- Netherlands
- Saudi Arabia
- South Africa
- Switzerland
- United Arab Emirates
- United Kingdom
Children's Education

The education system in Portugal
Discover all you need to know about the education system in Portugal, including resources, financial aid, support, and more.
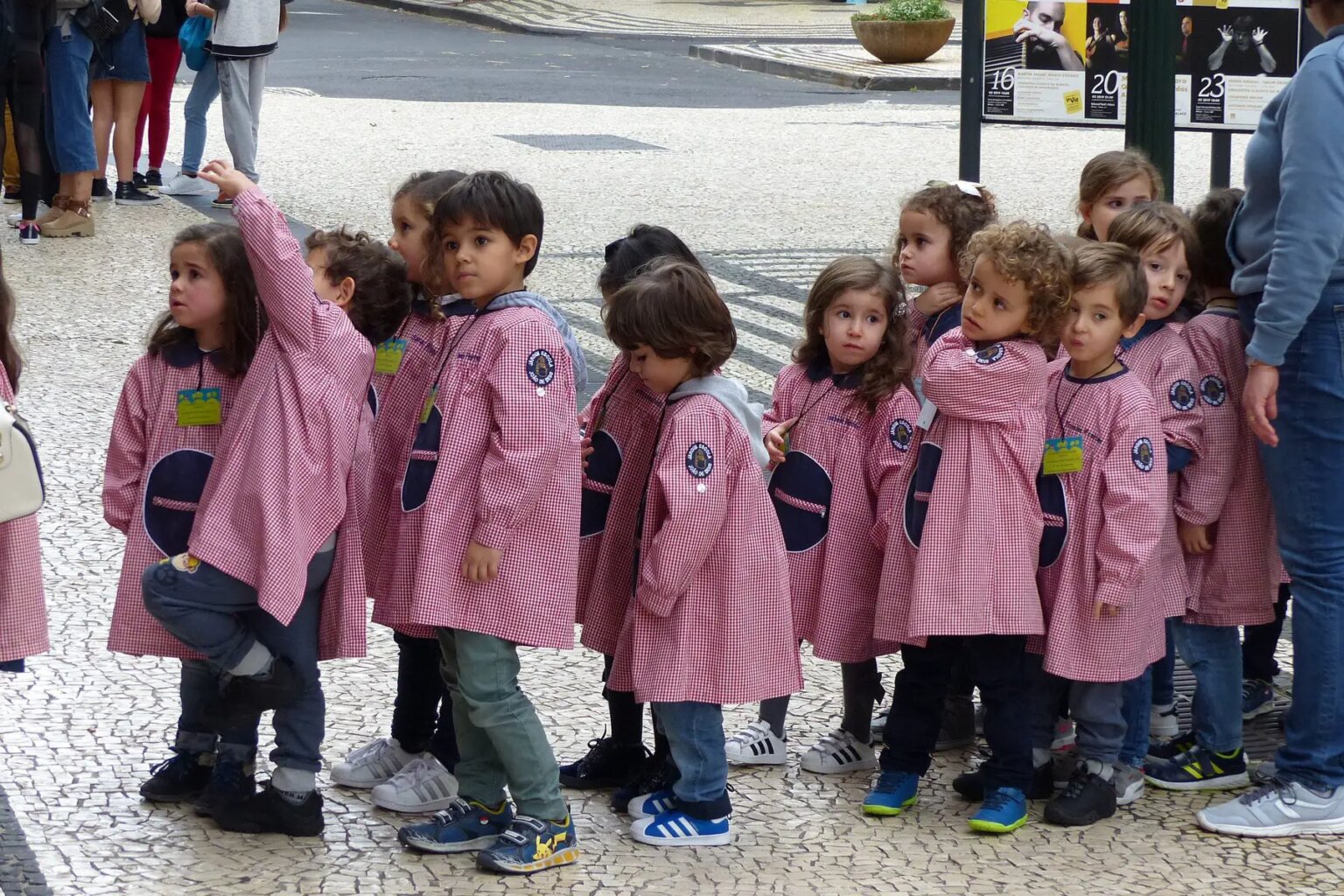
By Joana Taborda
Updated 4-6-2024
Learning how to navigate a country’s education system can be challenging for many expat parents . Depending on your child’s age, education in Portugal can follow different paths. They can study at a private or international school , a free state school, or join a vocational course that is adapted to a specific career.
If you’re feeling a bit lost, this article will help you understand every step of the process. From childcare for your little ones to secondary school options, we’ll discuss the following:
The structure of education in Portugal
Preschool education in portugal, the primary school system in portugal, public primary schools in portugal, private primary schools in portugal, the secondary school system in portugal, public secondary schools in portugal, religious schools, international schools, boarding schools, the international baccalaureate (ib) in portugal, graduating in portugal, financial aid and scholarships in portugal, educational support for expat students in portugal, support for children with special educational needs (sen), changing schools in portugal, chances for parental involvement in schooling in portugal, homeschooling in portugal, useful resources, bright international school.
Bright International School in Loulé, the Algarve, offers the Cambridge International Curriculum for Pre-Reception to Year 9. With globally recognized programs for early years, primary, and lower secondary, your child’s education is in safe hands. Join an international community of 30+ different nationalities at Bright International School.
Go to website
Education in Portugal
The Ministry of Education ( Direção-Geral da Educação – DGE ) regulates the education system in Portugal, starting from pre-school up until secondary school . School groups ( agrupamentos escolares ) also work closely with local municipalities to assign funding and define the curriculum. The Ministry of Science, Technology, and Higher Education ( Ministério da Ciência, Tecnologia e Ensino Superior – MCTES ) oversees policies and budgets for tertiary education in the country.
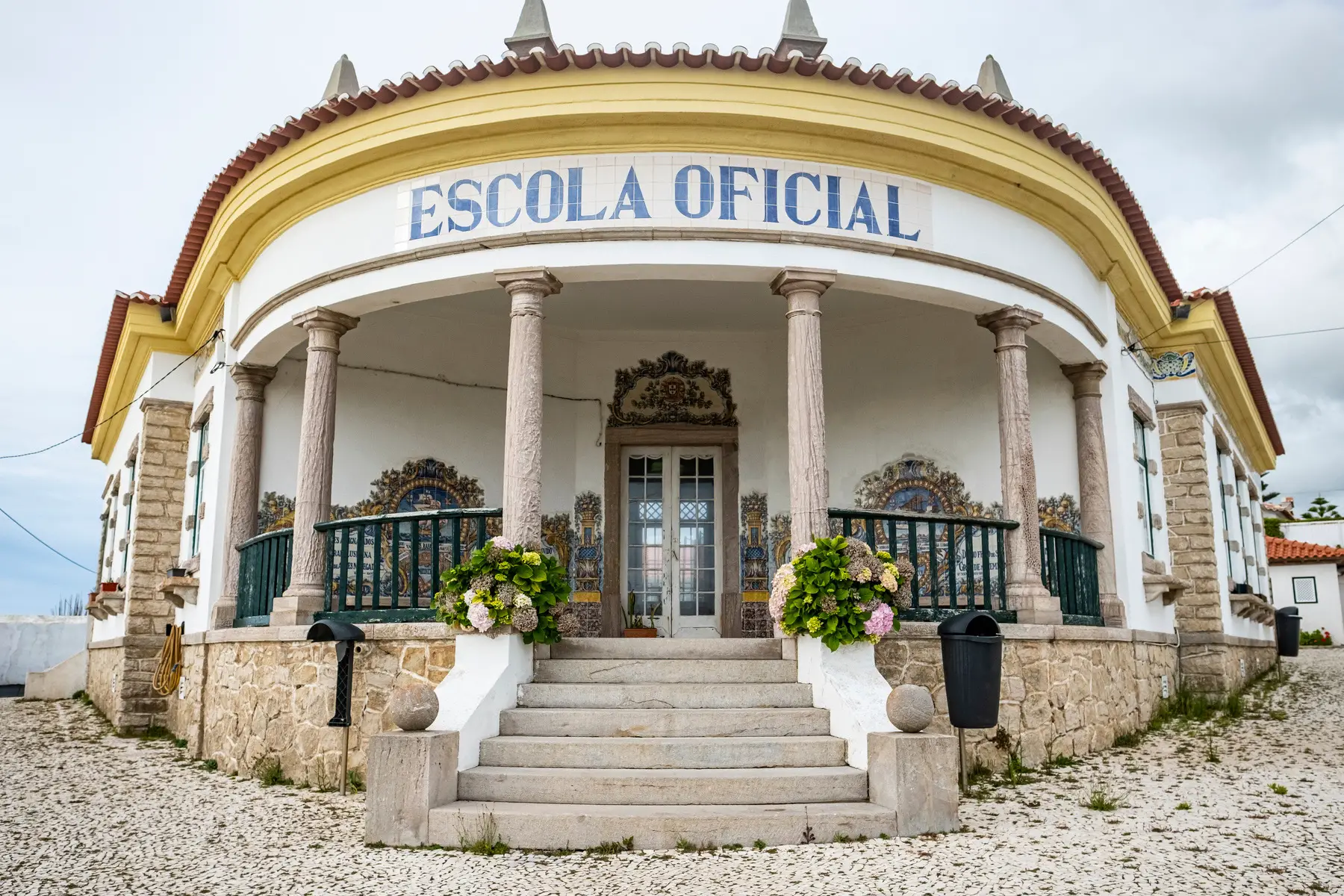
While Portugal offers a mix of state and private schools, most Portuguese families send their children to state schools because they are free and local. Many parents also enroll their children before the age of six, which is when mandatory education begins. However, private institutions have other benefits. For example, international schools cater primarily to expats and offer various curriculums from around the world. As a result, lessons can be taught in your child’s native language.
Despite the fact that Portugal has historically had a high illiteracy rate , the country’s current level of education is actually relatively good. For instance, students performed above average on the 2018 PISA tests, which cover reading, mathematics, and science. Furthermore, 92% of children attended pre-school in Portugal in 2019, which is higher than the OECD average of 88%.
The education system in Portugal consists of four stages , as follows:
- Pre-school education ( Educação Pré-escolar ): ages 3 to 6
- Primary education ( Ensino Básico ): ages 6 to 15
- Secondary education ( Ensino Secundário ): ages 15 to 18
- Higher education ( Ensino Superior ): ages 18 and above
School is mandatory from the ages of 6 to 18. After that, students can proceed to university or apply for a job .
Sadly, many immigrant children can feel segregated in schools. This is either due to the way that the class structure is organized (i.e. separating students that had to repeat a year) or by varying test results. As a result, many migrant children achieve lower grades in exams . However, new national reforms are creating an opportunity for students to have extra language and teaching support to improve their learning outcomes.
The Ministry of Labor, Solidarity, and Social Security ( Ministério do Trabalho, Solidariedade e Segurança Social ) governs Portugal’s childcare services under the age of three. However, early childcare institutions are mainly privatized. Parents can choose between kindergartens ( creches ) or childminders ( amas ) who come to their homes.
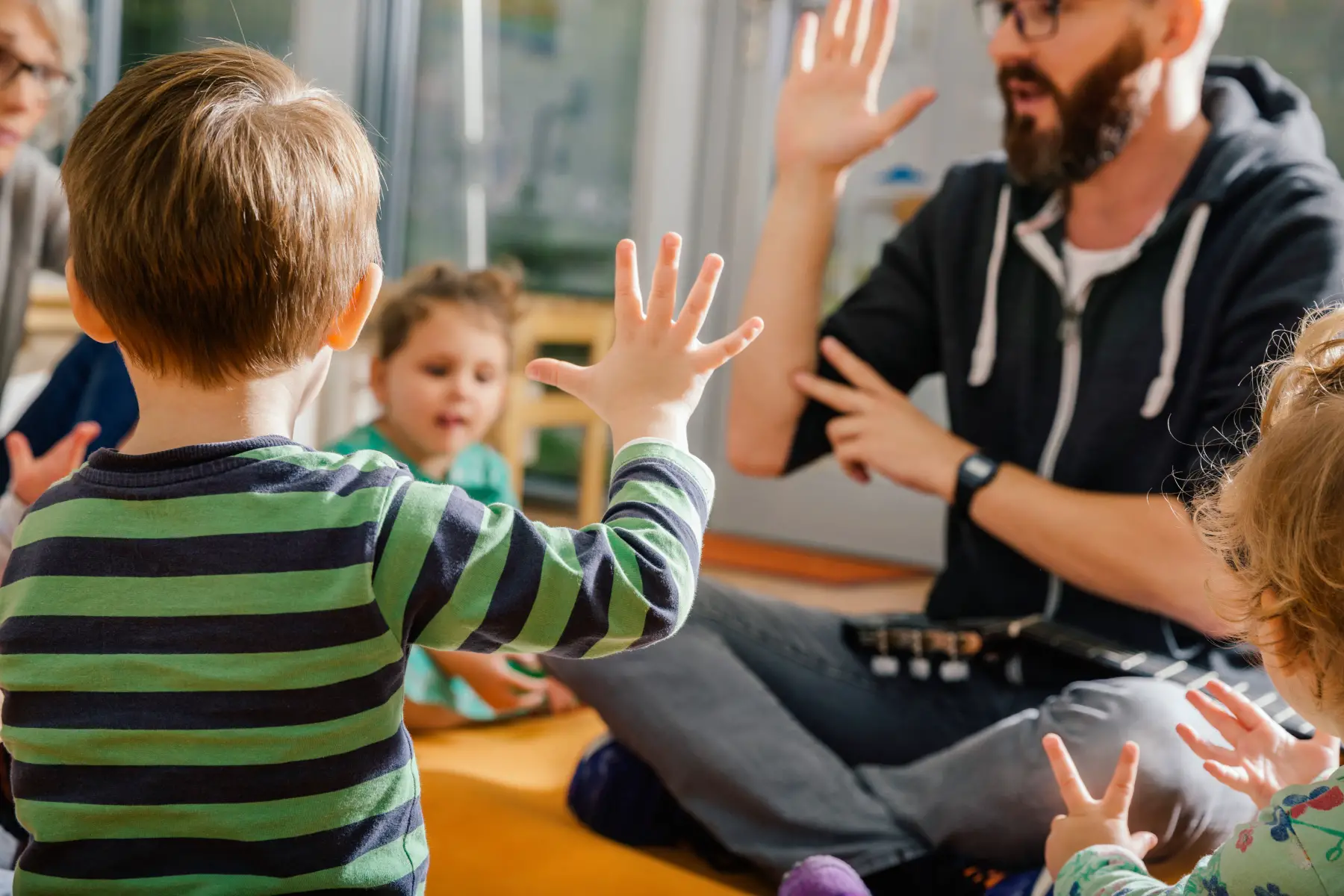
Childcare in Portugal
Children between the ages of three and six can attend preschool, and while this is not mandatory, many working parents choose this option. There are public and private preschools available in Portugal, and some charities and social institutions also offer childcare. Preschool education ( Educação Pré-escolar ) is free for 25 hours a week from the age of three. However, private pre-schools, including international schools, Montessori , and Waldorf institutions, charge tuition fees.
Primary education in Portugal
The Ministry of Education manages all policies regarding state schooling at the primary stage. Primary education ( Ensino Básico ) is compulsory in Portugal from the age of 6 to 15.
These schools are known as escolas básicas and include three cycles:
- First cycle ( primeiro ciclo or 1º ciclo ): Grades 1 to 4
- Second cycle ( segundo ciclo or 2º ciclo ): Grades 5 to 6
- Third cycle ( terceiro ciclo or 3º ciclo ): Grades 7 to 9
Because public education is free in Portugal, most locals choose this option. Indeed, caregivers only need to cover a few costs such as books, school meals, and the occasional field trip.
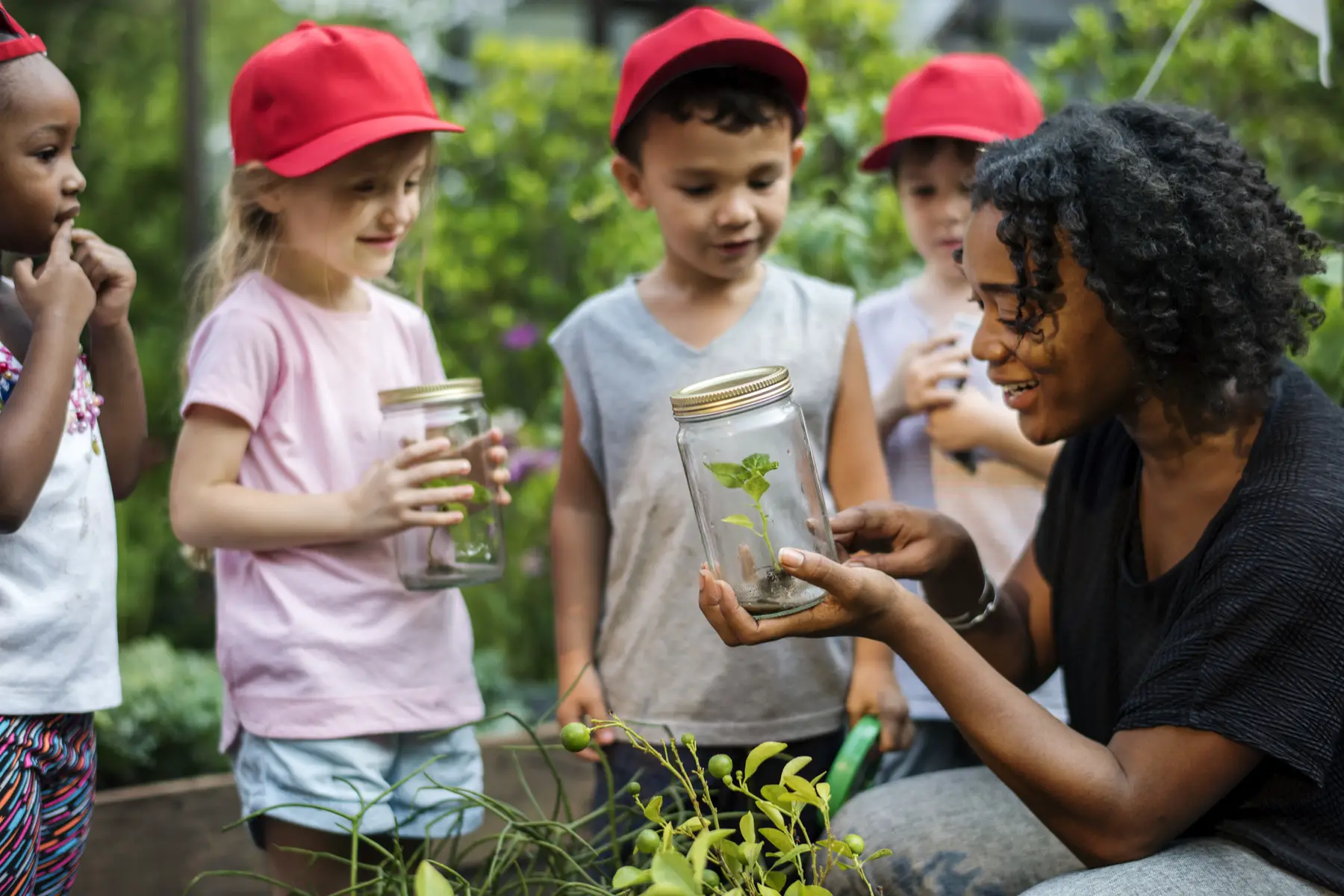
Overall, the quality of public education in Portugal is good. That said, international children might struggle if they don’t speak Portuguese, which is the instruction language. But if a child’s parents decide to stay longer in the country, then learning the language early may benefit them.
The national curriculum is similar for all public schools across the country. Pupils study Portuguese, mathematics, science, history, geography, and English which is a mandatory subject. This may be why Portugal has become one of the top 10 English-speaking countries in Europe. Additionally, from Grade 7, students can choose to study another foreign language, which is usually French or Spanish.

Primary schools in Portugal
It is important to be aware that you must choose a school that is close to your residence or place of work. Furthermore, when applying, you will need to show proof of your address. There can also be waiting lists, especially for popular public schools, so it’s best to register early.
In 2020, only 12.5% of students attended private primary schools in Portugal. However, international schools, which fall under this category, are often a good fit for expat children. This is because they allow students to study in their native language and interact with other children from across the globe. It also gives them continuity in their education if they relocate to another country or return home.
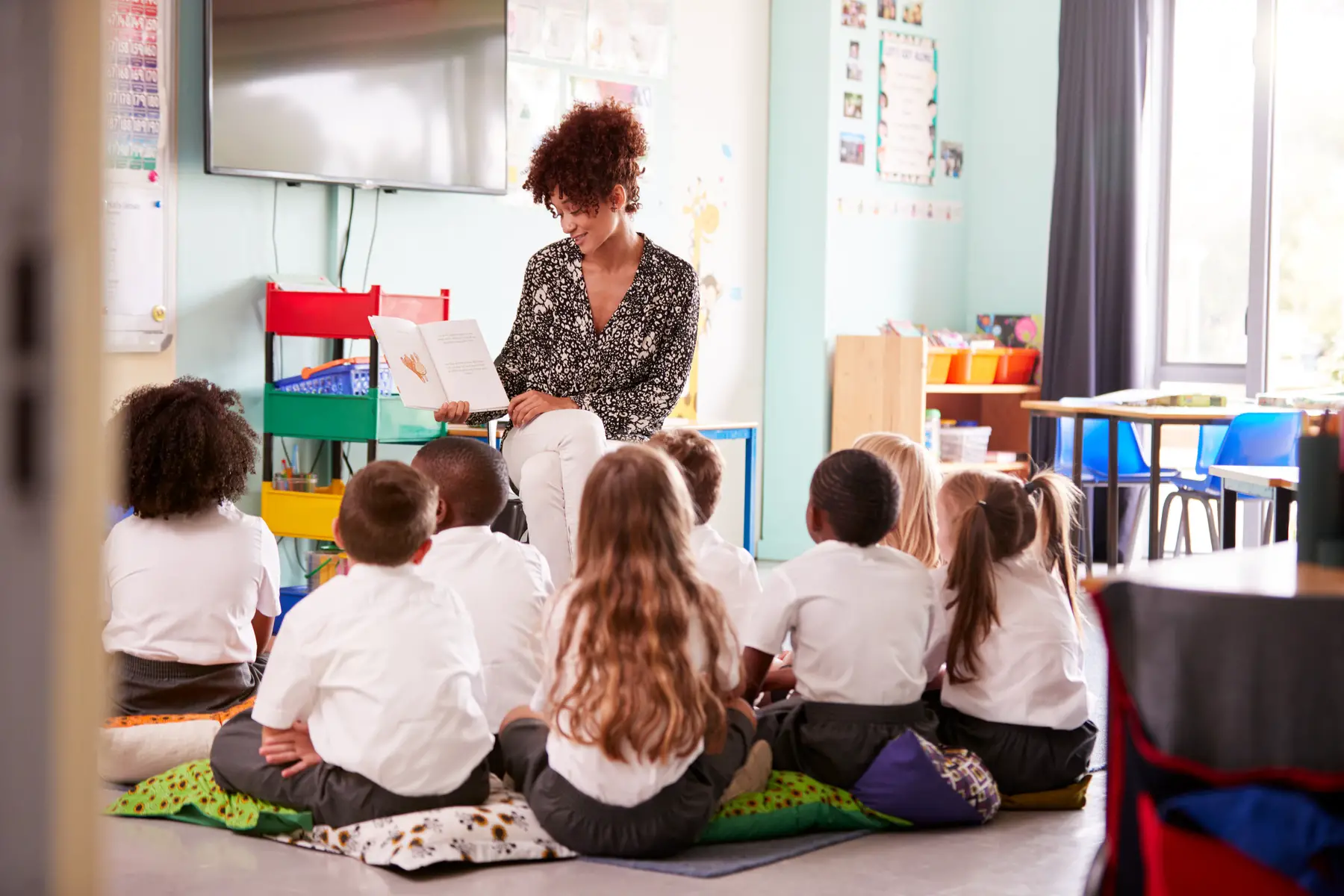
Religious and method schools, such as Montessori and Steiner-Waldorf, are independent in Portugal, and fees usually start at around €4,000 a year. However, apart from the international alternatives, most of these schools only teach in Portuguese.
Secondary education in Portugal
Once they have completed primary education, students must apply to a secondary school in Portugal . These are known as escolas secundárias and are mandatory for 15 to 18-year-olds. The Ministry of Education ( Ministério da Educação ) is responsible for overseeing secondary education in the country.

