk factors such as smoking, high blood pressure , obesity , high cholesterol levels, and physical inactivity. It is why, in routine physicals, healthcare providers check for high blood pressure, high cholesterol, unhealthy eating patterns, smoking, physical inactivity, and unhealthy weight. The FHS found that cigarette smoking increases the risk of heart disease. Researchers also showed that cardiovascular disease can affect people differently depending on sex or race, underscoring the need to address health disparities.
- Risk factors for Hispanic/Latino adults: The Hispanic Community Health Study/Study of Latinos (HCHS/SOL) found that heart disease risk factors are widespread among Hispanic/Latino adults in the United States , with 80% of men and 71% of women having at least one risk factor. Researchers also used HCHS/SOL genetic data to explore genes linked with central adiposity (the tendency to have excess body fat around the waist) in Hispanic/Latino adults. Before this study, genes linked with central adiposity, a risk factor for coronary heart disease, had been identified in people of European ancestry. These results showed that those genes also predict central adiposity for Hispanic/Latino communities. Some of the genes identified were more common among people with Mexican or Central/South American ancestry, while others were more common among people of Caribbean ancestry.
- Risk factors for African Americans: The Jackson Heart Study (JHS) began in 1997 and includes more than 5,300 African American men and women in Jackson, Mississippi. It has studied genetic and environmental factors that raise the risk of heart problems, especially high blood pressure, coronary heart disease, heart failure , stroke , and peripheral artery disease (PAD) . Researchers discovered a gene variant in African American individuals that doubles the risk of heart disease. They also found that even small spikes in blood pressure can lead to a higher risk of death. A community engagement component of the JHS is putting 20 years of the study’s findings into action by turning traditional gathering places, such as barbershops and churches, into health information hubs.
- Risk factors for American Indians: The NHLBI actively supports the Strong Heart Study , a long-term study that began in 1988 to examine cardiovascular disease and its risk factors among American Indian men and women. The Strong Heart Study is one of the largest epidemiological studies of American Indian people ever undertaken. It involves a partnership with 12 Tribal Nations and has followed more than 8,000 participants, many of whom live in low-income rural areas of Arizona, Oklahoma, and the Dakotas. Cardiovascular disease remains the leading cause of death for American Indian people. Yet the prevalence and severity of cardiovascular disease among American Indian people has been challenging to study because of the small sizes of the communities, as well as the relatively young age, cultural diversity, and wide geographic distribution of the population. In 2019, the NHLBI renewed its commitment to the Strong Heart Study with a new study phase that includes more funding for community-driven pilot projects and a continued emphasis on training and development. Read more about the goals and key findings of the Strong Heart Study.
Current research funded by the NHLBI
Within our Division of Cardiovascular Sciences , the Atherothrombosis and Coronary Artery Disease Branch of its Adult and Pediatric Cardiac Research Program and the Center for Translation Research and Implementation Science oversee much of our funded research on coronary heart disease.
Research funding
Find funding opportunities and program contacts for research on coronary heart disease.
Current research on preventing coronary heart disease
- Blood cholesterol and coronary heart disease: The NHLBI supports new research into lowering the risk of coronary heart disease by reducing levels of cholesterol in the blood. High levels of blood cholesterol, especially a type called low-density lipoprotein (LDL) cholesterol, raise the risk of coronary heart disease. However, even with medicine that lowers LDL cholesterol, there is still a risk of coronary heart disease due to other proteins, called triglyceride-rich ApoB-containing lipoproteins (ApoBCLs), that circulate in the blood. Researchers are working to find innovative ways to reduce the levels of ApoBCLs, which may help prevent coronary heart disease and other cardiovascular conditions.
- Pregnancy, preeclampsia, and coronary heart disease risk: NHLBI-supported researchers are investigating the link between developing preeclampsia during pregnancy and an increased risk for heart disease over the lifespan . This project uses “omics” data – such as genomics, proteomics, and other research areas – from three different cohorts of women to define and assess preeclampsia biomarkers associated with cardiovascular health outcomes. Researchers have determined that high blood pressure during pregnancy and low birth weight are predictors of atherosclerotic cardiovascular disease in women . Ultimately, these findings can inform new preventive strategies to lower the risk of coronary heart disease.
- Community-level efforts to lower heart disease risk among African American people: The NHLBI is funding initiatives to partner with churches in order to engage with African American communities and lower disparities in heart health . Studies have found that church-led interventions reduce risk factors for coronary heart disease and other cardiovascular conditions. NHLBI-supported researchers assessed data from more than 17,000 participants across multiple studies and determined that these community-based approaches are effective in lowering heart disease risk factors .
Find more NHLBI-funded studies on preventing coronary heart disease on the NIH RePORTER.

Learn about the impact of COVID-19 on your risk of coronary heart disease.
Current research on understanding the causes of coronary heart disease
- Pregnancy and long-term heart disease: NHLBI researchers are continuing the Nulliparous Pregnancy Outcomes Study: Monitoring Mothers-to-be (nuMoM2b) study to understand the relationship between pregnancy-related problems, such as gestational hypertension, and heart problems. The study also looks at how problems during pregnancy may increase risk factors for heart disease later in life. NuMoM2b launched in 2010, and long-term studies are ongoing, with the goal of collecting high-quality data and understanding how heart disease develops in women after pregnancy.
- How coronary artery disease affects heart attack risk: NHLBI-funded researchers are investigating why some people with coronary artery disease are more at risk for heart attacks than others. Researchers have found that people with coronary artery disease who have high-risk coronary plaques are more likely to have serious cardiac events, including heart attacks. However, we do not know why some people develop high-risk coronary plaques and others do not. Researchers hope that this study will help providers better identify which people are most at risk of heart attacks before they occur.
- Genetics of coronary heart disease: The NHLBI supports studies to identify genetic variants associated with coronary heart disease . Researchers are investigating how genes affect important molecular cascades involved in the development of coronary heart disease . This deeper understanding of the underlying causes for plaque buildup and damage to the blood vessels can inform prevention strategies and help healthcare providers develop personalized treatment for people with coronary heart disease caused by specific genetic mutations.
Find more NHLBI-funded studies on understanding the causes of coronary heart disease on the NIH RePORTER.

Recent findings suggest that cholesterol-lowering treatment can lower the risk of heart disease complications in people with HIV.
Current research on treatments for coronary heart disease
- Insight into new molecular targets for treatment: NHLBI-supported researchers are investigating the role of high-density lipoprotein (HDL) cholesterol in coronary heart disease and other medical conditions . Understanding how the molecular pathways of cholesterol affect the disease mechanism for atherosclerosis and plaque buildup in the blood vessels of the heart can lead to new therapeutic approaches for the treatment of coronary heart disease. Researchers have found evidence that treatments that boost HDL function can lower systemic inflammation and slow down plaque buildup . This mechanism could be targeted to develop a new treatment approach for coronary heart disease.
- Long-term studies of treatment effectiveness: The NHLBI is supporting the International Study of Comparative Health Effectiveness with Medical and Invasive Approaches (ISCHEMIA) trial EXTENDed Follow-up (EXTEND) , which compares the long-term outcomes of an initial invasive versus conservative strategy for more than 5,000 surviving participants of the original ISCHEMIA trial. Researchers have found no difference in mortality outcomes between invasive and conservative management strategies for patients with chronic coronary heart disease after more than 3 years. They will continue to follow up with participants for up to 10 years. Researchers are also assessing the impact of nonfatal events on long-term heart disease and mortality. A more accurate heart disease risk score will be constructed to help healthcare providers deliver more precise care for their patients.
- Evaluating a new therapy for protecting new mothers: The NHLBI is supporting the Randomized Evaluation of Bromocriptine In Myocardial Recovery Therapy for Peripartum Cardiomyopathy (REBIRTH) , for determining the role of bromocriptine as a treatment for peripartum cardiomyopathy (PPCM). Previous research suggests that prolactin, a hormone that stimulates the production of milk for breastfeeding, may contribute to the development of cardiomyopathy late in pregnancy or the first several months postpartum. Bromocriptine, once commonly used in the United States to stop milk production, has shown promising results in studies conducted in South Africa and Germany. Researchers will enroll approximately 200 women across North America who have been diagnosed with PPCM and assess their heart function after 6 months.
- Impact of mental health on response to treatment: NHLBI-supported researchers are investigating how mental health conditions can affect treatment effectiveness for people with coronary heart disease. Studies show that depression is linked to a higher risk for negative outcomes from coronary heart disease. Researchers found that having depression is associated with poor adherence to medical treatment for coronary heart disease . This means that people with depression are less likely to follow through with their heart disease treatment plans, possibly contributing to their chances of experiencing worse outcomes. Researchers are also studying new ways to treat depression in patients with coronary heart disease .
Find more NHLBI-funded studies on treating coronary heart disease on the NIH RePORTER.

Researchers have found no clear difference in patient survival or heart attack risk between managing heart disease through medication and lifestyle changes compared with invasive procedures.
Coronary heart disease research labs at the NHLBI
- Laboratory of Cardiac Physiology
- Laboratory of Cardiovascular Biology
- Minority Health and Health Disparities Population Laboratory
- Social Determinants of Obesity and Cardiovascular Risk Laboratory
- Laboratory for Cardiovascular Epidemiology and Genomics
- Laboratory for Hemostasis and Platelet Biology
Related coronary heart disease programs
- In 2002, the NHLBI launched The Heart Truth® , the first federally sponsored national health education program designed to raise awareness about heart disease as the leading cause of death in women. The NHLBI and The Heart Truth® supported the creation of the Red Dress® as the national symbol for awareness about women and heart disease, and also coordinate National Wear Red Day ® and American Heart Month each February.
- The Biologic Specimen and Data Repository Information Coordinating Center (BioLINCC) facilitates access to and maximizes the scientific value of NHLBI biospecimen and data collections. A main goal is to promote the use of these scientific resources by the broader research community. BioLINCC serves to coordinate searches across data and biospecimen collections and provide an electronic means for requesting additional information and submitting requests for collections. Researchers wanting to submit biospecimen collections to the NHLBI Biorepository to share with qualified investigators may also use the website to initiate the application process.
- Our Trans-Omics for Precision Medicine (TOPMed) Program studies the ways genetic information, along with information about health status, lifestyle, and the environment, can be used to predict the best ways to prevent and treat heart, lung, blood, and sleep disorders. TOPMed specifically supports NHLBI’s Precision Medicine Activities.
- NHLBI population and epidemiology studies in different groups of people, including the Atherosclerosis Risk in Communities (ARIC) Study , the Multi-Ethnic Study of Atherosclerosis (MESA) , and the Cardiovascular Health Study (CHS) , have made major contributions to understanding the causes and prevention of heart and vascular diseases, including coronary heart disease.
- The Cardiothoracic Surgical Trials Network (CTSN) is an international clinical research enterprise that studies heart valve disease , arrhythmias , heart failure, coronary heart disease, and surgical complications. The trials span all phases of development, from early translation to completion, and have more than 14,000 participants. The trials include six completed randomized clinical trials, three large observational studies, and many other smaller studies.

Learn how heart disease may be different for women than for men.
Explore more NHLBI research on coronary heart disease
The sections above provide you with the highlights of NHLBI-supported research on coronary heart disease. You can explore the full list of NHLBI-funded studies on the NIH RePORTER .
To find more studies:
- Type your search words into the Quick Search box and press enter.
- Check Active Projects if you want current research.
- Select the Agencies arrow, then the NIH arrow, then check NHLBI .
If you want to sort the projects by budget size — from the biggest to the smallest — click on the FY Total Cost by IC column heading.
Thank you for visiting nature.com. You are using a browser version with limited support for CSS. To obtain the best experience, we recommend you use a more up to date browser (or turn off compatibility mode in Internet Explorer). In the meantime, to ensure continued support, we are displaying the site without styles and JavaScript.
- View all journals
- Explore content
- About the journal
- Publish with us
- Sign up for alerts
- Open access
- Published: 12 November 2020
Early and accurate detection and diagnosis of heart disease using intelligent computational model
- Yar Muhammad 1 ,
- Muhammad Tahir 1 ,
- Maqsood Hayat 1 &
- Kil To Chong 2
Scientific Reports volume 10 , Article number: 19747 ( 2020 ) Cite this article
26k Accesses
77 Citations
Metrics details
- Cardiovascular diseases
- Computational biology and bioinformatics
- Health care
- Heart failure
Heart disease is a fatal human disease, rapidly increases globally in both developed and undeveloped countries and consequently, causes death. Normally, in this disease, the heart fails to supply a sufficient amount of blood to other parts of the body in order to accomplish their normal functionalities. Early and on-time diagnosing of this problem is very essential for preventing patients from more damage and saving their lives. Among the conventional invasive-based techniques, angiography is considered to be the most well-known technique for diagnosing heart problems but it has some limitations. On the other hand, the non-invasive based methods, like intelligent learning-based computational techniques are found more upright and effectual for the heart disease diagnosis. Here, an intelligent computational predictive system is introduced for the identification and diagnosis of cardiac disease. In this study, various machine learning classification algorithms are investigated. In order to remove irrelevant and noisy data from extracted feature space, four distinct feature selection algorithms are applied and the results of each feature selection algorithm along with classifiers are analyzed. Several performance metrics namely: accuracy, sensitivity, specificity, AUC, F1-score, MCC, and ROC curve are used to observe the effectiveness and strength of the developed model. The classification rates of the developed system are examined on both full and optimal feature spaces, consequently, the performance of the developed model is boosted in case of high variated optimal feature space. In addition, P-value and Chi-square are also computed for the ET classifier along with each feature selection technique. It is anticipated that the proposed system will be useful and helpful for the physician to diagnose heart disease accurately and effectively.
Similar content being viewed by others
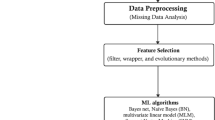
Analyzing the impact of feature selection methods on machine learning algorithms for heart disease prediction
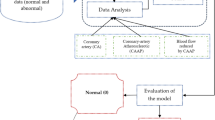
An active learning machine technique based prediction of cardiovascular heart disease from UCI-repository database
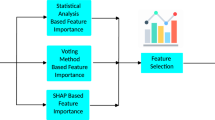
Finding the influential clinical traits that impact on the diagnosis of heart disease using statistical and machine-learning techniques
Introduction.
Heart disease is considered one of the most perilous and life snatching chronic diseases all over the world. In heart disease, normally the heart fails to supply sufficient blood to other parts of the body to accomplish their normal functionality 1 . Heart failure occurs due to blockage and narrowing of coronary arteries. Coronary arteries are responsible for the supply of blood to the heart itself 2 . A recent survey reveals that the United States is the most affected country by heart disease where the ratio of heart disease patients is very high 3 . The most common symptoms of heart disease include physical body weakness, shortness of breath, feet swollen, and weariness with associated signs, etc. 4 . The risk of heart disease may be increased by the lifestyle of a person like smoking, unhealthy diet, high cholesterol level, high blood pressure, deficiency of exercise and fitness, etc. 5 . Heart disease has several types in which coronary artery disease (CAD) is the common one that can lead to chest pain, stroke, and heart attack. The other types of heart disease include heart rhythm problems, congestive heart failure, congenital heart disease (birth time heart disease), and cardiovascular disease (CVD). Initially, traditional investigation techniques were used for the identification of heart disease, however, they were found complex 6 . Owing to the non-availability of medical diagnosing tools and medical experts specifically in undeveloped countries, diagnosis and cure of heart disease are very complex 7 . However, the precise and appropriate diagnosis of heart disease is very imperative to prevent the patient from more damage 8 . Heart disease is a fatal disease that rapidly increases in both economically developed and undeveloped countries. According to a report generated by the World Health Organization (WHO), an average of 17.90 million humans died from CVD in 2016. This amount represents approximately 30% of all global deaths. According to a report, 0.2 million people die from heart disease annually in Pakistan. Every year, the number of victimizing people is rapidly increasing. European Society of Cardiology (ESC) has published a report in which 26.5 million adults were identified having heart disease and 3.8 million were identified each year. About 50–55% of heart disease patients die within the initial 1–3 years, and the cost of heart disease treatment is about 4% of the overall healthcare annual budget 9 .
Conventional invasive-based methods used for the diagnosis of heart disease which were based on the medical history of a patient, physical test results, and investigation of related symptoms by the doctors 10 . Among the conventional methods, angiography is considered one of the most precise technique for the identification of heart problems. Conversely, angiography has some drawbacks like high cost, various side effects, and strong technological knowledge 11 . Conventional methods often lead to imprecise diagnosis and take more time due to human mistakes. In addition, it is a very expensive and computational intensive approach for the diagnosis of disease and takes time in assessment 12 .
To overcome the issues in conventional invasive-based methods for the identification of heart disease, researchers attempted to develop different non-invasive smart healthcare systems based on predictive machine learning techniques namely: Support Vector Machine (SVM), K-Nearest Neighbor (KNN), Naïve Bayes (NB), and Decision Tree (DT), etc. 13 . As a result, the death ratio of heart disease patients has been decreased 14 . In literature, the Cleveland heart disease dataset is extensively utilized by the researchers 15 , 16 .
In this regard, Robert et al . 17 have used a logistic regression classification algorithm for heart disease detection and obtained an accuracy of 77.1%. Similarly, Wankhade et al . 18 have used a multi-layer perceptron (MLP) classifier for heart disease diagnosis and attained accuracy of 80%. Likewise, Allahverdi et al . 19 have developed a heart disease classification system in which they integrated neural networks with an artificial neural network and attained an accuracy of 82.4%. In a sequel, Awang et al . 20 have used NB and DT for the diagnosis and prediction of heart disease and achieved reasonable results in terms of accuracy. They achieved an accuracy of 82.7% with NB and 80.4% with DT. Oyedodum and Olaniye 21 have proposed a three-phase system for the prediction of heart disease using ANN. Das and Turkoglu 22 have proposed an ANN ensemble-based predictive model for the prediction of heart disease. Similarly, Paul and Robin 23 have used the adaptive fuzzy ensemble method for the prediction of heart disease. Likewise, Tomov et al. 24 have introduced a deep neural network for heart disease prediction and his proposed model performed well and produced good outcomes. Further, Manogaran and Varatharajan 25 have introduced the concept of a hybrid recommendation system for diagnosing heart disease and their model has given considerable results. Alizadehsani et al . 26 have developed a non-invasive based model for the prediction of coronary artery disease and showed some good results regarding the accuracy and other performance assessment metrics. Amin et al . 27 have proposed a framework of a hybrid system for the identification of cardiac disease, using machine learning, and attained an accuracy of 86.0%. Similarly, Mohan et al . 28 have proposed another intelligent system that integrates RF with a linear model for the prediction of heart disease and achieved the classification accuracy of 88.7%. Likewise, Liaqat et al . 29 have developed an expert system that uses stacked SVM for the prediction of heart disease and obtained 91.11% classification accuracy on selected features.
The contribution of the current work is to introduce an intelligent medical decision system for the diagnosis of heart disease based on contemporary machine learning algorithms. In this study, 10 different nature of machine learning classification algorithms such as Logistic Regression (LR), Decision Tree (DT), Naïve Bayes (NB), Random Forest (RF), Artificial Neural Network (ANN), etc. are implemented in order to select the best model for timely and accurate detection of heart disease at an early stage. Four feature selection algorithms, Fast Correlation-Based Filter Solution (FCBF), minimal redundancy maximal relevance (mRMR), Least Absolute Shrinkage and Selection Operator (LASSO), and Relief have been used for selecting the vital and more correlated features that have truly reflect the motif of the desired target. Our developed system has been trained and tested on the Cleveland (S 1 ) and Hungarian (S 2 ) heart disease datasets which are available online on the UCI machine learning repository. All the processing and computations were performed using Anaconda IDE. Python has been used as a tool for implementing all the classifiers. The main packages and libraries used include pandas, NumPy, matplotlib, sci-kit learn (sklearn), and seaborn. The main contribution of our proposed work is given below:
The performance of all classifiers has been tested on full feature spaces in terms of all performance evaluation matrices specifically accuracy.
The performances of the classifiers are tested on selected feature spaces, selected through various feature selection algorithms mentioned above.
The research study recommends that which feature selection algorithm is feasible with which classification algorithm for developing a high-level intelligence system for the diagnosing of heart disease patients.
The rest of the paper is organized as: “ Results and discussion ” section represents the results and discussion, “ Material and methods ” section describes the material and methods used in this paper. Finally, we conclude our proposed research work in “ Conclusion ” section.
Results and discussion
This section of the paper discusses the experimental results of various contemporary classification algorithms. At first, the performance of all used classification models i.e. K-Nearest Neighbors (KNN), Decision Tree (DT), Extra-Tree Classifier (ETC), Random Forest (RF), Logistic Regression (LR), Naïve Bayes (NB), Artificial Neural Network (ANN), Support Vector Machine (SVM), Adaboost (AB), and Gradient Boosting (GB) along with full feature space is evaluated. After that, four feature selection algorithms (FSA): Fast Correlation-Based Filter (FCBF), Minimal Redundancy Maximal Relevance (mRMR), Least Absolute Shrinkage and Selection Operator (LASSO), and Relief are applied to select the prominent and high variant features from feature space. Furthermore, the selected feature spaces are provided to classification algorithms as input to analyze the significance of feature selection techniques. The cross-validation techniques i.e. k-fold (10-fold) are applied on both the full and selected feature spaces to analyze the generalization power of the proposed model. Various performance evaluation metrics are implemented for measuring the performances of the classification models.
Classifiers’ predictive outcomes on full feature space
The experimental outcomes of the applied classification algorithms on the full feature space of the two benchmark datasets by using 10-fold cross-validation (CV) techniques are shown in Tables 1 and 2 , respectively.
The experimental results demonstrated that the ET classifier performed quite well in terms of all performance evaluation metrics compared to the other classifiers using 10-fold CV. ET achieved 92.09% accuracy, 91.82% sensitivity, 92.38% specificity, 97.92% AUC, 92.84% Precision, 0.92 F1-Score and 0.84 MCC. The specificity indicates that the diagnosed test was negative and the individual doesn't have the disease. While the sensitivity indicates the diagnostic test was positive and the patient has heart disease. In the case of the KNN classification model, multiple experiments were accomplished by considering various values for k i.e. k = 3, 5, 7, 9, 13, and 15, respectively. Consequently, KNN has shown the best performance at value k = 7 and achieved a classification accuracy of 85.55%, 85.93% sensitivity, 85.17% specificity, 95.64% AUC, 86.09% Precision, 0.86 F1-Score, and 0.71 MCC. Similarly, DT classifier has achieved accuracy of 86.82%, 89.73% sensitivity, 83.76% specificity, 91.89% AUC, 85.40% Precision, 0.87 F1-Score, and 0.73 MCC. Likewise, GB classifier has yielded accuracy of 91.34%, 90.32% sensitivity, 91.52% specificity, 96.87% AUC, 92.14% Precision, 0.92 F1-Score, and 0.83 MCC. After empirically evaluating the success rates of all classifiers, it is observed that ET Classifier out-performed among all the used classification algorithms in terms of accuracy, sensitivity, and specificity. Whereas, NB shows the lowest performance in terms of accuracy, sensitivity, and specificity. The ROC curve of all classification algorithms on full feature space is represented in Fig. 1 .
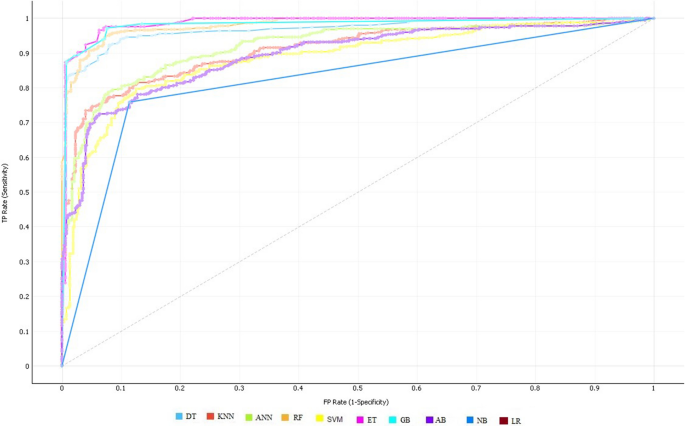
ROC curves of all classifiers on full feature space using 10-fold cross-validation on S 1 .
In the case of dataset S 2 , composed of 1025 total instances in which 525 belong to the positive class and 500 instances of having negative class, again ET has obtained quite well results compared to other classifiers using a 10-fold cross-validation test, which are 96.74% accuracy, 96.36 sensitivity, 97.40% specificity, and 0.93 MCC as shown in Table 2 .
Classifiers’ predictive outcomes on selected feature space
Fcbf feature selection technique.
FCBF feature selection technique is applied to select the best subset of feature space. In this attempt, various length of subspaces is generated and tested. Finally, the best results are achieved by classification algorithms on the subset of feature space (n = 6) using a 10-fold CV. Table 3 shows various performance measures of classifiers executed on the selected features space of FCBF.
Table 3 demonstrates that the ET classifier obtained quite good results including accuracy of 94.14%, 94.29% sensitivity, and specificity of 93.98%. In contrast, NB reported the lowest performance compared to the other classification algorithms. The performance of classification algorithms is also illustrated in Fig. 2 by using ROC curves.
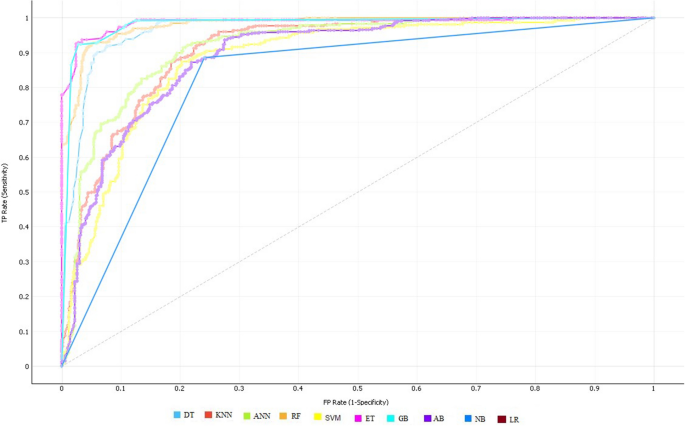
ROC curve of all classifiers on selected features by FCBF feature selection algorithm.
mRMR feature selection technique
mRMR feature selection technique is used in order to select a subset of features that enhance the performance of classifiers. The best results reported on a subset of n = 6 of feature space which is shown in Table 4 .
In the case of mRMR, still, the success rates of the ET classifier are well in terms of all performance evaluation metrics compared to the other classifiers. ET has attained 93.42% accuracy, 93.92% sensitivity, and specificity of 93.88%. In contrast, NB has achieved the lowest outcomes which are 81.84% accuracy. Figure 3 shows the ROC curve of all ten classifiers using the mRMR feature selection algorithm.
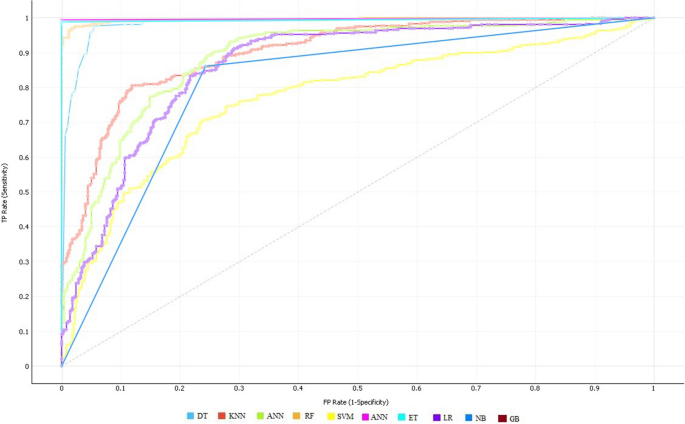
ROC curve of all classifiers on selected features using the mRMR feature selection algorithm.
LASSO feature selection technique
In order to choose the optimal feature space which not only reduces computational cost but also progresses the performance of the classifiers, LASSO feature selection technique is applied. After performing various experiments on different subsets of feature space, the best results are still noted on the subspace of (n = 6). The predicted outcomes of the best-selected feature space are reported in Table 5 using the 10-fold CV.
Table 5 demonstrated that the predicted outcomes of the ET classifier are considerable and better compared to the other classifiers. ET has achieved 89.36% accuracy, 88.21% sensitivity, and specificity of 90.58%. Likewise, GB has yielded the second-best result which is the accuracy of 88.47%, 89.54% sensitivity, and specificity of 87.37%. Whereas, LR has performed worse results and achieved 80.77% accuracy, 83.46% sensitivity, and specificity of 77.95%. ROC curves of the classifiers are shown in Fig. 4 .
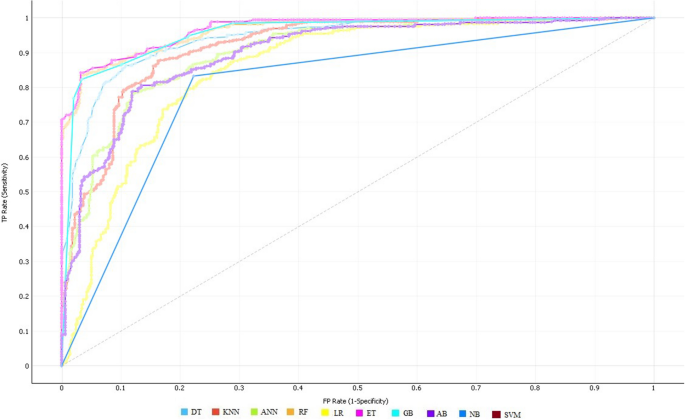
ROC curve of all classifiers on selected feature space using the LASSO feature selection algorithm.
Relief feature selection technique
In a sequel, another feature selection technique Relief is applied to investigate the performance of classifiers on different sub-feature spaces by using the wrapper method. After empirically analyzing the results of the classifiers on a different subset of feature spaces, it is observed that the performance of classifiers is outstanding on the sub-space of length (n = 6). The results of the optimal feature space on the 10-fold CV technique are listed in Table 6 .
Again, the ET classifier performed outstandingly in terms of all performance evaluation metrics as compared to other classifiers. ET has obtained an accuracy of 94.41%, 94.93% sensitivity, and specificity of 94.89%. In contrast, NB has shown the lowest performance and achieved 80.29% accuracy, 81.93% sensitivity, and specificity of 78.55%. The ROC curves of the classifiers are demonstrated in Fig. 5 .
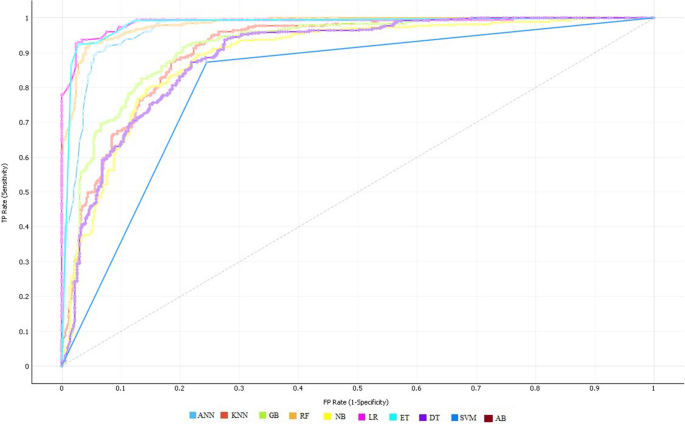
ROC curve of all classifiers on selected features selected by the Relief feature selection algorithm.
After executing classification algorithms along with full and selected feature spaces in order to select the optimal algorithm for the operational engine, the empirical results have revealed that ET performed well not only on all feature space but also on optimal selected feature space among all the used classification algorithms. Furthermore, the ET classifier obtained quite promising accuracy in the case of the Relief feature selection technique which is 94.41%. Overall, the performance of ET is reported better in terms of most of the measures while other classifiers have shown good results in one measure while worse in other measures. In addition, the performance of the ET classifier is also evaluated on a 10-fold CV in combination with different sub-feature spaces of varying length starting from 1 to 12 with a step size of 1 to check the stability and discrimination power of the classifier as described in 30 . Doing so will assist the readers to have a better understanding of the impact, of the number of selected features on the performance of the classifiers. The same process is repeated for another dataset i.e. S 2 (Hungarian heart disease dataset) as well, to know the impact of selected features on the classification performance.
Tables 7 and 8 shows the performance of the ET classifier using 10-fold CV in combination with different feature sub-spaces starting from 1 to 12 with a step size of 1. The experimental results show that the performance of the ET classifier is affected significantly by using the varying length of sub-feature spaces. Finally, it is concluded that all these achievements are ascribed with the best selection of Relief feature selection technique which not only reduces the feature space but also enhances the predictive power of classifiers. In addition, the ET classifier has also played a quite promising role in these achievements because it has clearly and precisely learned the motif of the target class and reflected it truly. In addition, the performance of the ET classifier is also evaluated on 5-fold and 7-fold CV in combination with different sub-spaces of length 5 and 7 to check the stability and discrimination power of the classifier. It is also tested on another dataset S 2 (Hungarian heart disease dataset). The results are shown in supplementary materials .
In Table 9 , P-value and Chi-Square values are also computed for the ET classifier in combination with the optimal feature spaces of different feature selection techniques.
Performance comparison with existing models
Further, a comparative study of the developed system is conducted with other states of the art machine learning approaches discussed in the literature. Table 10 represents, a brief description and classification accuracies of those approaches. The results demonstrate that our proposed model success rate is high compared to existing models in the literature.
Material and methods
The subsections represent the materials and the methods that are used in this paper.
The first and rudimentary step of developing an intelligent computational model is to construct or develop a problem-related dataset that truly and effectively reflects the pattern of the target class. Well organized and problem-related dataset has a high influence on the performance of the computational model. Looking at the significance of the dataset, two datasets i.e. the Cleveland heart disease dataset S 1 and Hungarian heart disease dataset (S 2 ) are used, which are available online at the University of California Irvine (UCI) machine learning repository and UCI Kaggle repository, and various researchers have used it for conducting their research studies 28 , 31 , 32 . The S1 consists of 304 instances, where each instance has distinct 13 attributes along with the target labels and are selected for training. The dataset is composed of two classes, presence or absence of heart disease. The S 2 is composed of 1025 instances in which 525 instances belong to positive class while the rest of 500 instances have negative class. The description of attributes of both the datasets is the same, and both have similar attributes. The complete description and information of the datasets with 13 attributes are given in Table 11 .
Proposed system methodology
The main theme of the developed system is to identify heart problems in human beings. In this study, four distant feature selection techniques namely: FCBF, mRMR, Relief, and LASSO are applied on the provided dataset in order to remove noisy, redundant features and select variant features, consequently may cause of enhancing the performance of the proposed model. Various machine learning classification algorithms are used in this study which include, KNN, DT, ETC, RF, LR, NB, ANN, SVM, AB, and GB. Different evaluation metrics are computed to assess the performance of classification algorithms. The methodology of the proposed system is carried out in five stages which include dataset preprocessing, selection of features, cross-validation technique, classification algorithms, and performance evaluation of classifiers. The framework of the proposed system is illustrated in Fig. 6 .
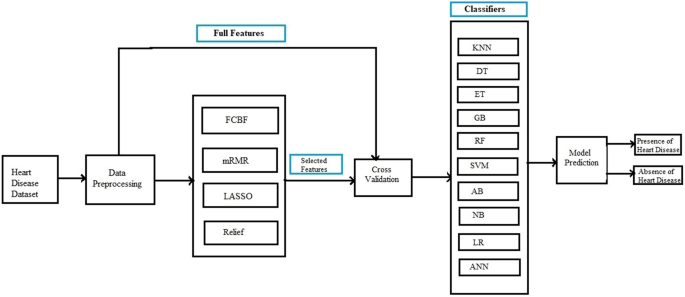
An Intelligent Hybrid Framework for the prediction of heart disease.
Preprocessing of data
Data preprocessing is the process of transforming raw data into meaningful patterns. It is very crucial for a good representation of data. Various preprocessing approaches such as missing values removal, standard scalar, and Min–Max scalar are used on the dataset in order to make it more effective for classification.
Feature selection algorithms
Feature selection technique selects the optimal features sub-space among all the features in a dataset. It is very crucial because sometimes, the classification performance degrades due to irrelevant features in the dataset. The feature selection technique improves the performance of classification algorithms and also reduces their execution time. In this research study, four feature selection techniques are used and are listed below:
Fast correlation-based filter (FCBF): FCBF feature selection algorithm follows a sequential search strategy. It first selects full features and then uses symmetric uncertainty for measuring the dependencies of the features on each other and how they affect the target output label. After this, it selects the most important features using the backward sequential search strategy. FCBF outperforms on high dimensional datasets. Table 12 shows the results of the selected features (n = 6) by using the FCBF feature selection algorithm. Each attribute is given a weight based on its importance. According to the FCBF feature selection technique, the most important features are THA and CPT as shown in Table 12 . The ranking that the FCBF gives to all the features of the dataset is shown in Fig. 7 .
Minimal redundancy maximal relevance (mRMR): mRMR uses the heuristic approach for selecting the most vital features that have minimum redundancy and maximum relevance. It selects those features which are useful and relevant to the target. As it follows a heuristic approach so, it checks one feature at a time and then computes its pairwise redundancy with the other features. The mRMR feature selection algorithm is not suitable for high domain feature problems 33 . The results of selected features by the mRMR feature selection algorithm (n = 6) are listed in Table 13 . In addition, among these attributes, PES and CPT have the highest score. Figure 7 describes the attributes ranking given by the mRMR feature selection algorithm to all attributes in the feature space.
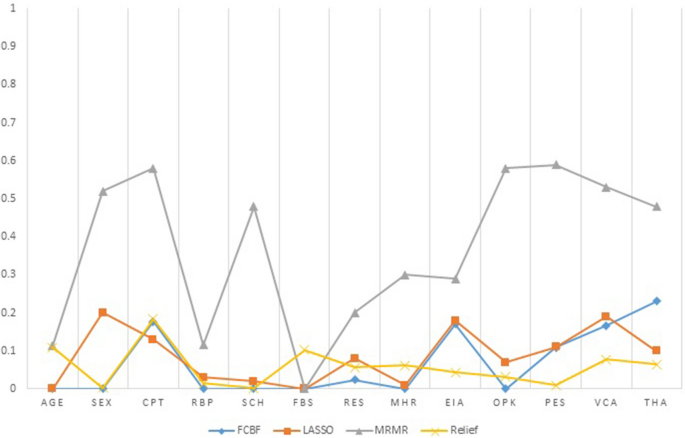
Features ranking by four feature selection algorithms (FCBF, LASSO, mRMR, Relief).
Least absolute shrinkage and selection operator (LASSO) LASSO selects features based on updating the absolute value of the features coefficient. In updating the features coefficient values, zero becoming values are removed from the features subset. LASSO outperforms with low feature coefficient values. The features having high coefficient values will be selected in the subset of features and the rest will be eliminated. Moreover, some irrelevant features with higher coefficient values may be selected and are included in the subset of features 30 . Table 14 represents the six most profound attributes which have a great correlation with the target and their scores selected by the LASSO feature selection algorithm. Figure 7 represents the important features and their scoring values given by the LASSO feature selection algorithm.
Relief feature selection algorithm Relief utilizes the concept of instance-based learning which allocates weight to each attribute based on its significance. The weight of each attribute demonstrates its capability to differentiate among class values. Attributes are rated by weights, and those attributes whose weight is exceeding a user-specified cutoff, are chosen as the final subset 34 . The relief feature selection algorithm selects the most significant attributes which have more effect on the target 35 . The algorithm operates by selecting instances randomly from the training samples. The nearest instance of the same class (nearest hit) and opposite class (nearest miss) is identified for each sampled instance. The weight of an attribute is updated according to how well its values differentiate between the sampled instance and its nearest miss and hit. If an attribute discriminates amongst instances from different classes and has the same value for instances of the same class, it will get a high weight.
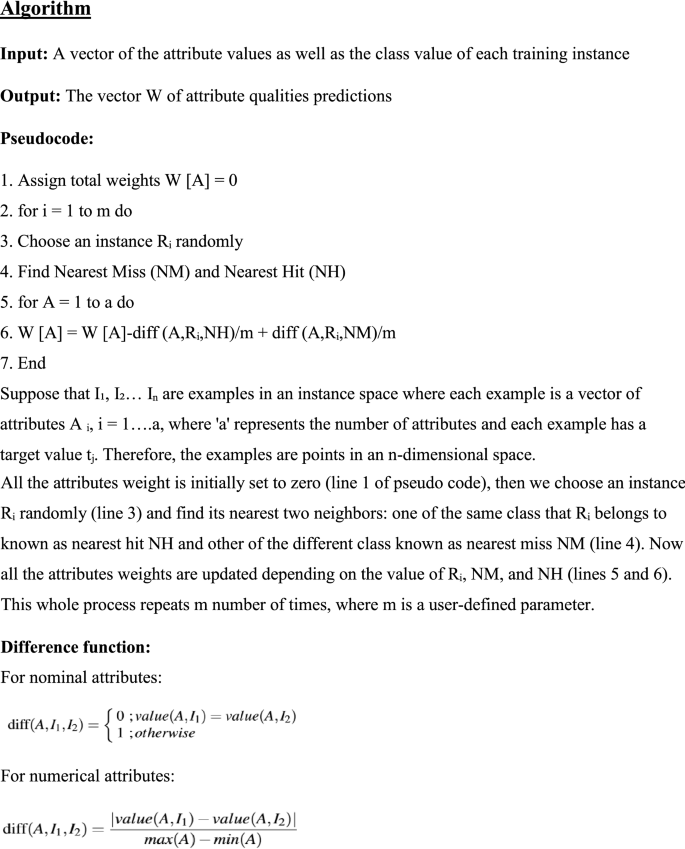
The weight updating of attributes works on a simple idea (line 6). That if instance R i and NH have dissimilar value (i.e. the diff value is large), that means the attribute splits two instances with the same class which is not worthwhile, and thus we reduce the attributes weight. On the other hand, if the instance R i and NM have a distinct value that means the attribute separates the two instances with a different class, which is desirable. The six most important features selected by the Relief algorithm are listed in descending order in Table 15 . Based on weight values the most vital features are CPT and Age. Figure 7 demonstrates the important features and their ranking given by the Relief feature selection algorithm.
Machine learning classification algorithms
Various machine learning classification algorithms are investigated for early detection of heart disease, in this study. Each classification algorithm has its significance and the importance is reported varied from application to application. In this paper, 10 distant nature of classification algorithms namely: KNN, DT, ET, GB, RF, SVM, AB, NB, LR, and ANN are applied to select the best and generalize prediction model.
Classifier validation method
Validation of the prediction model is an essential step in machine learning processes. In this paper, the K-Fold cross-validation method is applied to validating the results of the above-mentioned classification models.
K-fold cross validation (CV)
In K-Fold CV, the whole dataset is split into k equal parts. The (k-1) parts are utilized for training and the rest is used for the testing at each iteration. This process continues for k-iteration. Various researchers have used different values of k for CV. Here k = 10 is used for experimental work because it produces good results. In tenfold CV, 90% of data is utilized for training the model and the remaining 10% of data is used for the testing of the model at each iteration. At last, the mean of the results of each step is taken which is the final result.
Performance evaluation metrics
For measuring the performance of the classification algorithms used in this paper, various evaluation matrices have been implemented including accuracy, sensitivity, specificity, f1-score, recall, Mathew Correlation-coefficient (MCC), AUC-score, and ROC curve. All these measures are calculated from the confusion matrix described in Table 16 .
In confusion matrix True Negative (TN) shows that the patient has not heart disease and the model also predicts the same i.e. a healthy person is correctly classified by the model.
True Positive (TP) represents that the patient has heart disease and the model also predicts the same result i.e. a person having heart disease is correctly classified by the model.
False Positive (FP) demonstrates that the patient has not heart disease but the model predicted that the patient has i.e. a healthy person is incorrectly classified by the model. This is also called a type-1 error.
False Negative (FN) notifies that the patient has heart disease but the model predicted that the patient has not i.e. a person having heart disease is incorrectly classified by the model. This is also called a type-2 error.
Accuracy Accuracy of the classification model shows the overall performance of the model and can be calculated by the formula given below:
Specificity specificity is a ratio of the recently classified healthy people to the total number of healthy people. It means the prediction is negative and the person is healthy. The formula for calculating specificity is given as follows:
Sensitivity Sensitivity is the ratio of recently classified heart patients to the total patients having heart disease. It means the model prediction is positive and the person has heart disease. The formula for calculating sensitivity is given below:
Precision: Precision is the ratio of the actual positive score and the positive score predicted by the classification model/algorithm. Precision can be calculated by the following formula:
F1-score F1 is the weighted measure of both recall precision and sensitivity. Its value ranges between 0 and 1. If its value is one then it means the good performance of the classification algorithm and if its value is 0 then it means the bad performance of the classification algorithm.
MCC It is a correlation coefficient between the actual and predicted results. MCC gives resulting values between − 1 and + 1. Where − 1 represents the completely wrong prediction of the classifier.0 means that the classifier generates random prediction and + 1 represents the ideal prediction of the classification models. The formula for calculating MCC values is given below:
Finally, we will examine the predictability of the machine learning classification algorithms with the help of the receiver optimistic curve (ROC) which represents a graphical demonstration of the performance of ML classifiers. The area under the curve (AUC) describes the ROC of a classifier and the performance of the classification algorithms is directly linked with AUC i.e. larger the value of AUC greater will be the performance of the classification algorithm.
In this study, 10 different machine learning classification algorithms namely: LR, DT, NB, RF, ANN, KNN, GB, SVM, AB, and ET are implemented in order to select the best model for early and accurate detection of heart disease. Four feature selection algorithms such as FCBF, mRMR, LASSO, and Relief have been used to select the most vital and correlated features that truly reflect the motif of the desired target. Our developed intelligent computational model has been trained and tested on two datasets i.e. Cleveland (S1) and Hungarian (S2) heart disease datasets. Python has been used as a tool for implementation and simulating the results of all the utilized classification algorithms.
The performance of all classification models has been tested in terms of various performance metrics on full feature space as well as selected feature spaces, selected through various feature selection algorithms. This research study recommends that which feature selection algorithm is feasible with which classification model for developing a high-level intelligent system for the diagnosis of a patient having heart disease. From simulation results, it is observed that ET is the best classifier while relief is the optimal feature selection algorithm. In addition, P-value and Chi-square are also computed for the ET classifier along with each feature selection algorithm. It is anticipated that the proposed system will be useful and helpful for the doctors and other care-givers to diagnose a patient having heart disease accurately and effectively at the early stages.
Heart disease is one of the most devastating and fatal chronic diseases that rapidly increase in both economically developed and undeveloped countries and causes death. This damage can be reduced considerably if the patient is diagnosed in the early stages and proper treatment is provided to her. In this paper, we developed an intelligent predictive system based on contemporary machine learning algorithms for the prediction and diagnosis of heart disease. The developed system was checked on two datasets i.e. Cleveland (S1) and Hungarian (S2) heart disease datasets. The developed system was trained and tested on full features and optimal features as well. Ten classification algorithms including, KNN, DT, RF, NB, SVM, AB, ET, GB, LR, and ANN, and four feature selection algorithms such as FCBF, mRMR, LASSO, and Relief are used. The feature selection algorithm selects the most significant features from the feature space, which not only reduces the classification errors but also shrink the feature space. To assess the performance of classification algorithms various performance evaluation metrics were used such as accuracy, sensitivity, specificity, AUC, F1-score, MCC, and ROC curve. The classification accuracies of the top two classification algorithms i.e. ET and GB on full features were 92.09% and 91.34% respectively. After applying feature selection algorithms, the classification accuracy of ET with the relief feature selection algorithm increases from 92.09 to 94.41%. The accuracy of GB increases from 91.34 to 93.36% with the FCBF feature selection algorithm. So, the ET classifier with the relief feature selection algorithm performs excellently. P-value and Chi-square are also computed for the ET classifier with each feature selection technique. The future work of this research study is to use more optimization techniques, feature selection algorithms, and classification algorithms to improve the performance of the predictive system for the diagnosis of heart disease.
Bui, A. L., Horwich, T. B. & Fonarow, G. C. Epidemiology and risk profile of heart failure. Nat. Rev. Cardiol. 8 , 30 (2011).
Article PubMed Google Scholar
Polat, K. & Güneş, S. Artificial immune recognition system with fuzzy resource allocation mechanism classifier, principal component analysis, and FFT method based new hybrid automated identification system for classification of EEG signals. Expert Syst. Appl. 34 , 2039–2048 (2010).
Article Google Scholar
Heidenreich, P. A. et al. Forecasting the future of cardiovascular disease in the United States: A policy statement from the American Heart Association. Circulation 123 , 933–944 (2011).
Durairaj, M. & Ramasamy, N. A comparison of the perceptive approaches for preprocessing the data set for predicting fertility success rate. Int. J. Control Theory Appl. 9 , 255–260 (2016).
Google Scholar
Das, R., Turkoglu, I. & Sengur, A. Effective diagnosis of heart disease through neural networks ensembles. Expert Syst. Appl. 36 , 7675–7680 (2012).
Allen, L. A. et al. Decision making in advanced heart failure: A scientific statement from the American Heart Association. Circulation 125 , 1928–1952 (2014).
Yang, H. & Garibaldi, J. M. A hybrid model for automatic identification of risk factors for heart disease. J. Biomed. Inform. 58 , S171–S182 (2015).
Article PubMed PubMed Central Google Scholar
Alizadehsani, R., Hosseini, M. J., Sani, Z. A., Ghandeharioun, A. & Boghrati, R. In 2012 IEEE 12th International Conference on Data Mining Workshops. 9–16 (IEEE, New York).
Arabasadi, Z., Alizadehsani, R., Roshanzamir, M., Moosaei, H. & Yarifard, A. A. Computer aided decision making for heart disease detection using hybrid neural network-Genetic algorithm. Comput. Methods Programs Biomed. 141 , 19–26 (2017).
Samuel, O. W., Asogbon, G. M., Sangaiah, A. K., Fang, P. & Li, G. An integrated decision support system based on ANN and Fuzzy_AHP for heart failure risk prediction. Expert Syst. Appl. 68 , 163–172 (2017).
Patil, S. B. & Kumaraswamy, Y. Intelligent and effective heart attack prediction system using data mining and artificial neural network. Eur. J. Sci. Res. 31 , 642–656 (2009).
Vanisree, K. & Singaraju, J. Decision support system for congenital heart disease diagnosis based on signs and symptoms using neural networks. Int. J. Comput. Appl. 19 , 6–12 (2015).
B. Edmonds. In Proceedings of AISB Symposium on Socially Inspired Computing 1–12 (Hatfield, 2005).
Methaila, A., Kansal, P., Arya, H. & Kumar, P. Early heart disease prediction using data mining techniques. Comput. Sci. Inf. Technol. J. https://doi.org/10.5121/csit.2014.4807 (2014).
Samuel, O. W., Asogbon, G. M., Sangaiah, A. K., Fang, P. & Li, G. An integrated decision support system based on ANN and Fuzzy_AHP for heart failure risk prediction. Expert Syst. Appl. 68 , 163–172 (2018).
Nazir, S., Shahzad, S., Mahfooz, S. & Nazir, M. Fuzzy logic based decision support system for component security evaluation. Int. Arab J. Inf. Technol. 15 , 224–231 (2018).
Detrano, R. et al. International application of a new probability algorithm for the diagnosis of coronary artery disease. Am. J. Cardiol. 64 , 304–310 (2009).
Gudadhe, M., Wankhade, K. & Dongre, S. In 2010 International Conference on Computer and Communication Technology (ICCCT) , 741–745 (IEEE, New York).
Kahramanli, H. & Allahverdi, N. Design of a hybrid system for the diabetes and heart diseases. Expert Syst. Appl. 35 , 82–89 (2013).
Palaniappan, S. & Awang, R. In 2012 IEEE/ACS International Conference on Computer Systems and Applications 108–115 (IEEE, New York).
Olaniyi, E. O., Oyedotun, O. K. & Adnan, K. Heart diseases diagnosis using neural networks arbitration. Int. J. Intel. Syst. Appl. 7 , 72 (2015).
Das, R., Turkoglu, I. & Sengur, A. Effective diagnosis of heart disease through neural networks ensembles. Expert Syst. Appl. 36 , 7675–7680 (2011).
Paul, A. K., Shill, P. C., Rabin, M. R. I. & Murase, K. Adaptive weighted fuzzy rule-based system for the risk level assessment of heart disease. Applied Intelligence 48 , 1739–1756 (2018).
Tomov, N.-S. & Tomov, S. On deep neural networks for detecting heart disease. arXiv:1808.07168 (2018).
Manogaran, G., Varatharajan, R. & Priyan, M. Hybrid recommendation system for heart disease diagnosis based on multiple kernel learning with adaptive neuro-fuzzy inference system. Multimedia Tools Appl. 77 , 4379–4399 (2018).
Alizadehsani, R. et al. Non-invasive detection of coronary artery disease in high-risk patients based on the stenosis prediction of separate coronary arteries. Comput. Methods Programs Biomed. 162 , 119–127 (2018).
Haq, A. U., Li, J. P., Memon, M. H., Nazir, S. & Sun, R. A hybrid intelligent system framework for the prediction of heart disease using machine learning algorithms. Mobile Inf. Syst. 2018 , 3860146. https://doi.org/10.1155/2018/3860146 (2018).
Mohan, S., Thirumalai, C. & Srivastava, G. Effective heart disease prediction using hybrid machine learning techniques. IEEE Access 7 , 81542–81554 (2019).
Ali, L. et al. An optimized stacked support vector machines based expert system for the effective prediction of heart failure. IEEE Access 7 , 54007–54014 (2019).
Peng, H., Long, F. & Ding, C. Feature selection based on mutual information criteria of max-dependency, max-relevance, and min-redundancy. IEEE Trans. Pattern Anal. Mach. Intell. 27 (8), 1226–1238 (2005).
Palaniappan, S. & Awang, R. In 2008 IEEE/ACS International Conference on Computer Systems and Applications 108–115 (IEEE, New York).
Ali, L., Niamat, A., Golilarz, N. A., Ali, A. & Xingzhong, X. An expert system based on optimized stacked support vector machines for effective diagnosis of heart disease. IEEE Access (2019).
Pérez, N. P., López, M. A. G., Silva, A. & Ramos, I. Improving the Mann-Whitney statistical test for feature selection: An approach in breast cancer diagnosis on mammography. Artif. Intell. Med. 63 , 19–31 (2015).
Tibshirani, R. Regression shrinkage and selection via the lasso: A retrospective. J. R. Stat. Soc. Ser. B Stat. Methodol. 73 , 273–282 (2011).
Article MathSciNet Google Scholar
Peng, H., Long, F. & Ding, C. Feature selection based on mutual information criteria of max-dependency, max-relevance, and min-redundancy. IEEE Trans. Pattern Anal. Mach. Intell. 27 , 1226–1238 (2012).
de Silva, A. M. & Leong, P. H. Grammar-Based Feature Generation for Time-Series Prediction (Springer, Berlin, 2015).
Book Google Scholar
Download references
Acknowledgements
This research was supported by the Brain Research Program of the National Research Foundation (NRF) funded by the Korean government (MSIT) (No. NRF-2017M3C7A1044815).
Author information
Authors and affiliations.
Department of Computer Science, Abdul Wali Khan University Mardan, Mardan, 23200, KP, Pakistan
Yar Muhammad, Muhammad Tahir & Maqsood Hayat
Department of Electronic and Information Engineering, Jeonbuk National University, Jeonju, 54896, South Korea
Kil To Chong
You can also search for this author in PubMed Google Scholar
Contributions
All authors have equal contributions.
Corresponding authors
Correspondence to Maqsood Hayat or Kil To Chong .
Ethics declarations
Competing interests.
The authors declare no competing interests.
Additional information
Publisher's note.
Springer Nature remains neutral with regard to jurisdictional claims in published maps and institutional affiliations.
Supplementary information
Supplementary information., rights and permissions.
Open Access This article is licensed under a Creative Commons Attribution 4.0 International License, which permits use, sharing, adaptation, distribution and reproduction in any medium or format, as long as you give appropriate credit to the original author(s) and the source, provide a link to the Creative Commons licence, and indicate if changes were made. The images or other third party material in this article are included in the article's Creative Commons licence, unless indicated otherwise in a credit line to the material. If material is not included in the article's Creative Commons licence and your intended use is not permitted by statutory regulation or exceeds the permitted use, you will need to obtain permission directly from the copyright holder. To view a copy of this licence, visit http://creativecommons.org/licenses/by/4.0/ .
Reprints and permissions
About this article
Cite this article.
Muhammad, Y., Tahir, M., Hayat, M. et al. Early and accurate detection and diagnosis of heart disease using intelligent computational model. Sci Rep 10 , 19747 (2020). https://doi.org/10.1038/s41598-020-76635-9
Download citation
Received : 03 April 2020
Accepted : 28 October 2020
Published : 12 November 2020
DOI : https://doi.org/10.1038/s41598-020-76635-9
Share this article
Anyone you share the following link with will be able to read this content:
Sorry, a shareable link is not currently available for this article.
Provided by the Springer Nature SharedIt content-sharing initiative
This article is cited by
Comprehensive evaluation and performance analysis of machine learning in heart disease prediction.
- Halah A. Al-Alshaikh
- Abeer A. AlSanad
Scientific Reports (2024)
Future prediction for precautionary measures associated with heart-related issues based on IoT prototype
- Ganesh Keshaorao Yenurkar
- Aniket Pathade
Multimedia Tools and Applications (2024)
An improved machine learning-based prediction framework for early detection of events in heart failure patients using mHealth
- Deepak Kumar
- Keerthiveena Balraj
- Anurag S. Rathore
Health and Technology (2024)
Identification and classification of pneumonia disease using a deep learning-based intelligent computational framework
- Lanying Tang
Neural Computing and Applications (2023)
Back propagation artificial neural network for diagnose of the heart disease
- Jagmohan Kaur
- Baljit S. Khehra
- Amarinder Singh
Journal of Reliable Intelligent Environments (2023)
By submitting a comment you agree to abide by our Terms and Community Guidelines . If you find something abusive or that does not comply with our terms or guidelines please flag it as inappropriate.
Quick links
- Explore articles by subject
- Guide to authors
- Editorial policies
Sign up for the Nature Briefing newsletter — what matters in science, free to your inbox daily.