Secondary schools in Portugal
Secondary education lasts for three years and includes Grades 10, 11, and 12. When enrolling in secondary school, students can choose several stream options that suit their interests or career objectives. While some courses focus on further academic pursuits (i.e. preparing students for university), others are tied directly to work placements. Additionally, many schools set aptitude tests and provide guidance at the end of primary school to help students choose the most appropriate track.
Public secondary education ( Ensino Secundário ) is free in Portugal, and the quality is generally good. Students must study core subjects, such as Portuguese, philosophy, physical education (PE), and a foreign language. However, the rest of the curriculum will depend on their chosen stream.
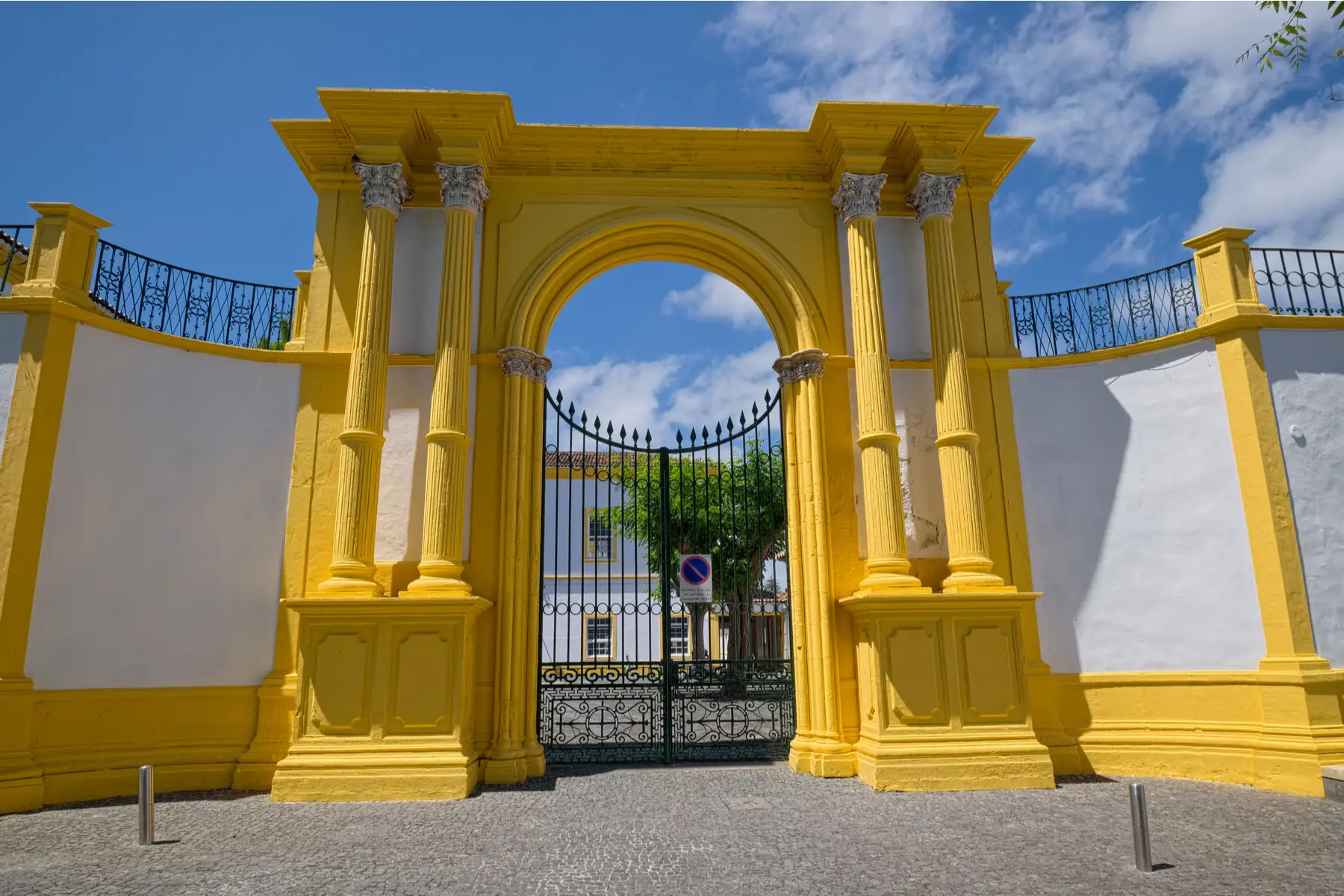
As such, students can choose between vocational ( Cursos Profissionais ) or science-humanities streams ( Cursos Científico-Humanísticos ). Those following the Cursos Profissionais study to achieve trade qualifications, gaining direct access to careers in fields such as IT, design, or hospitality. Meanwhile, Cursos Científico-Humanísticos streams are more academic and include subjects like visual arts, sciences, and humanities. Students looking to pursue a career in a creative field can also choose a third option and take a specialized artistic course ( Cursos Artísticos Especializados ).
Private secondary schools in Portugal
In Portugal, you can choose between different types of private schools, including religious, international, and boarding options.
Most private secondary schools in Portugal are Catholic institutions. Alongside the core curriculum, pupils also study religious subjects. Many parents choose these schools because they perform better in the annual exams (in Portuguese) and offer a wider range of extramural activities. Some schools also teach in English and students can graduate with the International Baccalaureate (IB) diploma. School fees can range between €2,500 and €10,000 a year.
Expats living in Portugal often prefer the multicultural environment that an international school can provide. Parents can choose between British, American, French, and German schools, which is ideal if they prefer their child to learn in their home language or be familiar with the curriculum.
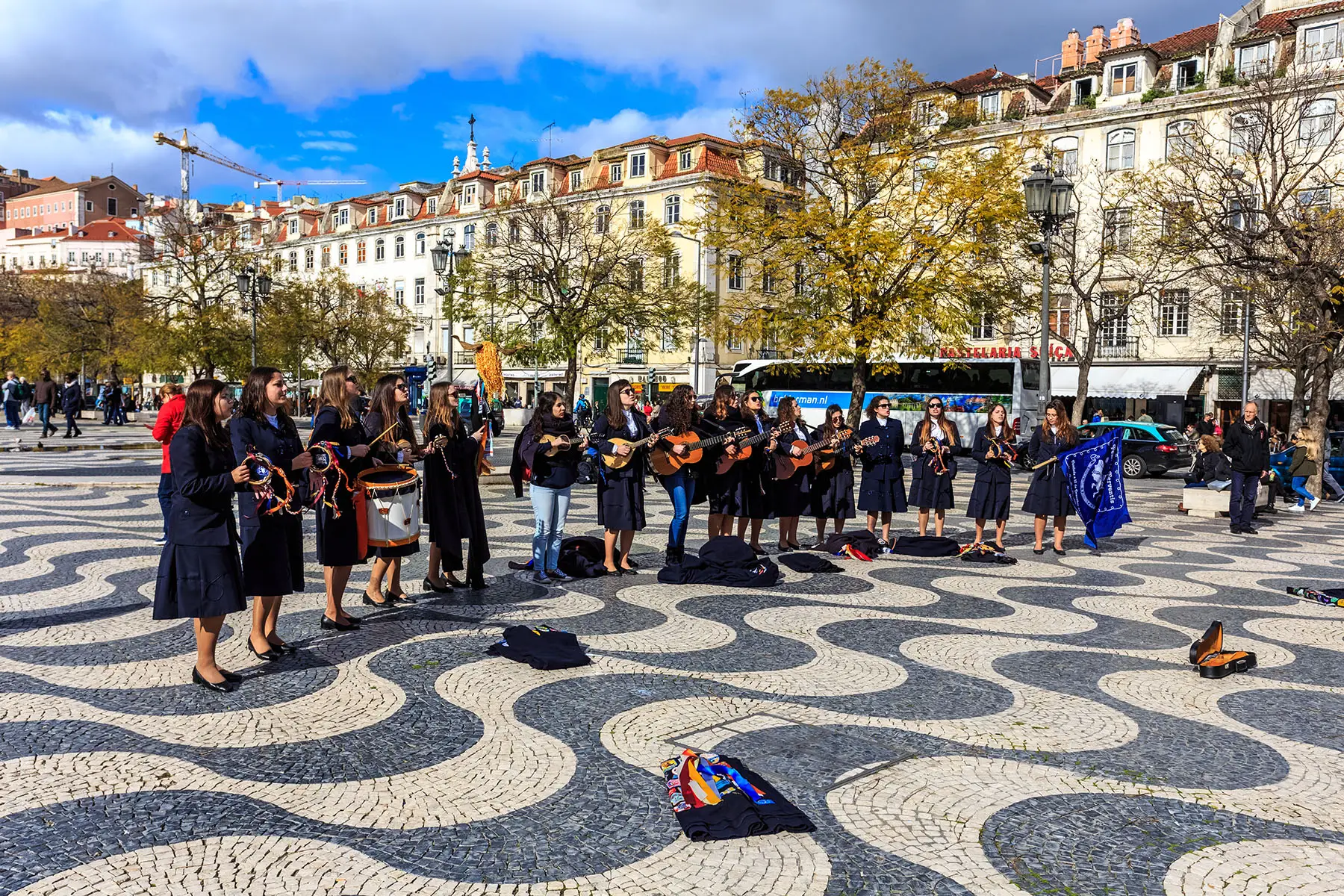
International schools in Portugal
Fortunately, international schools fees are relatively affordable in Portugal and start at around €5,000 a year.
There are only a few boarding schools in Portugal and these are mostly located around Lisbon and the Algarve. Before applying, it is advisable to visit the school to explore the facilities and get a feel for the program. Tuition fees are typically around €30,000 a year.
Students can graduate from secondary school with the International Baccalaureate (IB) . Globally, universities recognize this qualification. The course is designed to develop children’s creativity and sense of community. The IB Diploma is available at 14 schools in Portugal, which include international schools and a few private Portuguese institutions.
Students following the national curriculum must pass all their subjects to graduate from secondary school. Written assessments, class participation, and homework all count towards the final grade. They must also take three mandatory exams: two at the end of Grade 11 and one at the end of Grade 12. Students studying vocational courses, on the other hand, don’t need to sit these final exams to graduate. However, if they want to apply to a university, they will need to take them.
Children from low-income families can apply for government support in Portugal. Parents can request financial aid, which is known as Ação Social Escolar (ASE) , through their school. They can also apply for a family allowance ( abono de família ).
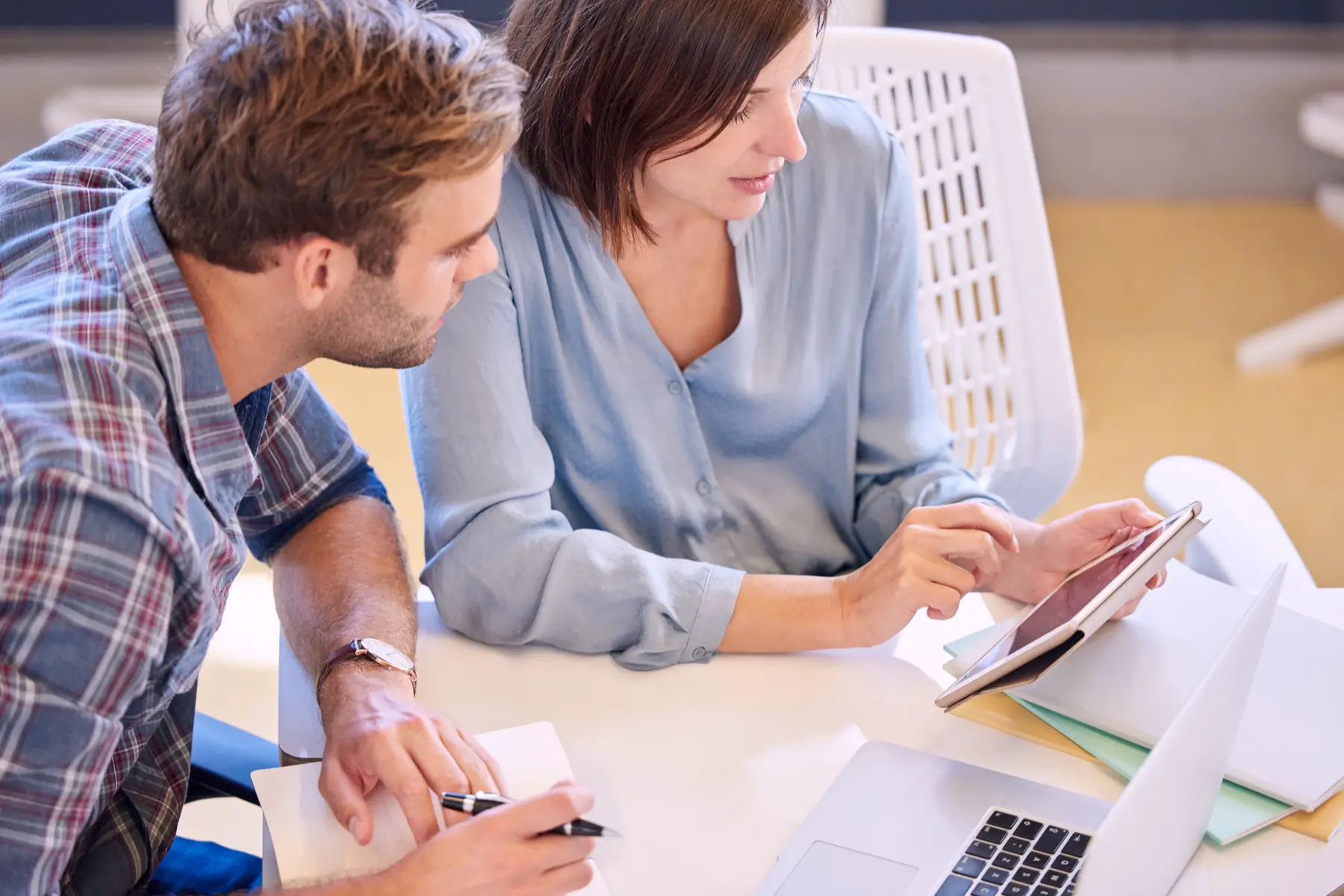
Financial support is further available for children with disabilities, and families can receive at least one of the following subsidies:
- Disability bonus ( Bonificação do abono de família para crianças e jovens com deficiência )
- Special schooling subsidy ( Subsídio de educação especial)
- Support for assistance by a third person ( Subsídio por assistência de 3ª pessoa )
These schemes help cover the costs of education, therapy, and special carers. You can claim any of these benefits through the Portuguese social security system ( Segurança Social ).
Students attending private or international schools may apply for grants to help cover their tuition fees. Parents should contact the school directly for specific details about scholarships.
International children who don’t yet speak Portuguese may find attending a local school overwhelming. However, many state schools offer language support classes. Students can also take the final Portuguese exams adapted for non-native speakers.

Where to learn Portuguese
Unfortunately, not every school has the means to provide this support, especially in rural communities. Therefore, it is a good idea to check the resources available for international learners before enrolling your child in a state school. Alternatively, you might want to consider schools that offer bilingual programs .
You can contact the High Commission for Migration ( Alto Comissariado para as Migrações – ACM ) with any education-related questions. They aim to help migrant families integrate into Portuguese society .
Portugal wants to create an inclusive educational system ( educação inclusiv a). As a result, most mainstream schools offer special educational needs (SEN) programs. Moreover, the Ministry of Education has issued a list of state schools (in Portuguese) that actively promote inclusion.
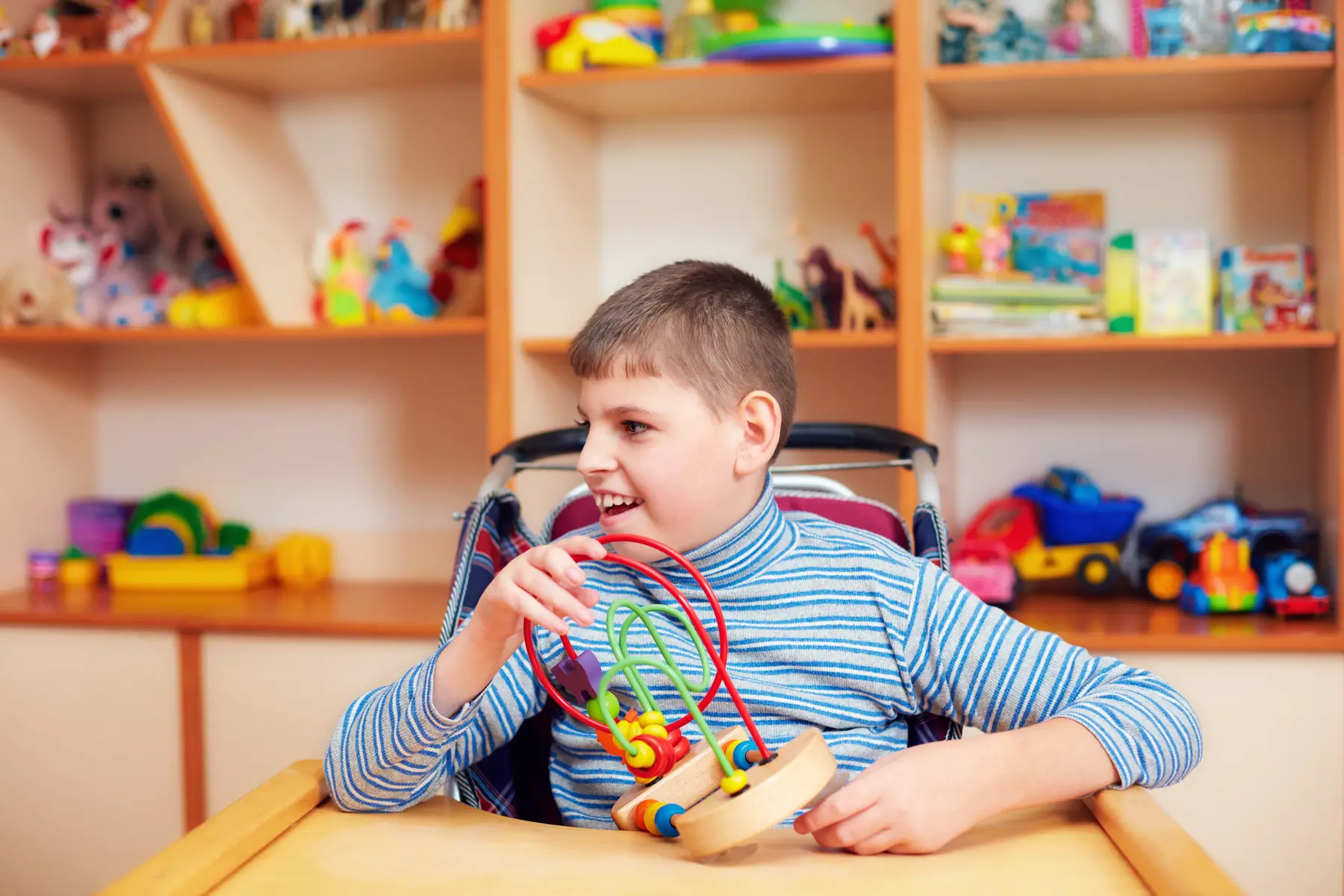
Children under the age of six can study at home or in a kindergarten with the help of a special education teacher. After that, they can attend their local primary school. At this point, they receive an Individual Educational Plan (IEP) which is adapted to their learning needs. For example, it can include specific learning tools or special conditions for exams. Children also receive a plan at the end of school to help them transition into adult life. However, if the plan and resources at the mainstream school are not sufficient to meet their needs, they could also consider a specialist school.
Special education schools are available in most big cities in Portugal. For instance, there are facilities for children who have hearing or visual impairments or developmental disabilities. Furthermore, specific organizations can provide additional support. For example, CADIn is a non-profit organization that helps families whose children have neurodevelopmental disorders.
One requirement for applying to a public school is that it must be near your work or home . Still, children can change schools at any time during the year. Naturally, you need to justify this transfer, such as a change of address. Notably, many parents wait until the end of a cycle to ease the transition.
There is no cost involved when it comes to changing schools in Portugal. Parents can apply via the Portal das Matrículas if they have a Portuguese citizen card or directly with the school. However, you should contact the school board for private and international schools first.
If you want to be more involved with your child’s school, you can join a Parent Teacher Association (PTA). These are known as Associação de Pais , and most schools have one. However, the meetings are mainly in Portuguese, which can be challenging if you are not fluent in the language. International schools also have similar groups, and some allow you to join the Board of Governors.
Although homeschooling is legal in Portugal, it is not a popular choice. In fact, only 568 students (in Portuguese) enrolled in homeschooling or individual teaching from 2019 to 2020. The difference between homeschooling and individual teaching is that a tutor (classed as individual teaching) teaches a child instead of a relative (classed as homeschooling).
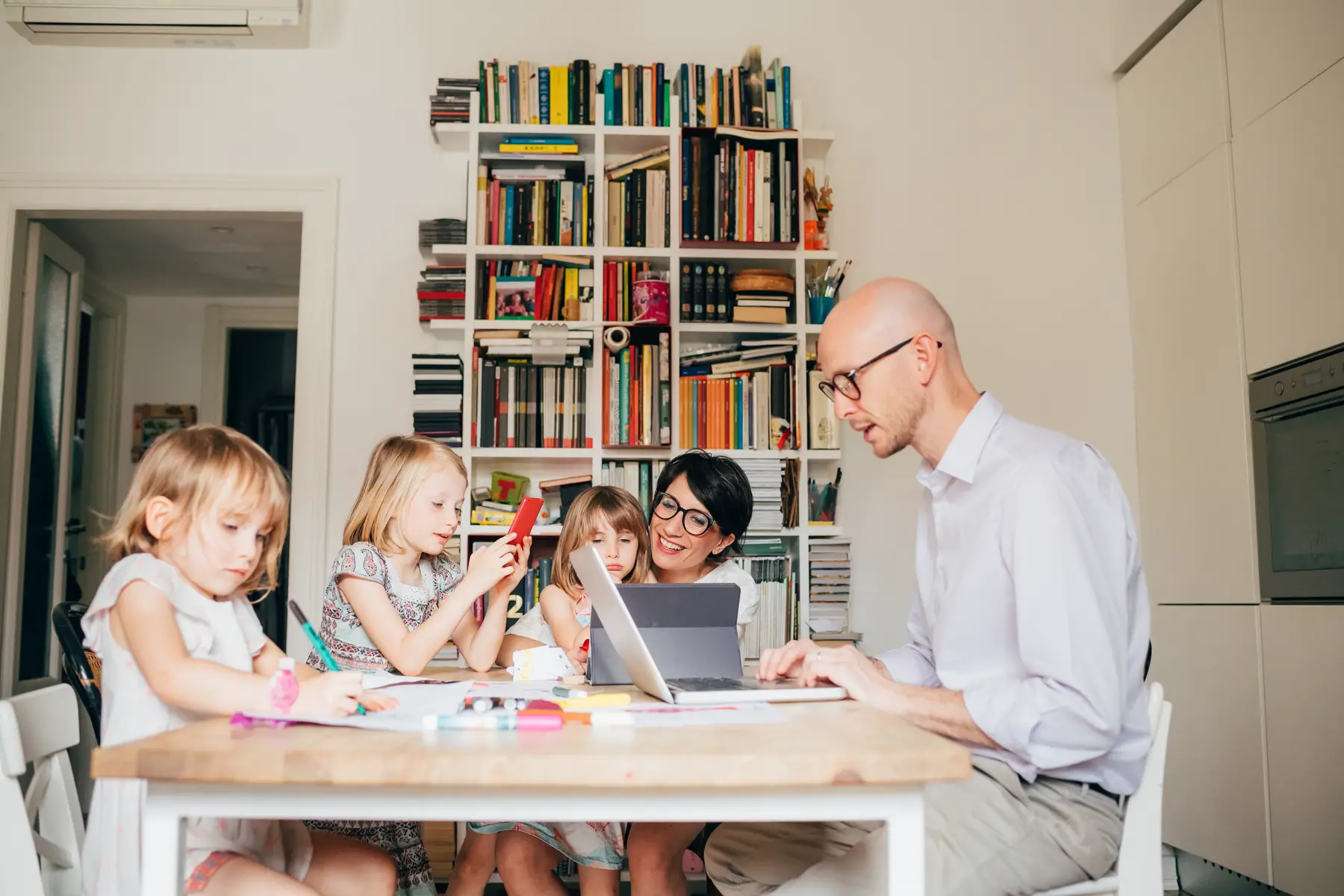
Notably, recent reforms have made it more difficult to homeschool in Portugal. For instance, parents or legal tutors must have a university degree and live in the same house as the child. Children must also be registered at a public school and follow the national curriculum as external students. This includes sitting the annual exams, which are in Portuguese. For more information, you can visit the website of Movimento Educação Livre (MEL) , which is one of the leading homeschool associations in Portugal.
- Direção-Geral da Educação (DGE) – the Ministry of Education, provides information about the school curriculum
- Eurydice – the European Commission, offers information about the Portuguese education system
- Alto Comissariado para as Migrações (ACM) – an organization that supports children from migrant families
- Associação Nacional de Apoio à Criança Especial (ANACE) – a non-profit association that helps children with special educational needs (SEN)
- Doutor Finanças (in Portuguese) – provides information on how to apply for financial school aid in Portugal
- Ericeira Living – answers frequently asked questions about homeschooling in Portugal
Related Articles
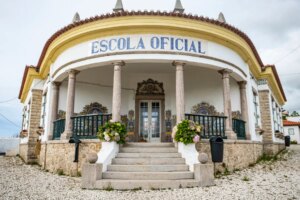
Lease agreement
This badge is awarded to listings where the previous tenant(s) experienced a smooth move-in and move-out, as evidenced by either a positive review or no bad review from them, indicating a smooth handover of the rental unit to the landlord.
The badge is awarded to listings where a Flatio team member was physically present, whether to take photos, create a virtual tour, or stay in the rental unit for various reasons – either alone or with friends and family.
StayProtection for Tenants is a Flatio coverage that applies to you at all times. It was designed to protect you, your money, and your peace of mind. StayProtection also includes an exclusive Move-in Guarantee, ensuring you'll have a place to stay even if things don't go as planned.
Stay Benefits is a special part of the StayProtection coverage which doesn't come with all Flatio properties. They include liability insurance, assistant services, and 24/7 online support for all situations covered by this package.
You can read more about the StayProtection and Stay Benefits packages and when they are applicable on this page .
This badge is awarded to properties that have been inspected by a Nomad Inspector and verified as suitable for remote work in terms of Wi-Fi speed, workspace, location, etc. A Nomad Inspector is a digital nomad influencer who is a member of Flatio's Nomad Inspectors Club.
In our comparison, we mainly considered platforms that have a similar focus as Flatio and are also suitable for medium- or long-term stays. In particular, we analyzed data from Booking.com, Spotahome, Homelike and Uniplaces.
A guide to Portugal education system - schools, universities, Erasmus and more
Are you considering studying abroad? Think of Portugal — a country with over 200 days of sunshine in its capital Lisbon . Portuguese universities are known for quality education in a friendly environment, making them a great choice for those looking to study in Portugal.
In addition to teaching, the country also offers beautiful landscapes, good food and even activities such as surfing. This makes it an ideal place for those who want to study in Portugal themselves or enroll their children in a school in Portugal.
In this guide, we cover important aspects such as:
- English-speaking schools
- Public schools
- Private schools
International schools
- Higher education
- Top universities
- Cheapest universities
- Student visa
- Learning Portuguese
- Cost of living
- Accommodation
Portugal education ranking
Portugal is widely recognized for its high-quality education system . The government has made substantial investments in schools and universities to uphold and sustain an excellent standard.
In the 2023 Best Countries for Education rankings by U.S. News, Portugal holds the 24th position . These rankings are based on a global survey involving over 17,000 participants , reflecting respondents' perceptions of countries with respect to various factors.
Criteria include the presence of a robust public education system, the desirability of attending university in that country, and the existence of high-quality universities .
Portugal education system
Some technical details, just in case you want to compare the Portuguese education system with that of your country.
The education system in Portugal follows the Basic Law of the Educational System , split into:
- higher education
Higher education in Portugal has two parts: university and polytechnic education, taught in public and private schools.
University education includes universities and similar institutions, while polytechnic education includes polytechnic institutes and related schools
In 2005, the education law was updated to follow the Bologna Process , bringing in the European Credit Transfer System (ECTS) and other changes. Higher education now has three study cycles, namely bachelor's , master's , and doctoral studies.
This new system began in 2006 and was fully in place in Portugal by the 2009/2010 school year.
The education system in Portugal is overseen by the Ministry of Education (Direção-Geral da Educação – DGE), covering pre-school through secondary school.
Private schools in Portugal require approval from the Ministry of Higher Education .
School groups (agrupamentos escolares) collaborate with local municipalities to allocate funding and establish the curriculum. Policies and budgets for higher education are managed by the Ministry of Science, Technology, and Higher Education (Ministério da Ciência, Tecnologia e Ensino Superior – MCTES ).
English-language schools and programs
In this section, you’ll find information on whether it’s possible to study in Portugal in English. In Portugal, public schools primarily use the Portuguese language , but some provide language support classes for expat children. You might consider international schools in Portugal if your child is older. Private schools have various language programs, with many using English as a primary language. Look into schools offering bilingual programs for more options.
For higher education, most bachelor's level courses in Portugal are conducted in Portuguese. Yet, at the graduate level, you'll find numerous programs taught in English , aligning with patterns seen in European universities. Further information can be found further down in this article.
Explore more : How to Become a Digital Nomad with a Family
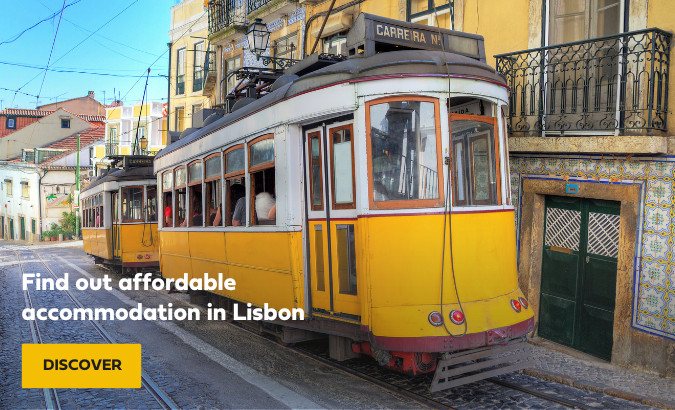
Structure of education in Portugal
In this section, the Portugal school system is explained. In Portugal, you have the option of enrolling in a private or a public school . If you choose a private school, make sure that it is certified by the relevant ministry .
Whether you go for a state or private school, the education system in Portugal follows the same structure, consisting of four stages with these educational levels:
- Pre-school education (Educação Pré-escolar): Ages 3 to 6
- Primary education (Ensino Básico): Ages 6 to 15
- Secondary education (Ensino Secundário): Ages 15 to 18
- Higher education (Ensino Superior): Ages 18 and above
School is mandatory from ages 6 to 18 . After this, students can decide whether to continue to university. Even in international schools, it's recommended that children learn Portuguese . The state provides free language courses and support to help them become familiar with Portuguese from a young age.
School year and hours
The school year in Portugal begins in mid-September and ends in mid-June . And if you’re wondering how long a school day is in Portugal, you should know that the school hours in Portugal vary based on factors such as the chosen school (public, private, or international schools), the educational stage (primary or secondary), and your child's involvement in extracurricular activities.
Typically, schools start between 8:30 and 9:30 and conclude around 16:00 or 18:00 . Each school day must consist of five hours, including breaks.
School holidays in Portugal
The Ministry of Education establishes the dates for school holidays in Portugal. While general holiday dates are consistent nationwide, there might be variations in additional holidays or days off between regional districts and schools. To get specific information, you can inquire with your individual school or the regional educational authority ( Direção Regional de Educação in Portuguese ).
Private international schools in Portugal may have their own holiday schedules, potentially differing from the general dates. It's advisable to check with your child's school for the specific holiday dates.
Typically, Portuguese schools observe four main holidays throughout the year:
- Christmas holidays (Os feriados de Natal): Two weeks off
- Mardi Gras carnival (Feriados de carnaval): A few days off in February/March
- Easter holidays (Férias da Páscoa): Two weeks off
- Summer break (Férias de verão): Between June and September
Additionally, there may be some extra days off for Portuguese public holidays and other school-related activities.
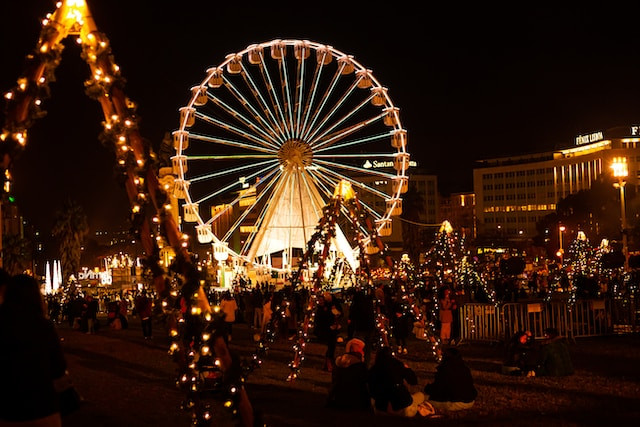
Pre-school education in Portugal
Pre-school education, or Educação Pré-escolar, is optional for children aged three to five and is provided by kindergartens in Portugal. The Ministry of Labor, Solidarity, and Social Security (Ministério do Trabalho, Solidariedade e Segurança Social) oversees these institutions, most of which are privatized. Parents can choose between kindergartens ( creches ) and childminders ( amas ) who come to their homes.
Find the perfect accommodation in Portugal
Free pre-school education (educação pré-escolar) is available for 25 hours per week from age three . However, private pre-schools, including international schools, Montessori, and Waldorf institutions, charge tuition fees.
The following information is for those looking for a Waldorf school or a Montessori school in Portugal:
- The Montessori schools in Portugal use the Montessori educational approach. It focuses on children's natural interests and activities, emphasizing hands-on learning and the development of real-world skills. In a Montessori school, formal teaching methods take a backseat.
- The Waldorf schools in Portugal are based on Waldorf education, also known as Steiner education, which follows the philosophy of Rudolf Steiner, the founder of anthroposophy. This holistic educational style aims to cultivate students' intellectual, artistic, and practical skills, with a particular emphasis on fostering imagination and creativity.
A 2022 government initiative made all social sector daycare centers free for children born on or after September 1, 2021 , covering basic education, food, insurance, and physical education, with no tuition fees. The scheme aimed to benefit 100,000 children by 2024, irrespective of family income.
While optional, pre-school is chosen by working parents for its convenience and its role in preparing children for primary school, both socially and psychologically.
Unfortunately, there is a shortage of spaces in creches for children under the age of three, with waiting lists for government-subsidized creches being challenging. Pre-registering is recommended , although it doesn't guarantee a spot, and choosing a private pre-school is suggested to avoid long delays.
Explore more : How Digital Nomad Families Can Provide Their Children with the Best Academic Support
In Portugal, primary education consists of nine years, organized into three cycles lasting four, two, and three years respectively. All children aged six by September 15 must be enrolled in school for that calendar year. Primary school is also referred to as elementary school.
The Ministry of Education oversees policies for state schools, and these are three primary cycles (escolas básicas):
- First cycle (primeiro ciclo or 1º ciclo): Grades 1 to 4 - This initial four-year phase has a single teacher responsible for teaching students.
- Second cycle (segundo ciclo or 2º ciclo): Grades 5 to 6 - This two-year cycle involves different teachers teaching various subjects.
- Third cycle (terceiro ciclo or 3º ciclo): Grades 7 to 9 - Lasting three years, one teacher is responsible for teaching specific subjects, including vocational studies. A diploma/certificate is awarded at the end of this cycle.
Public primary schools in Portugal
Many locals choose public schools in Portugal because they are tuition-free . Parents only need to cover minor costs like books, school meals, and occasional field trips.
The national curriculum is consistent across public schools, including subjects like:
- mathematics
Starting from Grade 7, students can also choose another foreign language , usually French or Spanish.
It's essential to select a school near your home or work and provide proof of address during the application. Waiting lists, especially for popular schools, exist, so early registration is recommended .
While Portugal's public schools have a great reputation, expatriate parents may opt for international schools or learn Portuguese to assist their children in becoming fluent in the language .
Private primary schools in Portugal
Private schools in Portugal provide an opportunity for children to learn in their native language while engaging with peers globally, ensuring educational continuity if they relocate . These schools offer benefits like smaller class sizes, robust extra-curricular activities, and modern facilities. Some even provide boarding options .
International schools maintain high education standards and diverse curricula . Admissions processes vary, with some requiring entrance exams, school records, and personal interviews. Many parents choose private schools, confident that their children will receive a top-notch education, preparing them to compete globally in university-level higher education.
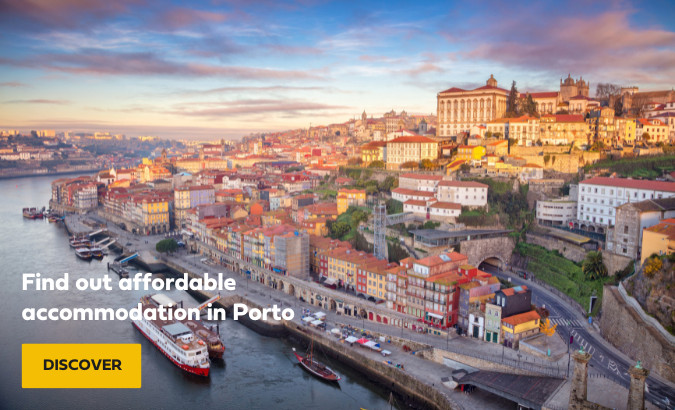
Secondary education in Portugal
Compulsory secondary education in Portugal aims to advance children's knowledge and build on the skills acquired in primary school. This phase is crucial as it prepares students for higher education and the job market.
Spanning three years from Grade 10 to Grade 12 , entry requires a certificate of primary school education. Secondary education includes general, artistic, and vocational or technical courses.
To successfully complete secondary education, students must pass all subjects . Internal assessments contribute 70 percent to the final grade, with the remaining 30 percent based on four mandatory national exams.
Grade 11 involves two finals (based on the scientific/humanities courses chosen), and Grade 12 includes exams in Portuguese and another subject of the student's choice.
Successful completion results in a diploma and certificate , and the national exam results are vital for third-level education admissions.
Public secondary schools in Portugal
Public secondary education (Ensino Secundário) is free in Portugal and generally of high quality . Core subjects, including Portuguese, philosophy, physical education (PE), and a foreign language, are compulsory . However, the rest of the curriculum depends on the chosen educational stream.
Students can opt for either vocational ( Cursos Profissionais ) or science-humanities streams ( Cursos Científico-Humanísticos ). In the Cursos Profissionais, students focus on gaining trade qualifications, opening pathways to careers in IT, design, or hospitality.
On the other hand, Cursos Científico-Humanísticos streams are more academically oriented , covering subjects like visual arts, sciences, and humanities. For those interested in pursuing a creative career, there's a third option : taking a specialized artistic course (Cursos Artísticos Especializados).
Private secondary schools in Portugal
Find the perfect accommodation in Lisbon
Independent organizations , including religious orders or private companies, manage private secondary schools in Portugal. These schools typically adopt a more personalized teaching approach , featuring smaller class sizes and increased individual attention from teachers. In return for tuition fees, private institutions usually have access to more resources than public secondary education institutions, offering modern classrooms and technology-based learning tools.
Private schools are commonly regarded as an effective means for students to ready themselves for higher education and pursue their chosen career paths. They create a more challenging academic environment, aiding students in developing the skills and knowledge necessary for future success .
Religious schools
Religious schools in Portugal, managed by religious orders like the Catholic Church, emphasize a robust moral and religious education . They impart knowledge about faith and emphasize its significance in their lives. In addition to core subjects like Math, Science, and Languages, religious educational institutions delve into the history and culture of the students' faith, illustrating its influence on their lives . These schools offer a diverse range of extracurricular activities .
For expats in Portugal , international schools stand out as a top choice . International schools in Portugal create a multicultural environment, offering global skills and the opportunity for students to engage with diverse cultures while learning in their native languages.
Parents can choose from British , American , French , and German schools, making it ideal for those who prefer their child to learn in their home language or follow a familiar curriculum. There are also adaptive learning schools and various schooling options, ensuring a range of choices.
International schools play a crucial role in the public or private school discussion, especially for expat families. Moreover, the fees for Portugal international schools are relatively affordable compared to other European countries, starting at around €5,000 per year .
Among the notable international schools in Portugal are:
- St. Julian’s School in Carcavelos
- United Lisbon International School in Lisbon
- The Carlucci American International School of Lisbon in Sintra (This is a good option for those looking for an American school in Portugal.)
- Oporto British School in Porto
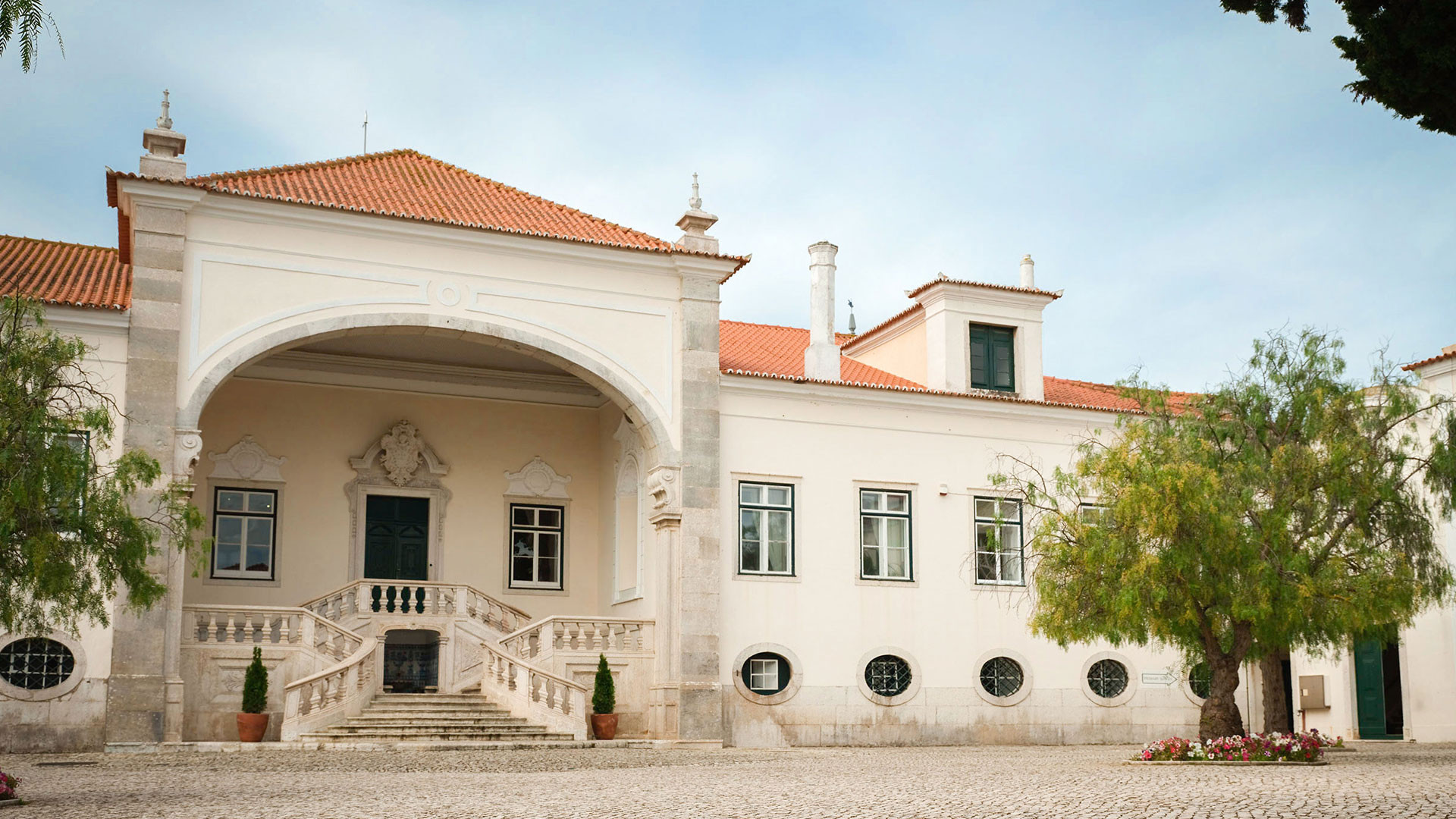
Boarding schools
For families seeking boarding schools in Portugal, there are distinguished institutions , each tailored to the individuality of its students. These schools are relatively scarce in Portugal , mainly situated near Lisbon and the Algarve.
It is recommended to visit the school before applying to familiarize yourself with the facilities and the program. Tuition fees generally hover around €30,000 per year .
Explore more : How Can Digital Nomad Families Find Private Teachers for Their Children
International Baccalaureate (IB) in Portugal
In Portugal, the International Baccalaureate (IB) is a globally recognized pre-university curriculum offered mainly in international secondary schools. This program, taught in English, prepares students for higher education and results in an IB diploma upon secondary school graduation.
The IB curriculum, available in both public and private schools in Portugal, fosters creativity and a sense of community. It is often viewed as an alternative to the traditional national education system , providing students with a comprehensive and well-rounded education. Recognized worldwide, the IB Diploma Program is a challenging two-year curriculum, emphasizing critical thinking, intercultural understanding, and independent research.
The IB Diploma is available at at least 12 schools in Portugal, encompassing, including both international and private Portuguese educational institutions.
Higher education in Portugal
Higher education in Portugal is well-regarded worldwide and is divided into two subsystems:
Universities
In Portugal, universities provide degrees at the bachelor's , master's, and doctorate levels, focusing on theoretical education and research . The country has both private and public universities, with private ones obviously being more expensive.
Higher education institutions have unique admission processes for sportspeople, mature applicants over 23, international students, and those transferring from other educational institutions. Portugal boasts some of Europe's oldest universities, like the University of Coimbra founded in 1290 .
A noteworthy feature of Portuguese universities is their emphasis on internationalization. Many have global partnerships, allowing students to study abroad and gain a worldwide perspective. Additionally, many universities offer programs in English, making them accessible to international students .
In terms of programs and specializations, Portuguese universities provide a broad range of options , from traditional fields like law and medicine to specialized areas like renewable energy and biotechnology.
Polytechnics
Polytechnic institutes in Portugal offer both undergraduate and master’s degree programs, emphasizing education and training in specific fields, making them ideal for vocational courses like engineering and nursing.
These institutes are distinguished for their hands-on learning approach, providing a wide array of technical courses and vocational programs in areas such as engineering, design, and technology. Notably, they focus on project-based learning, encouraging students to apply their skills to real-world challenges, fostering critical thinking, problem-solving, and teamwork.
The curriculum is highly flexible , enabling students to tailor their studies to their interests and career goals. Elective courses are diverse, and students are encouraged to engage in internships and co-op programs for practical experience.
One prominent polytechnic institute in Portugal is the Polytechnic Institute of Porto , renowned for its programs in engineering, design, and technology. The institute is also home to cutting-edge research centers focusing on technologies like renewable energy, smart cities, and sustainable transportation.
Scholarships and financial aid
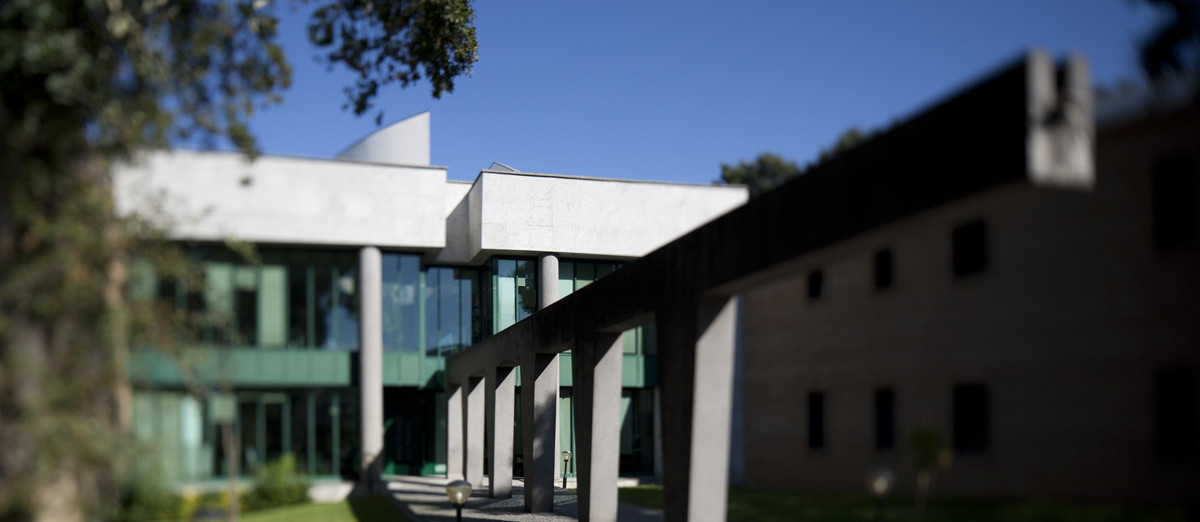
Students, both local and international, have access to various Portugal scholarship programs offered by the government and higher education institutions.
Find the perfect accommodation in Porto
These are some of the institutions that offer scholarships or funding:
- DGES : It offers scholarships, including merit scholarships and student loans at public and private universities.
- FCT : It offers various funding opportunities for doctoral students.
- Calouste Gulbenkian Foundation : It offers scholarship opportunities to graduate students researching in Armenian studies and for students involved in research projects on Portuguese language and culture.
As an EU member, Portugal participates in EU-based scholarships such as Erasmus Mundus programs . Additionally, private and semi-private organizations offer scholarships covering studies in Portuguese language and culture, sciences, and technology.
For children from low-income families, government support is available. Financial aid, known as Ação Social Escolar (ASE), can be requested through the school. Families can also apply for a family allowance ( Abono de família ).
The Portuguese government provides financial support for children with disabilities.
Through the social security system ( Segurança Social ), families can apply for subsidies such as:
- Disability bonus (Bonificação do abono de família para crianças e jovens com deficiência)
- Special schooling subsidy (Subsídio de educação especial)
- Support for assistance by a third person (Subsídio por assistência de 3ª pessoa)
Various financial support options are available for covering school tuition fees. Parents can discuss their low-income status with school management to explore suitable options. It's important to note that private universities are generally more expensive than public universities.
Erasmus in Portugal
If you're planning a short-term stay in Portugal , you may want to consider the long-standing Erasmus initiative , which has been helping higher education students travel for 30 years.
If you're from the European Union, Iceland, Liechtenstein, Norway, Turkey, or Macedonia, check your institution's agreements with Portuguese universities. Erasmus can support your studies or internships in Portugal, lasting from two to 12 months in each study cycle and for recent graduates.
If you're from other countries, you might also benefit from the International Credit Mobility. Get details from your institution.
Introduction to Erasmus Portugal
How to apply.
Consult your home university's Erasmus office and inquire about partner universities in Portugal offering courses of interest. Once you've made your selection, apply to the universities you're interested in.
Upon acceptance, kickstart your preparations for your Erasmus experience in Portugal.
Best cities for Erasmus in Portugal
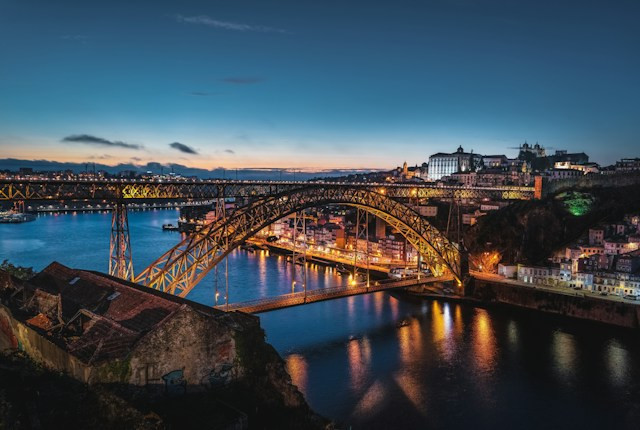
Erasmus in Lisbon
Lisbon, the capital, is known for its dynamic and student-friendly atmosphere. The city offers remarkable scenery, distinctive architecture, and an active nightlife.
Erasmus in Porto
Porto, often designated as Portugal's second city, is located along the Atlantic Ocean and is marked by a distinctive character. The presence of a high-caliber university, amicable locals, and a lively nightlife enhances Porto's appeal as a destination for Erasmus participants.
Erasmus in Coimbra
Coimbra , home to Portugal's oldest university, is a historically significant city with well-preserved ancient structures. It serves as a focal point for students, offering a scenic backdrop for academic pursuits and exploration.
Erasmus in Faro
Faro , the capital of the Algarve region in the south, stands out as a noteworthy Erasmus destination. In addition to its scenic beaches and resorts, Faro serves as a gateway to the Ria Formosa Islands, a natural park that adds to the city's appeal for students wishing to explore the southern region of Portugal.
Top Erasmus universities in Portugal
You can consider these well-known universities for your Erasmus experience:
- University of Lisbon
- Nova University of Lisbon
- University of Porto
- University of Coimbra
- University of Algarve
Best time for Erasmus in Portugal
The second semester, usually from February to mid-June, is ideal to go on Erasmus in Portugal. As winter gives way to warmer temperatures, this period allows for ideal exploration without the usual large crowds of tourists.
Pros and cons
Disadvantages.
- Transportation challenges : Outside major cities, transportation may be challenging, especially during rush hours.
- Bureaucracy : Portugal's bureaucratic processes might require extra paperwork.
- Classes in Portuguese : Although some courses are taught in Portuguese, they also offer a unique opportunity to learn the language.
- Affordability : Portugal is a beautiful and cost-effective country to explore.
- Rich culture : You can immerse yourself in a culturally rich experience.
- Welcoming schools : Portuguese universities warmly welcome Erasmus students.
- Safety : Portugal is known for its safety.
- Lifelong connections : You can make lasting friendships during your Erasmus stay because Portugal offers a multinational and multicultural environment.
Educational support for expat students in Portugal
Find the perfect accommodation in Madeira
Portugal welcomes expats and their children , and certain schools provide support classes for students unfamiliar with the Portuguese language. However, it's important to note that this language support may not be available in every city; it is more common in larger cities like Lisbon or Porto . Alternatively, you may explore schools offering bilingual programs.
If you are wondering what papers you need to enroll in a high school in Portugal, or if you have other education-related inquiries, you can reach out to the High Commission for Migration (Alto Comissariado para as Migrações–ACM).
Support for children with special educational needs (SEN)
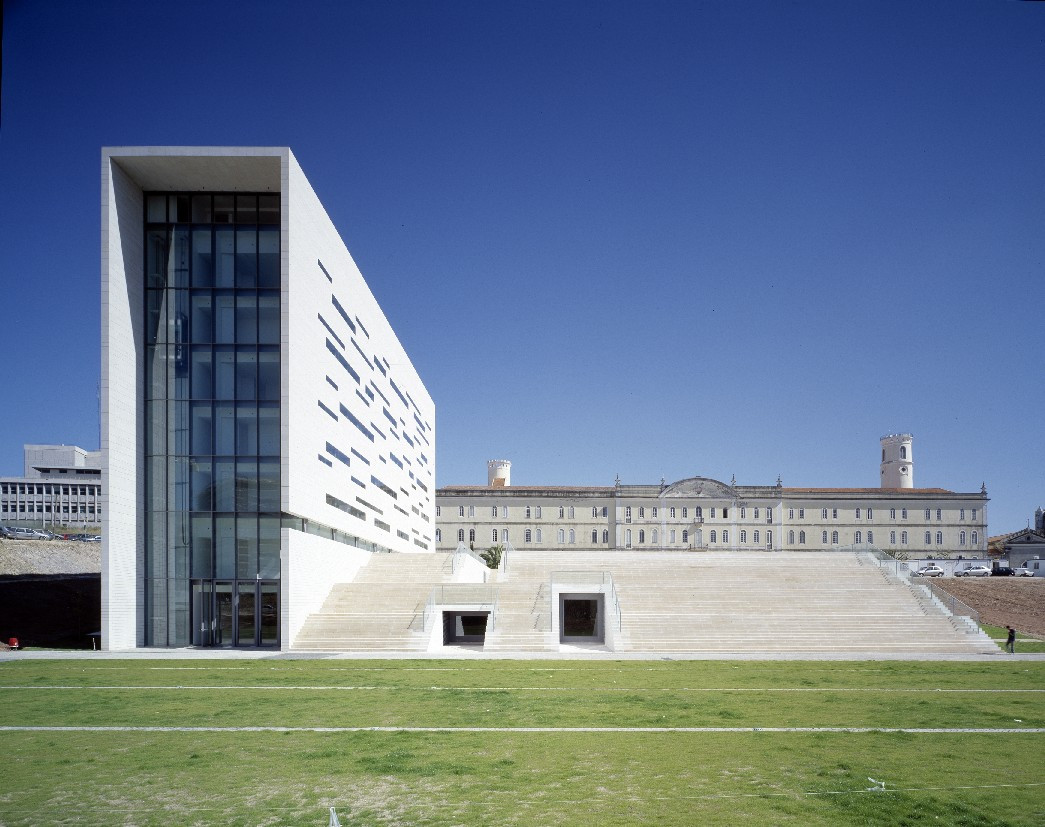
Portugal aims to establish an inclusive educational system (educação inclusive) to ensure equal opportunities for children with special needs. Most mainstream schools offer special educational needs (SEN) programs under this initiative. The Ministry of Education has identified state schools (in Portuguese) actively promoting inclusion.
Children under six can receive specialized teachers at home or attend a kindergarten in Portugal, especially if their parents work. Upon entering primary school, they receive an Individual Educational Plan (IEP) tailored to their learning needs. This plan may include specific learning tools or exam accommodations. At the end of their schooling, children receive a plan to assist their transition into adulthood.
Special education schools are available in major Portuguese cities, equipped to support children with hearing or visual impairments or developmental disabilities. Additional support is provided by organizations like ( CADIn ), a non-profit assisting families with children having neurodevelopmental disorders.
Changing schools, parental involvement, and homeschooling
In Portugal, changing schools is a flexible process , and children can transfer freely. When applying for a new school or switching, providing your home address is a key requirement. Transfers can occur at any time during the year, typically with a valid reason such as a change in residence.
For parents interested in actively participating in their children's school, joining the Associação de Pais - Parent Teacher Association (PTA) is a valuable option. It's important to note that PTA meetings are primarily conducted in Portuguese.
Homeschooling is legally allowed in Portugal but is not widely adopted. A small number of children are enrolled in homeschooling or individual teaching. Most parents opt for traditional schools due to convenience. Homeschooled children must have an individual tutor with a university degree residing in the same house and undergo annual exams conducted in Portuguese.
For further information on homeschooling, you can explore the website of Movimento Educação Livre (MEL), one of the prominent homeschool associations in Portugal.
Portugal student visa
If you're accepted to a Portuguese higher education institution, you'll probably need to apply for a student visa . Here is a quick guide to applying for a student visa in Portugal:
- For courses over three months, a visa is likely needed.
- Non-EU/EEA/Swiss citizens need a student visa. EU/EEA/Swiss citizens need to register their stay.
Documents needed:
- Valid passport (or travel document)
- University acceptance letter
- Proof of tuition payment
- Passport photos
- Completed visa application form (usually provided by the embassy)
- Financial proof
- Accommodation details
- Criminal record certificate
- Schengen travel health insurance (After your arrival in Portugal, you must also take out appropriate health insurance with a private company.)
Application process:
- Contact the Portuguese embassy.
- Schedule an appointment.
- Submit required documents.
- Wait for processing (weeks to months)
Note: Visit the website of the Portuguese Ministry of Foreign Affairs for more information on the application process.
- Embassy fee : €90
- Residence permit application : €83
- Residence permit issuance : €72
- Note : Check the fees on the official auhotirites’ websites as they are updated regularly.
Family members:
- Dependents can join if financially supported.
- Spouse, children, and dependent adult children qualify.
- Visa valid for three months.
- Residence permit issued for one year, renewable annually. It replaces the visa.
Post-study:
- Can stay if job offer secured.
- Switch to work visa before student visa expires.
Permanent residency:
- Student years don't count.
- Need job offer, temporary residence, then apply for permanent residency after five years.
Work allowance:
- Allowed to work up to 20 hours/week during semesters, full-time during breaks and holidays.
- EU/EEA/Swiss students have no work restrictions.
Dependent visa:
- Family members can study and work if accompanying a Portuguese citizen or working resident.
Researcher visa:
Another type of student visa for Portugal.
For non-EU citizens in doctoral programs or recognized research institutions.
Other Portugal visa options
If you plan to immigrate to Portugal first and then apply to study in Portugal, there are a few other visa options you can consider.
The following articles provide information on Portugal immigration policy and Portugal immigration requirements, including Portugal visa options, that are available (such as Portugal digital nomad visa known as Portugal D8 visa, Portugal D7 visa , and Portugal D2 visa) or the ones that have changed ( Portugal Golden Visa ):
- Portugal Digital Nomad Visa D8 : How to Apply, Requirements, and Alternatives (D7 and D2)
- Portugal Takes Steps to Ease Housing Crisis : Portugal Golden Visa to Be Scrapped, Airbnbs to Be Restricted
Best Portugal universities for international students
Here’s a list of the best universities in Portugal for international students that you can consider if you want to study abroad . Please note that this university list features the best English-speaking universities in Portugal.
- European University of Lisbon
- ISCTE – University Institute of Lisbon
- Polytechnic Institute of Leiria
- Catholic University of Portugal
Cheapest universities in Portugal for international students
If you’re looking for budget-friendly universities in Portugal, here is a list of the most affordable universities where you can study without spending a fortune.
- Escola Superior Náutica Infante D. Henrique
- Fernando Pessoa University
- Higher Institute of Administration and Languages
- Higher Institute of Entre Douro and Vouga
- Instituto Superior Politecnico Gaya
- Lusofona University of Porto
- Politécnico de Lisboa
- Polytechnic Institute of Braganca
- Polytechnic Institute of Coimbra
- Polytechnic Institute of Setubal
- Polytechnic Institute of Tomar
- University of Evora
- University of Minho
- University of the Algarve
Learning Portuguese in Portugal
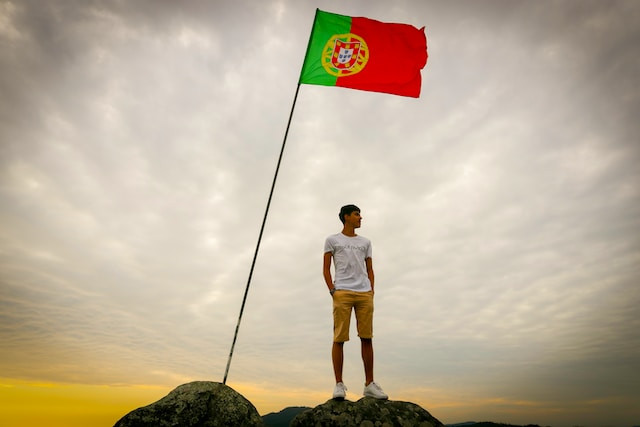
Find the perfect accommodation in Algarve
It’s a good idea to learn Portuguese before or after moving to Portugal, as knowing the language will make it easier to adapt to local life , especially if you're planning a longer stay. This includes tasks such as finding accommodation, organizing utilities, using public transport and shopping in local supermarkets.
Why learn Portuguese?
Portuguese is spoken by over 260 million people worldwide , ranking as the ninth most spoken language . While it's mainly used in Portugal and Brazil, it's also an official language in eight other countries across four continents, including:
- Guinea-Bissau
- Equatorial Guinea,
- São Tomé and Príncipe
In Portugal, 95.6% of the population speaks Portuguese as their native or second language. Learning Portuguese is definitely a valuable endeavor.
Portuguese language schools and programs
Learning Portuguese in Portugal offers several advantages , including access to a diverse range of classes such as conversational lessons, intensive courses, and summer camps in various cities. The flexibility to choose different teachers allows learners to find a suitable match for their preferred learning style.
Additionally, daily practice in real-life settings, such as cafes and restaurants, enables the acquisition of informal Portuguese expressions .
Portugal's universities are excellent options for language learning, with many offering courses for foreigners. These courses provide a chance to immerse oneself in local culture and study in historically significant buildings, such as the Universidade de Coimbra , Portugal’s oldest university. However, it's important to note that university courses may be less flexible in terms of curriculum .
The Instituto de Camões and the Institute for Employment and Vocational Training (IEFP) provide information on universities and centers offering Portuguese language courses. Various language schools across Portugal cater to different needs:
- Alliance Française offers Portuguese courses for expats in locations such as Lisbon, Porto, and Faro.
- Cambridge School provides official certification in Portuguese and prepares students for language exams in cities like Lisbon, Coimbra, Porto, and Funchal (Madeira).
- Home Language International offers immersive experiences by allowing students to live with accredited teachers in Lisbon.
- International House World Organisation has locations in Braga, Coimbra, Torres Vedras, and Viseu, offering flexible schedules for all levels.
- Lusa Language School in Lisbon provides part-time and intensive courses, along with an online learning platform.
- Portuguese Connection, located in Lisbon, offers both online and in-person courses with individual or group options.
The Portuguese government supports language integration through free Portuguese Host Language (PLA) courses under the Português para Todos program. These courses, available to individuals aged 16 or older , offer certification from A1 to B2 levels. Local councils and the Plataforma de Português Online also contribute to language education for expats.
To enroll in PLA courses, individuals can contact local institutions, the Portuguese Language Learning Promotion Office (GLPt), or the Migrant Support Line for information on registration processes. Applicants need a resident permit or specific documents, such as proof of a visa request or Social Security Identification Number (NISS) attribution.
Can you study in Portugal without IELTS?
Many universities in Portugal don't demand IELTS if the student's prior education was in English. Note that in some cases, the university might not require IELTS, but it could still be necessary for the visa application .
Cost of living in Portugal for international students
If you are planning to study abroad in Portugal, here is a brief overview of the costs you can expect in addition to the tuition fees .
Accommodation:
- On-campus housing: €150 to €500 per month.
- Off-campus options (shared/private): €200 to €800 per month.
- Utilities and Internet: They may cost an additional €50 to €150.
Food and groceries:
- Eating out: €5 to €10 at budget-friendly places.
- Monthly grocery bill: Estimated €150 to €250.
Transportation:
- Monthly public transport pass: €25 to €40.
- Walking and biking: They’re very common, especially in smaller cities.
Entertainment and socializing:
- Cinema tickets: €6 to €9.
- Museums: They often offer student discounts.
- Bar and club prices: €1.50 to €5 for drinks.
Student accommodation in Portugal
The common options for student housing in Portugal are either on-campus halls of residence (dormitories) or off-campus accommodation in shared or private apartments or houses.
If you’re looking for accommodation, you may want to consider listings on Flatio, a rental housing platform operating in over 300 destinations in Europe and beyond. In Portugal, we have listings in popular destinations such as:
We focus on monthly stays (rooms, flats, and houses), but short-term rentals are also possible from 5 days . No deposit is required for stays under 180 days unless local laws require otherwise.
Utilities and Wi-Fi are included in our lease agreements, which can be used for visa purposes and as proof of address. We offer a secure payment system, including a Bitcoin option, and daily customer service, including a 24/7 emergency line.
(Sources: U.S. News , DGES , Expatica (1), Expatica (2), Study & Research in Portugal , bePortugal , VisaGuide.World , Beyond Abroad , Jeduka , Study Abroad Aide , and Masters Portal )
What will you get?
€20 discount on your first stay
All the Flatio benefits for free
Special offers for special prices - only for registered people!
Decide which categories of cookies we can use:
We use cookies, including third-party cookies, to operate this marketplace with monthly stays. In addition to the operational ones necessary for the actual functioning of Flatio, we also use preferential, analytical, and marketing cookies. By clicking on Customise Cookies, you can decide on each category or refuse all cookies other than the basic ones. In addition, you can read detailed information on a particular page dedicated to the privacy of our visitors and clients.
Welcome to our list of destinations where we have some amazing accommodations ready for you.
Attention landlords: You have the opportunity to rent anywhere in the world, regardless of the country! Don’t worry if your city is not displayed here - just list your property and we’ll add your destination to our listing. Be the first one to offer a warm and welcoming place to stay in your city!
- North America
- South America
#LeadingSDG4 | Education2030