An official website of the United States government
The .gov means it’s official. Federal government websites often end in .gov or .mil. Before sharing sensitive information, make sure you’re on a federal government site.
The site is secure. The https:// ensures that you are connecting to the official website and that any information you provide is encrypted and transmitted securely.
- Publications
- Account settings
- My Bibliography
- Collections
- Citation manager
Save citation to file
Email citation, add to collections.
- Create a new collection
- Add to an existing collection
Add to My Bibliography
Your saved search, create a file for external citation management software, your rss feed.
- Search in PubMed
- Search in NLM Catalog
- Add to Search
Most important outcomes research papers on treatment of stable coronary artery disease
- PMID: 23674308
- DOI: 10.1161/CIRCOUTCOMES.113.000310
The following are highlights from the new series, Circulation: Cardiovascular Quality and Outcomes Topic Review. This series will summarize the most important manuscripts, as selected by the Editor, that have been published in the Circulation portfolio. The objective of this series is to provide our readership with a timely, comprehensive selection of important papers that are relevant to the quality and outcomes, and general cardiology audience. The studies included in this article represent the most significant research related to treatment of stable coronary artery disease (CAD).
PubMed Disclaimer
Similar articles
- Most important outcomes research papers on hypertension. Chen R, Dharmarajan K, Kulkarni VT, Punnanithinont N, Gupta A, Bikdeli B, Mody PS, Ranasinghe I. Chen R, et al. Circ Cardiovasc Qual Outcomes. 2013 Jul;6(4):e26-35. doi: 10.1161/CIRCOUTCOMES.113.000424. Epub 2013 Jul 9. Circ Cardiovasc Qual Outcomes. 2013. PMID: 23838106 Free PMC article. Review.
- Most important outcomes research papers on anticoagulation for cardiovascular disease. Bikdeli B, Gupta A, Mody P, Lampropulos JF, Dharmarajan K. Bikdeli B, et al. Circ Cardiovasc Qual Outcomes. 2012 Sep 1;5(5):e65-74. doi: 10.1161/CIRCOUTCOMES.112.968701. Circ Cardiovasc Qual Outcomes. 2012. PMID: 22991352 Review.
- Most important articles on cardiovascular disease among racial and ethnic minorities. Mody P, Gupta A, Bikdeli B, Lampropulos JF, Dharmarajan K. Mody P, et al. Circ Cardiovasc Qual Outcomes. 2012 Jul 1;5(4):e33-41. doi: 10.1161/CIRCOUTCOMES.112.967638. Circ Cardiovasc Qual Outcomes. 2012. PMID: 22811508 Review.
- Most important outcomes research papers in cardiovascular disease in the elderly. Gupta A, Mody P, Bikdeli B, Lampropulos JF, Dharmarajan K. Gupta A, et al. Circ Cardiovasc Qual Outcomes. 2012 May;5(3):e17-26. doi: 10.1161/CIRCOUTCOMES.112.966531. Circ Cardiovasc Qual Outcomes. 2012. PMID: 22592757 Review.
- Most important papers in health costs, cost-effectiveness, and resource utilization. Dharmarajan K, Lampropulos J, Bikdeli B, Mody P, Gupta A. Dharmarajan K, et al. Circ Cardiovasc Qual Outcomes. 2012 Mar 1;5(2):e9-15. doi: 10.1161/CIRCOUTCOMES.112.965830. Circ Cardiovasc Qual Outcomes. 2012. PMID: 22438466 Review.
- The effects of aerobic and anaerobic exercises on circulating soluble-Klotho and IGF-I in young and elderly adults and in CAD patients. Saghiv MS, Sira DB, Goldhammer E, Sagiv M. Saghiv MS, et al. J Circ Biomark. 2017 Sep 28;6:1849454417733388. doi: 10.1177/1849454417733388. eCollection 2017 Jan-Dec. J Circ Biomark. 2017. PMID: 29081845 Free PMC article. Review.
Publication types
- Search in MeSH
Grants and funding
- T32 HL007854/HL/NHLBI NIH HHS/United States
- HL007854/HL/NHLBI NIH HHS/United States
LinkOut - more resources
Full text sources.
- Ovid Technologies, Inc.

Other Literature Sources
- scite Smart Citations
- MedlinePlus Health Information
Miscellaneous
- NCI CPTAC Assay Portal
- Citation Manager
NCBI Literature Resources
MeSH PMC Bookshelf Disclaimer
The PubMed wordmark and PubMed logo are registered trademarks of the U.S. Department of Health and Human Services (HHS). Unauthorized use of these marks is strictly prohibited.
- Twin Cities
- Other Locations
Reflection and perspective are invited, collected, and presented here about the causes, care, control, prevention, epidemiology, and public policy of heart attacks.
Title | Category |
---|---|
Coronary Disease-CVD-Trends | |
References | |
Institutions-Reports | |
Personalities | |
Memoirs | |
Personalities | |
Studies | |
Studies | |
Institutions-Reports | |
Perspective | |
Study Design-Methods | |
Institutions-Reports | |
Institutions-Reports | |
Perspective | |
Institutions-Reports | |
Institutions-Reports | |
Institutions-Reports | |
Institutions-Reports | |
Studies | |
Study Design-Methods | |
Diet-Heart: Hypothesis to Theory to Practice to Policy | |
Other Risk Factors | |
Diet-Heart: Hypothesis to Theory to Practice to Policy | |
Institutions-Reports | |
Institutions-Reports | |
Study Design-Methods | |
Study Design-Methods | |
Diet-Heart: Hypothesis to Theory to Practice to Policy | |
Diet-Heart: Hypothesis to Theory to Practice to Policy | |
Diet-Heart: Hypothesis to Theory to Practice to Policy | |
Diet-Heart: Hypothesis to Theory to Practice to Policy | |
Study Design-Methods | |
Institutions-Reports | |
Perspective | |
Perspective | |
Institutions-Reports | |
Study Design-Methods | |
Other Risk Factors | |
Perspective | |
Stories | |
Institutions-Reports | |
Institutions-Reports | |
Institutions-Reports | |
Institutions-Reports | |
Stories | |
Studies | |
Study Design-Methods | |
Perspective | |
Studies | |
Coronary Disease-CVD-Trends | |
Perspective | |
Perspective | |
Diet-Heart: Hypothesis to Theory to Practice to Policy | |
Perspective | |
Perspective | |
Diet-Heart: Hypothesis to Theory to Practice to Policy | |
Institutions-Reports | |
Diet-Heart: Hypothesis to Theory to Practice to Policy | |
Study Design-Methods | |
Institutions-Reports | |
Studies | |
Diet-Heart: Hypothesis to Theory to Practice to Policy | |
Diet-Heart: Hypothesis to Theory to Practice to Policy | |
Perspective | |
Perspective | |
Institutions-Reports | |
Other Risk Factors | |
Studies | |
Diet-Heart: Hypothesis to Theory to Practice to Policy | |
Other Risk Factors | |
Other Risk Factors | |
Perspective | |
Institutions-Reports | |
Perspective | |
Institutions-Reports | |
Diet-Heart: Hypothesis to Theory to Practice to Policy | |
Perspective | |
Studies | |
Perspective | |
Perspective | |
Perspective | |
Perspective | |
Institutions-Reports | |
Institutions-Reports | |
Institutions-Reports | |
Perspective | |
Institutions-Reports | |
Perspective | |
Perspective | |
Study Design-Methods | |
Perspective | |
Institutions-Reports | |
Institutions-Reports | |
Institutions-Reports | |
Institutions-Reports | |
Study Design-Methods | |
Other Risk Factors | |
Studies | |
Studies | |
Studies | |
Perspective | |
Other Risk Factors | |
Diet-Heart: Hypothesis to Theory to Practice to Policy | |
Institutions-Reports | |
Studies | |
Study Design-Methods | |
Study Design-Methods | |
Study Design-Methods | |
Perspective | |
Study Design-Methods | |
Study Design-Methods | |
Institutions-Reports | |
Study Design-Methods | |
Perspective | |
Perspective | |
Institutions-Reports | |
Study Design-Methods | |
Institutions-Reports | |
Studies | |
Coronary Disease-CVD-Trends | |
Other Risk Factors | |
Other Risk Factors | |
Other Risk Factors | |
Other Risk Factors | |
Diet-Heart: Hypothesis to Theory to Practice to Policy | |
Coronary Disease-CVD-Trends | |
Institutions-Reports | |
Perspective | |
Perspective | |
Perspective | |
Perspective | |
Perspective | |
Diet-Heart: Hypothesis to Theory to Practice to Policy | |
Diet-Heart: Hypothesis to Theory to Practice to Policy | |
Perspective | |
Perspective | |
Institutions-Reports | |
Perspective | |
Diet-Heart: Hypothesis to Theory to Practice to Policy | |
Diet-Heart: Hypothesis to Theory to Practice to Policy | |
Coronary Disease-CVD-Trends | |
Studies | |
Personalities | |
Personalities | |
Coronary Disease-CVD-Trends | |
Perspective | |
Perspective | |
Studies | |
Diet-Heart: Hypothesis to Theory to Practice to Policy | |
Studies | |
Institutions-Reports | |
Perspective | |
Coronary Disease-CVD-Trends | |
Other Risk Factors | |
Other Risk Factors | |
Diet-Heart: Hypothesis to Theory to Practice to Policy | |
Institutions-Reports | |
Diet-Heart: Hypothesis to Theory to Practice to Policy | |
Study Design-Methods | |
- Coronary Disease-CVD-Trends
- Diet-Heart: Hypothesis to Theory to Practice to Policy
- Institutions-Reports
- Other Risk Factors
- Personalities
- Perspective
- Study Design-Methods
Information
- Author Services
Initiatives
You are accessing a machine-readable page. In order to be human-readable, please install an RSS reader.
All articles published by MDPI are made immediately available worldwide under an open access license. No special permission is required to reuse all or part of the article published by MDPI, including figures and tables. For articles published under an open access Creative Common CC BY license, any part of the article may be reused without permission provided that the original article is clearly cited. For more information, please refer to https://www.mdpi.com/openaccess .
Feature papers represent the most advanced research with significant potential for high impact in the field. A Feature Paper should be a substantial original Article that involves several techniques or approaches, provides an outlook for future research directions and describes possible research applications.
Feature papers are submitted upon individual invitation or recommendation by the scientific editors and must receive positive feedback from the reviewers.
Editor’s Choice articles are based on recommendations by the scientific editors of MDPI journals from around the world. Editors select a small number of articles recently published in the journal that they believe will be particularly interesting to readers, or important in the respective research area. The aim is to provide a snapshot of some of the most exciting work published in the various research areas of the journal.
Original Submission Date Received: .
- Active Journals
- Find a Journal
- Proceedings Series
- For Authors
- For Reviewers
- For Editors
- For Librarians
- For Publishers
- For Societies
- For Conference Organizers
- Open Access Policy
- Institutional Open Access Program
- Special Issues Guidelines
- Editorial Process
- Research and Publication Ethics
- Article Processing Charges
- Testimonials
- Preprints.org
- SciProfiles
- Encyclopedia
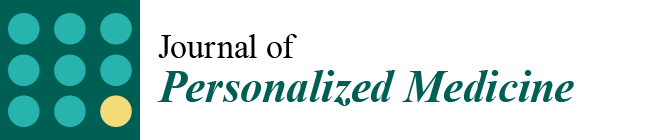
Article Menu
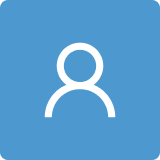
- Subscribe SciFeed
- Recommended Articles
- Google Scholar
- on Google Scholar
- Table of Contents
Find support for a specific problem in the support section of our website.
Please let us know what you think of our products and services.
Visit our dedicated information section to learn more about MDPI.
JSmol Viewer
Future horizons: the potential role of artificial intelligence in cardiology.
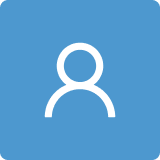
1. Introduction
1.1. terminology, 1.1.1. machine learning (ml), 1.1.2. deep learning (dl), 1.1.3. artificial neural networks (anns), 1.1.4. convolutional neural networks (cnns), 2. materials and methods, 3.1. electrocardiography (ecg), 3.1.1. echocardiography.
- Assessing and monitoring the left ventricular systolic and diastolic function;
- Evaluation of the right ventricular function;
- Evaluation and quantification of the cardiac chamber size;
- Assessing the functional significance of a valvular lesion and the evaluation of the prosthetic valve structure and function;
- Identification of the cardiac source of embolism and the evaluation of the cardiac masses;
- Evaluation of pericardial diseases [ 58 ].
3.1.2. Coronary Angiography
3.1.3. cardiac computed angiography, 3.1.4. computed tomography, 3.1.5. cardiac mri, 4. discussions, 4.1. challenges in ai implementation, 4.2. limitations, 5. conclusions, author contributions, institutional review board statement, informed consent statement, data availability statement, conflicts of interest.
- Flora, G.D.; Nayak, M.K. A Brief Review of Cardiovascular Diseases, Associated Risk Factors and Current Treatment Regimes. Curr. Pharm. Des. 2019 , 25 , 4063–4084. [ Google Scholar ] [ CrossRef ]
- Roth, G.A.; Mensah, G.A.; Johnson, C.O.; Addolorato, G.; Ammirati, E.; Baddour, L.M.; Barengo, N.C.; Beaton, A.Z.; Benjamin, E.J.; Benziger, C.P.; et al. Global Burden of Cardiovascular Diseases and Risk Factors, 1990–2019: Update from the GBD 2019 Study. J. Am. Coll. Cardiol. 2020 , 76 , 2982–3021. [ Google Scholar ] [ CrossRef ]
- Haq, I.U.; Chhatwal, K.; Sanaka, K.; Xu, B. Artificial Intelligence in Cardiovascular Medicine: Current Insights and Future Prospects. Vasc. Health Risk Manag. 2022 , 18 , 517. [ Google Scholar ] [ CrossRef ]
- Amisha; Malik, P.; Pathania, M.; Rathaur, V.K. Overview of artificial intelligence in medicine. J. Fam. Med. Prim. Care 2019 , 8 , 2328. [ Google Scholar ] [ CrossRef ]
- Hamet, P.; Tremblay, J. Artificial intelligence in medicine. Metabolism 2017 , 69S , S36–S40. [ Google Scholar ] [ CrossRef ]
- Poalelungi, D.G.; Musat, C.L.; Fulga, A.; Neagu, M.; Neagu, A.I.; Piraianu, A.I.; Fulga, I. Advancing Patient Care: How Artificial Intelligence Is Transforming Healthcare. J. Pers. Med. 2023 , 13 , 1214. [ Google Scholar ] [ CrossRef ]
- Xu, N.; Yang, D.; Arikawa, K.; Bai, C. Application of artificial intelligence in modern medicine. Clin. eHealth 2023 , 6 , 130–137. [ Google Scholar ] [ CrossRef ]
- Fogel, A.L.; Kvedar, J.C. Artificial intelligence powers digital medicine. NPJ Digit. Med. 2018 , 1 , 5. [ Google Scholar ] [ CrossRef ]
- Zhang, J.; Zhang, J.; Jin, J.; Jiang, X.; Yang, L.; Fan, S.; Zhang, Q.; Chi, M. Artificial intelligence applied in cardiovascular disease: A bibliometric and visual analysis. Front. Cardiovasc. Med. 2024 , 11 , 1323918. [ Google Scholar ] [ CrossRef ]
- Noorbakhsh-Sabet, N.; Zand, R.; Zhang, Y.; Abedi, V. Artificial Intelligence Transforms the Future of Healthcare. Am. J. Med. 2019 , 132 , 795. [ Google Scholar ] [ CrossRef ]
- Bostrom, N. How Long Before Superintelligence? Int. J. Future Stud. 1998 , 2 , 11–30. [ Google Scholar ]
- Sun, K.; Roy, A.; Tobin, J.M. Artificial intelligence and machine learning: Definition of terms and current concepts in critical care research. J. Crit. Care 2024 , 82 , 154792. [ Google Scholar ] [ CrossRef ]
- Chakraborty, C.; Bhattacharya, M.; Pal, S.; Lee, S.-S. From machine learning to deep learning: Advances of the recent data-driven paradigm shift in medicine and healthcare. Curr. Res. Biotechnol. 2024 , 7 , 100164. [ Google Scholar ] [ CrossRef ]
- Botvinick, M.; Ritter, S.; Wang, J.X.; Kurth-Nelson, Z.; Blundell, C.; Hassabis, D. Reinforcement Learning, Fast and Slow. Trends Cogn. Sci. 2019 , 23 , 408–422. [ Google Scholar ] [ CrossRef ]
- Jakhar, D.; Kaur, I. Artificial intelligence, machine learning and deep learning: Definitions and differences. Clin. Exp. Dermatol. 2020 , 45 , 131–132. [ Google Scholar ] [ CrossRef ]
- Piccialli, F.; Di Somma, V.; Giampaolo, F.; Cuomo, S.; Fortino, G. A survey on deep learning in medicine: Why, how and when? Inf. Fusion. 2021 , 66 , 111–137. [ Google Scholar ] [ CrossRef ]
- Dongare, A.D.; Kharde, R.R.; Kachare, A.D. Introduction to Artificial Neural Network. Certif. Int. J. Eng. Innov. Technol. (IJEIT) 2008 , 9001 , 2277–3754. [ Google Scholar ]
- Micheli-Tzanakou, E. Artificial Neural Networks: Definitions, Methods, Applications. In Supervised and Unsupervised Pattern Recognition ; CRC Press: Boca Raton, FL, USA, 2017; pp. 61–78. [ Google Scholar ]
- Zhou, S.K.; Rueckert, D.; Fichtinger, G. (Eds.) Handbook of Medical Image Computing and Computer Assisted Intervention ; Academic Press: Cambridge, MA, USA, 2020. [ Google Scholar ]
- Berezsky, O.; Liashchynskyi, P.; Pitsun, O.; Izonin, I. Synthesis of Convolutional Neural Network architectures for biomedical image classification. Biomed. Signal Process Control 2024 , 95 , 106325. [ Google Scholar ] [ CrossRef ]
- Piraianu, A.I.; Fulga, A.; Musat, C.L.; Ciobotaru, O.R.; Poalelungi, D.G.; Stamate, E.; Ciobotaru, O.; Fulga, I. Enhancing the Evidence with Algorithms: How Artificial Intelligence Is Transforming Forensic Medicine. Diagnostics 2023 , 13 , 2992. [ Google Scholar ] [ CrossRef ]
- Herman, R.; Meyers, H.P.; Smith, S.W.; Bertolone, D.T.; Leone, A.; Bermpeis, K.; Viscusi, M.M.; Belmonte, M.; Demolder, A.; Boza, V.; et al. International evaluation of an artificial intelligence-powered electrocardiogram model detecting acute coronary occlusion myocardial infarction. Eur. Heart J. Digit. Health 2023 , 5 , 123–133. [ Google Scholar ] [ CrossRef ]
- Nogimori, Y.; Sato, K.; Takamizawa, K.; Ogawa, Y.; Tanaka, Y.; Shiraga, K.; Masuda, H.; Matsui, H.; Kato, M.; Daimon, M.; et al. Prediction of adverse cardiovascular events in children using artificial intelligence-based electrocardiogram. Int. J. Cardiol. 2024 , 406 , 132019. [ Google Scholar ] [ CrossRef ]
- Hillis, J.; Bizzo, B.C.; Mercaldo, S.; Ghatak, A.; Macdonald, A.; Halle, M.; Schultz, A.; L’Italien, E.; Tam, V.; Awad, A.; et al. Detection of Hypertrophic Cardiomyopathy on Electrocardiogram Using Artificial Intelligence. J. Am. Coll. Cardiol. 2024 , 83 , 2609. [ Google Scholar ] [ CrossRef ]
- Haimovich, J.S.; Diamant, N.; Khurshid, S.; Di Achille, P.; Reeder, C.; Friedman, S.; Singh, P.; Spurlock, W.; Ellinor, P.T.; Philippakis, A.; et al. Artificial intelligence–enabled classification of hypertrophic heart diseases using electrocardiograms. Cardiovasc. Digit. Health J. 2023 , 4 , 48–59. [ Google Scholar ] [ CrossRef ]
- Harmon, D.M.; Mangold, K.; Baez Suarez, A.; Scott, C.G.; Murphree, D.H.; Malik, A.; Attia, Z.I.; Lopez-Jimenez, F.; Friedman, P.A.; Dispenzieri, A.; et al. Postdevelopment Performance and Validation of the Artificial Intelligence-Enhanced Electrocardiogram for Detection of Cardiac Amyloidosis. JACC Adv. 2023 , 2 , 100612. [ Google Scholar ] [ CrossRef ]
- Butler, L.; Karabayir, I.; Kitzman, D.W.; Alonso, A.; Tison, G.H.; Chen, L.Y.; Chang, P.P.; Clifford, G.; Soliman, E.Z.; Akbilgic, O. A generalizable electrocardiogram-based artificial intelligence model for 10-year heart failure risk prediction. Cardiovasc. Digit. Health J. 2023 , 4 , 183–190. [ Google Scholar ] [ CrossRef ]
- Awasthi, S.; Sachdeva, N.; Gupta, Y.; Anto, A.G.; Asfahan, S.; Abbou, R.; Bade, S.; Sood, S.; Hegstrom, L.; Vellanki, N.; et al. Identification and risk stratification of coronary disease by artificial intelligence-enabled ECG. eClinicalMedicine 2023 , 65 , 102259. [ Google Scholar ] [ CrossRef ]
- Lee, Y.H.; Hsieh, M.T.; Chang, C.C.; Tsai, Y.L.; Chou, R.H.; Lu, H.H.S.; Huang, P.-H. Improving detection of obstructive coronary artery disease with an artificial intelligence-enabled electrocardiogram algorithm. Atherosclerosis 2023 , 381 , 117238. [ Google Scholar ] [ CrossRef ]
- Valente Silva, B.; Marques, J.; Nobre Menezes, M.; Oliveira, A.L.; Pinto, F.J. Artificial intelligence-based diagnosis of acute pulmonary embolism: Development of a machine learning model using 12-lead electrocardiogram. Rev. Port. Cardiol. 2023 , 42 , 643–651. [ Google Scholar ] [ CrossRef ]
- Sau, A.; Ibrahim, S.; Kramer, D.B.; Waks, J.W.; Qureshi, N.; Koa-Wing, M.; Keene, D.; Malcolme-Lawes, L.; Lefroy, D.C.; Linton, N.W.; et al. Artificial intelligence–enabled electrocardiogram to distinguish atrioventricular re-entrant tachycardia from atrioventricular nodal re-entrant tachycardia. Cardiovasc. Digit. Health J. 2023 , 4 , 60–67. [ Google Scholar ] [ CrossRef ]
- Shimojo, M.; Inden, Y.; Yanagisawa, S.; Suzuki, N.; Tsurumi, N.; Watanabe, R.; Nakagomi, T.; Okajima, T.; Suga, K.; Tsuji, Y.; et al. A novel practical algorithm using machine learning to differentiate outflow tract ventricular arrhythmia origins. J. Cardiovasc. Electrophysiol. 2023 , 34 , 627–637. [ Google Scholar ] [ CrossRef ]
- Shiokawa, N.; Izumo, M.; Shimamura, T.; Kurosaka, Y.; Sato, Y.; Okamura, T.; Akashi, Y.J. Accuracy and Efficacy of Artificial Intelligence-Derived Automatic Measurements of Transthoracic Echocardiography in Routine Clinical Practice. J. Clin. Med. 2024 , 13 , 1861. [ Google Scholar ] [ CrossRef ]
- Sveric, K.M.; Ulbrich, S.; Dindane, Z.; Winkler, A.; Botan, R.; Mierke, J.; Trausch, A.; Heidrich, F.; Linke, A. Improved assessment of left ventricular ejection fraction using artificial intelligence in echocardiography: A comparative analysis with cardiac magnetic resonance imaging. Int. J. Cardiol. 2024 , 394 , 131383. [ Google Scholar ] [ CrossRef ]
- Slivnick, J.A.; Gessert, N.T.; Cotella, J.I.; Oliveira, L.; Pezzotti, N.; Eslami, P.; Sadeghi, A.; Wehle, S.; Prabhu, D.; Waechter-Stehle, I.; et al. Echocardiographic Detection of Regional Wall Motion Abnormalities Using Artificial Intelligence Compared to Human Readers. J. Am. Soc. Echocardiogr. 2024. [ CrossRef ]
- Kampaktsis, P.N.; Bohoran, T.A.; Lebehn, M.; McLaughlin, L.; Leb, J.; Liu, Z.; Moustakidis, S.; Siouras, A.; Singh, A.; Hahn, R.T.; et al. An attention-based deep learning method for right ventricular quantification using 2D echocardiography: Feasibility and accuracy. Echocardiography 2024 , 41 , e15719. [ Google Scholar ] [ CrossRef ]
- Murayama, M.; Sugimori, H.; Yoshimura, T.; Kaga, S.; Shima, H.; Tsuneta, S.; Mukai, A.; Nagai, Y.; Yokoyama, S.; Nishino, H.; et al. Deep learning to assess right ventricular ejection fraction from two-dimensional echocardiograms in precapillary pulmonary hypertension. Echocardiography 2024 , 41 , e15812. [ Google Scholar ] [ CrossRef ]
- Hsia, B.C.; Lai, A.; Singh, S.; Samtani, R.; Bienstock, S.; Liao, S.; Stern, E.; LaRocca, G.; Sanz, J.; Lerakis, S.; et al. Validation of American Society of Echocardiography Guideline-Recommended Parameters of Right Ventricular Dysfunction Using Artificial Intelligence Compared with Cardiac Magnetic Resonance Imaging. J. Am. Soc. Echocardiogr. 2023 , 36 , 967–977. [ Google Scholar ] [ CrossRef ]
- Anand, V.; Weston, A.D.; Scott, C.G.; Kane, G.C.; Pellikka, P.A.; Carter, R.E. Machine Learning for Diagnosis of Pulmonary Hypertension by Echocardiography. Mayo Clin. Proc. 2024 , 99 , 260–270. [ Google Scholar ] [ CrossRef ]
- Oikonomou, E.K.; Holste, G.; Yuan, N.; Coppi, A.; McNamara, R.L.; Haynes, N.A.; Vora, A.N.; Velazquez, E.J.; Li, F.; Menon, V.; et al. A Multimodal Video-Based AI Biomarker for Aortic Stenosis Development and Progression. JAMA Cardiol. 2024 , 9 , 534–544. [ Google Scholar ] [ CrossRef ]
- Krishna, H.; Desai, K.; Slostad, B.; Bhayani, S.; Arnold, J.H.; Ouwerkerk, W.; Hummel, Y.; Lam, C.S.; Ezekowitz, J.; Frost, M.; et al. Fully Automated Artificial Intelligence Assessment of Aortic Stenosis by Echocardiography. J. Am. Soc. Echocardiogr. 2023 , 36 , 769–777. [ Google Scholar ] [ CrossRef ]
- Guo, Y.; Xia, C.; Zhong, Y.; Wei, Y.; Zhu, H.; Ma, J.; Li, G.; Meng, X.; Yang, C.; Wang, X.; et al. Machine learning-enhanced echocardiography for screening coronary artery disease. Biomed. Eng. Online 2023 , 22 , 44. [ Google Scholar ] [ CrossRef ]
- Molenaar, M.A.; Bouma, B.J.; Asselbergs, F.W.; Verouden, N.J.; Selder, J.L.; Chamuleau, S.A.J.; Schuuring, M.J. Explainable machine learning using echocardiography to improve risk prediction in patients with chronic coronary syndrome. Eur. Heart J. Digit. Health 2024 , 5 , 170–182. [ Google Scholar ] [ CrossRef ]
- Lu, N.; Vaseli, H.; Mahdavi, M.; Taheri Dezaki, F.; Luong, C.; Yeung, D.; Gin, K.; Tsang, M.; Nair, P.; Jue, J.; et al. Automated Atrial Fibrillation Diagnosis by Echocardiography without ECG: Accuracy and Applications of a New Deep Learning Approach. Diseases 2024 , 12 , 35. [ Google Scholar ] [ CrossRef ]
- Brown, K.; Roshanitabrizi, P.; Rwebembera, J.; Okello, E.; Beaton, A.; Linguraru, M.G.; Sable, C.A. Using Artificial Intelligence for Rheumatic Heart Disease Detection by Echocardiography: Focus on Mitral Regurgitation. J. Am. Heart Assoc. 2024 , 13 , e031257. [ Google Scholar ] [ CrossRef ]
- Steffner, K.R.; Christensen, M.; Gill, G.; Bowdish, M.; Rhee, J.; Kumaresan, A.; He, B.; Zou, J.; Ouyang, D. Deep learning for transesophageal echocardiography view classification. Sci. Rep. 2024 , 14 , 11. [ Google Scholar ] [ CrossRef ]
- In Kim, Y.; Roh, J.H.; Kweon, J.; Kwon, H.; Chae, J.; Park, K.; Lee, J.-H.; Jeong, J.-O.; Kang, D.-Y.; Lee, P.H.; et al. Artificial intelligence-based quantitative coronary angiography of major vessels using deep-learning. Int. J. Cardiol. 2024 , 405 , 131945. [ Google Scholar ] [ CrossRef ]
- Rinehart, S.; Raible, S.J.; Ng, N.; Mullen, S.; Huey, W.; Rogers, C.; Pursnani, A. Utility of Artificial Intelligence Plaque Quantification: Results of the DECODE Study. J. Soc. Cardiovasc. Angiogr. Interv. 2024 , 3 , 101296. [ Google Scholar ] [ CrossRef ]
- Omori, H.; Matsuo, H.; Fujimoto, S.; Sobue, Y.; Nozaki, Y.; Nakazawa, G.; Takahashi, K.; Osawa, K.; Okubo, R.; Kaneko, U.; et al. Determination of lipid-rich plaques by artificial intelligence-enabled quantitative computed tomography using near-infrared spectroscopy as reference. Atherosclerosis 2023 , 386 , 117363. [ Google Scholar ] [ CrossRef ]
- Toggweiler, S.; Wyler von Ballmoos, M.C.; Moccetti, F.; Douverny, A.; Wolfrum, M.; Imamoglu, Z.; Mohler, A.; Gülan, U.; Kim, W.-K. A fully automated artificial intelligence-driven software for planning of transcatheter aortic valve replacement. Cardiovasc. Revascularization Med. 2024. [ CrossRef ]
- Salehi, M.; Maiter, A.; Strickland, S.; Aldabbagh, Z.; Karunasaagarar, K.; Thomas, R.; Lopez-Dee, T.; Capener, D.; Dwivedi, K.; Sharkey, M.; et al. Clinical assessment of an AI tool for measuring biventricular parameters on cardiac MR. Front. Cardiovasc. Med. 2024 , 11 , 1279298. [ Google Scholar ] [ CrossRef ]
- Ghanbari, F.; Joyce, T.; Lorenzoni, V.; Guaricci, A.I.; Pavon, A.G.; Fusini, L.; Andreini, D.; Rabbat, M.G.; Aquaro, G.D.; Abete, R.; et al. AI Cardiac MRI Scar Analysis Aids Prediction of Major Arrhythmic Events in the Multicenter DERIVATE Registry. Radiology 2023 , 307 , e222239. [ Google Scholar ] [ CrossRef ]
- Kaplan Berkaya, S.; Uysal, A.K.; Sora Gunal, E.; Ergin, S.; Gunal, S.; Gulmezoglu, M.B. A survey on ECG analysis. Biomed. Signal Process Control 2018 , 43 , 216–235. [ Google Scholar ] [ CrossRef ]
- Ogah, O.S.; Oladapo, O.O.; Adebiyi, A.A.; Adebayo, A.K.; Aje, A.; Ojji, D.B. Electrocardiographic left ventricular hypertrophy with strain pattern: Prevalence, mechanisms and prognostic implications. Cardiovasc. J. Afr. 2008 , 19 , 39. [ Google Scholar ]
- Bornstein, A.B.; Rao, S.S.; Marwaha, K. Left Ventricular Hypertrophy. [Updated 8 August 2023]. In StatPearls [Internet] ; StatPearls Publishing: Treasure Island, FL, USA, 2024. Available online: https://www.ncbi.nlm.nih.gov/books/NBK557534/ (accessed on 11 June 2024).
- Savarese, G.; Becher, P.M.; Lund, L.H.; Seferovic, P.; Rosano, G.M.C.; Coats, A.J.S. Global burden of heart failure: A comprehensive and updated review of epidemiology. Cardiovasc. Res. 2023 , 118 , 3272–3287. [ Google Scholar ] [ CrossRef ]
- Ojha, M.K.; Wadhwani, S.; Wadhwani, A.K.; Shukla, A. Automatic detection of arrhythmias from an ECG signal using an auto-encoder and SVM classifier. Phys. Eng. Sci. Med. 2022 , 45 , 665–674. [ Google Scholar ] [ CrossRef ]
- Ahmed, I.; Sasikumar, N. Echocardiography Imaging Techniques. [Updated 30 July 2023]. In StatPearls [Internet] ; StatPearls Publishing: Treasure Island, FL, USA, 2024. Available online: https://www.ncbi.nlm.nih.gov/books/NBK572130/ (accessed on 11 June 2024).
- Vidal-Perez, R.; Grapsa, J.; Bouzas-Mosquera, A.; Fontes-Carvalho, R.; Vazquez-Rodriguez, J.M. Current role and future perspectives of artificial intelligence in echocardiography. World J. Cardiol. 2023 , 15 , 284. [ Google Scholar ] [ CrossRef ]
- Carabello, B.A.; Paulus, W.J. Aortic stenosis. Lancet 2009 , 373 , 956–966. [ Google Scholar ] [ CrossRef ]
- Ring, L.; Shah, B.N.; Bhattacharyya, S.; Harkness, A.; Belham, M.; Oxborough, D.; Pearce, K.; Rana, B.S.; Augustine, D.X.; Robinson, S.; et al. Echocardiographic assessment of aortic stenosis: A practical guideline from the British Society of Echocardiography. Echo Res. Pract. 2021 , 8 , G19. [ Google Scholar ] [ CrossRef ]
- Stamate, E.; Piraianu, A.-I.; Ciobotaru, O.R.; Crassas, R.; Duca, O.; Fulga, A.; Grigore, I.; Vintila, V.; Fulga, I.; Ciobotaru, O.C. Revolutionizing Cardiology through Artificial Intelligence—Big Data from Proactive Prevention to Precise Diagnostics and Cutting-Edge Treatment—A Comprehensive Review of the Past 5 Years. Diagnostics 2024 , 14 , 1103. [ Google Scholar ] [ CrossRef ]
- Suzuki, N.; Asano, T.; Nakazawa, G.; Aoki, J.; Tanabe, K.; Hibi, K.; Ikari, Y.; Kozuma, K. Clinical expert consensus document on quantitative coronary angiography from the Japanese Association of Cardiovascular Intervention and Therapeutics. Cardiovasc. Interv. Ther. 2020 , 35 , 105. [ Google Scholar ] [ CrossRef ]
- Ramjattan, N.A.; Lala, V.; Kousa, O.; Shams, P.; Makaryus, A.N. Coronary CT Angiography. [Updated 19 January 2024]. In StatPearls [Internet] ; StatPearls Publishing: Treasure Island, FL, USA, 2024. Available online: https://www.ncbi.nlm.nih.gov/books/NBK470279/ (accessed on 11 June 2024).
- Blanke, P.; Schoepf, U.J.; Leipsic, J.A. CT Transcatheter Aortic Valve Replacement. Radiology 2013 , 269 , 650–669. [ Google Scholar ] [ CrossRef ]
- Tseng, W.Y.I.; Su, M.Y.M.; Tseng, Y.H.E. Introduction to Cardiovascular Magnetic Resonance: Technical Principles and Clinical Applications. Acta Cardiol. Sin. 2016 , 32 , 129. [ Google Scholar ] [ CrossRef ]
- He, J.; Baxter, S.L.; Xu, J.; Xu, J.; Zhou, X.; Zhang, K. The practical implementation of artificial intelligence technologies in medicine. Nat. Med. 2019 , 25 , 30. [ Google Scholar ] [ CrossRef ]
- Kolla, L.; Parikh, R.B. Uses and limitations of artificial intelligence for oncology. Cancer 2024 , 130 , 2101–2107. [ Google Scholar ] [ CrossRef ]
- Oh, S.; Kim, J.H.; Choi, S.W.; Lee, H.J.; Hong, J.; Kwon, S.H. Physician Confidence in Artificial Intelligence: An Online Mobile Survey. J. Med. Internet Res. 2019 , 21 , e12422. [ Google Scholar ] [ CrossRef ]
- Patel, V.; Shah, M. Artificial intelligence and machine learning in drug discovery and development. Intell. Med. 2022 , 2 , 134–140. [ Google Scholar ] [ CrossRef ]
- Busnatu, Ș.; Niculescu, A.G.; Bolocan, A.; Petrescu, G.E.D.; Păduraru, D.N.; Năstasă, I.; Lupușoru, M.; Geantă, M.; Andronic, O.; Grumezescu, A.M.; et al. Clinical Applications of Artificial Intelligence—An Updated Overview. J. Clin. Med. 2022 , 11 , 2265. [ Google Scholar ] [ CrossRef ]
- Chan, B. Black-box assisted medical decisions: AI power vs. ethical physician care. Med. Health Care Philos. 2023 , 26 , 285. [ Google Scholar ] [ CrossRef ]
- Poon, A.I.F.; Sung, J.J.Y. Opening the black box of AI-Medicine. J. Gastroenterol. Hepatol. 2021 , 36 , 581–584. [ Google Scholar ] [ CrossRef ]
- Durán, J.M.; Jongsma, K.R. Who is afraid of black box algorithms? On the epistemological and ethical basis of trust in medical AI. J. Med. Ethics 2021 , 47 , 329–335. [ Google Scholar ] [ CrossRef ]
- Gerke, S.; Minssen, T.; Cohen, G. Ethical and legal challenges of artificial intelligence-driven healthcare. Artif. Intell. Healthc. 2020 , 295–336. [ Google Scholar ] [ CrossRef ]
- Tang, L.; Li, J.; Fantus, S. Medical artificial intelligence ethics: A systematic review of empirical studies. Digit. Health 2023 , 9 . [ Google Scholar ] [ CrossRef ]
- Sana, M.K.; Hussain, Z.M.; Maqsood, M.H.; Shah, P.A. Artificial intelligence in celiac disease. Comput. Biol. Med. 2020 , 125 , 103996. [ Google Scholar ] [ CrossRef ]
- Vinny, P.W.; Vishnu, V.Y.; Padma Srivastava, M.V. Artificial Intelligence shaping the future of neurology practice. Med. J. Armed Forces India 2021 , 77 , 276–282. [ Google Scholar ] [ CrossRef ]
Click here to enlarge figure
Paraclinical Investigation | Author | Year of Study | Application |
---|---|---|---|
Herman R. [ ] | 2024 | Detection of occlusion myocardial infarction. | |
Nogimori Y. [ ] | 2024 | ECG-derived CNN is a novel marker of HF in children with different prognostic potential from BNP. | |
Hillis J. [ ] | 2024 | Identification of hypertrophic cardiomyopathy on a 12 lead ECG. Classification of hypertrophic cardiomyopathy, cardiac amyloidosis, and echocardiographic LVH. Detection of cardiac amyloidosis. | |
Haimovich J. [ ] | 2023 | ||
Harmon D. [ ] | 2023 | ||
Butler L. [ ] | 2023 | Early Heart Failure prediction using ECG-AI models. | |
Awasthi S. [ ] | 2023 | Assessing the risk stratification of CAD. | |
Lee Y. [ ] | 2023 | ||
Valente Silva B. [ ] | 2023 | Diagnosis of Acute Pulmonary Embolism. | |
Sau A. [ ] | 2023 | Distinguish AVRT from AVNRT. | |
Shimojo M. [ ] | 2024 | Identification of the origin of outflow tract ventricular arrhythmia. | |
Shiokawa N [ ] | 2024 | Automatic measurements of transthoracic echocardiography. | |
Sveric K. [ ] | 2024 | Calculation of left ventricular ejection fraction. | |
Slivnick J. [ ] | 2024 | Detection of Regional Wall Motion Abnormalities. | |
Kampaktsis P. [ ] | 2024 | Quantification of the right ventricle. | |
Murayama M [ ] | 2024 | Measuring the right ventricle ejection fraction. | |
Hsia B. [ ] | 2023 | Assessing the parameters of right ventricular dysfunction. | |
Anand V. [ ] | 2024 | Diagnosis of pulmonary hypertension. | |
Oikonomu E. [ ] | 2024 | A video-based biomarker for detection of severe aortic stenosis. | |
Krinsha H. [ ] | 2023 | Assessment of aortic stenosis. | |
Guo Y. [ ] | 2023 | Detection of coronary artery disease. | |
Molenaar M. [ ] | 2024 | Identifying high-risk chronic coronary syndrome patients. | |
Lu N. [ ] | 2024 | Detection of atrial fibrillation on echocardiography without ECG. | |
Brown K. [ ] | 2024 | Detecting rheumatic heart disease. | |
Steffner K. [ ] | 2024 | Identification of standardized Transesophageal Echocardiography views. | |
In Kim Y. [ ] | 2024 | Quantitative assessment of coronary lesions. | |
Rinehart S. [ ] | 2024 | Plaque quantification. | |
Omori H. [ ] | 2023 | Morphology of coronary plaque. | |
Toggweiler S. [ ] | 2024 | Planning of transcatheter aortic valve replacements. | |
Salehi M. [ ] | 2024 | Automated segmentation of both ventricles on CMR by an automatic tool. | |
Ghanbari F. [ ] | 2023 | Prediction of major arrhythmic events by analyzing cardiac MRI scar. |
The statements, opinions and data contained in all publications are solely those of the individual author(s) and contributor(s) and not of MDPI and/or the editor(s). MDPI and/or the editor(s) disclaim responsibility for any injury to people or property resulting from any ideas, methods, instructions or products referred to in the content. |
Share and Cite
Patrascanu, O.S.; Tutunaru, D.; Musat, C.L.; Dragostin, O.M.; Fulga, A.; Nechita, L.; Ciubara, A.B.; Piraianu, A.I.; Stamate, E.; Poalelungi, D.G.; et al. Future Horizons: The Potential Role of Artificial Intelligence in Cardiology. J. Pers. Med. 2024 , 14 , 656. https://doi.org/10.3390/jpm14060656
Patrascanu OS, Tutunaru D, Musat CL, Dragostin OM, Fulga A, Nechita L, Ciubara AB, Piraianu AI, Stamate E, Poalelungi DG, et al. Future Horizons: The Potential Role of Artificial Intelligence in Cardiology. Journal of Personalized Medicine . 2024; 14(6):656. https://doi.org/10.3390/jpm14060656
Patrascanu, Octavian Stefan, Dana Tutunaru, Carmina Liana Musat, Oana Maria Dragostin, Ana Fulga, Luiza Nechita, Alexandru Bogdan Ciubara, Alin Ionut Piraianu, Elena Stamate, Diana Gina Poalelungi, and et al. 2024. "Future Horizons: The Potential Role of Artificial Intelligence in Cardiology" Journal of Personalized Medicine 14, no. 6: 656. https://doi.org/10.3390/jpm14060656
Article Metrics
Article access statistics, further information, mdpi initiatives, follow mdpi.
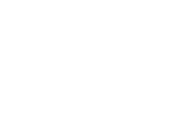
Subscribe to receive issue release notifications and newsletters from MDPI journals
Efficacy and Safety of Salvia Miltiorrhiza Bunge Extract on Coronary Heart Disease Comorbidity with Heart Failure: A Systematic Review and Meta-Analysis
35 Pages Posted: 18 Jun 2024 Publication Status: Under Review
Jiangxi University of Traditional Chinese Medicine
Shanghai University of Traditional Chinese Medicine
Shanghai University of Traditional Chinese Medicine - Department of Dermatology
Mei-jiao Mao
Ying-li zhao, wang-kang niku, shuai zhang.
Background: Coronary heart disease (CHD) is a key cause of heart failure (HF). Many Salvia miltiorrhiza Bunge Extract (SmE) preparations have emerged. However, it is still unclear the clinical efficacy and safety in the treatment of CHD comorbidity with HF. Purpose: To investigate the efficacy and safety of SmE in patients with CHD comorbidity with HF. Study Design: After searching the database and selecting relevant literature, a meta-analysis was conducted on the relevant outcomes to evaluate the efficacy and safety of SmE agents. Methods: Randomized controlled trials (RCTs) of SmE in the treatment of CHD comorbidity with HF were searched in six databases from their establishment to December 2023. Review manager 5.4.1 was utilized for analysis. Subgroup, sensitivity analyses were done to assess heterogeneity. Publication bias was evaluated by STATA SE 15.1. This study was registered with PROSPERO, number CRD42023449562. Results: Incorporating 19 studies in this meta-analysis, it has demonstrated significant improvement in left ventricular ejection fraction (LVEF) and 6-minute walking test (6MWT) and reduction in left ventricular end-diastolic diameter (LVEDD) and Brain natriuretic peptide (BNP) for both the sodium tanshinone IIA sulfonate (STS) injection and the Salvianolate injection (SI) groups. Lee's heart failure score and cardiac fatty acid binding protein (H-FABP) were significantly decreased in the SI group. However, there is no statistical significance in N-terminal pro-B-type natriuretic peptide (NT-pro-BNP) in the SI group. Adverse events in the SmE recipients were similar to controls. Conclusions: SmE agents combined with conventional medicine shows superior clinical efficacy in CHD comorbidity with HF.
Note: Funding Information: This work was supported by the fourth batch of national excellent talents in Chinese medicine project (No. 18401971500) and the Third Batch of Excellent Young Talents Clinical Competency Enhancement Program of Longhua Hospital (No. RC-2020-01-12). Conflict of Interests: None to declare.
Keywords: Coronary Heart Disease, Heart Failure, Salvia Miltiorrhiza Bunge Extract, Meta-Analysis, Systematic Review
Suggested Citation: Suggested Citation
Jiangxi University of Traditional Chinese Medicine ( email )
Nanchang China
Shanghai University of Traditional Chinese Medicine ( email )
Shanghai university of traditional chinese medicine - department of dermatology ( email ).
No. 1200, Cailun Road Shanghai, 201203 China
Shuai Zhang (Contact Author)
Do you have a job opening that you would like to promote on ssrn, paper statistics, related ejournals, cardiovascular medicine ejournal.
Subscribe to this fee journal for more curated articles on this topic