Transforming Education in Portugal: Empowering a student movement and beefing up digital learning
In the SDG 4-Education 2030 High-Level Steering Committee Sherpa meeting in May 2023, Luísa Ucha, Advisor to the Ministry of Education of the Portuguese Republic, unveiled an inspiring movement that is sweeping through the nation’s schools, empowering students, and making sure they are engaged in reshaping the future of education.
Following their participation in the Transforming Education Summit (TES) held at the United Nations in New York in September 2022, Portuguese students have translated the Youth Declaration into Portuguese thus igniting a nationwide conversation about student involvement in curriculum assessments and management.
Throughout the school year, the Youth Declaration was tackled in school classes, inter-school assemblies led by students of the Portuguese TES School Network (TSN), as well as in activities developed by the students of the Portuguese School Library Network (SLN). The result of this movement is the presentation of the findings and recommendations to the Minister of Education , by a group of 20 student delegates and SDG 4 Youth & Student Network members led by Mariana Antunes, representing TSN, and João Martins, representing SLN, during the Voice of the Students event in June 2023. The event is associated with the recommendations put forth by the Global Youth Initiative to guarantee the engagement of young people in formulating educational policies.
Additionally, with the support of the National Plan for the Arts, a dynamic initiative that has engaged more than half of the schools in the country, an upcoming international conference in November 2023 will provide a platform for student voices to shape cultural engagement.
Complementing the youth engagement efforts, the Portuguese Ministry of Education also made significant strides in digital competency and technology integration. Ucha revealed that every student in public schools now possesses a device, ensuring equitable access to digital tools and facilitating connectivity with teachers and other learners. This investment is not confined to hardware alone; it encompasses a comprehensive training program to empower teachers with the necessary skills to facilitate online learning and assessment.
The multifaceted approach underscores Portugal’s belief in the transformative power of education and the vital role that students play in building a brighter future. As Portugal continues to set an example on the global stage, this movement that encourages students to have a voice and be engaged is not only redefining education but also empowering the next generation to become active contributors to their communities and society at large.
Related items
- Country page: Portugal
- Region: Europe and North America
- SDG: SDG 4 - Ensure inclusive and equitable quality education and promote lifelong learning opportunities for all

Other recent stories

Complete Guide on the Education System in Portugal
For the most part, the education system in Portugal works in a similar way to those of most neighbouring EU nations. There is an abundance of friendly and accommodating private and public schools in Portugal for expats. So it should be fairly easy to find the perfect place to enrol kids of all ages. Come find out more with our complete guide on the Portuguese education system!
- Our Privacy Policy is accepted*
- I agree with the terms and conditions*
Sign up for our newsletter and don’t miss the latest news and offers!
What is the Education System Like in Portugal?
Education in Portugal is regulated by the Ministry of Education (Direção-Geral da Educação – DGE). Mandatory education begins at the age of five/six and continues through secondary school. However, it’s the norm for Portuguese families to send their kids to pre-school before that.
A basic overview of the structure of the education system in Portugal is as follows:
- Pre-school education ( Educação Pré-escolar ): ages 3 to 6;
- Primary education ( Ensino Básico ): ages 6 to 15;
- Secondary education ( Ensino Secundário ): ages 15 to 18;
- Higher education ( Ensino Superior ): ages 18 and above
All children aged 6 to 18 are required by law to attend school, unless there are mitigating circumstances that prevent them to do so. While most standard schools in Portugal conduct lessons exclusively in Portuguese, there are plenty of international schools where children are taught in their native tongues.
Pre-School Education in Portugal
Children aged between three and six can attend pre-school in Portugal. Doing so is not mandatory, but many parents choose to enrol their kids in pre-school education as it is free. Every child is granted 25 hours per week of free education from the age of three, but this does not apply to most international schools. Specialist schools that cater to kids from overseas in different languages charge tuition fees for their services.
Find your dream home easily with our help. We know the best spots and prices, so you can relax and enjoy your new place.
Need a place to stay? We got you covered! Tell us what you need, and we’ll find the perfect spot for you, no stress!
We have listed attractive offers from selected partners. Do not miss any opportunity!
Basic Education in Portugal
The basic education school system in Portugal caters to children aged 6 to 15, over the course of three cycles:
- First cycle ( primeiro ciclo or 1º ciclo ): Grades 1 to 4
- Second cycle ( segundo ciclo or 2º ciclo ): Grades 5 to 6
- Third cycle ( terceiro ciclo or 3º ciclo ): Grades 7 to 9
Public school is free, and therefore the preferred option for the vast majority of Portuguese families. Public schools cover most of the educational costs of the students, depending on their education aid.
Elsewhere, it is estimated that only around 12% of children in Portugal attend private primary schools. Many of them being children of families from overseas.
Secondary School
It lasts 3 years, from the 10th to the 12th grade. Students need to choose from the following areas: science, art, humanities or economics in general education schools. There are other options in vocational schools, though. Subjects then depend on the area chosen. Four exams must be taken, depending on what the students want to study in university.
Financial Aid and Scholarships
Children from low-income families may qualify for financial aid to support their education. Also, children with disabilities receive financial support in the form of one or more of these:
- Disability bonus ( Bonificação do abono de família para crianças e jovens com deficiência );
- Special schooling subsidy ( Subsídio de educação especial);
- Support for assistance by a third person ( Subsídio por assistência de 3ª pessoa )
These grants and bursaries are provided to help cover the costs of children’s special care. Therapy and education requirements are also covered throughout their mandatory and subsequent education.
Scholarships covering some or all of the tuition fees may be available by contacting private schools directly.
Support for Expat Students in Portugal
Different schools have different policies when it comes to the way they cater to international children. Most local public schools conduct lessons exclusively in Portuguese. It may therefore be overwhelming for children who don’t yet speak the local language.
However, there are many schools in major towns and cities that have made good accommodations to support expat students, where most lessons (and final exams) can be adapted for those who do not yet speak Portuguese.
Support for Children with Special Educational Needs (SEN)
Portugal has made huge strides over recent decades to become as inclusive as possible, and to offer children from all backgrounds the best possible educational experience. The country’s inclusive educational system (educação inclusiva) is one of the best in Europe. Most mainstream schools in Portugal now offer their own special educational needs programs in-house. This means that SEN pupils may not need to attend a special school, but can instead learn alongside their peers in a regular school.
Share on social media
Leave a reply cancel reply.
Your email address will not be published. Required fields are marked *
Save my name, email, and website in this browser for the next time I comment.
+351 220 106 777
Rua de Camões 218 1. Floor, Office 2 4000-138 Porto Portugal
How can we help you?
English • Portuguese • Spanish • German • Italian • French
Schedule a meeting for:
Call us now, send us a message.
- I agree with the terms and conditions of Pearls of Portugal*
Don't miss out on offers or news!
- Buy / Rent / Sell a Property
- Taxes / NHR /NIF
- Lawyer Services
- Renovation / Construction
- Our Privacy Policy is accepted *
- I agree with the terms and conditions of Pearls of Portugal *
- Portugal Guides
Location Guides
Best destinations in portugal, buying in portugal, moving to portugal, living in portugal, select a language.

Your account
- My enquiries
- My favourites
- Portuguese Regions
- Portuguese Districts and Islands
- View all Location Guides
- Destinations
- All Best Destinations in Portugal
- The Buying Process
- Portugal Property News
- All Buying in Portugal
- Why move to Portugal
- Work and Jobs
- View all Moving to Portugal
- Schools And Education
- Transport and Driving
- View all Living in Portugal
Schools & Education in Portugal

In this article:
Different types of school in portugal.
- Pre-school education in Portugal
- Primary schools in Portugal
- Private Primary Schools in Portugal
Secondary education in Portugal
Special needs education and schooling options in portugal, homeschooling in portugal.
- Extracurricular activities
- University options
With its beautiful environment, warm climate and good living standards, Portugal is a popular choice for expats searching for a place in the sun.
Whether you already have children, or you’re making plans to have them in the future, Portugal is a wonderful place to raise a family. The Portuguese welcome children into their culture, so it’s not unusual to see families with children out in the evening, whether they’re enjoying dinner in a restaurant or attending a local event. As a result, children who grow up in Portugal are often mature, well-rounded individuals.
Share this:
Thanks to the climate, the Portuguese lifestyle is centred on spending time outdoors. There are plenty of facilities to keep children occupied, from playgrounds and skate parks to sports courts and football pitches. Portugal is ranked above the UK and the United States in Unicef’s latest league table of child well-being outcomes , scoring particularly well in the category for mental health.
If you’re thinking about moving to Portugal with your children, learning about the education system on offer should be a key priority.
Overview
The Portuguese education system is split into three terms. These run from mid-September to mid-December; the beginning of January to the Easter break; and from April to the end of June.
The Portuguese education system is divided into four sections:
● Pre-school from ages three to six
● Primary from ages six to 15 ( 1º Ciclo)
● Secondary from ages 15 to 18 ( 2º Ciclo)
● Higher for ages 18 and over ( 3º Ciclo)
Attending school is compulsory for children aged between 6 and 18 years. Once your child turns 18, they can either move on to university education or apply for work.
In Portugal, the entire education system is regulated by the Portuguese Ministry of Education (DGE). This covers all age groups, from pre-schoolers to secondary school students. School groups work alongside local municipalities to allocate funding and agree on the curriculum. The Ministry for Science, Technology, and Higher Education (MCTES) plays a key role in overseeing budgets and policies for tertiary education providers in Portugal.
For expats, it can be difficult to get your head around the many different education options in Portugal. Your child’s learning path will largely depend on their age.
The different types of school in Portugal include free state schools, private schools, international schools, and vocational learning pathways. If you’re searching for English-speaking schools in Portugal, international schools are a good option to consider. Lisbon is home to the International Sharing School Taguspark , widely considered as one of the best international schools in Portugal.
Although there are a range of international and private schools in Portugal, most parents in Portugal choose to send their children to the local state school, which is free of charge. Mandatory education starts at age 6, although most children start before then. As an expat, private education institutions may offer other benefits. International schools are designed to cater to the needs of expats, so they offer curriculums from all around the world, which can be taught in your child’s native language.
Not sure where to begin? We’ve mapped out all the key points to consider. Whether you need to arrange childcare for your pre-school age child, or find the best schools in Portugal for your teenager, we’re on hand to help.
Pre-school education in Portugal
The pre-school education system Portugal provides is run by the Ministry of Labor, Solidarity, and Social Security .
Most early childcare providers, such as kindergartens and childminders, are run privately. If you want your young children to learn Portuguese, arranging for them to attend pre-school from an early age is sensible.
Children aged between 3 and 6 may attend pre-school. It is not mandatory, but it is a popular option for many working parents. There are a range of different private and public pre-schools in Portugal, and you can also access childcare through social institutions and charities. Most pre-schools offer opening hours that fit around parents’ work schedules, with many staying open until 17:00 and beyond.
f you choose to use a private pre-school, you will be required to pay tuition fees. Fees are usually means-tested, which means families who earn more will have to pay higher fees. That said, the fees for putting a child into full-time pre-school in Portugal are less than you would pay in the UK or USA, even if you are classed as a high earner. When your child reaches the age of three, you will be entitled to 25 hours per week of free childcare.
Primary schools in Portugal
In Portugal, the primary school day usually runs from 09:00 to 15:30. Attendance at primary school is compulsory for children aged between 6 and 15. Your child’s time at primary school will be split into three cycles. The first cycle covers Grades 1 to 4, the second cycle covers Grades 5 to 6, and the third cycle covers Grades 7 to 9.
Since public education is free in Portugal and the standard of education is good, most locals send their children to a public primary school. This means there are few associated costs to consider, such as school meals, books and occasional day trips.
Expat children may find attending a public primary school difficult if they don’t already speak Portuguese. If you’re planning to stay in Portugal long-term, the whole family will benefit from learning to speak the Portuguese language. Young children are likely to pick up the language more quickly than older kids, so it’s best to start as early as possible.
The Portuguese primary national curriculum covers Portuguese, Maths, Science, Humanities and English. From Grade 7, pupils can opt to study another foreign language, such as Spanish or French.
When applying for public primary schools, you must choose one that is close to your home or place of work. You will be asked to provide evidence of your address, and there may be a waiting list, so it's important to be organised and apply early.
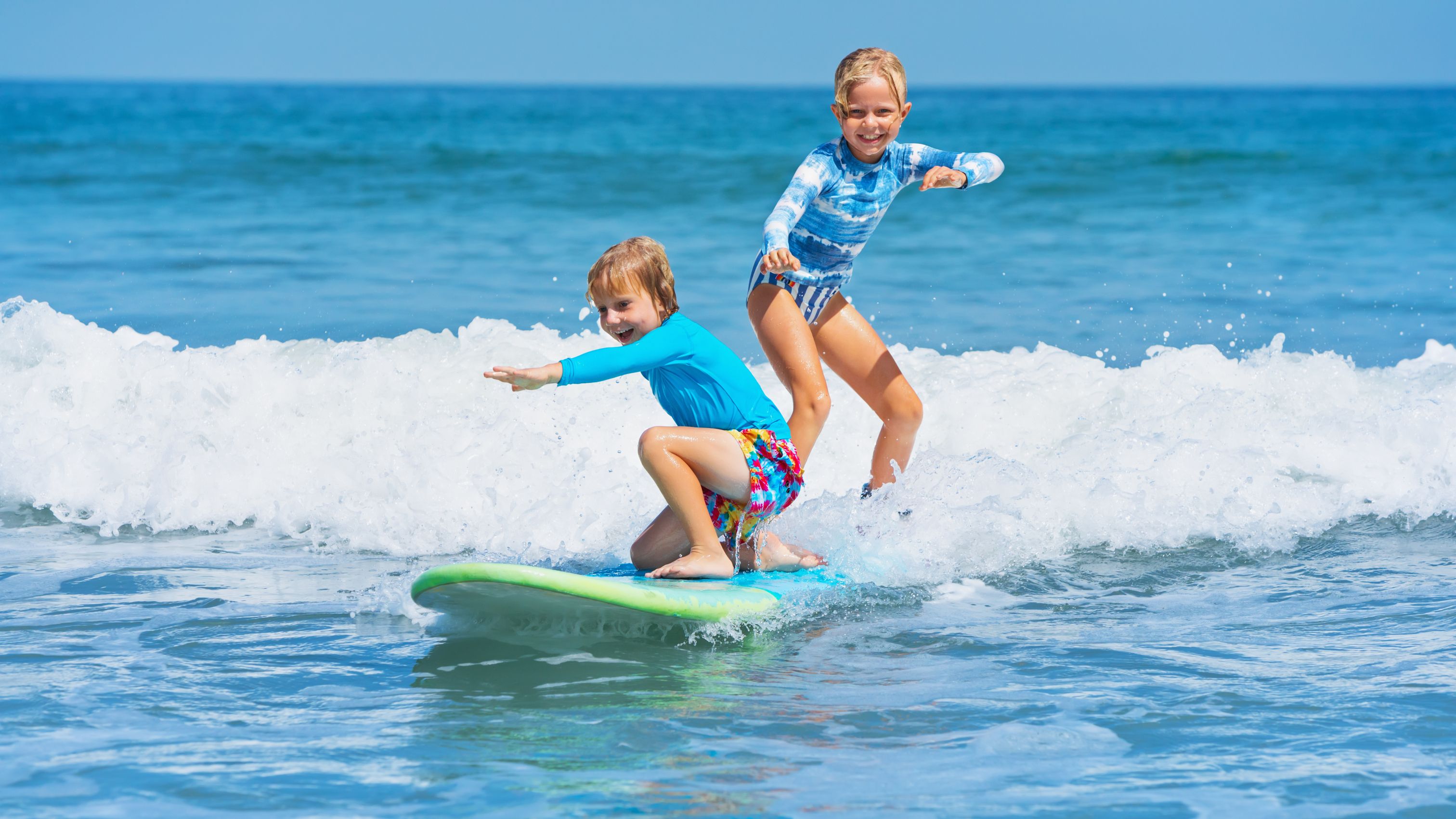
Private Primary Schools in Portugal
International schools in Portugal are classed as private primary schools. If you can afford to pay the fees, they are a good option for expat children. Your child will be able to study in their main language, while building friendships with children from all over the world. Other types of private primary schools in Portugal include Steiner-Waldorf and Montessori . Fees for these schools are typically at least 4,000 EUR per year. Class sizes tend to be smaller, and lessons are usually taught in Portuguese.
The Ministry of Education oversees the provision of secondary education in Portugal.
Upon completing primary education, students in Portugal have to apply for secondary school, which covers grades 10, 11 and 12. Attending secondary school, or escolas secundarias, is mandatory for children between 15 and 18 years of age.
The secondary school day is often long, with most secondary school students expected to stay at school until around 16:45. This can be useful if you’re trying to balance a job with childcare.
During the secondary school enrolment process, students can select from a range of study stream options. This means they can choose a route that suits their hobbies and career plans.
Some streams are designed to support students to prepare for university education, while others are work-based or vocational. Many primary schools use aptitude tests and offer guidance to assist students in choosing the best secondary school study route.
Public secondary schools are free in Portugal, and the standard of education is generally good. Pupils must study a set of core subjects, including Physical Education (PE), Portuguese, Philosophy, and a Foreign Language, which is usually English. The remaining topics of the curriculum vary according to their chosen study stream.
A range of different private secondary schools are available in Portugal. These include international, religious (usually Catholic) and boarding schools. Religious schools are a popular choice for parents, as they achieve the best results in the annual exams, and have a higher budget for extra-curricular activities. Fees range from 2,500 EUR to 10,000 EUR per year, although this varies between schools.
International secondary schools offer students a multicultural learning environment, which is often appealing to expat parents. An international school is probably the best choice if you are moving part-way through your child’s education, as they provide a familiar environment for learning.
You can choose from American, British, French and German international schools, with children able to learn in their native language and study a curriculum they are familiar with. Some international schools in Portugal, such as the Aljezur International School , use the UK curriculum, including the International General Certificate of Secondary Education (IGCSEs). Others allow students to choose between the British and Portuguese curriculums. The annual fees for international secondary schools in Lisbon are usually between 5,000 EUR and 15,000 EUR.
Options for boarding schools are limited in Portugal. If you’re considering boarding schools in Portugal, these tend to be in and around Lisbon and the Algarve. You can expect to pay fees in the region of 30,000 EUR per year.
Secondary school students in certain schools can study for the International Baccalaureate Portugal (IB) Diploma . This qualification is recognised by universities all over the world. It is designed to help nurture creativity and instill a sense of community. In Portugal, 12 schools offer the programme. These include PaRK International School , Colegio Atlantico , and St. Dominic’s International School .
To graduate from secondary school, students must successfully pass all of their subjects. Repeating a grade during primary or secondary education is not unusual in Portugal. Final grades are based on several different factors, including class participation, formal assessments, and homework. There are also two compulsory exams at the end of Grade 11, and one at the end of Grade 12, although these are not mandatory for students who have chosen to study a vocational stream. That said, all students applying for higher education will be required to sit these exams.
Portugal strives to provide an inclusive educational system, so most of the mainstream schools offer support to students with special education needs (SEN). The Ministry of Education also publishes a list of the state schools that actively prioritise inclusion.
Children below the age of 6 can be supported at home or in kindergarten by a special education teacher. Once they reach compulsory school age, they will be able to attend their local primary school.
The school will work with the child to develop an Individual Educational Plan (IEP), which will be tailored to their individual learning requirements. It might provide details or certain learning tools, or special allowances to be made in formal exams. Upon leaving school, the student will be issued with a plan to help them successfully transition into adult life. If the IEP or resources at the mainstream school do not meet the child’s needs, parents may wish to consider enrolling them at a specialist school.
Portugal has several different special education schools to choose from. Most of these are found in larger towns and cities. Each school is different, so it’s important to research the facilities and choose one that will meet your child’s individual needs. Some schools are well-equipped to support children with visual or hearing problems, whereas others are suitable for children with developmental disabilities. You can seek additional help from non-profit organisations, such as CADin , which supports families who have children with neurodevelopmental disorders.
Homeschooling is legal in Portugal , but not many families choose this option. In recent years, it has become harder to homeschool in Portugal. If you wish to homeschool, you must be university-educated and live in the same house as the student. The child has to be registered with a public school, and the teaching you provide must be in line with the national curriculum. Homeschooled students are expected to sit the annual exams, which are in Portuguese.
Extracurricular activities
In Portugal, extracurricular activities are seen as a priority, with most schools offering a diverse programme to complement the formal learning timetable. From sports and drama to choir and computer clubs, there is something to suit every student.
Extracurricular activities can help to improve a student’s career prospects, as well as help them build new friendships and improve their mental health. If your child attends a private school in Portugal, you can expect a more comprehensive programme of extracurricular activities.
University options
Higher education in Portugal is offered through universities and polytechnics. University degrees are based on research and theory, whereas polytechnics offer practical training in vocational professions. If you want to study a subject like law, medicine, economics or psychology, you will need to attend university. If you wish to become a nurse, accountant or teacher, you will need to apply to a polytechnic. Some fields are taught by both types of institutions, for example management, engineering, and agriculture.
Students who wish to pursue a career in the military or police may choose to attend state-run institutions, such as the Military Academy, Naval School, Air Force Academy, or Instituto Superior de Ciencias Policiais e Segurança Interna.
Prospective higher education students must apply in July or early August, either in their area of residence or online. The different levels of university education in Portugal include graduate degrees, Master’s degrees, and doctorates.
Admissions are highly competitive, and you will need to hold the diploma de ensino secundario or equivalent. In addition, you will be expected to pass other legal requirements, which may include minimum grades in certain national examinations.
Expat students must hold an academic qualification equivalent to the 12th year of the Portuguese education system.
The education system Portugal runs is a high-quality option for expat families. Whether your children attend a state school, private school, or international school, you can expect well-structured learning pathways, with plenty of extra-curricular activities to improve their leadership and communication skills.
Be the first to comment!
Add your voice
Get property bargains in your inbox
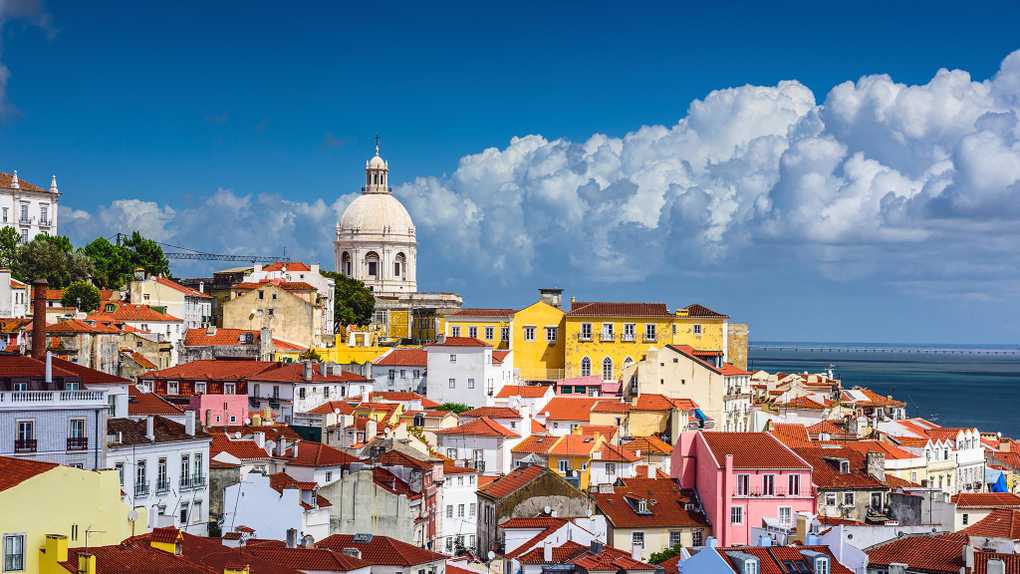
The world's largest expat community
A Guide to Education & International Schools in Portugal
Connect with fellow expats in Portugal
Join exciting events and groups for expats
Get information in our expat guides
Exchange tips about expat life in Portugal
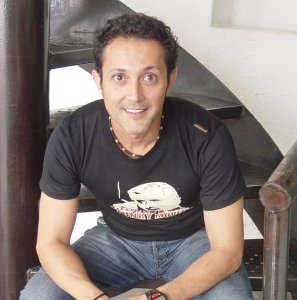
The quality of InterNations' members convinced me to join. And I've indeed received a very warm welcome from fellow Lisbon expats..
If your plan is for your children to study in Portugal, you can learn all about the best schools and higher education institutions in this guide.
You can find both public and private schools in the country, although you should expect to pay for your child’s schooling if you want the best quality education. The list of best schools in the country is topped by private institutions which are mostly Catholic schools.
For higher education, however, state universities are the better choice. The most prestigious universities in Portugal are run by the state and require only a co-payment of less than 900 EUR (990 USD) a year. Private universities are much more expensive, but a few of these are also featured in a world ranking of the best universities.
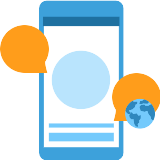
Connect with like-minded expatriates
Ask other international parents about schools in Portugal
An amazing community is waiting for you!