An official website of the United States government
Official websites use .gov A .gov website belongs to an official government organization in the United States.
Secure .gov websites use HTTPS A lock ( ) or https:// means you’ve safely connected to the .gov website. Share sensitive information only on official, secure websites.
No Permission
You do not have permission to view this page.
- NHLBI Facebook profile
- NHLBI Twitter profile
- NHLBI Youtube profile
- NHLBI Linkedin profile
- Search Menu
- Sign in through your institution
- Advance Articles
- Editor's Choice
- Braunwald's Corner
- ESC Guidelines
- EHJ Dialogues
- Issue @ a Glance Podcasts
- CardioPulse
- Weekly Journal Scan
- European Heart Journal Supplements
- Year in Cardiovascular Medicine
- Asia in EHJ
- Most Cited Articles
- ESC Content Collections
- Author Guidelines
- Submission Site
- Why publish with EHJ?
- Open Access Options
- Submit from medRxiv or bioRxiv
- Author Resources
- Self-Archiving Policy
- Read & Publish
- Advertising and Corporate Services
- Advertising
- Reprints and ePrints
- Sponsored Supplements
- Journals Career Network
- About European Heart Journal
- Editorial Board
- About the European Society of Cardiology
- ESC Publications
- War in Ukraine
- ESC Membership
- ESC Journals App
- Developing Countries Initiative
- Dispatch Dates
- Terms and Conditions
- Journals on Oxford Academic
- Books on Oxford Academic
Article Contents
Declarations, therapeutic potentials of sound waves in cardiovascular medicine: further important evidence.

The opinions expressed in this article are not necessarily those of the Editors of the European Heart Journal or of the European Society of Cardiology.
- Article contents
- Figures & tables
- Supplementary Data
Hiroaki Shimokawa, Therapeutic potentials of sound waves in cardiovascular medicine: further important evidence, European Heart Journal , 2024;, ehae253, https://doi.org/10.1093/eurheartj/ehae253
- Permissions Icon Permissions