Meet internacional people at local events

Socialize, enjoy hobbies, and make friends

Get info, and feel at home abroad!
The Education System in Portugal
Portugal’s education system is mixed, with both public and private institutions providing education from kindergarten through to higher education.
Education Facts about Portugal
- Portugal has a mixed public-private school system.
- School is mandatory from six to 18 years of age.
- Public education is free with the exception of universities, for which you have to pay a relatively small fee.
- Some of Portugal’s universities feature in the QS World University Rankings.
What is the Education System Like?
Public school is free for all citizens and foreign residents.
Below are the different school levels and corresponding ages in Portugal.
School Level Ages Nursery ( Infantário ) 0-2 Kindergarten ( Jardim de Infância ) 3-5
Basic Education, 1st Cycle ( Ensino Básico, 1º ciclo )
School Year Age 1st 6-7 2nd 7-8 3rd 8-9 4th 9-10
Basic Education, 2nd cycle ( Ensino Básico, 2º ciclo )
School Year Age 5th 10-11 6th 11-12
Basic Education, 3rd cycle ( Ensino Básico, 3º ciclo )
School Year Age 7th 12-13 8th 13-14 9th 14-15
Secondary Education ( Ensino Secundário )
School Year Age 10th 15-16 11th 16-17 12th 17-18
The school year starts mid-September and ends in June. There are three school terms in a year, usually preceding holiday breaks.
The first term is from September to December, followed by two weeks of holiday for Christmas and New Year. The second term starts in January and ends two weeks before Easter. After Easter break comes the third and final school term, from the Tuesday after Easter to mid-June.
Grading System in Portugal
When attending a Portuguese school, you should expect different grading scales in different school levels.
Basic education (years 1–9) uses a five-point qualitative grading scale:
Percentage Grade in Portuguese English translation 90-100% Muito bom Very Good 70-89% Bom Good 50-69% Suficiente Satisfactory 20-49% Insuficiente Unsatisfactory 0-19% Mau Fail
The minimum passing grade is 50%, which corresponds to suficiente or satisfactory.
Secondary Education (grade 10–12) and higher education use a 20-point scale.
Scale Grade 17.5–20 Excelente (Excellent) 15.5–17.4 Muito bom (Very good) 13.5–15.4 Bom (Good) 9.5–13.4 Suficiente (Satisfactory) 3.5–9.5 Insuficiente (Unsatisfactory) 0–3.4 Mau (Fail)
With this scale, decimals from 0.5 and higher are rounded up to the nearest whole number. For example, a 9.5 becomes a 10, which makes 9.5 the minimum passing grade.
What are the Main Differences Between Public and Private Schools?
Public schools in Portugal are state-subsidized. This means education is free for all Portuguese nationals and foreign resident children. The quality of education in public schools has been improving over the years. However, public schools still struggle with issues like an aging teaching workforce and low graduation rates.
State schools’ curriculum is taught in Portuguese. If your kid does not speak the language yet, it is a good idea to enroll them in an international school in the country. You can choose an international school that follows the Portuguese curriculum to ease the process of integrating them into public schools later on.
Private schools in Portugal are perceived as being of better quality versus public schools. These tend to be stricter with school hours, attendance, and compliance with school rules. For this reason, parents may choose to send their kids to private school to ensure they have a stricter and more demanding learning environment.
How to Enroll Your Child in School: Requirements and Documents
Enrollment for first-year students is done between April and June. For all other school levels, children must be enrolled three working days after their final grades are released.
You can enroll your child in public school in two ways—online at Portal das Matrículas or in person at the public school of your municipality. Each school typically provides a list of required documents on their website and you may also call in advance to know which documents you should have handy. The standard required documents for most schools are:
- child’s ID or birth certificate;
- one photograph of the child;
- completed enrollment form (provided to you by the school);
- child’s immunization records ( boletim de vacinas) .
Besides these standard documents, private schools may also require an interview.
Appointed Parent or Legal Guardian (Encarregado De Educação)
Encarregado de educação (appointed legal guardian for schooling purposes) is the person who assumes the main role in the child’s education and handles all matters related to school. You are asked to appoint an encarregado de educação for your kid at Portuguese schools. This is typically one of the parents or legal guardians, but in case this is not possible, it can be whoever is in charge of caring for the child.
Being the encarregado de educação means you become the school’s first point of contact and official spokesperson for the child. For example, they can be asked to sign the child’s tests, official notes the child may bring to or from school, and attend parent-teacher meetings, among other responsibilities. You can even incur a fine (up to 88 EUR (96 USD)) if you miss an official request to attend your child’s school.
Daycare and Kindergarten
Whether you need to find options for daycare, pre-school, or kindergarten in Portugal, we guide you through early education in the country.
Nurseries: Infantários
Nurseries are known as infantários or creches in Portugal. These take in children as young as four months old (which is when maternity leave ends) until the age of three.
Most nurseries in Portugal are private. The few public nurseries available in the country are run by Social Security and are usually reserved for families with low income.
Pre-school: Pré-Primária
Pre-school or kindergarten is not mandatory in Portugal. This school level is known as pré-primária or jardim de infância . Children start kindergarten at the age of three and finish at the age of five or six, when they are ready to attend primary school.
You can find both public and private pre-schools in the country. The educational approach will vary slightly from school to school, but should cover five hours of educational activities for their social, affective, cognitive, psychomotor, and moral development.
Other options for daycare include ATLs which stand for Atividades de Tempos Livres . These are private institutions that receive children from six years of age, and usually fill the gap between school hours and when parents finish work.
Cost of Pre-school
Public pre-schools are free of charge. Since vacancies are limited, priority is given to children between four and five years of age, and to children affected by social disadvantages (e.g., disability, children of young parents (minors), etc.).
Almost half of Portuguese children attend private pre-school for lack of vacancies at public pre-schools. Private pre-schools in Portugal are the second most expensive in Europe, ranking only behind the UK.
Private pre-schools or kindergartens cost between 300 and 400 EUR (330–440 USD) a month, but prices can be even higher. Some municipalities have agreements with private schools to take in children when there are no vacancies in public pre-schools. Even then, parents can be made to pay up to 70% of the cost.
Daycare and childcare fees can also go up to 400 EUR (440 USD) a month, and add up to 4,500 EUR (5000 USD) a year.
Talk to other international parents with young children in Portugal
Primary and Secondary Schools
Get to know the best primary and secondary schools in Portugal.
Basic Education: Primary School
Primary school, or primária in Portugal, corresponds to the first cycle of basic education, years 1–4. This is the equivalent of elementary school in the US.
School hours in the country vary as these are established at a municipal level. School could start anywhere between 8:30 and 9:30 and last until 16:00 or 17:00. In total, schooling must make up five hours a day, including breaks.
You can choose to send your kids to public or private school. Public schools give priority to students who live in the area or whose parents work in the area, but you can apply to any school in the country, provided they have vacancies. You can list up to five schools of your choice, in order of preference, and your kid will be placed in the first one with available spots.
Children learn the following subjects:
- arts and sports
- English (in 3rd and 4th grade)
Basic Education: Second and Third Cycles
These two cycles, which correspond to grades five through nine, are often grouped together. Schools are called EB 2/3 (abbreviation for ensino básico de 2º e 3º ciclo ), or simply básico .
School hours depend on the school, since these are chosen at a municipal level as well. However, they must meet the minimum of 25 hours weekly.
The following subjects are taught in 5th and 6th grade: Portuguese, English, history and geography of Portugal, math, sciences, physical education (PE), visual education, information technologies, music. From 7th to 9th grade, the following subjects are added: second foreign language (Spanish, French, or German), history, geography, chemistry and physics. For all of these grades, moral and religious education is optional.
Secondary Education or High School
Ensino secundário , or simply secundário, is the equivalent of high school in the US. It is at this level that the Portuguese education system starts branching out, preparing students for either university or the workforce.
The most common courses at this stage are:
- científico-humanísticos courses—for students who wish to proceed to university;
- científico-tecnológico courses—for students who wish to study in university but with an added practical and professional component;
- professional courses.
Courses to Enroll in University
If students choose one of the courses that grant access to higher education, they will need to pick a main area of specialization:
- sciences and technologies;
- socioeconomic sciences;
- languages and humanities;
These share a common curriculum—Portuguese, foreign language (German, Spanish, or French), philosophy, and PE. The other five subjects are related to the field of study.
At the end of high school, students take national exams which make up a percentage of their final grade. Universities typically list the national exam that is required for entry in their courses.
School Costs
Public schools up to this education level are free for all citizens and foreign residents. Private schools, on the other hand, can be quite costly. Prices will vary from school to school, but on average, you will pay between 400 and 500 EUR (440–550 USD) a month for private schooling, from primary level to high school.
You are asked to pay enrolment fees, which are around 300 EUR (330 USD) a year, and you should take into account that books can cost up to 300 EUR (330 USD) as well.
Best Schools in Portugal
According to a national ranking, the best basic education schools are:
- Colégio Nossa Senhora do Rosário in Porto;
- Colégio D. Diogo de Sousa in Braga;
- Colégio Nossa Senhora da Conceição in Guimarães;
- Colégio dos Plátanos in Lisbon;
- Grande Colégio Universal in Porto.
The best high schools in Portugal are:
- Colégio da Rainha Santa Isabel in Coimbra;
- Grande Colégio Universal in Porto;
- Colégio Luso-Francês in Porto.
While escola is a more universal term, it usually refers to public schools. Private schools are almost always colégios . As private schools are known to be of much better quality than public schools, it is no surprise the list of best schools in Portugal is topped by private schools or colégios.
International Schools
Some of the best international schools in Portugal can be found in Lisbon, Porto, and the Algarve, as these are home to a significant number of expats. In this section, we introduce you to some of the best schools in Portugal for international students, from British, to French, or German schools, along with their costs.
International Schools: Admission Requirements
Usually schools post the required documents for admission on their websites, so it is always a good idea to read up on your preferred schools.
You will typically need the following documents and requirements:
- school’s enrollment form (available at the school or on their website);
- parent’s and child’s IDs;
- three passport photos of both the student and the encarregado de educação;
- proof of legal residence;
- school reports from previous years;
- medical certificates or proof of the child’s immunizations;
- proof of payment of the application fee;
- language tests, if needed.
Most schools require the child to be interviewed by the school director or other school staff as part of the admission process.
International Schools: Tuition Fees
If you are thinking of enrolling your kids at an international school, be prepared to pay plenty in tuition fees. Monthly fees are usually around 800 EUR (880 USD). The minimum you would pay at an international school in Portugal is 400 EUR (440 USD), but prices can go as high as 1,800 EUR (2,000 USD) a month. Prices tend to go up the higher the school level, even at the same schools.
For most of these schools, you pay a nonrefundable fee for submitting an application, between 30 and 70 EUR (33–77 USD). Besides this, you must pay annual enrollment fees of 200 to 500 EUR (220–550 USD). Besides books and other school supplies, some schools require the use of a uniform, so consider those added costs as well.
British Schools in Portugal
Portugal is home to a few British schools. The best ones are:
- Julian’s School in Lisbon;
- Peter’s International School in Setúbal;
- Oporto British School in Porto;
- CLIP in Porto.
The Oporto British School is the only school in the north that offers an International Baccalaureate (IB).
French Schools in Portugal
The best French schools in the country are:
- Lycée Français Charles Lepierre in Lisbon;
- Lycée Français International de Porto in Porto;
German Schools in Portugal
- Deutsche Schule zu Porto in Porto;
- Deutsche Schule Lissabon in Lisbon;
- Deutsche Schule Algarve in the Algarve.
Catholic Schools in Portugal
Portugal has a long tradition of Catholic schools and these tend to be the best schools in the country.
Catholic schools in Portugal teach Catholic Religion and follow Catholic values. Although no child is excluded on the basis of the family’s religion, parents should be aware that Catholicism will play some part in their child’s education at these schools.
However, this pedagogic approach can be very different from school to school. While some Catholic schools may simply include the subject of Moral and Religion as part of their curriculum, others such as Opus Dei schools may have compulsory prayer before classes, preparation for confession and first communion, and other religious celebrations, either mandatory or optional.
Some of the best catholic schools are:
- Colégio Rainha Santa Isabel in Coimbra;
- Salesianos do Estoril in Lisbon;
- Colégio Manuel Bernardes in Lisbon.
Higher Education
Most of the best universities in Portugal are public schools, although you will find some private schools near the top of the rankings as well. These are also the best universities for international students in Portugal. Education is high quality and classes will be taught in English whenever possible to accommodate international students.
You are considered an international student if you are not a national of Portugal or the EU. However, if you have been a legal resident in Portugal for two years, or you have been living in Portugal as of January 1st of the year you wish to enroll in university, you may apply as a resident. All other students are subject to a quota of 30% of the enrollment of a given course.
Best Universities in Portugal
According to the QS World University Rankings, these are best higher education institutions in Portugal:
- Universidade do Porto
- Universidade de Lisboa
- Universidade Nova de Lisboa
- Universidade de Coimbra
- Universidade de Aveiro
University Degrees in Portugal
Higher education in Portugal is organized in the following education levels:
- Licenciatura (bachelor’s degree)—three years
- Mestrado (master’s degree)—two years
- Doutoramento (doctorate)—varies
Many courses in Portugal, known as “ mestrado integrado ,” combine both bachelor’s and master’s degrees. For these degrees, students would enroll only once and continue their school years all the way to completion of their masters. These are common for medicine, engineering, architecture, and psychology.
You may also obtain a pós-graduação (postgraduation), usually after your master’s degree, which is considered a specialization and not a university degree.
How Much Does it Cost to Study in Portugal for International Students?
Unlike other school levels which are entirely free in public institutions, higher education in Portugal is not free. Students are asked to co-pay some of the costs.
Public Universities
Tuition fees for public university in Portugal vary by course, but these cannot surpass the maximum amount established by law, which are subject to change every year. The maximum fee for an undergraduate degree for the 2019 and 2020 academic year at a public university is 872 EUR (960 USD) a year. For some courses, tuition fees can start at 500 EUR (550 USD).
Private Universities
Private schools are free to choose their own pricing, which can be two to four times higher than public school fees. For private universities, annual fees tend to average 3,000 EUR (3,300 USD).
Ask other international parents already living in Portugal
Join one of our many local parent groups and get advice on which schools to choose.
Language Schools
Attending language school is the fastest way of learning the local language. There are several language schools in Portugal for foreigners, and you will find no shortage of options with a quick online search. Besides specialized schools, you can take Portuguese classes with several public universities as well such as Universidade do Porto, Coimbra, and Lisbon, to name a few.
You will find courses with different durations, from short courses of just a few hours, to more intensive classes of several weeks. Language school fees are usually around 300 EUR to 400 EUR (330–440 USD) for a 60-hour course.
Programa PPT
A government initiative called Programa PPT offers language classes free of charge. This is available to all foreign residents in Portugal regardless of their employment status or earnings. These have a duration of 150 hours for an A2 or a B2 certification. The entire course can be spread throughout several weeks or months, depending on the education center.
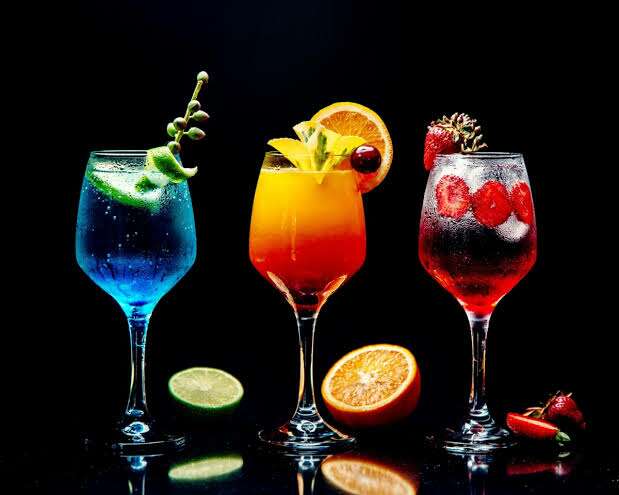
See all upcoming events for expats in Portugal
Our global partners.
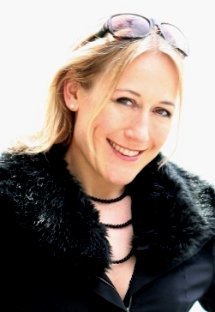
InterNations Events in Lisbon are great - I love the rightfully popular events organized by our friendly Ambassador team.
Communities in Portugal
Like-minded expatriates in portugal, brazilians in portugal, brits in portugal, french in portugal, americans in portugal, germans in portugal, italians in portugal, dutch in portugal, spaniards in portugal, south africans in portugal, russians in portugal, portugal guide topics.
Tax, Business, Property Law and Foreign Investment
Immigration law, portuguese citizenship, intellectual property, notarial acts, data protection.
- Português ( Portuguese (Brazil) )
Portugal’s education system: all you need to know
If you are moving to Portugal, especially with children and teenagers, you must be wondering and researching how the education system works in Portugal.
In Portugal, the public education system is the most used and best implemented – but there are also private school options at all levels of education. Here we will explain everything you need to know about Portugal’s education system before you move there.
How does the education system work in Portugal?
The education system in Portugal is regulated by the State through the Ministry of Education and the Ministry of Science, Technology and Higher Education. As we said, the public education system is the most used and best implemented, but there are excellent private schools at all levels of education.
The school year configuration in Portugal consists of: the first semester starts in September – after the summer season – and ends in January, while the second semester runs from February to June. The school vacations are from June to September, which coincides with the summer months in Europe.
How is the educational system divided in Portugal?
The education system in the country is divided into different levels, more specifically, into four categories: Kindergarten, Primary, Secondary and Higher Education, where Higher Education is not part of compulsory schooling.
Kindergarten
Primary and compulsory education in Portugal starts at the age of six. Before that, it is possible to use the so-called nursery and they accept babies from 3 months of age.
There are few openings in kindergarten, whether public or private. To get a place in public day care centers, for example, it is necessary to apply for a place for the child and join a waiting list.
Elementary Education
The Portuguese compulsory basic education system is divided into three sequential cycles. The child starts school at age 6 and goes through 12 years of study in total. In terms of structure, Basic Education in Portugal is divided as follows:
- 1st to 4th grade;
- expected age: 6 to 10 years.
- 5th and 6th grade;
- expected age: 10 to 12 years.
- 7th to 9th grade;
- expected age: 12 to 15 years;
Students in the 1st cycle need to fulfill a workload of 25 hours per week. In the 2nd and 3rd grades, the workload becomes 30 hours per week.
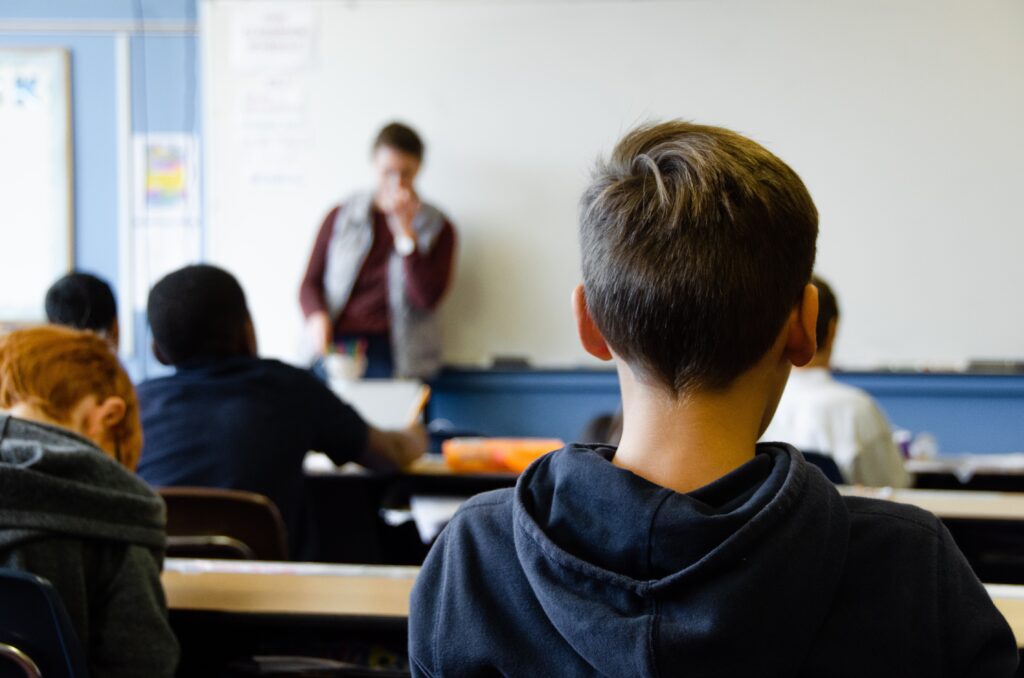
High School
Secondary education in Portugal corresponds to a three-year cycle, which includes five types of courses: scientific-humanistic, professional, artistic, specialized, courses with their own plans, and apprenticeship. In it, the students are already directed to the relative areas in which they wish to practice their profession.
Higher Education
Aimed at students who have successfully completed high school or have a legally equivalent qualification, Portugal, like 29 other countries in Europe, is structured according to the principles of the Bologna Treaty, which determines that Higher Education courses must last at least three years.
Education in Portugal is binary, divided into two modalities. The first is the modality taught at classical universities, focused on research and scientific investigation. The other is the education system of polytechnic institutes, which are practice-oriented courses.
In addition, the higher education courses follow a similar order to those in Brazil:
- Bachelor’s (undergraduate) degree lasting from two to five years;
- Master’s degree (professional and academic) lasting two years;
- PhD (doctorate in Portugal) lasting three to four years.
In Portugal, there is also the course called Integrated Master, in which in parallel to the undergraduate degree, a Master’s degree is also taken. Although it is a longer degree, at the end of the course the student is also awarded the title of Master.
Public institutions comprise 14 universities, 20 polytechnics, and 6 military and police higher education institutions. Private higher education institutions, on the other hand, comprise 36 universities and 64 polytechnic institutes. In the case of Brazilians, it is worth remembering that 34 Portuguese colleges allow Enem as a means of entry.
All colleges in Portugal are paid for – either with private funding or with state funding. The difference about how much it costs to study in Portugal at private universities is that, in general, there is no difference between the amount paid by Portuguese and foreigners, because the institutions do not count on government subsidies, while public universities do.
However, it is worth remembering that Portugal has agreements with countries in the CPLP – Community of Portuguese-speaking Countries – offering special rates for foreigners who are also part of this community.
Documents required to enroll in education in Portugal
To enroll a foreign student in Portugal’s educational system you need to bring the documents below – preferably before the school semester:
- Valid passport;
- Fill out the registration form (provided by the school itself);
- A current photo of the student (the school will indicate the format);
- School transcripts (recognized and apostilled in Brazil, according to the Hague Convention);
- Vaccination card;
- Medical certificate (in some schools);
- Proof of family residence in Portugal.
How much does it cost to study in Portugal?
In Portugal there are private and public education without and with government co-payment – in this case, the tuition is divided between the government and the parents. Public school, on the other hand, requires a payment according to the family’s income tax bracket. In addition, parents need to buy teaching materials and contribute to their children’s school meals (but, depending on the family’s financial condition, an agreement is made and part of the book costs are refunded).
In private schools, the tuition varies according to the institution. Tuition fees at the best schools in Portugal range from 500 to 1000 Euros. In these cases, it is possible, through legal counseling and representation, such as Moreira & Perfeito, to advance the pre-enrollment and pre-enrollment even before the arrival in Portugal.
In addition to tuition, the cost of registration, school insurance, and food must also be factored in.
Public education or private education in Portugal?
It is important to point out and be clear that, although education in Portugal is of the highest quality in both the public and private systems, there are differences.
The biggest differences between private and public education in Portugal is that private schools, besides offering more extra curricular activities, demand more from students in terms of preparation for exams – a vestibular, for example. Many also require an interview with the parents, albeit a virtual one in case they are not in Portugal.
The main advantages of studying in a private school in Portugal are being able to participate in various extracurricular activities, such as dancing, chess and music. In public education, on the other hand, the main advantage is in the costs. In public schools, parents must pay only for their children’s food and teaching materials. Another important advantage is the quality in education. A student will not learn less because he is studying in a public school!
In addition, Portuguese schools (public and private) operate full time, making it possible for those responsible for the children to work, without worrying about who to leave their children with.
It is necessary to balance and understand all the advantages and disadvantages of the education system in Portugal – be it public or private. Before making any decision, consult a specialist on the subject so that the choice is consistent with your wishes.
Here at Moreira & Perfeito we offer several services related to educational transfer to Portugal, such as enrollment in the school of your choice. Contact us to learn more!
Personalized service in which the client will present the specific case to lawyers who, based on their professional knowledge and vast experience, will provide the necessary clarifications.
It is important to mention that all information is protected by Attorney–client privilege and data protection laws.
Capabilities
- Advice on the practice of an isolated act in Portugal by a legal person located abroad
- Confidentiality Agreements and Commercial Dissolutions
- Constitution of companies in Portugal
- Legal consultancy for bank financing processes
- Legal consultancy for real estate investment
- Licensing for the exercise of Local Accommodation activity
- Negotiations and Investment
- Opening a bank account in Portugal for individuals and companies
- Representation in real estate deeds
- Tax Identification Number (NIF)
- Tax Litigation
- Tax planning in Brazil and Portugal
- Crediting of social security contribution time
- Employees Mobility
- Enrollment in educational institutions in Portugal
- Enrollment in public health centers in Portugal
- Exchange of Brazilian CNH for Portuguese driving license
- Migration of animals to Portugal
- Non-habitual resident status
- Registration in the Professional Class Order
- Registration of Residence in Portugal of Citizen of the E.U. and family
- Residence visa (longer than 12 months)
- Residency Permit for relatives of E.U. citizens/residents
- Statute of equal rights
- Temporary stay visa (less than 12 months)
- Validation of diplomas obtained abroad
- Averbação de Óbitos
- Civil Registry Rectification
- Civil Registry Rectification Law Suit
- Divorce Registration
- Issuance and search of Portuguese birth certificates
- Marriage Registration
- Portuguese Nationality Application
- Recognition of a foreign sentence
- Assignment and licensing of rights
- Combating unauthorized reproduction
- Litigation (administrative and judicial)
- Smart Contracts
- Trademark Applications
- Trademark Licensing
- Authenticated private documents (DPA)
- Certified copies
- Extrajudicial Notifications
- Powers of attorney
- Signature recognition
- Translations
- Conception of the data protection and privacy policy
- Implementation of data protection policies
- Outsourced Data Protection Officer (DPO)
- Personal data protection risk assessment
- Survey of processes that process personal data
- Survey of technical and operational needs that impact the organization’s compliance with LGPD and diagnosis of processes
Moreira & Perfeito
Practice Areas
Porto, Portugal
Rua Brito e Cunha, 254, 1º Dt.º Matosinhos, Portugal Zip Code: 4450-082 Waze | Google Maps +351 911 167 441
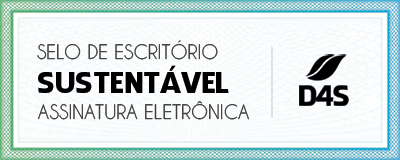
Moreira & Perfeito - Copyright © 2023 | All rights reserved. Created by Bravo Bird - Negócios Digitais