Therapeutic potentials of sound wave therapies with shock wave (SW) and low-intensity pulsed ultrasound (LIPUS). The SW and LIPUS therapies could activate self-healing abilities depending on disease-specific conditions, including angiogenesis in ischaemic tissue, lymph-angiogenesis in oedematous tissue, neurogenesis in damaged nervous tissue, and others. These sound therapies appear to have broad indications for the brain, heart, kidney and peripheral arteries in cardiovascular medicine. AMI, acute myocardial infarction; HFpEF, heart failure with preserved LV ejection fraction.
This editorial refers to ‘Cardiac shockwave therapy in addition to coronary bypass surgery improves myocardial function in ischaemic heart failure: the CAST-HF trial’, by J. Holfeld et al., https://doi.org/10.1093/eurheartj/ehae341 .
In our body, many potential self-healing abilities remain and could be activated in response to exercise and other adequate physical stimuli. Sound waves, such as low-intensity shock wave (SW) and low-intensity pulsed ultrasound (LIPUS), provide such adequate mechanical stimuli to promote various self-healing responses through the so-called ‘mechanotransduction’ mechanism. 1–3 Indeed, it is interesting to note that sound-wave therapies with SW or LIPUS induce disease-specific regenerative responses, including angiogenesis in ischaemic tissue, lymph-angiogenesis in oedematous tissue, neurogenesis in damaged nervous tissues, and others through improvement of microvascular dysfunction ( Graphical Abstract ). 1 This therapeutic approach to stimulate endogenous self-healing abilities with sound waves appears to be feasible and promising in terms of efficacy, safety and medical cost as compared with the molecular biological approach with exogenous materials, such as genes or cells. 1 Interestingly, SW and LIPUS share the same intracellular molecular mechanisms for mechanotransduction, involving the β1-integrin/caveolin-1 complex in the endothelial caveolae followed by up-regulation of endothelial nitric oxide synthase (eNOS). 4 , 5 Angiogenic and thus anti-ischaemic effects of low-energy SW were first described in a porcine model of chronic myocardial ischaemia 6 and then in patients with severe coronary artery disease. 7 , 8
In this issue of the Journal, 9 Holfeld et al . added important evidence to the literature of therapeutic potentials of sound waves in cardiovascular medicine. In their single-blind, parallel-group, sham-controlled trial (CAST-HF), patients with left ventricular ejection fraction (LVEF) < 40% requiring surgical revascularization were enrolled. The patients were randomly assigned to undergo direct cardiac SW therapy ( n = 30) or sham treatment ( n = 28) in addition to coronary artery bypass grafting (CABG) surgery and were followed up for one year. The results showed that improvement in LVEF measured by cardiac MRI (primary endpoint) was greater in the SW group than in the sham treatment group (△ from baseline to 360 days: SW 11.3% vs. Sham 6.3%, P = .0146), which was also the case for improvement in 6-min walk distance (△ from baseline to 360 days: SW 127.5 m vs. Sham 43.6 m, P = .028), whereas no difference was noted between the two groups for Minnesota Living with Heart Failure Questionnaire (SW 11.0 point vs. Sham 17.3 point, P = .15). In contrast to the previous studies with extracorporeal cardiac SW therapy, 7 , 8 the authors employed direct SW therapy during CABG surgery after complete revascularization, while still on cardiopulmonary bypass. 9 The SW irradiation conditions were also different; in this study by Holfeld et al ., 300 impulses for coronary supply territory for all three coronary territories due to three vessel disease, intensity of 0.38 mJ/mm 2 and a frequency of 3 Hz, 9 whereas in the previous studies, 200 shots/spot for 20∼40 spots (depending on the extent of ischaemic myocardium), intensity of 0.09 mJ/mm 2 and in a manner of heart rate synchronization. 7 , 8 Nonetheless, the impact of the SW therapy on cardiac function in the study by Holfeld et al . is meaningful in daily practice, suggesting the usefulness of the routine use of the SW therapy during CABG surgery for patients with ischaemic heart failure. It is also interesting to note that the authors demonstrated the involvement of innate immune receptor Toll-like receptor 3 (TLR3) pathway in the effects of the SW therapy. 10 This suggests the cross-talk between the TLR3 and the eNOS pathways in the effects of the SW therapy. In addition to the discussion by the authors on the study limitations, 9 several points remain to be examined. First, the potential usefulness of additional extracorporeal cardiac SW therapy after this intraoperative SW therapy remains to be examined in future studies. Second, it remains to be elucidated whether the intraoperative therapy improves cardiac diastolic function in addition to systolic function, as demonstrated with the LIPUS therapy. 11 Third, it remains to be examined whether this intraoperative SW therapy actually improves long-term prognosis of patients with ischaemic heart failure.
Some comments should be made on the LIPUS therapy. 1 Specific irradiation conditions of LIPUS (32 cycles, 0.25 W/cm 2 , 1.875 MHz for the heart and 0.5 MHz for the brain) have been demonstrated to induce similar biological effects as SW. 1 LIPUS therapy may have some advantages over SW therapy in terms of efficacy and safety, including shorter treatment time (as compared with extracorporeal SW therapy) and no harmful effects on the lung, etc. LIPUS therapy has been suggested to be effective and safe for severe angina 12 , 13 and also Alzheimer’s disease. 14 , 15 For Alzheimer’s disease, it has been demonstrated that multiple neuroprotective responses are up-regulated in the whole brain in response to the LIPUS stimulation. 14 This is in contrast to the fact that drug therapies need a certain therapeutic target for drug development, which may cause polypharmacy issue, and cell therapies, where the fate of exogenously administered cells may not be completely controlled. Currently, new therapies remain to be developed for major organ diseases, including dementia in the brain, heart failure in the heart and chronic kidney disease in the kidney, where microvascular dysfunction plays a major pathogenetic role. 1 Sound-wave therapies appear to be promising modalities to treat these diseases through improvement of microvascular dysfunction ( Graphical Abstract ). 1
In conclusion, sound-wave therapies with SW or LIPUS are a new emerging class of therapy to activate self-healing abilities depending on disease-specific conditions with reasonable efficacy and safety and appear to have broad indications as compared with current drug therapies and cell therapies ( Graphical Abstract ).
Disclosure of Interest
HS is the founder and executive chairman of the Sound Wave Innovation, Ltd. ( https://sw-innovation.com/ ).
Shindo T , Shimokawa H . Therapeutic angiogenesis with sound waves . Ann Vasc Dis 2020 ; 13 : 116 – 25 . https://doi.org/10.3400/avd.ra.20-00010
Google Scholar
Huang C , Hlfeld J , Schaden W , Orgill D , Ogawa R . Mechanotherapy: revisiting physical therapy and recruiting mechanobiology for a new era in medicine . Trends in Mol Med 2013 ; 19 : 555 – 64 . https://doi.org/10.1016/j.molmed.2013.05.005
Davies MJ , Earley S , Li Y-S , Chien S . Vascular mechanotransduction . Physiol Rev 2023 ; 103 : 1247 – 421 . https://doi.org/10.1152/physrev.00053.2021
Hatanaka K , Ito K , Shindo T , Kagaya Y , Ogata T , Eguchi K , et al. Molecular mechanisms of the angiogenic effects of low-energy shock wave therapy: roles of mechanotransduction . Am J Physiol Cell Physiol 2016 ; 311 : C378 – 85 . https://doi.org/10.1152/ajpcell.00152.2016
Shindo T , Ito K , Ogata T , Hatanaka K , Kurosawa R , Eguchi K , et al. Low-intensity pulsed ultrasound enhances angiogenesis and ameliorates left ventricular dysfunction in a mouse model of acute myocardial infarction . Arterioscler Thromb Vasc Biol 2016 ; 36 : 1220 – 9 . https://doi.org/10.1161/ATVBAHA.115.306477
Nishida T , Shimokawa H , Oi K , Tatewaki H , Uwatoku T , Abe K , et al. Extracorporeal cardiac shock wave therapy markedly ameliorates ischemia-induced myocardial dysfunction in pigs in vivo . Circulation 2004 ; 110 : 3055 – 61 . https://doi.org/10.1161/01.CIR.0000148849.51177.97
Fukumoto Y , Ito A , Uwatoku T , Matoba T , Kishi T , Tanaka H , et al. Extracorporeal cardiac shock wave therapy ameliorates myocardial ischemia in patients with severe coronary artery disease . Coron Artery Dis 2006 ; 17 : 63 – 70 . https://doi.org/10.1097/00019501-200602000-00011
Kikuchi Y , Ito K , Ito Y , Shiroto T , Tsuburaya R , Aizawa K , et al. Double-blind and placebo-controlled study of the effectiveness and safety of extracorporeal cardiac shock wave therapy for severe angina pectoris . Circ J 2010 ; 74 : 589 – 91 . https://doi.org/10.1253/circj.CJ-09-1028
Holfeld J , Nagele F , Polzl L , Engler C , Graber M , et al. Direct cardiac shockwave therapy in addition to coronary bypass surgery for improvement of myocardial function in ischemic heart failure (CAST-HF): an investigator-initiated, sham-controlled trial . Eur Heart J .
Holfeld J , Tepekoylu C , Reissig C , Lobenwein D , Scheller B , Kirchmar E , et al. Toll-like receptor 3 signalling mediates angiogenic response upon shock wave treatment of ishaemic muscle . Cardiovasc Res 2016 ; 109 : 331 – 43 . https://doi:10.1093/cvr/cvv272
Monma Y , Shindo T , Eguchi K , Kurosawa R , Kagaya Y , Ikumi Y , et al. Low-intensity pulsed ultrasound ameliorates cardiac diastolic dysfunction in mice: a possible novel therapy for heart failure with preserved left ventricular ejection fraction . Cardiovasc Res 2017 ; 117 : 1325 – 38 . https://doi.org/10.1093/cvr/cvaa221
Hanawa K , Ito K , Aizawa K , Shindo T , Nishimiya K , Hasebe Y , et al. Low-intensity pulsed ultrasound induces angiogenesis and ameliorates left ventricular dysfunction in a porcine model of chronic myocardial ischemia . PLoS One 2014 ; 9 : e104863 . https://doi.org/10.1371/journal.pone.0104863
Shindo T , Ito K , Ogata T , Kurosawa R , Eguchi K , Kagaya Y , et al. A randomized, double-blind, placebo-controlled pilot trial of low-intensity pulsed ultrasound therapy for refractory angina pectoris . PLoS One 2023 ; 18 : e0287714 . https://doi.org/10.1371/journal.pone.0287714
Eguchi K , Shindo T , Ito K , Ogata T , Kurosawa R , Kagaya Y , et al. Whole-brain low-intensity pulsed ultrasound therapy markedly improves cognitive dysfunctions in mouse models of dementia—crucial roles of endothelial nitric oxide synthase . Brain Stim 2018 ; 11 : 959 – 73 . https://doi.org/10.1016/j.brs.2018.05.012
Shimokawa H , Shindo T , Ishiki A , Tomita N , Ichijyo S , Watanabe T , et al. A pilot study of whole-brain low-intensity pulsed ultrasound therapy for early stage of Alzheimer's disease (LIPUS-AD): a randomized, double-blind, placebo-controlled trial . Tohoku J Exp Med 2022 ; 258 : 167 – 75 . https://doi.org/10.1620/tjem.2022.J078
Author notes
Month: | Total Views: |
---|---|
June 2024 | 32 |
Email alerts
Companion article.
- Cardiac shockwave therapy in addition to coronary bypass surgery improves myocardial function in ischaemic heart failure: the CAST-HF trial
Citing articles via
Looking for your next opportunity, affiliations.
- Online ISSN 1522-9645
- Print ISSN 0195-668X
- Copyright © 2024 European Society of Cardiology
- About Oxford Academic
- Publish journals with us
- University press partners
- What we publish
- New features
- Open access
- Institutional account management
- Rights and permissions
- Get help with access
- Accessibility
- Media enquiries
- Oxford University Press
- Oxford Languages
- University of Oxford
Oxford University Press is a department of the University of Oxford. It furthers the University's objective of excellence in research, scholarship, and education by publishing worldwide
- Copyright © 2024 Oxford University Press
- Cookie settings
- Cookie policy
- Privacy policy
- Legal notice
This Feature Is Available To Subscribers Only
Sign In or Create an Account
This PDF is available to Subscribers Only
For full access to this pdf, sign in to an existing account, or purchase an annual subscription.
- DOI: 10.1016/j.phymed.2024.155773
- Corpus ID: 270082896
Dan-Lou tablets reduce inflammatory response by inhibiting the activation of NLRP3 inflammasome for coronary heart disease.
- Shan Gao , Yuanyuan He , +7 authors Zhu Li
- Published in Phytomedicine 1 May 2024
30 References
Dan-lou tablets reduces inflammatory response via suppression of the myd88/p38 mapk/nf-κb signaling pathway in raw 264.7 macrophages induced by ox-ldl., anti-inflammatory and antioxidative effects of dan-lou tablets in the treatment of coronary heart disease revealed by metabolomics integrated with molecular mechanism studies., dan-lou prescription inhibits foam cell formation induced by ox-ldl via the tlr4/nf-κb and pparγ signaling pathways, danlou tablet inhibits the inflammatory reaction of high-fat diet-induced atherosclerosis in apoe knockout mice with myocardial ischemia via the nf-κb signaling pathway., effects of the tlr4/myd88/nf-κb signaling pathway on nlrp3 inflammasome in coronary microembolization-induced myocardial injury, nlrp3 inflammasome as a key driver of vascular disease., nlpr3 inflammasomes and their significance for atherosclerosis, the nlrp3 inflammasome: mechanism of action, role in disease and therapies., the anti-inflammatory activities of ethanol extract from dan-lou prescription in vivo and in vitro, the nlrp3 inflammasome: an overview of mechanisms of activation and regulation, related papers.
Showing 1 through 3 of 0 Related Papers

An official website of the United States government
The .gov means it’s official. Federal government websites often end in .gov or .mil. Before sharing sensitive information, make sure you’re on a federal government site.
The site is secure. The https:// ensures that you are connecting to the official website and that any information you provide is encrypted and transmitted securely.
- Publications
- Account settings
Preview improvements coming to the PMC website in October 2024. Learn More or Try it out now .
- Advanced Search
- Journal List
- Vasc Health Risk Manag
- PMC10128075
A Heart-Healthy Diet for Cardiovascular Disease Prevention: Where Are We Now?
1 Department of Epidemiology, Johns Hopkins Bloomberg School of Public Health, Baltimore, MD, USA
2 Department of Medicine, Greater Baltimore Medical Center, Baltimore, MD, USA
L Nedda Dastmalchi
3 Division of Cardiology, Temple University Hospital, Philadelphia, PA, USA
Martha Gulati
4 Smidt Heart Institute, Cedars-Sinai Medical Center, Los Angeles, LA, USA
Erin D Michos
5 Division of Cardiology, Department of Medicine, Johns Hopkins University School of Medicine, Baltimore, MD, USA
Purpose of Review
The relationship between cardiovascular health and diet is evolving. Lifestyle modifications including diet changes are the primary approach in managing cardiometabolic risk factors. Thus, understanding different diets and their impact on cardiovascular health is important in guiding primary and secondary prevention of cardiovascular disease (CVD). Yet, there are many barriers and limitations to adopting a heart healthy diet.
Recent Findings
Diets rich in fruits, vegetables, legumes, whole grains, and lean protein sources, with minimization/avoidance of processed foods, trans-fats, and sugar sweetened beverages, are recommended by prevention guidelines. The Mediterranean, DASH, and plant-based diets have all proven cardioprotective in varying degrees and are endorsed by professional healthcare societies, while other emerging diets such as the ketogenic diet and intermittent fasting require more long-term study. The effects of diet on the gut microbiome and on cardiovascular health have opened a new path for precision medicine to improve cardiometabolic risk factors. The effects of certain dietary metabolites, such as trimethylamine N-oxide, on cardiometabolic risk factors, along with the changes in the gut microbiome diversity and gene pathways in relation to CVD management, are being explored.
In this review, we provide a comprehensive up-to-date overview on established and emerging diets in cardiovascular health. We discuss the effectiveness of various diets and most importantly the approaches to nutritional counseling where traditional and non-traditional approaches are being practiced, helping patients adopt heart healthy diets. We address the limitations to adopting a heart healthy diet regarding food insecurity, poor access, and socioeconomic burden. Lastly, we discuss the need for a multidisciplinary team-based approach, including the role of a nutrition specialist, in implementing culturally-tailored dietary recommendations. Understanding the limitations and finding ways to overcome the barriers in implementing heart-healthy diets will take us miles in the path to CVD prevention and management.
Plain Language Summary
The Mediterranean, DASH, and plant-based diets remain the leading heart healthy diets that match cardiology professional society recommendations. Emerging diets like the ketogenic diet and intermittent fasting are effective weight loss diets with unknown heart protective benefits, and require further studies on whether the benefits outweigh the risks. Adopting a heart-healthy diet is a foundational component for cardiovascular disease prevention, but barriers and limitations to adopting heart healthy diets exist and need to be assessed as part of cardiovascular risk assessment.
Introduction
Despite the advances in medical management and evidence-supported dietary interventions for improved heart health, cardiovascular disease (CVD) remains the leading cause of death in the United States. 1 Over time, various diets have been studied for their effectiveness in CVD prevention. 2 One of the earliest epidemiological studies investigating the link between diet and CVD was the Seven Countries Study led by Ancel Keys between 1958 and 1964, which demonstrated that the myocardial infarction (MI) rate was lower in countries where fruits, vegetables, grains, beans, and fish formed the major part of the diet. 3 , 4 The INTERHEART Study then showed that 90% of MIs were due to preventable factors, with daily consumption of fruits and vegetables, along with regular physical activity, being associated with a 40% reduction in MI [Odds Ratio (OR)=0.60 (95% CI=0.51–0.71)]. 5 The Prospective Urban Rural Epidemiology (PURE) study conducted in over 130,000 individuals from 18 countries demonstrated that diets rich in fruits, vegetables, and legumes were associated with 19% lower risk of all-cause mortality [Hazard Ratio (HR)=0.81 (95% CI=0.68–0.96)] over 7-years of follow-up. 6
Lifestyle changes, including a heart-healthy diet, are the foundation of all CVD prevention guidelines. 7–10 The main cardiometabolic risk factors are diabetes, hypertension, dyslipidemia, and excess abdominal fat, which are all affected by dietary changes. 11 Unfortunately, there has been a worsening trend in CVD risk factors globally. 12 In the United States, the prevalence of type 2 diabetes (T2D) is 37 million adults, or 11% of the adult population, but an estimated 88 million US adults have prediabetes. 13 Globally, in 2021, 537 million individuals were affected by T2D, corresponding to 10.5% of the world’s population. 14 On a similar trend, the prevalence of controlled blood pressure, which had improved between 1999–2008, did not significantly change from 2007–2014, and then decreased after 2014. 15 Globally, between 1975 and 2016, the prevalence of obesity [defined in this study as a Body Mass Index (BMI) ≥25 kg/m 2 )] has tripled in adults. 16 In the US in 2017–2018, the age-adjusted prevalence of obesity (BMI ≥30 kg/m 2 ) among adults was 42% and severe obesity (BMI ≥40 kg/m 2 ) was 9%. 17 Increased visceral adiposity is a main driver of excess cardiometabolic risk, and increased abdominal waist circumference has become a CVD risk marker by itself. 18
Lifestyle modifications from exercise and dietary interventions have been well studied and proven effective toward CVD prevention and management. A healthy diet is one of the American Heart Association (AHA)’s “Life’s Essential 8” cardiovascular health metrics, which also include favorable levels of physical activity, sleep, nicotine exposure, BMI, blood lipids, blood glucose, and blood pressure. 19 However, <1% of US adults met “ideal” criteria for the AHA’s healthy diet metric in 2013–2014. 20
Diets rich in fruits, vegetables, legumes, whole grains, and lean protein sources, with minimization/avoidance of processed foods, trans-fats, and sugar sweetened beverages, are recommended by prevention guidelines. The Mediterranean, the Dietary Approaches to Stop Hypertension (DASH), and plant-based diets all have proven cardioprotective in varying degrees and are endorsed by professional healthcare societies, while other emerging diets such as the ketogenic diet and intermittent fasting require more long-term study. The effects of diet on the gut microbiome and on cardiovascular health have opened a new path for precision medicine to improve cardiometabolic risk factors. The effects of certain dietary metabolites, such as trimethylamine N-oxide (TMAO), on cardiometabolic risk factors, along with the changes in the gut microbiome diversity and gene pathways in relation to CVD management, are being explored.
In this review, we will provide a comprehensive up-to-date overview on established and emerging diets for cardiovascular health and CVD prevention. We will address the limitations to adopting a heart healthy diet from food insecurity, poor access, and socioeconomic burden. Lastly, we will discuss the need for additional nutritional education in health professional schools, and the importance of a multidisciplinary team to partner with patients to improve their nutritional knowledge and self-efficacy in adopting heart healthy diets.
Established Heart Healthy Diets
Three major dietary patterns (the Mediterranean diet, the DASH diet, and the healthy plant-based diet) ( Figure 1 ) have the most evidence for CVD prevention and are described below.