How are public schools in Portugal? Tips for expats
Maryam siddiqui.
- April 13, 2022
- Living in Portugal , Studying
Last Updated on April 16, 2024 by
As a parent, the first thing you do is ensure that your children feel welcome in a new country. It’s quite natural for expats to look for quality education and an environment that will nurture growth. This article will help you understand how public schools in Portugal offer a conducive learning environment for your children.
Keep reading to learn how Portugal’s public education system works and the key differences between the two systems. We’ll also review a list of Portugal’s best public schools and the enrollment process.
How do public schools in Portugal work?
Relocating to Portugal comes with challenges, but thankfully, we have a community here to help you. Before enrolling your children in a school, knowing the differences between public and private schools is better.
The structure of schooling is divided into two parts; Basic and Secondary education. Here are the details of each:
Basic Education
Basic Education, or Primary Education, is completely free and has a universal curriculum that’s easy to follow. It’s also compulsory to attain Basic Education, after which you may proceed to Secondary Education.
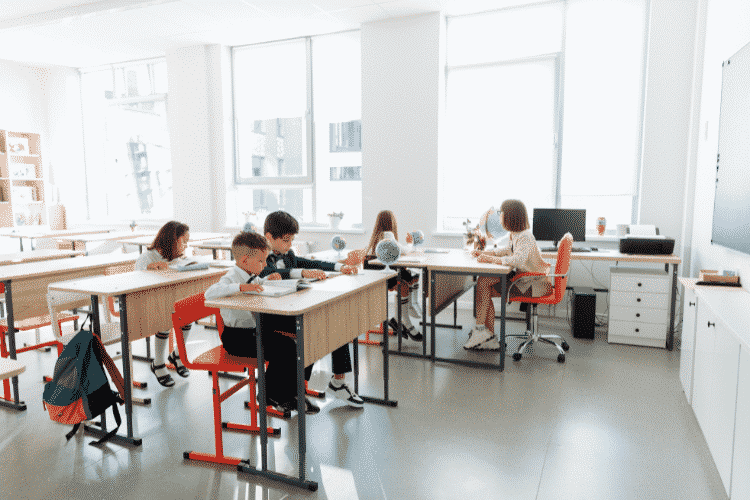
The aim is to acquire skills and fundamental knowledge that enhance comprehension and excellence. The years of Basic Education are divided as follows:
- First Cycle: Grades 1 to 4
- Second Cycle: Grades 5 and 6
- Third Cycle: Grades 7 to 9
Secondary Education
Secondary Education equals high school, which is essentially Grades 10 to 12. Students are encouraged to choose courses according to their preferences to make university education easier if they opt for it. One of the following fields is to be chosen:
- Language & Humanities
- Socioeconomic Sciences
- Sciences & Technology
At this stage, students have an idea of what career options they’d like to explore. Hence, it’s better to choose a field that is relevant to their interests. We also recommend giving your child the freedom to decide for themselves to motivate their personal growth.
Do public schools in Portugal teach in English?
All public education is done in Portuguese, but learning English is a mandatory part of the curriculum.
We understand that this language barrier might be a concern if your children are older. Some people prefer International Schools in Portugal to overcome the possible complications of not knowing the local language.
It’s important to note that expats from Britain, France, and the US have adapted pretty well to the lifestyle. Enrolling your children in a public school is particularly beneficial if you’ve decided to stay in the country for a long time.
Young minds learn Portuguese quickly and will feel a heightened sense of belonging once they can speak eloquently. Unlike other languages, Portuguese is relatively easier to understand.
- Read our article Learning Portuguese to know whether expats are bound to learn the local language.
Public school Vs International school in Portugal
There really isn’t a “Which one is better?” debate. Both choices are equally valid and have an excellent quality of education. Of course, it’s better to know why expats prefer one of the two over the other. We’ve helped you assess both options by preparing a list of pros for public and international schools:
Pros Of Enrolling Your Children In A Public School In Portugal
Let’s look at the most popular reasons why expats prefer enrolling their children in a public school:
✔️ Free of cost
Sometimes, the biggest deciding factor can be your financial circumstances. It’s a viable option to save on basic education expenses and invest in university fees later.
Plus, expats already have a lot of expenses lined up during relocation, from housing, food, and transportation to other necessities. By offering free public schools, Portugal helps alleviate some of the financial burdens of education and helps families save money.
Also, children from all socio-economic backgrounds can access quality education. This free-of-cost education actually helps promote diverse opportunities for your child.
✔️ They’ll dive into Portuguese culture
Relocating to a new country for the rest of your life can be a big decision. Getting to know the culture will help your children form lifelong connections and feel a sense of belonging. Starting primary education in Portuguese schools will help your child be closer to the culture and deeply understand things.
In public schools, expat children can make friends with local children. This can help them to integrate into the local community, feel more connected to their new home, and adapt to their new environment. The closeness to the culture can also help them learn the language at a faster pace during primary school.

✔️ Easier to learn the local language
As an expat, you might eventually have to learn the language at some point. By enrolling your children in a public school, you won’t have to spend on extra language classes. Learning Portuguese will also be significantly easier if they get a public education.
This is because public schools tend to provide intensive language classes. The more intense the class, the more exposure you will have to the language, which will make it easier to learn. Even so, it’s important to keep in mind that learning a language takes time, effort, and dedication, so try not to pressure yourself or your child.
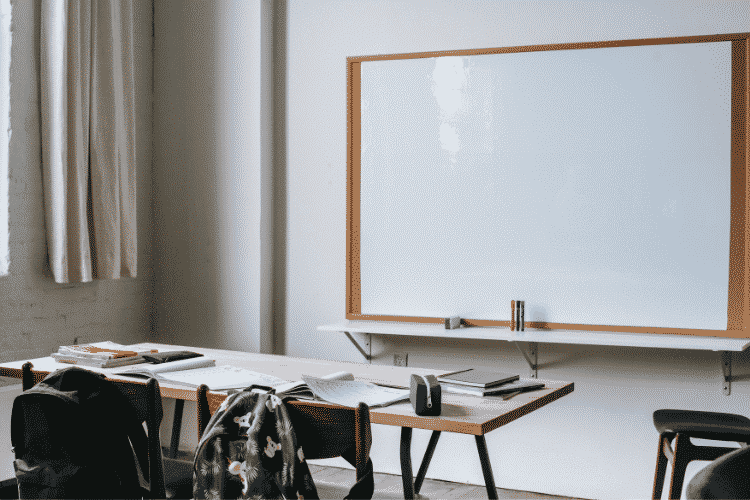
Pros of enrolling your children in International Schools in Portugal
These are the most popular reasons why expats go for private and international schools instead of public schooling:
✔️ Education is in English
If your children are older, their performance might be hindered slightly if they’re unable to understand Portuguese yet. In this case, it may be a better decision to enroll your child in private primary schools or private secondary schools, depending on your child’s age.
If your child is enrolled in an English-speaking school, they can easily communicate with teachers, classmates, and other people in their daily lives. This can help reduce the language barrier and make the transition to a new country smoother.
Plus, these schools offer a high-quality education, with experienced teachers and a rigorous curriculum.
Shifting schools in the middle of their education is a big decision in itself. So, it may be better to go for a curriculum in the language they’re comfortable with.
Most expats relocate to Portugal when their child is above the age of primary education.
Let’s Move to Europe
With Viv Europe your plans for Europe will come to a reality
✔️ International curriculum
The school curriculum includes but is not limited to, the International Baccalaureate and the National Curriculum for England. International schools usually have higher budgets to spend on their facilities and extra-curricular activities such as gardening, robotics, cooking, etc.
But the expenditure may be worth it as they are designed to be globally relevant so that expats can find a familiar educational approach to what they are comfortable with. This helps reduce culture shock and makes the transition to a new country smoother.
- Read Also: International Schools In Central Portugal: Enroll Your Expat Children .
✔️ Connection to multicultural families
Many expats from different countries have enrolled their children in International schools after relocation. One reason is that they get to connect with families from various cultures, which encourages diversity.
And if you’re an expat from France or China , for example, there’s a higher chance of your child being around someone with the same nationality. This is an experience that goes beyond physical education; your child’s emotional intelligence is more likely to develop when they interact with different cultures.
It’s also a great opportunity for children to learn about different cultures and make friends from around the world. Exposure to multicultural families can help expat children develop a global mindset and a greater appreciation for cultural differences.
For example, though the children may learn about the local culture well in public schools, they would get a chance to additionally explore German or French culture in International schools.
- Check out the best international schools in our article International Schools in Lisbon to find the perfect option for your children.
How much do public schools cost in Portugal?
As mentioned in the list of pros above, public schools in Portugal are free of cost for citizens and expats. The government funds these schools, and all basic educational expenses are waived. Though the quality of public schools can vary greatly, some are well-reputed and ranked higher than private schools.
Parents are only required to pay for meals that are given during school lunch breaks. The standardized menu includes soup, meat/fish on alternate days, bread, and fruits/jelly for dessert. The total expense for school meals is around €2 per day.
What is needed to enroll a child in a public school in Portugal?
The enrollment process for public schools is between January and May before the next academic year begins. You can also choose to register your children online through the Portal das Matrículas which the government regulates. If you’re confused about the process, you may visit the public school in your metropolis for more information.
The procedure is standardized among all schools, but it’s better to check in with the school’s administration beforehand. Typically, you would need the following documents when enrolling your child.
- The enrollment form (provided by the school)
- Your child’s ID card or birth certificate
- One photograph of your child
- Immunization and vaccine records
Can children of non-regularized foreigners study in public schools in Portugal?
Yes, they can. Education is a right given to all non-regularized and regularized foreigners in Portugal. All students are encouraged to perform better and surpass the average scores .
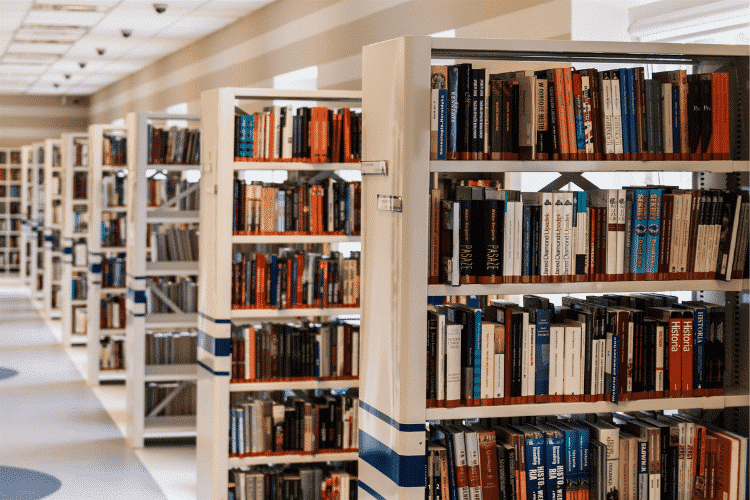
The International Convention on the Protection of the Rights of All Migrants guarantees equal education opportunities for non-regularized foreign children.
- Read Also: International Schools In The Algarve: Where To Enroll Your Child .
What are the best public schools in Portugal?
There are various public schools all over the country, so you’ll find a decent option regardless of where you live.
However, people have made an interesting observation regarding children with better grades studying at school. Students of public schools located in Lisbon, central and northern Portugal, have better grades than the rest.
This isn’t to say that other public schools aren’t worth attending. Here is a list of the top 10 Portuguese public schools:
- Primary and Secondary School José Relvas, Santarém
- Quinta das Palmeiras Secondary School, Covilhã
- Secondary School Dr. Joao Lopes de Morais, Mortágua
- Alves Martins Secondary School, Viseu
- Infanta D. Maria Secondary School, Coimbra
- Tomaz Pelayo Secondary School, Porto
- Primary and Secondary School of Vale do Tamel, Lijó, Barcelos
- Elementary and Secondary School, Oliveira de Azemeis
- Elementary and Secondary School Dr. Oak scallop, Maya
- Elementary and Secondary School of Arga e Lima, Lanheses, Viana do Castelo
Rankings are based on Observador’s list of Best Public Schools (as of April 2023).
When does the school year start in Portugal?
While the admission process starts in January, the academic year officially begins in September and continues until mid-June. During this time, you can prepare your children for new challenges to help them overcome their initial hesitancy.
A few suggestions we’d offer are taking them to explore the city with you and socializing with children their age. This will ease them a bit before their public education starts.
Public schools also have sets of holidays during the year. There is a two-week Christmas and Easter break each and a few days for the Mardi Gras Carnival in February.
Do you need help bringing your family to Portugal?
This article summed up almost everything you needed to know about public schools in Portugal. If you have any questions regarding the academic experiences of expat children, join our Facebook Group – All About Portugal For Expats to connect with expats.
Alternatively, you can also shoot us a message any time you like! Our team at Viv Europe is here to make the relocation process seamless for you and your family. Book a consultation and get help with enrolling your child, family regrouping, or Visa processes.
- Read Next: International Schools In Cascais: Top 5 Best Options .
Feature Articles
- Portugal or Spain? Best country for expats
- Portugal Golden Visa Updates: New Investments in 2024
- Buying a car in Portugal: Prices, taxes, and tips for first-timers
- Living in the Azores: An expat guide to the Portuguese islands
- Where do the rich live in Portugal? See the most exclusive regions
Subscribe to our newsletter for insights and updates on relocating to Europe!
Explore our latest Instagram posts to discover vibrant snapshots of European living, relocation tips, and inspiring stories from our community!
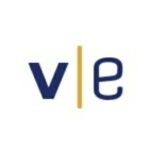
Check out our recent Facebook posts for valuable insights, engaging stories, and tips on making your European relocation dream a reality!

This content isn't available right now
Share on Facebook Share on Twitter Share on Linked In Share by Email
Get in Touch with Us
Viv Europe can help you practice your plans to move to Portugal. Our team of experts knows what it takes to handle all the paperwork to make your move as smooth as possible.
Latest Articles
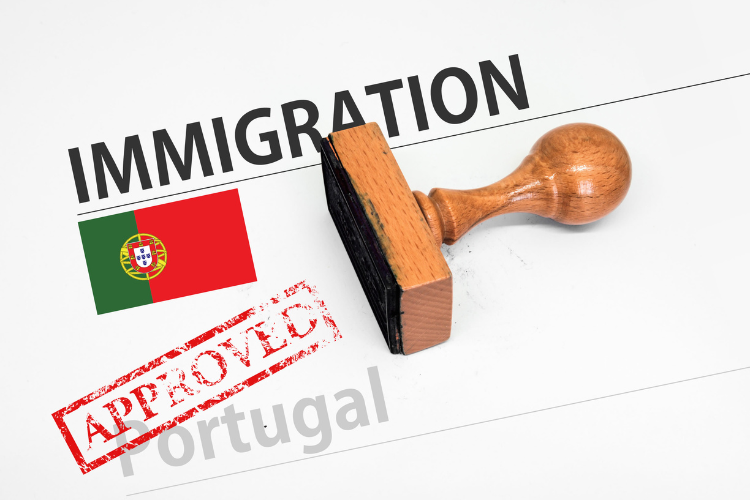
Expression Of Interest Portugal: What Does The End Mean?
Best Banks In Spain: Open a Bank Account Remotely
How Much Money Do You Need to Move to Portugal?
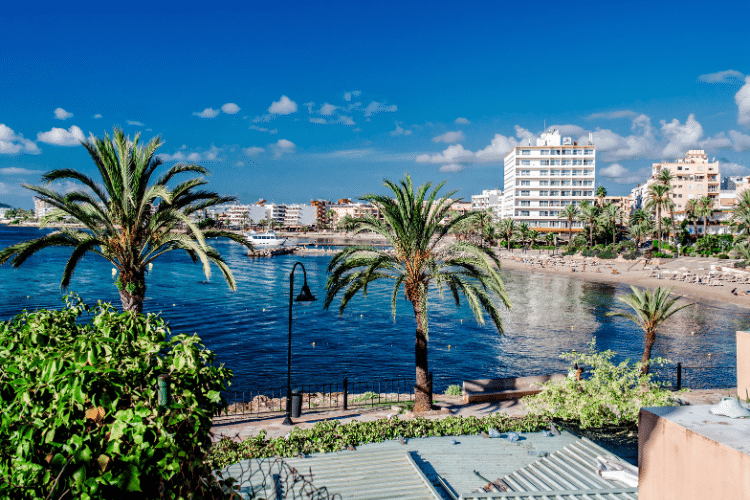
Moving To Spain From US: Complete Guide For Americans
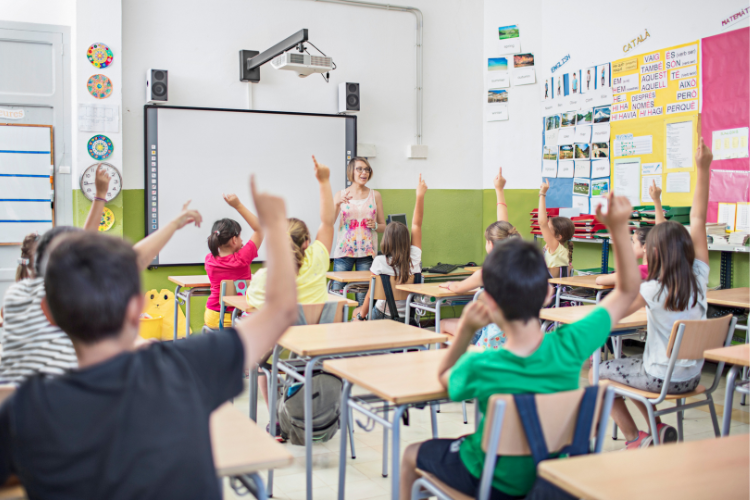
The Best International Schools In Spain: Enroll Your Child
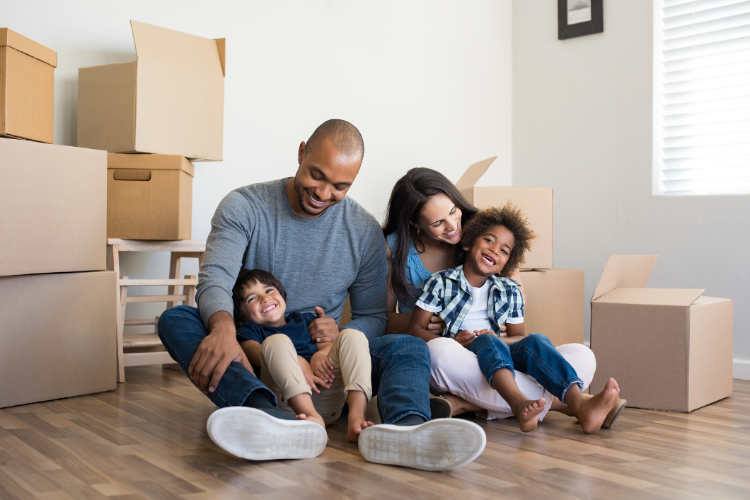
Portugal Residency Requirements For US Citizens
Create a world where borders fade away, making European countries accessible and welcoming to all.

- [email protected]
- +351 253 128 911
- NIPC: 515916145
- AMI Licence: 21426
- Praça da Justiça, n.º 168 - São Victor, 4715-125 - Braga - Portugal
Viv Europe is a relocation assistance company, and an external lawyer must conduct all legal procedures.
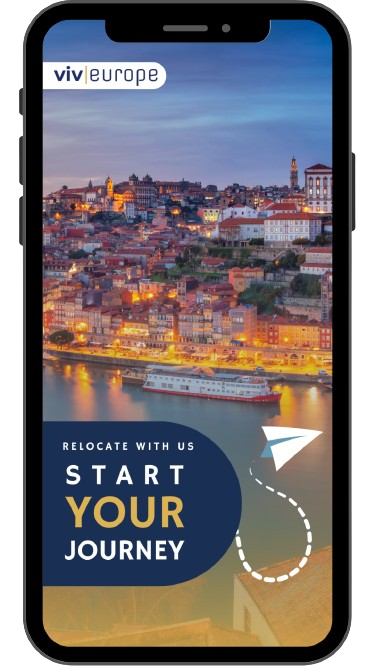
Looking For exclusive Relocation Tips?
Sign up for our Newsletter to receive the latest updates, visa tips, and more!
Back to Blog
International Schools In Portugal: How To Choose In 2024
Updated: April 3, 2024 | Zahra
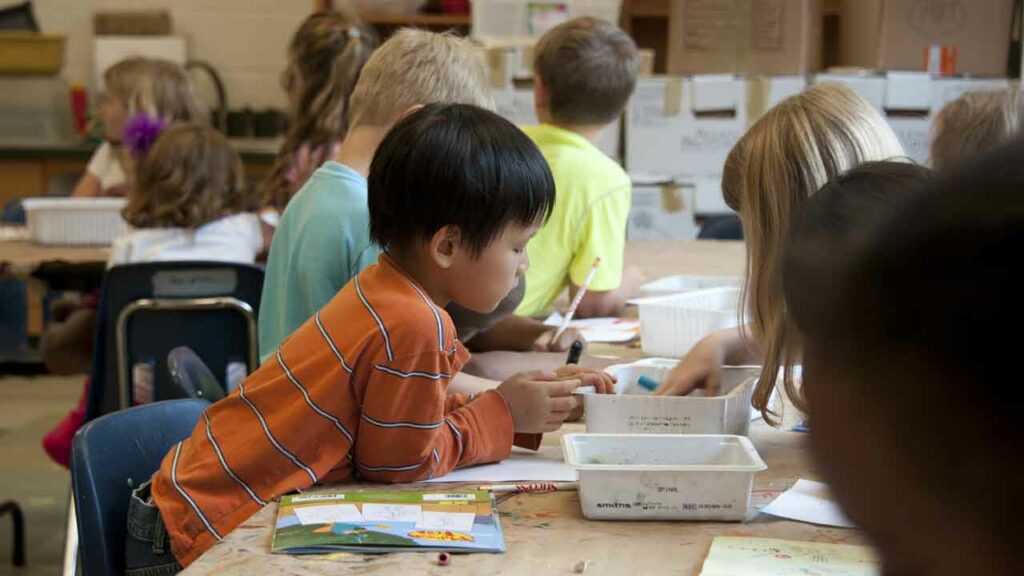
Are you considering relocating to Portugal with your family? If so, you're probably thinking about your child's education and school life and wondering what options are available to you.
Choosing the right school is a critical decision, and we want to help you make the best choice for your family. That's why we created this International School Guide, which covers everything you need to know about the best international schools in Portugal. From what's on offer to how to choose the right one and where to find them, we've got you covered. We'll also outline the top factors to consider when making your decision.
Here's what we'll cover in our international school guide:
- Tips to pick the best international school in Portugal
- The best international primary schools and secondary schools in Portugal
- An overview of the Portuguese education system, and more
What can international schools in Portugal offer my child?
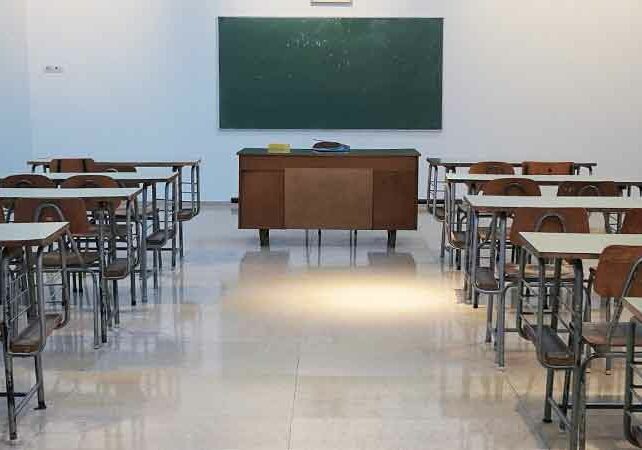
International schools in Portugal offer a high standard of education and are often accredited by international organizations. They provide a multicultural environment and an opportunity for children to learn and interact with students from across the world. This type of education encourages students to be open-minded, empathetic, and respectful of other cultures.
Additionally, international schools often have a wide range of extracurricular activities and programs that can help your child develop their skills, talents, and interests.
Moreover, many international higher education institutions follow the International Baccalaureate (IB) curriculum or the UK national curriculum, both recognized worldwide. These curricula are designed to promote critical thinking, creativity, and problem-solving skills, which are essential for success in the 21st century.
Thinking about the future? Many of Portugal's schools offer internationally recognized qualifications, such as the Cambridge International Examinations. These qualifications can give your child a competitive advantage when applying to universities around the world.
One of the advantages of attending an international school in Portugal is that it can open up a world of opportunities for your child. With a global education system, your child will be well-prepared for higher education in any part of the world.
Types of International Schools in Portugal
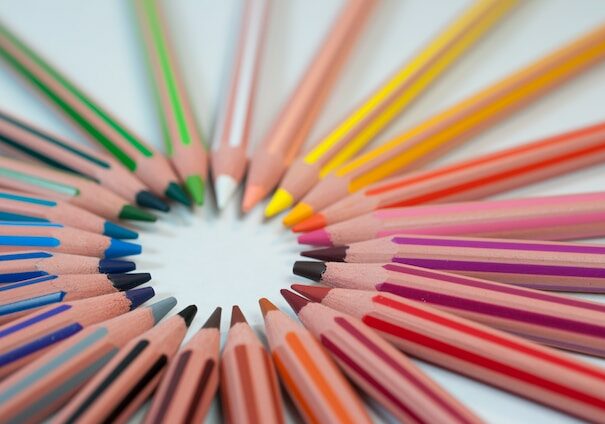
American Schools
American international schools in Portugal typically follow the American curriculum, providing students with an education system aligned with standards in the United States. These schools often incorporate Advanced Placement (AP) courses and may lead to an American high school diploma. English is the primary language of instruction, and these schools aim to create an environment familiar to students from the United States.
British Schools
British international schools in Portugal adhere to the British curriculum, offering programs such as the International General Certificate of Secondary Education (IGCSE) and A-levels. These schools follow the UK education system, emphasizing subjects like English, mathematics, and science. British schools often maintain a strong academic tradition and may prepare students for entry into universities in the United Kingdom.
Bilingual Schools
Bilingual international schools in Portugal offer a curriculum that integrates two languages, typically English and Portuguese. These schools aim to provide students with proficiency in both languages, fostering cultural awareness and linguistic diversity. Bilingual education often includes the incorporation of elements from international curricula to ensure a well-rounded educational experience. These schools are particularly suitable for students seeking fluency in both languages and a multicultural learning environment.
French Schools
French international schools in Portugal follow the French educational system, offering programs such as the Diplôme National du Brevet (DNB) and the French Baccalauréat. These schools provide education in French and often emphasize the French language, literature, and culture. French schools aim to maintain academic excellence while promoting French values and traditions.
German Schools
German international schools in Portugal follow the German curriculum, offering programs such as the Abitur. These schools provide education in German and often focus on maintaining strong ties to the German language, history, and culture. They may cater to families seeking continuity in their German-language education while residing in Portugal.
How to Choose an International School in Portugal
When choosing an international school in Portugal is crucial, and there are many factors to consider. The location of the school, the language of instruction, the curriculum, the fees, and the extracurricular activities are just some of the things you need to consider.
You can start by researching online and checking out school rankings and reviews. It's also a good idea to schedule visits to the schools you're interested in to see the facilities and meet the teachers and students. This will also help you get a feel for the school's atmosphere and community.
Portugal offers everything from German schools to British international schools to American international schools.
Don't forget to listen to your child's needs and preferences because, after all, they are the ones who will be attending the school. It's important to consider your child's needs and interests when choosing a school. For example, if your child is passionate about sports, you may want to look for a school with a strong athletic program. Likewise, if your child is interested in music or the arts, you may want to find a school with a robust performing arts curriculum.
Here are some top tips to help you make the best decision for your child:
Accreditation
Look for an international school that is accredited by a recognized organization, such as the Council of International Schools (CIS), the New England Association of Schools and Colleges (NEASC), or the International Baccalaureate Organization (IBO).
Accreditation ensures that the school meets high standards in areas such as curriculum, teaching, and facilities.
Consider the type of curriculum offered by the school. Some primary schools offer the international primary curriculum, and some high schools in Portugal offer the International Baccalaureate Programme (potentially leading to an International Baccalaureate Diploma), while others follow the British curriculum, the Cambridge curriculum, or the American curriculum, and others include a combination of international curricula and the Portuguese national curriculum.
As you explore the options available to you, consider which international curriculum best fits your child's needs and future goals.
Think about the school's location and how easy it will be for your child to commute there. Consider the proximity to your home or workplace and access to public transportation. The Greater Lisbon area is home to some of Portugal's best international schools, but there are great institutions all over the country.
Extracurricular activities
Check out the school's extracurricular offerings. Do they offer sports, music, drama, or other activities your child is interested in? Extracurricular activities can be an excellent way for your child to make friends, develop new skills, and improve their sense of well-being.
Teacher qualifications
Look into the capabilities of the school's teachers. Do they have the necessary certifications and experience to provide a high-quality education? A good teacher can significantly impact your child's learning experience.
By considering these factors, you can help ensure that you choose the best international school in Portugal for your child.
Depending on what stage your child is at, it might be worth considering what level of education is on offer. For example, whether you're interested in a school which offers both a primary school and secondary education. You might plan to continue higher education elsewhere, or you might want your child to stay in the same school.
The Number of International Schools in Portugal
Portugal has 51 international schools in seven different cities . Many are located in the Greater Lisbon area, Porto, and the Algarve region.
Lisbon neighborhoods with international schools include Cascais, Estoril, Sintra, Oeiras, and Carcavelos.
The Algarve region has international schools in Faro, Almancil, Lagoa, and Portimão. Other regions of Portugal, such as Braga, Madeira, and Leiria, also have international schools, although they are fewer in number.
Locations of International Schools in Portugal
International schools in lisbon.
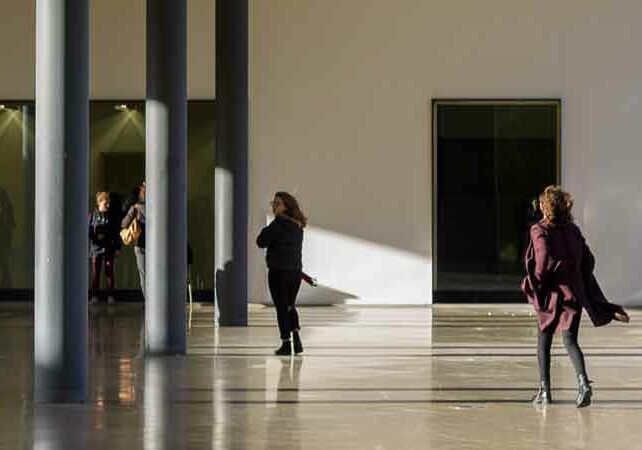
Some of the neighborhoods in Lisbon with international schools include Cascais, Estoril, Sintra, Oeiras, and Carcavelos. Each neighborhood offers a unique living experience and provides access to some of the best international schools in Portugal. How
Some of the best International schools in the Greater Lisbon area include the The British School of Lisbon and the The Carlucci American International School of Lisbon.
International Schools in the Algarve
If you're planning on living in the Algarve, you'll be happy to know that there are several international schools in the region.
Private options such as Nobel International School Algarve , Vale Verde International School , Eupheus International School , Aljezur International School , and the Vilamoura International School offer high-quality education and a range of extracurricular activities for students of all ages. These schools provide a nurturing environment that fosters academic excellence, personal growth, and cultural awareness.
International schools in other regions of Portugal
In addition to Lisbon and the Algarve, there are respected international schools located throughout different regions of Portugal.
Private schools like the French School of Porto , International Preparatory School , and Leiria International School offer exceptional education and a multicultural environment for students of all ages.
Schools like Oporto British School in Porto offer both primary education and secondary education. Boarding schools like St.Peter’s School also offer weekly or full boarding options.
The Top International Schools in Portugal
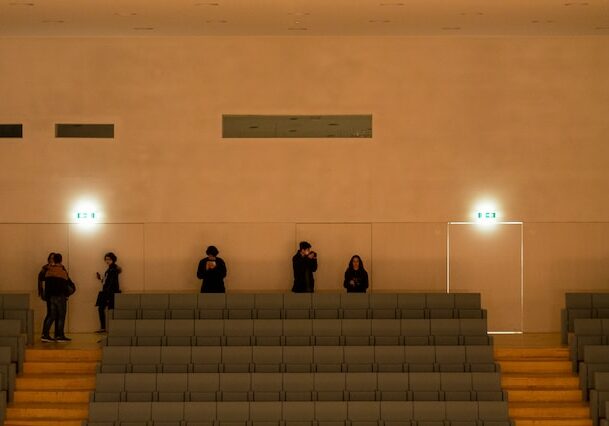
St. Julian’s School (Carcavelos): A well-established school offering an international program based on the English National Curriculum. On the Portuguese side, children access the Portuguese national curriculum.
The Carlucci American International School of Lisbon (Lisbon): The Carlucci American International School of Lisbon is located in Sintra and is the only US State Department school in Portugal. The oldest American school in the Iberian Peninsula, it's a prestigious, fully accredited institution. It offers elementary school, which offers a basic education all the way to secondary school.
International Christian School of Cascais (Cascais): The International Christian School of Cascais (ICSC) is an international school with a "family" environment that provides religious education alongside an American-style curriculum. Active learning is incorporated into classes alongside creative and critical thinking styles to ensure understanding of the subject. Fees are not public, as the school always tries to help families as much as possible. Students do not have to be of the Christian faith to attend the school.
St. Dominic’s International School (Cascais): Located in Cascais, in close proximity to Lisbon, St. Dominic's International School was established in 1954 by the Irish Dominican Sisters, making it an excellent choice for parents seeking the advantages of religious education. The school provides three distinctive programs: the diploma program, the middle years program, and the primary years program.
Prime School International (Multiple Locations): With campuses situated in regions like Cascais, Estoril, Lisbon, and Sintra, this global educational institution caters to students aged 3 to 18. The school adopts the Cambridge Curriculum, which is exclusively taught in English. For native Portuguese speakers, the option to participate in classes conducted in both English and Portuguese is available.
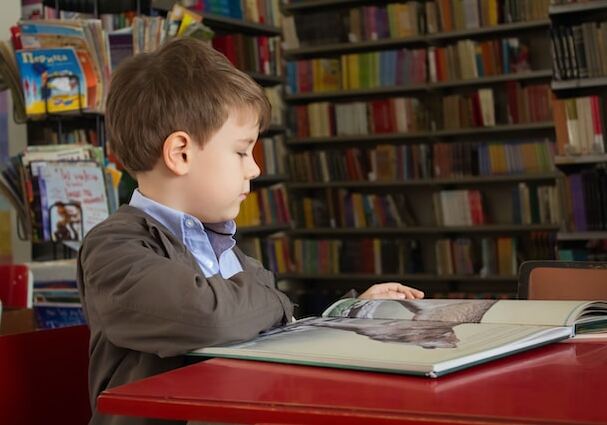
St. Peter’s International School (Setubal): This is a distinguished private international school offering premium education. Renowned for its comprehensive approach, the school features both national and international programs, encompassing prestigious curricula such as the Cambridge International General Certificate of Secondary Education (IGCSE) and the International Baccalaureate (IB) Diploma.
International Preparatory School (Porto): IPS is an international school that caters to children aged three to eleven. With a diverse student and faculty body representing various nationalities, the school provides an ideal setting for expatriate children to flourish in an international environment.
The Portuguese Education System
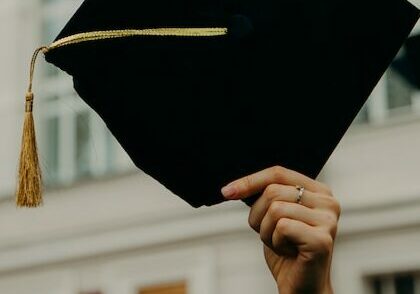
In recent years, Portugal has made efforts to modernize its educational infrastructure, incorporating innovative teaching methods and technology to enhance the learning experience.
The education system in Portugal is divided into the following educational levels:
- Preschool education (Educação Pré-escolar): Ages 3 to 6
- Primary education (Ensino Básico): Ages 6 to 15
- Secondary education (Ensino Secundário): Ages 15 to 18
- Higher education (Ensino Superior): Ages 18 and above
International Schools versus Public Schools in Portugal
When considering where to send your child to school in Portugal, you may be weighing the pros and cons of public schools versus international or private schools. Public schools in Portugal are generally well-regarded, with a strong emphasis on public education and a curriculum that is aligned with national standards.
Additionally, public schools are more affordable than private options, as they are funded by the government and do not require tuition fees. This makes them an attractive option for families who are budget-conscious or who prioritize the accessibility of education.
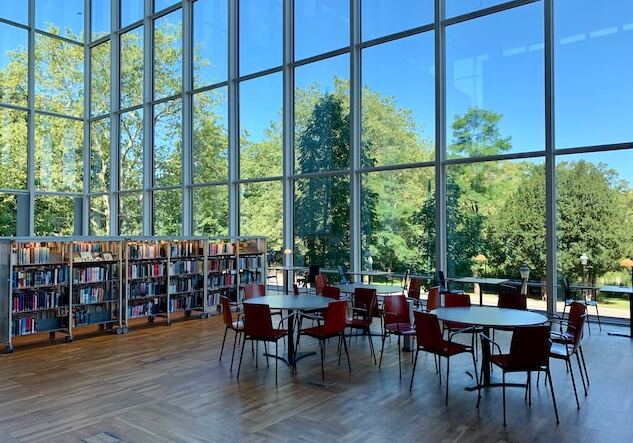
Additionally, international schools may offer a more rigorous academic program, with smaller class sizes and more individualized attention from teachers. While private school tuition fees may be higher than at public schools, many families feel that the benefits of an international or private education outweigh the costs.
Ultimately, the decision of whether to send your child to a public or private school in Portugal will depend on your individual priorities and circumstances.
International Schools in Portugal Fees
If you’re considering an international school for your children, expect higher tuition fees. Monthly costs typically hover around €800. The minimum monthly fee at an international school in Portugal is around €40, but these prices can reach as much as €1,800 depending on the school level. You should also factor in potential costs for uniforms, books, and other school supplies as you budget for education in Portugal.
What is the cheapest international school in Portugal?
All of the international schools in Portugal are private and charge tuition fees. Costs vary, but the Instituto Espanhol Giner de Los Rios and the International School of Madeira are two schools with lower-cost tuition fees.
Other Useful Resources
If you're planning on relocating to Portugal, you might find our following articles useful:
- Living in Portugal as an American
- Working in Portugal as an Expat
- Portugal Work Visa
- Digital Nomad Portugal
- Getting a NIF Number in Portugal
- How to Open a Bank Account in Portugal
Our partner, Global Citizen Solutions , has also created a detailed guide to International Schools in Portugal .
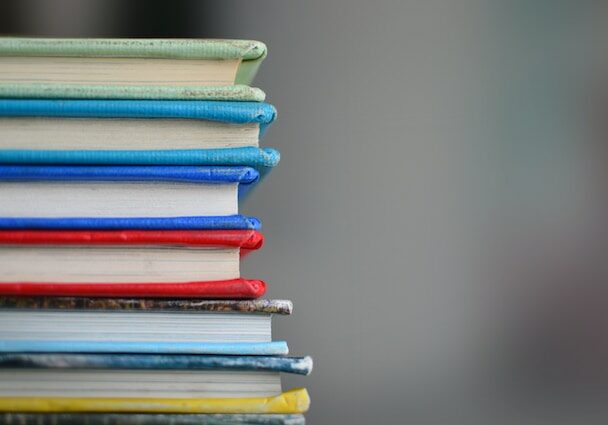
Private schools in Portugal offer world-class education, with experienced teachers and staff, state-of-the-art facilities, and a curriculum that emphasizes language learning and multilingual education.
Through a comprehensive program that includes foreign language instruction and a range of extracurricular activities, international schools in Portugal are committed to providing students with a well-rounded education that will equip them with the skills they need to succeed in the future. Whether you're a parent looking for the best education for your child or a student seeking a transformative learning experience, international schools in Portugal are an excellent choice.
Frequently Asked Questions about International Schools in Portugal
Does portugal have good international schools.
Yes, Portugal has good schools that provide quality education to international students. These schools follow international curricula and provide a diverse learning environment that helps students develop a global perspective.
The international education system in Portugal is also considered to be of a high standard, making it an attractive destination for families looking to provide their children with a good education.
Are international schools free in Portugal?
No, international schools in Portugal are not free and are considered private schools. The fees for international schools vary depending on the school and the grade level.
It is important to note that the fees for private international schools in Portugal are more expensive than the fees for local schools. Families should research and budget accordingly before deciding on an international school for their children.
How much do international schools cost in Portugal?
International school costs in Portugal vary depending on factors like location, curriculum, and grade level. Expect a range across different schools, with some reaching higher amounts. Be prepared for additional fees beyond tuition, such as application, enrollment, and materials.
Are there English-speaking schools in Portugal?
Yes, there are several English-speaking schools in Portugal providing education in English.
These schools are either international schools or bilingual schools that offer a curriculum in both Portuguese and English. In addition to English, some schools may provide instruction in other languages, such as French, German, and Spanish.
Are there British International Schools in Portugal?
There are around 14 British International Schools in Portugal. There's a slightly higher concentration of British schools in the Algarve region, where the largest British international school, Nobel Algarve British International School, is located.
How many international schools are in Portugal?
There are approximately 51 international schools in Portugal. These schools are located in different regions of the country, including Lisbon, Porto, the Algarve, and other areas.
What are the international schools in Lisbon?
Some of the best international schools in Lisbon include St. Julian's School, Carlucci American International School of Lisbon, International Preparatory School, and Oeiras International School.
What are the international schools in Porto?
Some schools in Porto include CLIP - Colégio Luso Internacional do Porto, International College of Porto, and Oporto British School.
What are the international schools in the Algarve?
Some schools in the Algarve include the Nobel International School Algarve, the International School of the Algarve, and the Vale Verde International School.
How can I choose from the best international schools in Portugal?
To choose the best international school in Portugal, families should consider factors such as the school's curriculum, teaching approach, facilities, location, and fees.
They should also research the school's reputation and talk to other families with children attending the school. Visiting the school and meeting with the staff can also provide valuable insight into the school's culture and values.
Can American kids go to school in Portugal?
American children living in Portugal can attend both local public schools (free, immersive, with language support) or international schools (English-speaking, familiar curriculum, tuition fees). The ideal choice hinges on your family's priorities, budget, and desired level of cultural integration.
Did you enjoy this article? Recommend it to your best friend, your mom, the stranger you met at Starbucks…or maybe even your arch-enemy? It’s up to you, I’m just throwing some ideas out there.
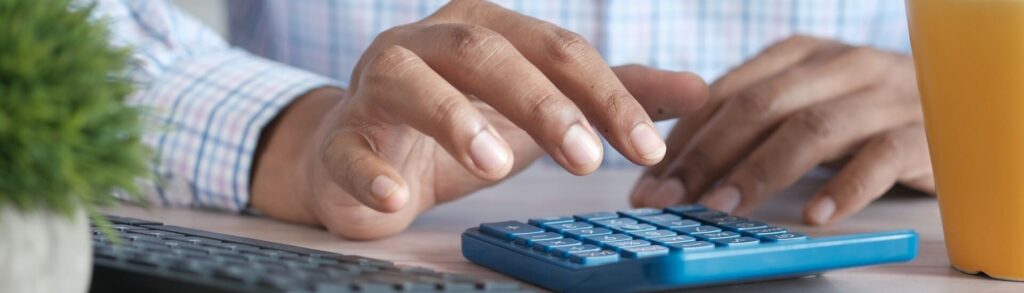
Cost of Living in Portugal vs the USA – A Guide for 2024
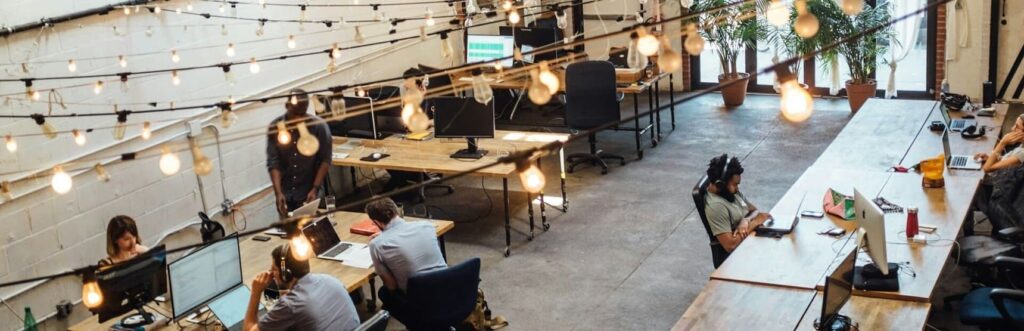
Coworking Portugal: A Complete Guide for 2024
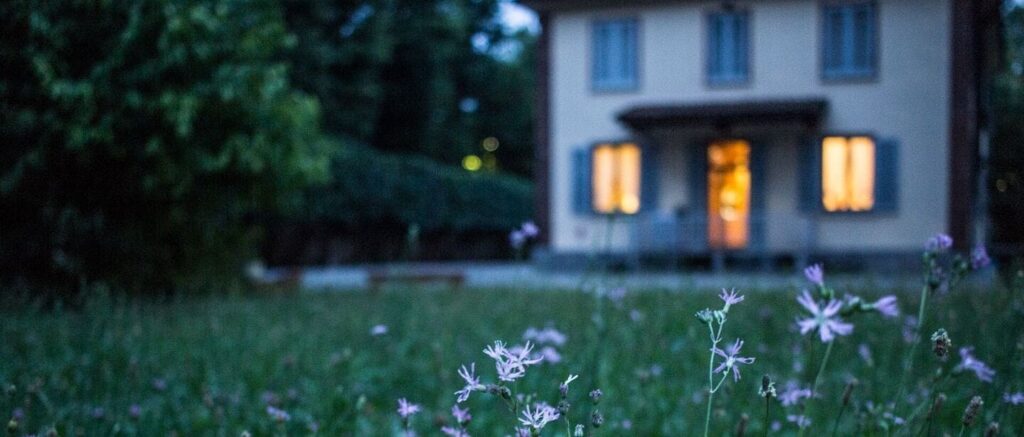
Home Insurance in Portugal: All You Need to Know to Choose the Best for You
The Easy way to get a Portuguese NIF
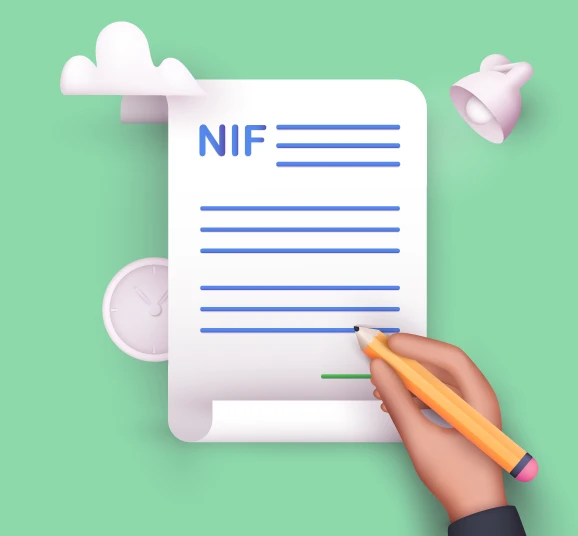
We use cookies to ensure that we give you the best experience on our website. Our
Terms and Conditions
About Cookies
Preferences, unclassified.
Living In Portugal: Essential Expat Guide 2024
Expatra Research
Last Updated:

Find The Best Quotes For Your Health Insurance Abroad
Compare quotes from the leading international health insurance providers.
This comprehensive guide on living in Portugal as an expat aims to provide essential information and insights into various aspects of moving to and settling down in Portugal, including residency, healthcare, cost of living, cultural experiences, and more.
In this guide:
- The legalities of moving to Portugal: visas and residency options for non-EU nationals.
- The cost of living : daily expenses, rentals, and real estate.
- Infrastructure : travel connections, public transport, internet.
- Healthcare and education .
- Taxes and bank accounts.
- Where to live in Portugal – an overview of the most popular expat locations.
- Culture , leisure, and socializing.
Portugal’s highlights
There are some good reasons – beyond the weather and excellent golf amenities – why Portugal is highly regarded as an ideal place to live. Its beautiful coastline, healthy lifestyle, and affordability make the country stand out among other popular southern European destinations.
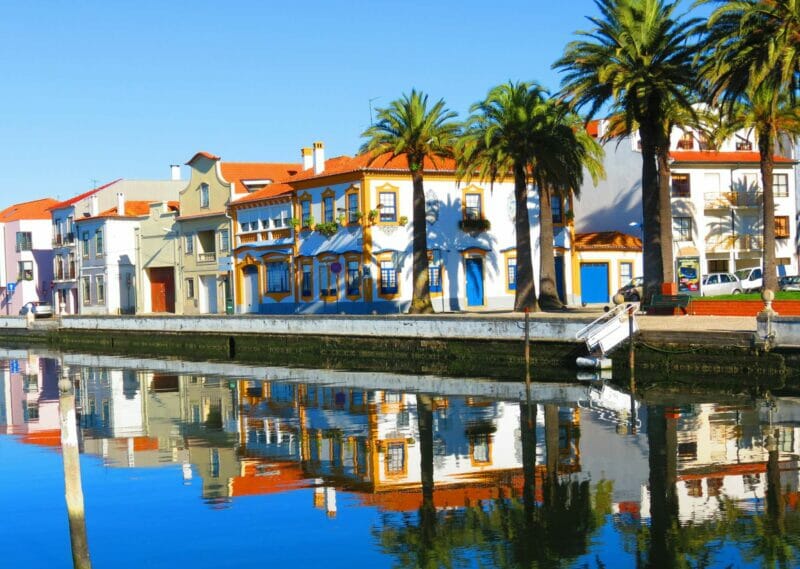
Here’s what makes Portugal such an attractive destination:
- Mild climate: Enjoy a Mediterranean climate with mild winters and sunny summers in most regions, making outdoor activities a year-round pleasure.
- Affordable cost of living: Portugal offers a relatively lower cost of living compared to other Western European and Northern American countries, especially in housing, groceries, and dining out.
- Stunning coastline: The country features beautiful beaches, cliffs, and picturesque seaside towns, ideal for leisure and relaxation.
- Rich history and culture: Portugal offers a rich history, beautiful architecture, and a diverse cultural environment to satisfy even the most demanding culture aficionados.
- Quality healthcare: Benefit from a high standard of healthcare services, including a well-established public healthcare system and access to private healthcare facilities.
- Safe and peaceful environment: The country has a low crime rate and a welcoming, friendly local population.
- Easy travel within Europe: Portugal’s convenient location provides easy access to other European countries for leisure or business.
- Diverse expat communities: Portugal is a truly global country where you can meet people and make friends from all over the world.
Portugal residency for non-EU citizens
Citizens of countries with visa agreements with the EU can travel to Portugal and stay there for up to 90 days as tourists without a visa. This includes citizens of the UK, USA, Canada, Australia and other countries.
To live and work in Portugal, you must apply for a visa and go through the immigration procedures set for all non-EU citizens, including Americans and British. The most common visas are the D7 and Portugal Golden Visa.
UK citizens can find more details in our Living In Portugal After Brexit guide.
Portugal’s Golden Visa route
A quicker option is Portugal’s Golden Visa route, where you invest a minimum of €500,000 in arts, R&D, or businesses in Portugal and gain a residency permit for a family, including dependent children.
Alternatively, you can invest in qualifying Portuguese funds, set up a business that creates jobs, contributes to R&D projects, or donate to national heritage projects.
A capital transfer of a minimum of €1,500,000 to a Portuguese bank can qualify you for a Golden Visa.
This program, among other benefits, could give you the right to apply for permanent residence and Portuguese citizenship.
If you have questions or need more information, please see our Guide to EU Golden Visa Programs or contact us via our page on Residency and Citizenship . We will be happy to help.
Portugal D7 residency visa
This is the best route to residency in Portugal for retirees and people with stable investment incomes.
You need to have sufficient passive income to qualify for this visa. The income can be received from pensions, property rentals, investments, dividends, etc.
You have to apply to the Portuguese consulate in your home country.
To qualify, you need to:
- Be a non-EU citizen.
- Have enough funds to support yourself during your stay in Portugal. Official figures say your income should at least be equal to the annual minimum wage in Portugal (which is currently €886.7) for the principal applicant and 50% of the minimum wage for a spouse. However, that might not be enough to get your application approved. Aim at a minimum of €12,000 annually to be on the safe side.
- Have a clean criminal record.
- Show a residence address in Portugal.
- Have valid health insurance.
The visa itself is permission to enter Portugal. With the visa, you can enter Portugal two times and remain there for a period of 4 months in total.
You must apply for a residence permit in Portugal during the four months. The first permit is granted for one year. After that, it can be renewed for two years.
After five years of residing in Portugal, you can convert it into a permanent residence permit.
Portugal Digital Nomad visa
The official name of the visa is “residence visa for the exercise of professional activity provided remotely outside the national territory”. This visa is designed for working professionals.
To qualify, you must:
- Come from a non-EU and non-EEA country.
- Be self-employed or employed by a company based outside Portugal
- Earn at least four times the Portuguese minimum wage per month – roughly €2,800.
The weather and various climate areas in Portugal
The weather and climate in Portugal are very diverse, so you can actually choose an area that suits your taste.