The Heart Healthy Dietary Patterns: Mediterranean Diet, DASH Diet, and Healthy Plant-Based Diet.
The Mediterranean Diet
The Mediterranean diet, which was inspired by the eating habits of countries that border the Mediterranean sea (ie, Spain, Italy, and Southern Greece) is one that is rich in whole-grains, leafy green vegetables, fruits, legumes, unsalted nuts, herbs, spices, and extra virgin olive oil (EVOO), with a moderate intake of lean proteins such as fish and poultry, moderate alcohol consumption, and limited intake of red meat and sweets. By its very nature, the Mediterranean diet limits or eliminates many of the culprits in the standard American diet that drive CVD – saturated fat, highly processed refined grains and sugars, and red meat. 2 , 10 , 21 The Mediterranean diet differs from other dietary patterns as there is a greater emphasis on EVOO, nuts, reduced dairy consumption, and eating with family. 22 The Mediterranean diet has been found to be favorable as a food pattern, rather than single nutrient supplementation, as it inherently provides anti-oxidants, reduces inflammation of the vascular wall, modulates pro-atherogenic genes, alters gut microbiome, and improves lipid panels by decreasing low density lipoprotein cholesterol (LDL-C) and raising high density lipoprotein cholesterol (HDL-C). 21 , 23–25
Nutritional genomic studies show that greater adherence to a Mediterranean diet blunt the development of an adverse cardiometabolic phenotype in genetically susceptible individuals, with interactions between the Mediterranean diet and polymorphisms of the cyclooxygenase-2, interleukin-6, apolipoprotein A2, cholesteryl ester transfer protein plasma, and transcription factor 7-like 2 genes. 26 One study found that the Mediterranean diet plus EVOO prevented increases in levels of pro-atherothrombotic genes such as Cox-2, low-density lipoprotein receptor-related protein, and monocyte chemoattractant protein 1 expression compared to a control diet. 27
As mentioned above, the benefits of the Mediterranean diet were first appreciated in the Seven Countries Study (Yugoslavia, Italy, Greece, Finland, the Netherlands, Japan, and the US), led by Ancel Keys in 1958, who found there was a higher rate of mortality from coronary heart disease (CHD) in men from the US and Northern Europe in comparison to regions in Southern Europe. 3 , 28 The countries in southern Europe had higher consumption of olive oil, fruits, vegetables and fish, and lower consumption of meat and animal fats, in comparison to populations in the US and Northern Europe. These populations were also found to be leaner and had active lifestyles, a stark contrast from the US and Northern Europe populations who were mainly sedentary. 28 Prospective observational studies also found similar correlations, where persons living in Greece who reported higher adherence to the Mediterranean dietary pattern (per 2 point increment in Mediterranean diet score) were found to have a significantly lower risk of CHD death with an adjusted HR of 0.67 (95% CI=0.47–0.94) over an average of 44 months. 29 In another cohort of 15,482 patients who had stable CHD, higher adherence to a Mediterranean-style diet (per 1 unit increase in scores above 12) was independently associated with a 5% reduction in cardiovascular events over 3.7 years (HR=0.95; 95% CI=0.91–0.98). 30 Closer adherence to a Mediterranean-style diet has also been shown to be associated with a lower risk of preeclampsia. 31
Randomized controlled trials (RCTs) followed these epidemiological studies and have demonstrated the cardiovascular benefits of the Mediterranean style diet. The Lyon Diet Heart Study was a RCT that assessed whether the Mediterranean diet was beneficial in the secondary prevention of CVD among patients after a first MI. 32 The authors found a greater than 70% reduction in recurrent non-fatal MI and greater than 50% reduction in mortality in the group adhering to the Mediterranean diet, compared to a prudent diet control group, over a 4-year period. 32 , 33
The Prevención con Dieta Mediterránea (PREDIMED) trial was a RCT that aimed to assess the role of the Mediterranean diet for the primary prevention of CVD among 7,447 patients at elevated cardiovascular risk. 34 Participants were randomized to one of three diets: the Mediterranean diet with higher amounts of EVOO, the Mediterranean diet with higher amounts of nuts, or a standard diet that was low in fat, with dietary guidance. 34 After 4.8 years, there was approximately a 30% reduction in MI, stroke, or CVD death among those consuming the Mediterranean diet with EVOO (HR=0.69; 95% CI=0.53–0.91) as well as in the group consuming the Mediterranean diet with nuts (HR=0.72; 95% CI=0.54–0.95), compared to the low-fat diet. 34 Risk factors for CVD were also shown to benefit with Mediterranean diet intervention, with the incidence of diabetes being 52% lower (95% CI=27–86%) in the two pooled Mediterranean diet groups compared to controls. 35 In a biomarkers subgroup analysis, patients following the Mediterranean diet had reduction in N-terminal pro-B-type natriuretic peptide, and those on the Mediterranean diet with EVOO were found to have a reduction in oxidized LDL and lipoprotein(a) plasma concentrations, in comparison to those on the low fat diet. 36 Overall the PREDIMED study was one of the groundbreaking trials to show that a Mediterranean diet rich in unsaturated fats and polyphenols can be useful for primary CVD prevention.
Further research has been done to understand why the Mediterranean diet is so valuable in reducing CVD risk. One meta-analysis of observational epidemiologic studies evaluated whether EVOO itself shows benefit in CVD. 37 Researchers found that a higher consumption of fats from EVOO had a reduced relative risk (RR) of 0.85 (95% CI=0.77–0.93) for CVD and of 0.83 (95% CI=0.77–0.90) for all-cause mortality. The researchers believed that the anti-inflammatory and antioxidant effects play a role through its abundance of phenolic compounds and vitamin E with the addition of substituting saturated fats from the diet with monounsaturated and polyunsaturated fats. 37 Additionally, the anti-inflammatory properties of EVOO play a role in reducing platelet aggregation. 21 The emphasis of high fiber foods, such as whole grains and vegetables, in the Mediterranean-style diet provides benefit in the gut microbiome. 38 With improvement in the biodiversity of the gut microbiome (as discussed further later in this review), beneficial effects of blood pressure control, reduction in metabolic syndrome and diabetes, lower levels of pro-atherogenic compounds, and ultimately lower rates of CVD have been demonstrated. 39 Red meat, which is minimized in the traditional Mediterranean diet, contains the nutrient L-Carnitine, which produces the pro-atherogenic compound γ-butyrobetaine (γBB) as it gets converted to trimethylamine and TMAO by the gut microbes, both of which accelerate atherosclerosis. 40 These favorable elements of the Mediterranean dietary pattern are further enhanced by the emphasis of physical activity, which has shown to improve cardiometabolic health and reduce CVD. 21
The DASH Diet
The DASH diet was created in 1997 by the National Heart Lung and Blood Institute to prevent and treat hypertension, a known risk factor for CVD. 41 The DASH diet is rich in fruits and vegetables, low-fat dairy, whole grain, poultry, fish, nuts, and seeds, while limiting fatty meats, sweets, sugar sweetened beverages, and full-fat dairy products. 42 The standard DASH diet restricts salt consumption to 2,300 mg per day. It meets the Dietary Guidelines for Americans’ recommendation of limiting daily salt consumption to less than 2,300 mg. A reduced-sodium version of DASH limits sodium intake to 1,500 mg per day. Significant reduction in cardiometabolic risk is seen with the DASH diet by lowering blood pressure, total cholesterol, and LDL-C, all of which are significant risk factors which are accounted for in the atherosclerotic CVD (ASCVD) risk score and eventually leads to improvement in cardiac function and decreased incidence of diabetes. 43–46 One of the main key elements of the DASH diet in lowering blood pressure is the sodium restriction in comparison to other diets. 41 , 42 , 46
A DASH diet has been shown to reduce blood pressure in persons with or without hypertension, where in an RCT, the DASH diet reduced blood pressure by 5–6 mmHg systolic and 3 mmHg diastolic, while also lowering LDL-C by 11 mg/dL. 41 In observational data, a DASH-style dietary pattern has also been associated with a lower risk of CVD, heart failure, and diabetes. 47 , 48 In the Dietary Intervention to Stop Coronary Atherosclerosis in Computed Tomography (DISCO) RCT, 92 individuals, 41% women, with non-obstructive coronary atherosclerosis were randomized to either DASH diet with optimal medical therapy or control group of just optimal medical treatment. 49 At a mean of just 67 weeks, a significant reduction in noncalcified plaque in the DASH diet intervention group was found in comparison to the medical treatment alone group. 49
The Healthy Plant-Based Diet
A healthy plant-based diet has been shown to be associated with lower cardiovascular risk. 50–55 Plant-based diets, a diet consistently of predominantly plants, can be diverse in content, patterns, and even have subsets. The subsets branch into vegetarian (which excludes meat, poultry, seafood, and fish) and vegan (in which all animal food and their products are eliminated from the diet). Other plant-based diet subsets include lacto-vegetarians, who consume dairy products, lacto-ovo-vegetarians, who consume dairy and eggs, pesco-vegetarians or pescatarians, who consume fish, dairy, and eggs, and lastly, semi-vegetarians, who exclude red meats, possibly poultry as well.
These diets are assessed based on the vegetable content of the diet, but do not necessarily account for the sugar, fat, and sodium content, which can decrease the cardioprotective nature of a plant-based diet. 51 Indeed, unhealthy plant-based diets (such as those higher in refined grains, potatoes/fries, juices/sweetened beverages, sweets) may have similar CVD risk as animal-based diets. 51 The main foundation of a healthy plant-based diet is the whole grains, fruits, vegetables, nuts, and legumes with the low frequency and content of animal food. It has low energy density, with low saturated fats and high fiber content, which triggers early satiety cues and helps with digestion by delaying gastric emptying, with moderate insulinemic and glycemic responses. 56 , 57 Furthermore, studies have shown improved lipid profiles in individuals who follow a plant-based diet due to increased fiber intake, as fat absorption decreases overall. 58
Many studies examined this type of diet from different aspects using a pro-vegetarian diet score, which assigns higher scores to plant foods and negative scores to animal foods, or a similar plant-based diet index. In the PREDIMED trial in Spain, vegetarian diets were associated with lower cardiovascular mortality (HR=0.47; 95% CI=0.21–1.04 for highest quintile compared to lowest; p -trend=0.039 across quintiles). 59 In two combined observational cohorts of more than 200,000 male and female health professionals in the US, a higher plant-based diet index was inversely associated with incidence of CVD (HR comparing extreme deciles: 0.92; 95% CI=0.83–1.01; p -trend=0.003 across deciles), a relationship which was stronger for a healthy plant-based diet index (HR 0.75; 95% CI=0.68–0.83; p -trend<0.001). 51 In another cohort of young and middle-aged adults from the Coronary Artery Risk Development in Young Adults (CARDIA) cohort followed for nearly 32 years, the highest vs lowest quintile of plant-based diet score (time-averaged) was associated with a 52% reduction in incident CVD (HR=0.48; 95% CI=0.28–0.81). 60
Furthermore, plant-based diets have low processed meat content or none. Preservatives found in processed meats such as nitrates and sodium contribute to blood pressure elevation, impaired insulin response, and endothelial dysfunction. 61 Substantial reductions in cardiometabolic modifiable risk factors, such as blood pressure, LDL-C, blood glucose levels, inflammatory markers, and unhealthy weight patterns, even with similar caloric intake, were seen in plant-based diets in comparison to omnivorous diets. 55 , 61–63 Thus, this type of diet has a great potential in CVD prevention. However, this diet tends to have lower vitamin B12, vitamin D, calcium, zinc, protein, and retinol in comparison to omnivorous diets; for example, those following a vegan diet may require additional supplementation with vitamin B12. 64
Reconciling the Healthy Diet Patterns and the Importance of Minimally Processed Food
The Mediterranean, DASH, and healthy plant-based diets have more similarities than differences. Each of these diets emphasize fruits, vegetables, legumes, nuts, and whole grains. Diets rich in fruits and vegetables have been shown to be associated with lower risk of CVD and mortality. 6 , 65 Increased whole grain consumption is also associated with lower risk of CVD and mortality. 66 Higher consumption of nuts has also been shown to be associated with lower CVD risk. 67
Eating minimally processed whole foods is an important component to all healthy diets, as consuming poor diet quality is one of the leading contributors to cardiometabolic disease globally. 68 , 69 Ultra-processed foods are high in refined carbohydrates, added sugars, saturated and trans fats, sodium, and possibly artificial colors, flavors, and preservatives, and have high energy density and glycemic load, which all contribute to increased cardiometabolic risk factors. They also tend to be highly palatable so that individuals eat more calories in total. 70 Furthermore, eating more ultra-processed foods can result in displacement of cardioprotective foods such as fruits and vegetables. In the Framingham Offspring Study, each additional serving a day of ultra-processed foods was associated with a 7% increased risk of incident CVD (HR=1.07; 95% CI=1.03–1.12). 70 Similarly, in another cohort from Spain, each additional serving/day of ultra-processed food was associated with 18% higher mortality (HR=1.18; 95% CI=1.05–1.33). 71 In controlled feeding trials comparing diets that were matched for total calories, sugar, fat, fiber, and macronutrients, participants allowed ad libitum food intake ate ~500 kcal/day on the ultra-processed diet vs an unprocessed diet, and body weight changes were correlated with dietary differences in energy intake. 72
Emerging or Controversial Diets
Additional emerging diet patterns have been studied for their impact on cardiometabolic risk; these are more controversial due to conflicting data regarding their net cardiovascular health benefits or insufficient data to determine their long-term cardiovascular benefits. Two of the more promising ones, the Keto Diet and Time-Restricted Eating (TRE), are discussed below.
The Keto and Other Very Low Carb Diets
The ketogenic (or “keto”) diet is generally characterized by intake of very-low carbohydrate (VLC), high fat, and moderate protein. The class ketogenic diet divided total daily energy intake to be 90% from fat, 7% from protein, and only 3% from carbohydrates. Since that time, other formulations of the ketogenic diet have been proposed to improve compliance, as well as other low or very low carbohydrate diets that generally follow similar patterns of high fat low carbs, but do not generate ketosis. VLC diets have been promoted for their effects on weight loss, improvement in insulin resistance, and glycemic control; however, the high fat diet can promote increases in LDL-C, particularly in the subset of individuals who are LDL hyper-responders, suggestive of latent genetic dyslipidemias. 73–75 This has limited the enthusiasm of many professional medical societies from endorsing its use. 73 , 75
The ketogenic diet emerged in the early 1920s as an anti-seizure intervention, but with medical innovation and the development of effective anti-seizure medication, the ketogenic diet is no longer used for management of epilepsy. 76 Throughout time the ketogenic diet has been revisited by many nutritionists and scientists to explore the ketosis state of the body. It has been found that antioxidants and anti-inflammatory functions of genes were activated by the beta-hydroxybutyrate (BHB) metabolite product of ketosis. 75 BHB has been found to reduce reactive oxygen species production mainly by activating the nuclear factor erythroid-derived 2-related factor 2 (Nrf2) which is the major inducer of detoxification genes. Furthermore, it is an endogenous inhibitor of class I and class IIa histone deacetylases which upregulates the transcription of detoxifying genes including catalase, mitochondrial superoxide dismutase and metallothionein 2. 77 In an open label non-randomized control study of the nutritional ketosis state of patients with T2D, reductions in diabetes medication use, hemoglobin A1c (HbA1c), and overall weight were seen after 1 year, while patients were being supported in a continuous care model. 78 Individuals with insulin resistance or diabetes cannot properly metabolize glucose in the blood, which makes excess carbohydrates intake unfavorable in terms of glycemic control, and as such, following a VLC diet helps improve glycemic control and HbA1c. 79 , 80 Other studies evaluating VLC diets in the management of obesity found it more effective than low fat diets in improvement in cardiometabolic risk factors. 80–83 Furthermore, increased satiety due to the high fat and protein content and possible effects of the ketones on the appetite was found in individuals following a ketogenic diet. 84
On the other hand, some of the adverse effects that limit adoption of the ketogenic diet are the increase in total cholesterol, LDL-C, non-HDL cholesterol, triglycerides, and total apoB, all of which increase the risk of CVD. 73 , 74 , 82 , 85 , 86 The effect of a ketogenic diet on LDL-C is inconsistent, 86 but some patients can have a very dramatic increase in LDL-C in response. This dramatic increase in LDL-C levels can possibly exacerbate development of hyperlipidemia if an underlying genetic predisposition is present. 87 Furthermore,a recent meta-analysis found the ketogenic diet conferred unfavorable effects on LDL-C, apoB, and total cholesterol in normal weight adults. 88 The potential deleterious effect on lipids needs to be considered carefully before initiating a ketogenic diet, especially for individuals at elevated CVD risk such as those with T2D, and lipid levels should be monitored in patients following a ketogenic diet. Theoretically, a plant-based, ketogenic diet that is low in saturated fats may limit these adverse consequences, but there are no trials examining this. Long-term data of the efficacy and safety of ketogenic diets are still lacking.
Intermittent Fasting/Intermittent Energy Restrictions
Intermittent fasting (IF) (also known as intermittent energy restriction) diets have different forms and patterns with three general goals: prolonged periods of fasting prompting a state of ketosis, decreasing oxidative stress, and feeding and circadian rhythm synchronization. 89 The IF strategy holds promise for cardiovascular health, but the evidence is still limited, and lacking long-term studies. 90–93 A recent systematic review of RCTs studied the cardiovascular health benefits of IF in comparison to continuous calories restriction; although IF was found to be more effective in weight loss, it was not clinically significant in reduction of cardiometabolic risk factors. 93 Benefits on the molecular and cellular level of improved mitochondrial health, DNA repair, autophagy, and promotion of stem cell-based regeneration were found in laboratory mice. 94 Reducing oxidative stress is thought to decrease mitochondrial energy production and in return free radical production leading to reduced overall inflammation. 95 Alternatively, the reduction in weight and, hence, cardiometabolic risk factors with IF type diets may simply be the result of fewer total calories consumed given the reduced/restricted hours of feeding.
There are two main patterns of IF: alternate day fasting (ADF) and time restricted eating (TRE). TRE limits consumption of calories to a certain window of time each day, usually a 6–10 hour period. The ADF pattern is having a fast day followed by a feast day. A systemic review and meta-analysis on whether ADF helps with weight loss found the cumulative evidence suggests that ADF does reduce weight, BMI, and total cholesterol. 96 However, the studies were of short duration (<6 months long). In an RCT studying the effects of ADF in comparison to a calorie restricted diet or control diet on cardiometabolic risk factors, during a 1 year follow-up period of 6 months weight loss and 6 months maintenance, it was found that ADF was not superior to calories restriction in weight loss, weight maintenance, and reduction of cardiometabolic risk factors. 97
Regarding TRE, in a study of 139 individuals with unhealthy weight, no significant weight loss difference was found between individuals who adopted TRE with calorie restriction of 1,500 to 1,900 calories in comparison to calorie restriction alone (net difference=−1.8 kg; 95% CI=−4.0 to 0.4; P =0.11). 98 On the other hand, several studies have shown that TRE from a 6 to 10 hours window was effective in weight loss, cardiometabolic health enhancement, while maintaining muscle mass. 99–101 In one recent study of firefighters who have a shift-work occupation, researchers demonstrated that a 10-hour/day TRE window was feasible with improvement in cardiometabolic risk factors over 12 weeks compared to a standard feeding pattern. 102
Another pattern of TRE is one that is focused on aligning diet with the circadian rhythm with the aim to improve insulin sensitivity and weight loss. 103 Having feeding in sync with the circadian rhythm is hypothesized to optimize energy metabolism with the active and inactive phases of the circadian rhythm. Studies have shown that when the circadian rhythm is set (whether it be nocturnal or diurnal), TRE during the active phase has more health benefits and improved glucose tolerance with lower insulin levels needed. 104 For example, for a nocturnal rhythm, the active phase would be the dark time.
The Gut Microbiome and Heart Health
Lifestyle modifications of diet and exercise have been discussed extensively, but the relationship between cardiometabolic risk factors and the gut microbiome (the heart–gut axis) ( Figure 2 ) is an emerging area of study. 39 , 105 The gut microbiome is affected by dietary intake, antimicrobials, pre-and pro-biotics, and fecal microbial transplant, with potential impact on cardiovascular risk factors. For example, probiotics use has shown benefits in mild reduction in blood pressure, blood glucose, and TMAO levels. 106 Higher fiber intake is associated with increased diversity of the gut microbiota, 38 and a higher fiber diet is associated with lower risk of hypertension and CVD. 107

The Heart–Gut axis (the role of the Gut Microbiome in Cardiovascular Health).
Individuals with hypertension were found to have altered microbial gut structure, function, and interaction. 108 While the autonomic nervous system plays a key role in regulating blood pressure, derangements in the gut microbiome triggers the release of inflammatory mediators and metabolites leading to neuroinflammation and increased blood pressure in return. 109 Furthermore, in resistant hypertension, researchers have found altered microbial pathways and metabolites that may drive the increase in blood pressure and be potential targets for new therapeutic approaches in hypertension management. 110 Additionally, certain dietary metabolites, such as TMAO, that are found in red meat, poultry, and fish, have been associated with increased risk of CVD. 111 TMAO have been linked to kidney fibrosis and loss of function, heart failure, atherosclerosis, and vascular inflammation, as well as thrombosis through platelet hyper-responsiveness. 39 , 111 , 112 One of the dietary protein metabolites of the gut microbiota is phenylacetic acid, which is metabolized by the liver and converted to phenylacetylglutamine that activates adrenergic receptors and in return increases platelets responsiveness and aggregation. 112 Understanding the pathways and interactions of the gut microbiome with diet and medication will help direct precision medicine in CVD management to have specific dietary recommendations, and possibly microbiome targeted therapies.
Cardiology Professional Society Recommendations
The AHA dietary guidance statement to promote cardiometabolic health was most recently released in 2021. 113 Diet recommendations were also included in the 2019 American College of Cardiology (ACC)/AHA Primary Prevention of CVD Guideline. 7 These guidelines recognize that sedentary lifestyle and increased caloric intake has led to increased excess body weight, which is a modifiable cardiometabolic risk factor. Thus, adjusting energy intake and expenditure to achieve and maintain a healthy body weight was the first recommendation in the dietary guidance. 113 These guidelines recognize the importance of healthy dietary patterns, rather than focusing on individual foods or nutrients, and recommend healthy nutrition to begin early in life. 113
Additionally the AHA statement recommends eating plenty and a variety of colorful fruits and vegetables; 113 “eating the rainbow” is one such approach. 114 Leafy green vegetables, other vegetables, whole fruits rather than juice, and legumes can be consumed in all forms such as fresh, frozen, or dried, although canned fruit which often contains added syrups should be avoided. 115 The guidelines also recommend choosing whole grain foods and products over refined grains. Whole grains, or products made with 51% whole grains, are rich in fiber which help support healthy weight patterns, improve digestion, and decrease absorption of cholesterol and associated with lower CVD risk. 57 , 107
The AHA statement also recommends eating healthy sources of protein, predominantly plant-based such as legumes and nuts. 113 Non-fried fish and seafood intake is recommended given the high omega-3 fatty acid content, especially when it substitutes animal sources of red and processed meat or full-fat dairy products, with ~2 to 3 servings per week of fish being associated with lower CVD incidences. 113 , 116 Other sources of protein include the low-fat or fat-free dairy products which have been shown to have neutral or beneficial effects on CVD risk. 113 , 117 If poultry or meat is desired, the AHA guidelines recommend eating lean and unprocessed forms. Several studies showed increased CVD and mortality incidence with increased intake of red meats. 118–121 Increased saturated fats, heme iron content, and l-carnitine metabolites such as TMAO from red meat contribute to atherosclerosis. 121 , 122
The AHA statement recommends using liquid plant oils instead of tropical oils or partially hydrogenated fats. 113 The benefits of EVOO, which is high in monounsaturated fat, was reviewed in the Mediterranean diet section. Dietary unsaturated fats, that are found in liquid plant oils such as soybean, corn, safflower and sunflower oils, walnuts, and flax seeds have shown cardiovascular health benefits of reducing LDL-C and total cholesterol levels. 123 On the other hand, tropical oils like coconut and palm oils, animal fats like butter and lard, as well as hydrogenated fats raise LDL-C and total cholesterol levels. 124 , 125 Another source of healthy fats is intake of fish that is rich in omega-3 fatty acids, which may have benefits on triglycerides and overall CVD risk, although little effect on LDL-C. 123
There had been some conflicting studies about whether linoleic acid (LA), which is found in vegetable oils such as corn, sunflower, and soybean oils, has a harmful effect on cancer and cardiovascular risk. However the purported pro-inflammatory effects of LA and other omega-6 fatty acids have not been conclusively confirmed, with even some studies showing a reduction in inflammation. 126 Furthermore, a meta-analysis found higher intakes of LA to be associated with lower (not higher) risk of CVD 127 and, along with other evidence, an author of a review concluded that LA intake within the range recommended by AHA has no harms associated with it. 128 Another more recent systematic review confirmed the reduction in CHD, CVD, and all-cause mortality with LA, but a slight increased risk in cancer mortality was noted (RR=1.06; 95% CI=1.02–1.11). 129 Other meta-analyses have not confirmed an excess cancer risk with higher LA intake. 130 Laboratory studies suggest, at higher thresholds, LA does not have tumor-promoting effects and may even be beneficial. 131 , 132 Although a small increase risk in cancer cannot be definitely excluded, there is not sufficient evidence that higher intake of LA substantially increases risk for breast, colorectal, or prostate cancer risk and, thus, should not be specifically avoided. 132
The ACC/AHA prevention guidelines discussed reducing added sugars and minimizing sugar-sweetened beverages, 7 , 113 as added sugars have been associated with increased risk of T2D, obesity, and CVD. 133 , 134 Additionally, the AHA guidance is to choose or prepare foods with little or no added salt, and to choose minimally processed foods instead of ultra-processed foods. 7 , 113 As for alcohol intake, the guidelines do not support initiation of alcohol intake for cardio-protection, and to limit alcohol if one does choose to drink. 113 Although moderate alcohol intake of 1–2 glasses per day has been associated with low risk of CHD and ischemic stroke, 135 the evidence behind this is not strong, 135 and some data suggest any alcohol intake, even one glass, is associated with increased risk of atrial fibrillation. 136
Approaches to Nutrition Counseling in CV Health
With the vast number of dietary behaviors proven to benefit cardiovascular health, implementation of these patterns can be cumbersome. Studies show that, despite ~80% of CVDs being preventable by adopting a healthier lifestyle, healthy eating behaviors are low, and there are numerous barriers to implementing these recommendations. Healthcare professionals have adopted multiple methods, such as nutrition counseling, culinary medicine, food pharmacies, and inpatient nutrition, all of which have been evaluated as effective means to implement dietary changes to patients. 137–139 Nutrition counseling through cognitive or behavioral theory has been shown to be most effective in dietary behaviors, weight, and cardiovascular risk factors as it is a supportive process that helps set priorities, establish goals, and create an individualized plan. 138–140 Motivational interviews modeled through behavioral theory, with self-monitoring, meal replacements, and/or meal plans help create a method of self-care. Client-centered counseling techniques in the long-term have been shown to be beneficial in effective chronic disease prevention where one study showed improvement in blood pressure and salt intake. 138 , 140 As nutrition counseling by a dietitian is shown to be beneficial, group counseling is a modality that takes the elements of individual nutrition counseling into a larger population through group therapy or culinary medicine. 137 , 138
Culinary medicine is an evidence-based approach to healthy eating where there is an emphasis on health promotion and illness management through dietary regimens. 141 Food pharmacies are designed to increase public access to fruits and vegetables and fully integrate the concept of “food is medicine” into health care systems. 142 These centers address financial barriers, knowledge gaps regarding healthy eating, and cooking skills by prescribing fruits and vegetables to patients. Community outreach programs have been successful in dietary changes through education, group counseling, and culinary medicine. 143 One such outreach program, “Shop With Your Doc”, has been shown to help implement positive dietary changes by patients gaining knowledge on how to read nutrition labels, read ingredients, and how to choose fresh produce. 144 Using surveys, one study found that there was an improvement in fresh vegetable consumption and less waste after produce allocation and educational intervention in low income populations. 143 Using the skills from nutrition counseling and applying them to an interactive community model, where patients can gain skills and apply them to their daily routines, may help implement larger scale changes. 141 , 143 , 144
The outpatient and community programs may offer guidance from a physician, dietitian, and a health coach to assist in not only improved diet, but also improve lifestyle with exercise programs with stress management techniques. Similarly, inpatient dietary guidance can be a method to implement the importance of nutrition when patients may be amenable to lifestyle changes. 139 , 145 Studies support that patients are most vulnerable to lifestyle changes after a life altering event, such as an MI. 146 In response to the data supporting the importance of nutrition in treating and preventing CVD, there has been greater pressure from hospital institutions in providing nutritious meals to inpatients. 139 Public policies, such as the Healthy Food in Health Care initiative, have been placed to assist hospitals in obtaining local produce and reducing meat products on the menu to improve patient nutrition and decrease environmental impact. 147 Despite these efforts to improve hospital menus, the effect on dietary behaviors needs to be further studied.
Limitations and Disparities to Implementing Dietary Changes
There are many challenges, but also many opportunities, to implementing healthy heart eating patterns ( Figure 3 ).

Challenges and Opportunities for Implementing a Heart Healthy Diet.
Some of the main challenges and barriers to behavior or diet change have to do with the compatibility of regimen with patient lifestyles, including social and cultural factors of socioeconomic status, lack of social support, and certain cultural values, all of which have to be taken into consideration when recommending dietary changes. All diet recommendations need to be considered in the context of social determinants of health and societal barriers that drive health inequities. 148–151 Underserved populations, ethnic minorities, gender, and sexual minorities have been found to have a higher incidence of CVD and suffer from greater mortality. 151 , 152 These same groups are also faced with social barriers that make it difficult to adhere to preferable dietary patterns to improve their cardiovascular health. Additionally, individuals of lower income have been shown to be less likely counseled on diet modifications for CVD prevention in a representative US sample. 153
“Food deserts” (or grocery areas that lack fresh produce), “food swamps” (with excess of ultra-processed poor quality foods), and food insecurity all are contributors to obesity and chronic disease. 150 , 154 , 155 Recent studies have shown that food insecurity is a growing problem in the United States. 156 As a result of the COVID-19 pandemic, food insecurity has tripled, with an even higher prevalence among US adults with an income below the poverty level. 156 In comparison to high and middle income families, persons of low socioeconomic class are more likely to under consume fruits, vegetables, and whole grains and instead consume highly processed meats and beverages. 143 The fluctuating nature of eating food insecurity causes has been found to increase insulin resistance, increase blood pressure, and lead to poor weight management, which ultimately increase CVD risk. 152 , 156 These groups of patients may also not be able to access health care or seek treatment, which could lead to undiagnosed cardiovascular risk factors; this trend has been seen in gender and sexual minorities. 152 Food insecurity thus may pose as a strong barrier to adhering to lifestyle interventions, thus social and political support to provide access is needed.
Opportunities remain for improvement. Community engagement, such as through faith-based organizations, has been demonstrated to be successful at promoting fruit and vegetable consumption, along with other preventive strategies. 157 Culturally relevant and language appropriate resources are needed to assist patients from diverse backgrounds in making healthier choices. Family meals eaten together can be an important factor for cardiovascular health promotion, especially with parental modeling of healthy behaviors such as intake of vegetables and fruits; companionship at mealtimes that establishes a positive atmosphere around meals has been associated with improved dietary quality. 158
Physicians and other healthcare professionals can be the greatest advocates for their patients and can help provide counseling and information to access foods. However, medical schools are lacking in nutrition education and on average provide 20 hours of nutrition education to medical students. 159 Additionally, there is little emphasis on counseling and teaching patients about diet and how to make foods on a budget while maintaining cultural appropriateness. In recent years, programs have been placed where students engage in community outreach and culinary medicine practices. 137 These programs help to improve confidence in the knowledge of nutrition and their ability to provide counseling. Improving the nutritional education of physicians and other healthcare professionals, working as part of interdisciplinary teams, can help improve partnerships with their patients in improving nutritional quality and dietary behaviors with the aim to reduce CVD. 159
Conclusions
The AHA highlights diet as one of “Life’s Essential Eight” to help promote cardiovascular health and avoid disease. The Mediterranean diet remains one of the most effective and recommended balanced diets for cardiovascular health promotion, followed by the DASH diet for hypertension prevention and management, and healthy plant-based diet with careful consideration to its sugar and salt content as well as nutrient deficiencies. Other emerging diets that are widely adopted for weight loss like the keto or VLC diets remain controversial on whether the benefits of weight loss outweigh potential adverse changes to the lipid profile for example, and whether IF patterns have additional cardiometabolic benefits beyond simply the reduction of total calorie intake. The effects of diet on the gut microbiome and the importance of the gut–heart axis may be the future of our cardiovascular dietary recommendations where precision nutrition guides precision prevention.
Funding Statement
Dr. Michos is funded by the Amato Fund for Women’s Cardiovascular Health at Johns Hopkins University and by an American Heart Association Award, Number: 946222. Dr. Gulati is funded by the Anita Dann Friedman Chair in Women’s Cardiovascular Medicine and Research, and by the Department of Defense (Award Number: W81XWH-17-2-0030).
Abbreviations
ACC, American College of Cardiology; AHA, American Heart Association; ADP, Alternate Day Fasting; BMI, Body Mass Index; CVD, Cardiovascular Disease; DASH, Dietary Approaches to Stop Hypertension; HDL-C, High Density Lipoprotein Cholesterol; IF, Intermittent Fasting; LDL-C, Low Density Lipoprotein Cholesterol; MI, Myocardial Infarction; RCT, Randomized Controlled Trial; T2D, Type 2 Diabetes; TRE, Time Restricted Eating; TMAO, Trimethylamine-N-oxide; US, United States; VLC, Very Low Carbohydrate.
Unrelated to this work, Dr. Michos has served on advisory boards for Amgen, Amarin, AstraZeneca, Bayer, Boehringer Ingelheim, Esperion, Novartis, Novo Nordisk, and Pfizer. Dr. Gulati has served on advisory boards for Novartis and Bayer. The authors report no other conflicts of interest in this work.

Create Free Account or

- Acute Coronary Syndromes
- Anticoagulation Management
- Arrhythmias and Clinical EP
- Cardiac Surgery
- Cardio-Oncology
- Cardiovascular Care Team
- Congenital Heart Disease and Pediatric Cardiology
- COVID-19 Hub
- Diabetes and Cardiometabolic Disease
- Dyslipidemia
- Geriatric Cardiology
- Heart Failure and Cardiomyopathies
- Invasive Cardiovascular Angiography and Intervention
- Noninvasive Imaging
- Pericardial Disease
- Pulmonary Hypertension and Venous Thromboembolism
- Sports and Exercise Cardiology
- Stable Ischemic Heart Disease
- Valvular Heart Disease
- Vascular Medicine
- Clinical Updates & Discoveries
- Advocacy & Policy
- Perspectives & Analysis
- Meeting Coverage
- ACC Member Publications
- ACC Podcasts
- View All Cardiology Updates
- Earn Credit
- View the Education Catalog
- ACC Anywhere: The Cardiology Video Library
- CardioSource Plus for Institutions and Practices
- ECG Drill and Practice
- Heart Songs
- Nuclear Cardiology
- Online Courses
- Collaborative Maintenance Pathway (CMP)
- Understanding MOC
- Image and Slide Gallery
- Annual Scientific Session and Related Events
- Chapter Meetings
- Live Meetings
- Live Meetings - International
- Webinars - Live
- Webinars - OnDemand
- Certificates and Certifications
- ACC Accreditation Services
- ACC Quality Improvement for Institutions Program
- CardioSmart
- National Cardiovascular Data Registry (NCDR)
- Advocacy at the ACC
- Cardiology as a Career Path
- Cardiology Careers
- Cardiovascular Buyers Guide
- Clinical Solutions
- Clinician Well-Being Portal
- Diversity and Inclusion
- Infographics
- Innovation Program
- Mobile and Web Apps
Study Finds Air Pollution Can Increase Cardiovascular Risk for Cancer Patients
Jun 18, 2024
Contact: Olivia Walther, [email protected] ,
WASHINGTON (Jun 18, 2024) -
Modern therapies have extended the lives of many cancer patients; however, survivors often live with chronic health conditions, including cardiovascular disease. New research published in JACC: CardioOncology shows that air pollution plays a significant role in increasing cardiovascular disease and mortality in cancer patients and contributes to health disparities related to these conditions.
“The review underscores the critical need to consider environmental factors, especially air pollution, in cardio-oncology risk assessment and patient management,” said Xiaoquan Rao, MD, PhD, senior author of the study and a cardiologist at Tongji Hospital at the Tongji Medical College in Wuhan, China.
“By highlighting the significant role of air pollution in the cardiovascular health of cancer patients, our work aims to catalyze further research in this field and inform clinical practices and public health policies,” Rao said.
Rao noted that air pollution has been recognized as a significant risk factor for both cardiovascular diseases and cancer; however, little research has been done to study its effects, specifically in cardio-oncology or the overlap of both diseases. The new study was motivated by a need to fill that gap, Rao said.
Researchers reviewed papers published between 2000 and 2023 and found eight studies that directly explored the combined effect of air pollution on cardiovascular disease and cancer.
They found that exposure to PM 2.5 , or fine particulates in the air, was significantly associated with higher rates of both incidence and mortality of cardiovascular disease among cancer patients — and vice versa. Rao noted that air pollution exposure appears to impact multiple common risk factors shared by both cancer and cardiovascular disease, including inflammatory and oxidative stress pathways.
According to researchers, a surprising finding was that even short-term exposure to high pollution levels rapidly impacted cancer patients’ cardiovascular health.
“This suggests that even temporary deteriorations in air quality can have immediate adverse effects on vulnerable populations such as cardio-oncology patients,” Rao said.
The paper also highlights how air pollution contributes to health disparities worldwide. Disadvantaged populations are exposed to higher levels of air pollution, and cancer patients with a lower socioeconomic status face a higher risk of cardiovascular disease mortality linked to air pollution than the general public, researchers said.
“More research is needed, including clinical studies, to understand the impacts of air pollution on cardiovascular disease and cancer in greater detail," Rao said.
Rao added that the findings can be used to better understand the risks of air pollution and help identify more vulnerable populations within cardio-oncology.
“This awareness is crucial for developing tailored air pollution exposure control measures and individualized patient management strategies aimed at mitigating cardiovascular disease risks among cancer patients,” Rao said.
Future research will focus on exploring air pollution-related health disparities across different types of cancer and cardiovascular diseases. Researchers also hope to evaluate the effectiveness of environmental interventions in reducing the impact of air pollution on cardio-oncology patients.
The paper, “Air Pollution in Cardio-oncology and Unraveling the Environmental Nexus,” is part of a JACC: CardioOncology focus issue on social and environmental determinants of health and health disparities.
For an embargoed copy of any paper in the focus issue, contact Olivia Walther at [email protected].
The American College of Cardiology (ACC) is the global leader in transforming cardiovascular care and improving heart health for all. As the preeminent source of professional medical education for the entire cardiovascular care team since 1949, ACC credentials cardiovascular professionals in over 140 countries who meet stringent qualifications and leads in the formation of health policy, standards and guidelines. Through its world-renowned family of JACC Journals, NCDR registries, ACC Accreditation Services, global network of Member Sections, CardioSmart patient resources and more, the College is committed to ensuring a world where science, knowledge and innovation optimize patient care and outcomes. Learn more at www.ACC.org or follow @ACCinTouch.
The ACC’s JACC Journals rank among the top cardiovascular journals in the world for scientific impact. The flagship journal, the Journal of the American College of Cardiology ( JACC) — and specialty journals consisting of JACC: Advances, JACC: Asia, JACC: Basic to Translational Science, JACC: CardioOncology, JACC: Cardiovascular Imaging , JACC: Cardiovascular Interventions , JACC: Case Reports, JACC: Clinical Electrophysiology and JACC: Heart Failure — pride themselves on publishing the top peer-reviewed research on all aspects of cardiovascular disease. Learn more at JACC .org .
< Back to Listings
You must be logged in to save to your library.
Jacc journals on acc.org.
- JACC: Advances
- JACC: Basic to Translational Science
- JACC: CardioOncology
- JACC: Cardiovascular Imaging
- JACC: Cardiovascular Interventions
- JACC: Case Reports
- JACC: Clinical Electrophysiology
- JACC: Heart Failure
- Current Members
- Campaign for the Future
- Become a Member
- Renew Your Membership
- Member Benefits and Resources
- Member Sections
- ACC Member Directory
- ACC Innovation Program
- Our Strategic Direction
- Our History
- Our Bylaws and Code of Ethics
- Leadership and Governance
- Annual Report
- Industry Relations
- Support the ACC
- Jobs at the ACC
- Press Releases
- Social Media
- Book Our Conference Center
Clinical Topics
- Chronic Angina
- Congenital Heart Disease and Pediatric Cardiology
- Diabetes and Cardiometabolic Disease
- Hypertriglyceridemia
- Invasive Cardiovascular Angiography and Intervention
- Pulmonary Hypertension and Venous Thromboembolism
Latest in Cardiology
Education and meetings.
- Online Learning Catalog
- Products and Resources
- Annual Scientific Session
Tools and Practice Support
- Quality Improvement for Institutions
- Accreditation Services
- Practice Solutions

Heart House
- 2400 N St. NW
- Washington , DC 20037
- Contact Member Care
- Phone: 1-202-375-6000
- Toll Free: 1-800-253-4636
- Fax: 1-202-375-6842
- Media Center
- Advertising & Sponsorship Policy
- Clinical Content Disclaimer
- Editorial Board
- Privacy Policy
- Registered User Agreement
- Terms of Service
- Cookie Policy
© 2024 American College of Cardiology Foundation. All rights reserved.

IMAGES
VIDEO
COMMENTS
Coronary Heart Disease Essay. Sort By: Page 1 of 50 - About 500 essays. Decent Essays. Coronary Heart Disease. 745 Words; 3 Pages; Coronary Heart Disease. According to the National Heart, Lung and Blood Institute (NHLBI), Coronary heart disease is a cardiovascular disease caused by a cholesterol substance termed plaque, accumulating within the ...
The coronary heart disease studies were not restricted during the search by sample size because of the limited number of studies in selected countries. ... The review included published papers covering both a healthy subject and a population with existing cardiovascular disease with different atherosclerosis risk factors during the literature ...
The Heart Protection Study (HPS) showed unambiguous benefit of statin administration in individuals aged 40 to 80 years with total cholesterol >135 mg/dL and at risk because of a previous MI or other coronary or noncoronary artery occlusive disease, diabetes mellitus, or treated hypertension. 19 The Physicians' Health Study (PHS) showed that ...
Heart disease, including coronary heart disease, remains the leading cause of death in the United States. However, the rate of heart disease deaths has declined by 70% over the past 50 years, thanks in part to NHLBI-funded research. Many current studies funded by the NHLBI focus on discovering genetic associations and finding new ways to ...
Cardiovascular disease (CVD) is an umbrella term for a number of linked pathologies, commonly defined as coronary heart disease (CHD), cerebrovascular disease, peripheral arterial disease, rheumatic and congenital heart diseases and venous thromboembolism. Globally CVD accounts for 31% of mortality, the majority of this in the form of CHD and ...
Individuals in the top decile compared with the lowest decile had a thirty-fold (men) to seventy-fold (women) difference in the incidence of coronary heart disease. Subsequent papers provided risk functions, or "risk profiles," that enabled physicians to directly calculate an individual's predicted risk of having a cardiovascular event.
Introduction. Thought of broadly, the management of patients with stable coronary artery disease (CAD) involves significant complexity including the diagnosis of CAD, assessment of associated risk, treatment, and monitoring of symptoms during follow up. 1 To provide sufficient focus in this month's topic review in Circulation: Cardiovascular Quality and Outcomes, we have concentrated on the ...
The strongest association was observed for smoking and coronary heart disease (relative risk of 5.48, 95% CI 4.67 to 6.42) for nurses who smoked >12 cigarettes per day compared with never-smokers. For alcohol consumption, exercise, body mass index, and diet score, the risk ratios varied between 1.41 and 1.90.
Heart disease is a fatal human disease, rapidly increases globally in both developed and undeveloped countries and consequently, causes death. Normally, in this disease, the heart fails to supply ...
No disease can have been so extensively studied. The work of recent years has yielded remarkable advances in our understanding, and in diagnostic and interventional cardiology, surgery, and pharmacology—effort well spent for a disease that carries such heavy social and economic costs. The clinical condition of coronary heart disease (CHD) presents as (i) angina, (ii) myocardial infarction ...
The objective of this series is to provide our readership with a timely, comprehensive selection of important papers that are relevant to the quality and outcomes, and general cardiology audience. The studies included in this article represent the most significant research related to treatment of stable coronary artery disease (CAD).
Essays. Reflection and perspective are invited, collected, and presented here about the causes, care, control, prevention, epidemiology, and public policy of heart attacks. A. Keys response to G. Mann's 1977 NEJM Op-Ed: "Diet-Heart. End of an Era.".
Essay on Coronary Heart Disease. Coronary heart disease occurs when the coronary arteries become partially blocked from, cholesterol and fat, circulating in the blood. This causes a build up inside the walls of the arteries. This only occurs if the levels of fat and cholesterol are extremely high in the blood that there is a greater chance that ...
Pathophysiology of Coronary Heart Disease: As previously touched upon, Coronary Heart Disease is caused by a build up of fat deposits within the coronary arteries and more than 110,000 people in the UK die each year because of this condition. These fatty deposits or atheroma as they are medically termed are a mixture of cholesterol and other ...
Percutaneous coronary intervention (PCI) is the standard treatment for ischemic heart disease. Precise evaluation of stenosis and the reference diameter is essential. Since visual assessments of lumen diameter and stenosis lack objectivity, quantitative coronary angiography (QCA) is commonly used in clinical settings to evaluate coronary artery ...
Essay On Coronary Heart Disease. Coronary heart disease makes up 56% of all deaths relating to heart conditions. The heart pumps blood around the body carrying oxygen and other essential nutrients to the areas in the body where they are needed.1 Coronary Heart disease is when this process is restricted or interrupted.
Coronary artery disease is a condition in which there is an inadequate supply of blood and oxygen to the myocardium. It results from occlusion of the coronary arteries and results in a demand-supply mismatch of oxygen. It typically involves the formation of plaques in the lumen of coronary arteries that impede blood flow. It is the major cause of death in the US and worldwide. At the beginning ...
Coronary Artery Disease. Since its inception, articles published in Arteriosclerosis, Thrombosis, and Vascular Biology ( ATVB) have contributed to our understanding of coronary artery disease (CAD) and its different complex pathophysiological processes. Here, we review articles related to CAD published in ATVB in the past 2 years from 2018 to 2019.
Keywords: coronary heart disease, biomarker, Circular RNA Suggested Citation: Suggested Citation Zhao, Xiya and Chen, Yudan and Chen, Xinyi and Mao, Ruoxin and Lu, Haoxuan and Xie, Yanqing and He, Fuwei and Chen, Xiaomin and He, Wenming, Clinical Significance of Enhanced Expression of Circular Rna Hsa_Circrna_405788 in Coronary Heart Disease.
Background: Coronary heart disease (CHD) is a key cause of heart failure (HF). Many Salvia miltiorrhiza Bunge Extract (SmE) preparations have emerged. However, it is still unclear the clinical efficacy and safety in the treatment of CHD comorbidity with HF.
Cardiovascular disease (CVD), particularly coronary heart disease (CHD), is the leading cause of death in the US, with a high economic impact. Coronary artery calcium (CAC) is a known marker for CHD and a useful tool for estimating the risk of atherosclerotic cardiovascular disease (ASCVD). ... Search 219,068,179 papers from all fields of ...
Stay Connected; Get Email Alerts; Receive automatic alerts about NHLBI related news and highlights from across the Institute.
Currently, new therapies remain to be developed for major organ diseases, including dementia in the brain, heart failure in the heart and chronic kidney disease in the kidney, where microvascular dysfunction plays a major pathogenetic role. 1 Sound-wave therapies appear to be promising modalities to treat these diseases through improvement of ...
@article{Gao2024DanLouTR, title={Dan-Lou tablets reduce inflammatory response by inhibiting the activation of NLRP3 inflammasome for coronary heart disease.}, author={Shan Gao and Yuanyuan He and Yutong Liu and Lu Yu and Qihong Cheng and Shuo Wang and Lin Li and Yuanlu Cui and Chunquan Yu and Zhu Li}, journal={Phytomedicine : international ...
1. Introduction. With the increasingly serious urban aging, more and more people suffer from cardiovascular disease (CVD) [].A recent epidemiological survey indicated that there are currently 290 million patients with CVD in China, of which more than 10 million are with coronary heart disease (CHD) and 4.5 million are patients with heart failure.
Decent Essays. 982 Words. 4 Pages. Open Document. Coronary Heart Disease. Coronary heart disease remains the most common cause of death in the United Kingdom. A statistic from the American Heart Association is that heart disease claims a life every 24 seconds. [www.americanheart.org] Another is that it is the largest single cause of premature ...
Coronary artery disease (CAD) is the world-wide leading cause of death not only in high-income countries but also increasingly in developing countries. 1 Although death rates from CAD have decreased in most high- and middle-income countries in the past 2 decades, there are worrying signs of a lessening trend in the United States, 2 and the dramatic increases of world-wide obesity 3 and ...
Cardiovascular disease (CVD) is a collective term designating all types of affliction affecting the blood circulatory system, including the heart and vasculature, which, respectively, displaces and conveys the blood. This multifactorial disorder encompasses numerous congenital and acquired maladies. CVD represents the leading noncommunicable cause of death in Europe (∼50% of all deaths; ∼ ...
A healthy diet is one of the American Heart Association (AHA)'s "Life's Essential 8" cardiovascular health metrics, which also include favorable levels of physical activity, sleep, nicotine exposure, BMI, blood lipids, blood glucose, and blood pressure. 19 However, <1% of US adults met "ideal" criteria for the AHA's healthy diet ...
Researchers reviewed papers published between 2000 and 2023 and found eight studies that directly explored the combined effect of air pollution on cardiovascular disease and cancer. They found that exposure to PM 2.5 , or fine particulates in the air, was significantly associated with higher rates of both incidence and mortality of ...