As you head further south, you’ll find hotter summers and more temperate winters. The Algarve is dry and sunny almost all year round.
If you like it seriously hot, head away from the Atlantic coastline towards the country’s beautiful interior. You’ll find the summers are baking hot!
Tip: Portugal experiences its highest temperatures inland. Amareleja, for example, in the Alentejo region, is the hottest places in Europe during the summer, having recorded 47.4°C (117.3 °F). Besides its extreme heat, Amareleja is renowned for its robust red wines and is even home to a prominent outdoor thermometer at the local Farmácia.
Here’s a brief overview of the areas with various climates:
Area | Locations | Climate |
---|---|---|
Southern Coastal Region | Algarve | Mediterranean, mild winters, hot summers |
Lisbon Metropolitan Area | Lisbon, Cascais | Mediterranean, mild winters, warm summers |
Central Interior Region | Coimbra, Tomar | Continental, cold winters, hot summers |
Northern Mountainous Region | Porto, Braga | Maritime, mild winters, mild summers |
Safety and crime
Portugal ranks as the 17th safest country in the world and second among the safest countries in the European Union. Violent crime is rare, and petty crime is largely limited to street crime during the busy tourist season.
Like in any country, there are areas with higher crime rates. Central parts of Porto and Lisbon and other urban centers tend to have higher reported crime rates compared to rural areas. This includes cities like Coimbra, Braga, Faro, and others.
The cost of living in Portugal
The cost of living is among the lowest in Western Europe.
Here is how Portugal’s cost of living, including rent, compares to some of the Northern European and North American countries:

How much money do you need to live comfortably in Portugal?
If you are going to rent (which, initially, is a good idea), two people can live comfortably in Portugal starting from about $2,000 a month, depending on the location.
The nearer to Lisbon you want to live, the bigger your budget should be.
For example, a couple can live relatively comfortably in the Algarve for $2,200 a month, including rent, occasional meals out, gym membership, and other moderate luxuries.
The same lifestyle in Cascais can cost closer to $3,100, including rent.
Housing costs
When it comes to buying a property in Portugal , the average cost across the country is cheaper than in the UK, North America, and Northern Europe.
However, if you are looking at the favorite destinations for expats retiring to Portugal, which are mostly around Lisbon, Porto, and the Algarve, you will probably find that property prices are the highest there.
For example, an average two-bedroom apartment on the Algarve coast costs at least twice as much as in central Porto. Still, for $370,000, you can buy a three-bed villa in a gated community, with access to a swimming pool and a five-minute walk to the beach.
The price of property away from the resorts and coast will surprise you even more. You can bag bargains in some stunning inland towns and villages and still only be a short drive from major urban areas and beaches.
It will mean looking at more traditional properties rather than developed resort-style homes, but you can certainly find an affordable property if you hunt for it in Portugal.
Rental costs
Rental prices can vary significantly across different regions in Portugal. Here’s a general overview:
Most expensive:
- Lisbon: The capital city, particularly areas like Chiado, Príncipe Real, and Avenida da Liberdade, tend to have higher rental prices due to high demand and a booming tourism industry.
- Porto: Porto, especially in the central and riverside districts, is known for relatively higher rental prices, although they may be slightly lower than in Lisbon.
- Cascais and Estoril: These affluent coastal areas near Lisbon are also known for higher rental prices, particularly for upscale properties and those with a sea view.
- Algarve: Certain popular tourist destinations in the Algarve region, such as Albufeira, Vilamoura, and Lagos, can have high rental prices, especially during the peak tourist season.
More affordable:
- Interior regions: Rural areas and smaller towns in the interior regions of Portugal, away from major cities and the coast, generally have more affordable rental options.
- Northern and Central Portugal: Cities like Braga, Coimbra, Aveiro, and smaller towns in the north and central parts of the country tend to offer more budget-friendly rental options than Lisbon and Porto.
- Alentejo: Rural areas and smaller towns in the Alentejo region can also provide more affordable rental rates than urban centers and coastal areas.
If you are unfamiliar with the rental process in Portugal, our guide to Renting In Portugal will provide all the necessary information on how it works and how to protect yourself as a tenant.
Retiring to Portugal
According to the Expatra Global Retirement Index , Portugal is in the top 20 countries for retiring abroad because of its climate, lifestyle, and the fact that it’s a great value-for-money destination.
The index is based on the Expatra Global Retirement Survey that asks international retirees to rate their retirement destination’s infrastructure, climate, ease of settling down, value for money, friendliness, and other aspects of life in their retirement destination.
Here’s how Portugal scores:

Retirement visa
For non-EU nationals, a D7 visa or a Golden Visa are the best options. Both options can lead to permanent residence and, eventually, citizenship. The most significant difference is that a D7 visa is cheaper to process and requires a holder to reside in Portugal permanently.
Discover more in our guide on retiring to Portugal.
Travel connections
Portugal is well-connected with Europe and major destinations worldwide.
Portugal has several major international airports, the busiest being Lisbon Airport (Humberto Delgado Airport), followed by Porto Airport (Francisco Sá Carneiro Airport) and Faro Airport (Aeroporto Internacional de Faro).
These airports have numerous direct flights from major cities across Europe, North America, South America, Africa, and Asia.
There are also connecting flights via major hub cities, including London, Amsterdam, Madrid, Frankfurt, and Dubai.
Portugal, with its extensive coastline and major ports like Lisbon, Porto, and Funchal (Madeira), is a popular stop for cruise ships in the Mediterranean and Atlantic routes.
High-speed trains, such as the Alfa Pendular and the Renfe-SNCF service, connect Portugal to Spain and further European destinations.
The renowned “Sud Express” provides an excellent link from Portugal to Central Europe. You can travel overnight from Lisbon to San Sebastian and Hendaye (France), where you can take the TGV to Paris.
Public transport
Major cities like Lisbon, Porto, and Coimbra have efficient and extensive public transportation systems, including metro, buses, trams, and suburban trains.

Rural areas, however, may have less frequent public transport options.
Trains are run by the national railway company CP (Comboios de Portugal). Alfa Pendular , a high-speed tilting train, offers a fast connection between the key cities.
Tickets and costs
Many urban areas have integrated ticketing systems, allowing passengers to use a single ticket for multiple modes of transport.
Type of Public Transport | Description | Cost |
---|---|---|
Metro | Underground train system in Lisbon and Porto. | Tickets start at around €1.50 for a single journey. |
Bus | Local and intercity buses. | Tickets usually start around €1.50 for a single journey within a city. Inter-city bus fares vary. |
Tram | Trams are mainly found in cities like Lisbon, Porto and Sintra. | Tickets typically start around €2 for a single journey. |
Train | Urban commuter trains and intercity trains. | Tickets start around €2 for short journeys within a city and can go up to €30 or more for long-distance trips between cities. |
Internet and mobile connection
Overall, you can expect a good internet experience, especially in urban areas and popular tourist destinations. Broadband or fiber optic is generally available almost everywhere and offers up to 1 Gb.
Depending on the package, it can cost you from €40 for 200 Mb to €100 for 10 Gb.
Almost everywhere in the country, you can get 4G or 4G+ service, with some cities offering 5G.
Major internet service and mobile providers are MEO, NOS, and Vodafone Portugal.
Taxes for expats in Portugal
If you spend more than 183 days in Portugal, you become a tax resident, and legally, you should now file a tax return and pay your taxes in Portugal.
To register as a tax resident in Portugal, you first must visit the town hall where you live and demonstrate that you have adequate financial means and social security to cover yourself. You then visit the local tax office and register as a tax resident.
The NHR has come to an end
One of the biggest benefits of living in Portugal as an expat, Portugal’s low-tax regime for expats, has ended.
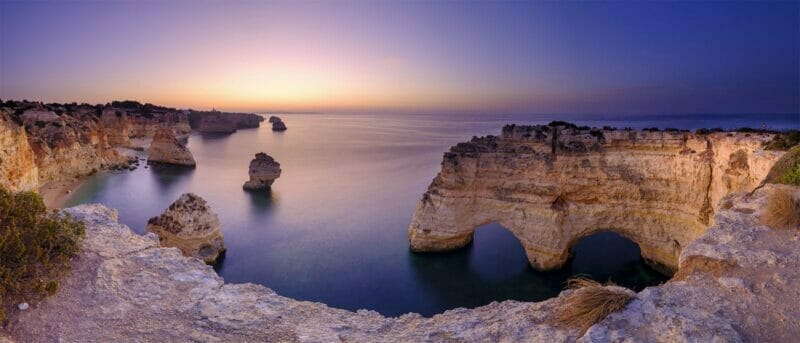
If you are planning to move to Portugal, keep in mind that the tax situation is changing, and you might have to pay Portugal’s general rates.
Various taxes and rates:
- Personal Income Tax (IRS) : Progressive rates range from 14.5% to 48%, depending on income level.
- Corporate Income Tax (IRC) : Flat rate of 21% for most businesses.
- Value Added Tax (VAT) : Standard rate of 23% for most goods and services, with reduced rates of 6% and 13% for specific items.
- Municipal Property Tax (IMI) : Varies between 0.3% and 0.45% of the property’s fiscal value.
- Stamp Duty: Varied rates depending on the type of transaction, typically ranging from 0.8% to 0.6%.
- Capital Gains Tax: Rates vary depending on the nature of the gains and the holding period, with rates ranging from 14.5% to 48%.
- Social Security Contributions: Rates range from 11% to 23.75%, depending on income and employment status.
Wealth tax in Portugal
Portugal’s wealth tax is essentially an extension of Portugal’s Imposto sobre Imóveis (IMI), or property tax. You will normally pay 0.3% for your Portuguese property if it’s worth more than EUR 600,000. If you are married or in a civil partnership, you as a couple will be granted a combined threshold of €1.2m.
For more information, read our Taxes In Portugal guide.
Where to live in Portugal
Expats’ favorite locations in Portugal can be found in and around Lisbon.
Many expats are living in Lisbon itself, with others moving to Sintra or living in Cascais to take advantage of a more relaxed lifestyle.

If you want a smaller town with a lower cost of living but still close to the capital city, consider moving to Setúbal . This lovely coastal town has everything you could only dream of while conveniently close to Lisbon.
The Algarve is another favorite expat destination , of course, where there is a large community of British, Scandinavian, French, Brazilian, and other expats.
(Approx.) | Further information | |||
---|---|---|---|---|
Lisbon | The capital city offers a vibrant cosmopolitan lifestyle. | Professionals, young couples, and digital nomads. | €400,000 – €600,000 | |
Porto | Known for its charming atmosphere and rich cultural scene. | Professionals, artists, and those seeking a bohemian vibe. | €350,000 – €450,000 | |
Cascais | A coastal town with beautiful beaches and a relaxed vibe. | Families, retirees, and beach lovers. | €450,000 – €650,000 | |
Algarve | Famous for its sunny climate and stunning coastline. | Retirees, expat communities, and golf enthusiasts. | €350,000 – €550,000 | |
Sintra | Surrounded by lush nature and historic castles. | Nature lovers, artists, and history aficionados. | €300,000 – €450,000 | |
Madeira | A tranquil island paradise with a mild climate. | Retirees, nature enthusiasts, and outdoor adventurers. | €230,000 – €350,000 | |
Faro | Located in the Algarve, offering a mix of beach and city. | Professionals, retirees, and lovers of coastal living. | €250,000 – €400,000 | |
Coimbra | Home to one of the oldest universities in the world. | Students, academics, and those seeking a cultural hub. | €220,000 – €350,000 | |
Aveiro | Known as the “Venice of Portugal” for its canals. | Families, retirees, and lovers of a picturesque lifestyle. | €240,000 – €370,000 | |
Braga | Rich in history and architectural treasures. | Young professionals, history buffs, and art enthusiasts. | €220,000 – €340,000 |
If you want to take the road less traveled, you might want to consider living in the Azores .
Island living is not for everyone, but if this is what you want, start with our guide on the best places to live in the Azores to find your perfect destination.
For more information on the most popular destinations, read our Best Places To Live In Portugal guide.
Schools and education in Portugal
If you are moving with children, schools are an essential subject. Depending on how long you are planning to stay and how old your children are, the following options are available:
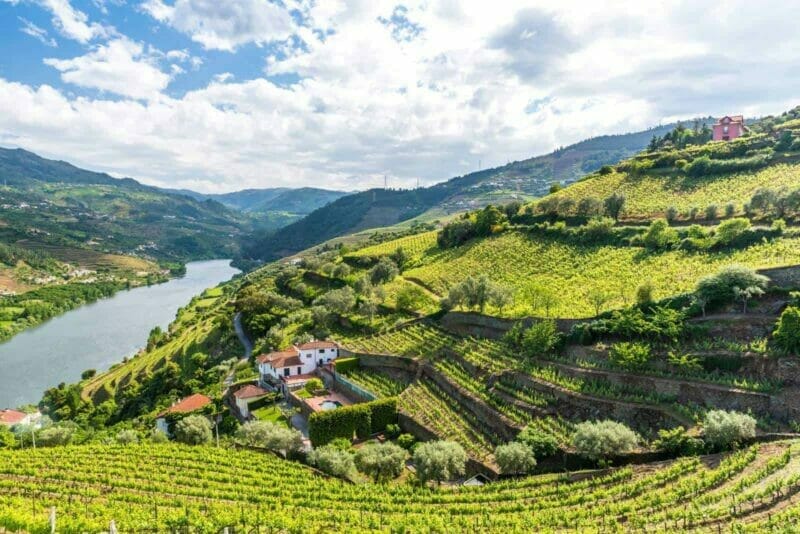
Public schools
These are state-funded schools teaching the Portuguese curriculum in the Portuguese language.
State education is quite good in Portugal, but there are a few problems, including funding, insufficient teachers, and class sizes.
Private schools
Many are faith-based, have smaller class sizes, and have more options for various clubs and activities. The costs are between €400 and €550 a month, depending on location.
International schools
They are by far the most expensive option but the best if you need to ensure your child keeps up with the UK or USA curriculum.
Most private and international schools are located in mainland Portugal in and around Porto, Lisbon, and the Algarve.
International School | Curriculum | Annual Fees (Approx.) | Location |
---|---|---|---|
International School of Lisbon | International Baccalaureate (IB) | €12,000 – €18,000 | Lisbon |
Carlucci American International School of Lisbon | American (AP) and International Baccalaureate (IB) | €15,000 – €20,000 | Lisbon |
Oeiras International School | International Baccalaureate (IB) | €10,000 – €15,000 | Oeiras, Lisbon |
St. Julian’s School | International Baccalaureate (IB) and British Curriculum (IGCSE and A-Levels) | €15,000 – €20,000 | Carcavelos, Cascais |
The British School of Lisbon | British Curriculum (IGCSE and A-Levels) | €10,000 – €15,000 | Linda-a-Velha, Lisbon |
International Preparatory School | American (AP) | €10,000 – €15,000 | Porto |
Nobel International School Algarve | British Curriculum (IGCSE and A-Levels) | €11,000 – €15,000 | Armação de Pêra, Algarve |
The English College in Portugal | British Curriculum (IGCSE and A-Levels) | €8,000 – €12,000 | Quinta do Lago, Algarve |
Vale Verde International School | International Baccalaureate (IB) | €8,000 – €12,000 | Loulé, Algarve |
Vilamoura International School | British Curriculum (IGCSE and A-Levels) | €10,000 – €15,000 | Vilamoura, Algarve |
Healthcare in Portugal
Portugal is famous for its fantastic state-funded healthcare system, which is called the Serviço Nacional de Saude , or SNS for short.
This scheme is open to all permanent legal residents in Portugal and those with temporary residency who are either working and actively making social security contributions or from a country that shares a reciprocal healthcare agreement.
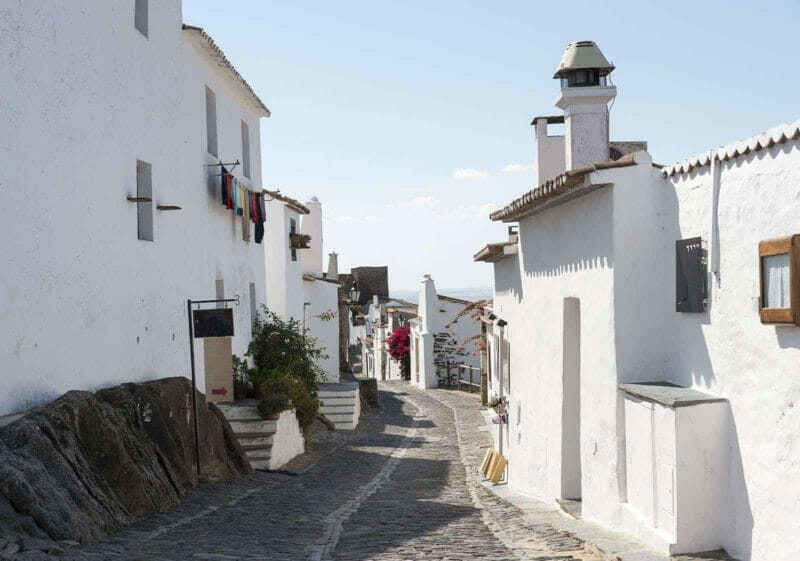
New arrivals in Portugal must live as temporary residents for five years before qualifying for permanent residency—so some retirees may need health insurance to bridge the gap.
Happily, private health insurance is far more affordable in Portugal than in most other countries too!
EU citizens arriving with a valid European Health Insurance Card (EHIC) or equivalent can use the Portuguese healthcare system for free for 90 days—allowing them time to get their Segurança Social registration in order.
Once eligible to enter the SNS system, expats will need to register at their local Centro de Saude , or health center, to receive a Numero de Utente and be assigned a family doctor.
Once you have your SNS patient number in hand, healthcare in Portugal is almost entirely free.
There are no costs for emergency healthcare—including trips to the emergency room and even a ride in an ambulance.
Non-essential healthcare can be accessed via small administrative charges called ‘ taxas moderadoras ’, which are so affordable that you’ll only need to pull €4.50 from your wallet to visit your family doctor!
Family dependents of those who qualify will also be able to register.
Health insurance
Private health insurance is quite popular in Portugal—in fact, many Portuguese citizens supplement their SNS healthcare to enjoy access to private services and potentially shorter wait times.
Health insurance in Portugal can begin from as little as €30 per month, making it an accessible option for most expats. However, do keep in mind that insurance will be subject to an initial health assessment, and costs can be higher for those with pre-existing conditions.
Also, if you are 65 and over, you will find that most insurers will be unwilling to provide you with a health plan. If this is the case, you should look at international health insurance. To ensure you get the best value for money, compare international health insurance options from various providers to find the best deal.
Because of the popularity of private services, private clinics can be found all over the country, and expats can choose to visit a private doctor without health insurance for as little as €40.
Wherever you go, either practice your Portuguese or get a translator ready on your smartphone. Not all doctors speak English, and, particularly in rural areas, it’s safe to assume that most won’t!
Hospital care and specialist services in Portugal
Unless it’s an emergency, treatment at a hospital requires a referral from a family doctor.
Referrals can also be provided for cheaper access to specialist services such as physiotherapy or mental healthcare.
The SNS system does not cover dental, except for those considered high-risk.
If opening a policy, your health insurance provider can include dental upon request.
However, once again, dental care is refreshingly affordable. With no coverage, a filling in Portugal costs only around €40, which should be cheap enough to keep you smiling.
Personal banking and bank accounts in Portugal for expats
You might want to open a bank account in Portugal before you move there. It can help you save money on charges for transactions abroad from your bank.
Opening a resident account in Portugal
Personal bank accounts in Portugal are not always free. Many current accounts have an admin charge of about €5 per month. You will also have to pay small fees to replace debit cards or counter withdrawals.
For that, you will get an assigned manager and have a direct line and mobile number. Whenever something happens that requires help, you can talk to them on the phone or go into your local branch and talk to them in person.
Documents needed to open a bank account in Portugal
To open a Portuguese bank account, you will need the following:
- your passport;
- your NIF (a Portuguese fiscal number which you can obtain at the local Financas );
- proof of income;
- proof of your Portuguese address;
- a mobile phone number with activated SMS.
Opening a non-resident bank account in Portugal
There might be circumstances when you need to have a Portuguese bank account before you apply for residency. This is what you can do to obtain a Portuguese bank account before you move to Portugal:
Some Portuguese banks offer this option. Banks such as ActivoBank and Banco CTT offer excellent services, which include no monthly fees and are free to use in the eurozone.
You have to be in the country to open a non-resident account, as it involves first obtaining a NIF ( Número de Identificação Fiscal ). You can get it from the nearest Finanças by providing your ID and proof of address (a recent bank statement that lists your non-Portuguese address will do perfectly well).
With your freshly obtained NIF, proof of address, and proof of income that states your profession or job title, you can apply for a non-resident bank account.
If you already have your NIF, both ActivoBank and Banco CTT (and several Portuguese banks) offer an online application that involves a video chat with a bank representative to confirm your identity.
How to open a bank account in Portugal online – a mobile-only bank account
You can opt for a mobile-only bank account. They are very easy to set up. The most popular mobile-only bank accounts are Borderless Account from TransferWise, DiPocket, Revolut, LeuPay, and N26.
With most of them, you can set up a bank account with a Portuguese IBAN on your mobile phone in minutes. You will need a smartphone and a valid ID, which will allow you to verify your identity either through a quick video call or with photos.
Cultural insights, leisure, and integration
Overall, Portugal is known for its welcoming and friendly culture, making it relatively easy for newcomers to integrate into the society.
However, it can be very helpful to familiarize yourself with certain aspects of Portuguese lifestyle and culture and, of course, make an effort to learn the language.
The language barrier
The most difficult thing about Portugal is the language. It is a very complicated and challenging language, and many people struggle to get further than the basics and everyday pleasantries.
If you live in the more urban and populated areas with many expats, international citizens, and professional Portuguese, you will find English is quite widely spoken. The Algarve , for example, is being anglicized rapidly thanks to international expats.
Step away from the major urban and tourist hubs, and you will be struggling with daily communications. So learning the language is highly beneficial and will help you integrate and make friends.
The major national holidays and festivals celebrated in Portugal
Besides traditional Christmas and New Year, here are some of the festivals you can enjoy in Portugal:
- Carnival (Carnaval) : A lively celebration in February or March with colorful parades, costumes, and street parties before the start of Lent.
- Good Friday (Sexta-feira Santa) : Observed during Holy Week on the Friday before Easter Sunday, commemorating the crucifixion of Jesus Christ.
- Easter Sunday (Páscoa) : Celebrated in March or April, it marks the resurrection of Jesus Christ. Families gather for special meals and festivities.
- Freedom Day (Dia da Liberdade) : Held on April 25th, commemorating the Carnation Revolution in 1974, signaling the end of the Estado Novo regime.
- Labour Day (Dia do Trabalhador) : Celebrated on May 1st to honor workers and their contributions. It is marked by rallies, demonstrations, and cultural events.
- Portugal Day (Dia de Portugal) : Celebrated on June 10th, it commemorates Portugal’s national identity and its greatest literary figure, Luís de Camões.
- Feast of St. John (Festa de São João) : Celebrated on the night of June 23rd, particularly in Porto, with lively festivities, music, dancing, and a traditional midnight bonfire.
- Assumption of Mary (Assunção de Nossa Senhora) : Observed on August 15th, it marks the assumption of the Virgin Mary into heaven, a significant religious holiday.
- Republic Day (Implantação da República) : Celebrated on October 5th, commemorating the establishment of the Portuguese Republic in 1910.
Things to do
Portugal is a brilliant country to be in if you are an active and outdoorsy type. The mild climate means various outdoor activities can be enjoyed all year round.
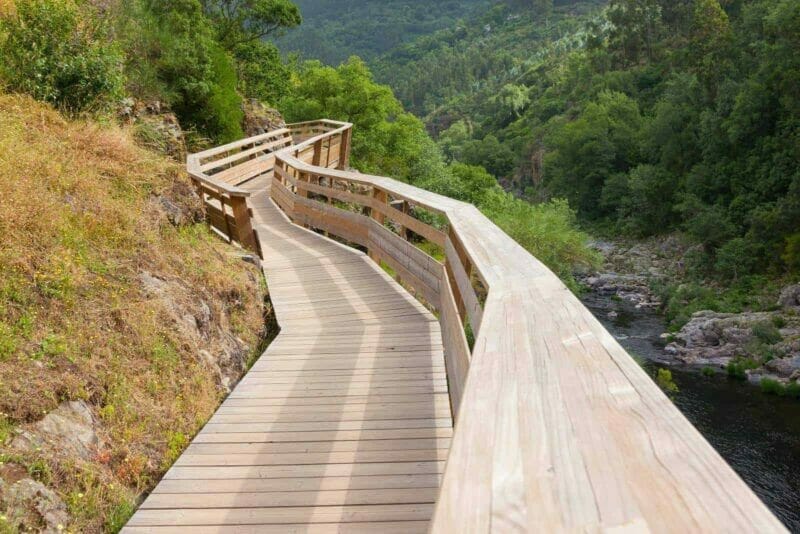
Surfing and water sports
Portugal’s extensive coastline provides excellent conditions for surfing, windsurfing, kitesurfing, and paddleboarding. Knowledgeable people say the country boasts 364 days of surf. Spots like Nazaré, Peniche, and Ericeira are renowned among surf enthusiasts.
Hiking and trekking
Explore the diverse landscapes of Portugal through its extensive network of hiking trails. The Douro Valley, Peneda-Gerês National Park, and Rota Vicentina offer stunning trekking opportunities.
Cycling and mountain biking
Discover Portugal’s picturesque countryside and challenging mountain terrains on two wheels. The Algarve, Douro Valley, and Serra da Estrela are popular cycling destinations.
Portugal is a prime golf destination with world-class golf courses.
There are only 70 or so golf courses in the country, and the total number of golf club members is below 20,000. But despite (or maybe thanks to) these modest numbers, the quality of the courses is very high, and the country’s popularity as a top golfing destination has been steadily growing.

The Algarve, Lisbon, and Porto regions are particularly known for their exceptional golfing facilities and scenic courses.
Rock climbing
Adventure seekers can enjoy rock climbing in various regions, including Sintra, Cascais, and the Arrábida Natural Park, offering diverse climbing experiences for enthusiasts.
Sailing and yachting
Portugal’s extensive coastline and marinas invite sailing and yachting enthusiasts. The Algarve, Lisbon, and Porto Santo are popular yachting destinations.
For all the yachting enthusiasts, here is the list of Portugal’s most popular marinas and yacht clubs:
- Marina de Vilamoura
- Marina de Lagos
- Marina de Cascais
- Marina de Portimão
- Marina de Albufeira
- Marina de Faro
- Marina de Sines
- Marina de Peniche
- Marina de Ponta Delgada
These marinas and yacht clubs offer excellent facilities, stunning coastal views, and a wide range of services to satisfy your needs.
Bird watching
Portugal’s diverse ecosystems provide rich birdwatching opportunities. Ria Formosa, Tejo Estuary, and Peneda-Gerês National Park are renowned birdwatching spots.
Dolphin and whale watching
Experience the thrill of observing dolphins and whales off the Portuguese coast. The Azores and Madeira are well-known for guided boat tours to witness these magnificent marine creatures.
Canoeing and kayaking
Enjoy paddling adventures in rivers, lakes, and coastal areas. The Douro River, Mondego River, and Aveiro Lagoon offer exciting canoeing and kayaking experiences.
Paragliding and skydiving
Adrenaline junkies can take to the skies for paragliding or skydiving experiences. Regions like Algarve, Madeira, and Porto offer thrilling opportunities for aerial adventures.
Final thoughts on living in Portugal
Portugal seems to have found the right recipe for becoming an attractive expat destination. It offers exactly what all expats are looking for when deciding to move: a good climate, great quality of life combined with a lower cost of living, and, a cherry on top of the cake, tax advantages.
This is exactly what you can get living in Portugal as an expat. Even if you cannot benefit from the tax advantages, all the other benefits are convincing enough to make Portugal a great destination to live in.
You might find helpful:
- Best Places To Live In Portugal – a detailed overview of Portugal’s most popular locations for expats.
- The Expat Guide To UK Pensions Abroad: Understand Your Options .
- The Essential Guide To Cost-Effective International Removals – read about international removals options and how you can keep your costs down while getting a quality service.
- Didn’t find what you were looking for or need further advice? Contact us or comment below with your question, and we will do our best to help.
Helpful external links:
- Online registration with a health center in Portugal – eportugal.gov.pt.
- Portugal public schools rating – observador.pt.
- Entering Portugal, the documents and travel information – eportugal.gov.pt.
- US citizen services in Portugal – pt.usembassy.gov.
- Portuguese public and private hospitals and their contact details – infarmed.pt.
- Online services for foreign nationals in Portugal – sef.pt .
- UK government guidance for British citizens moving to Portugal – www.gov.uk .
About the author

Our most popular evergreen guides are kept fresh and up-to-date by our in-house research team. If you have any questions about our guides or the country discussed feel free to contact us or leave a comment below and we’ll get back to you.
25 responses to “Living In Portugal: Essential Expat Guide 2024”
Just reading a lot of your info on moving to Portugal. Lots of things I see seem to indicate you need to be a high worth individual. Can folks with moderate retirement income still make the move from US to Portugal?

Hi Tracy, Thanks for the comment. You do not need to be a millionaire to retire to Portugal. As long as your income qualifies you for a D7 visa (which is under €10,000 a year) and you opt for an affordable area to live, it’s all doable. €1500 a month should be enough for a single person to live in a smaller town with all necessary amenities.
We’re ex-pats from the USA living in Porto. My son bought a house that we’re renovating. We have a plumbing problem we need solved. Whenever one of us takes a shower, no one can use the toilets, sinks or anything with water because the shower water suddenly blast very cold water. My son is disabled with spinal stenosis from the neck down. A cold blast of water results in great pain. Who or what plumbing company can help us?
Hi Grete, thanks for commenting. I asked around in our Porto’s network, someone came up with the following: “I can suggest two service platforms where you can submit a proposal, and providers are ranked by other users: fixando.pt and zaask.pt. I have personally used both platforms and achieved positive results for home services.”. I hope this will help,
My wife and I would like to retire in Portugal when we turn 65. We currently live in the US and are US citizens. Do you know if we will still be able to collect US social security while living in Portugal?
Hi Allan, yes, it looks like you will be able to receive your social security payments if you move to Portugal. Here’s the tool for you to check: https://www.usa.gov/social-security-abroad/
Wishing you all the best,
Hi, my husband and I plan on retiring in Portugal in the next couple of years. We would love for a recommendation of a Lawyer and Tax Attorney to discuss our situation. I’m finding conflicting information on how U.S. Social Security will be taxed and have questions on the D7 Visa and NHR Discount. Thank you so much for any assistance!

Hi Lianne, We have an amazing expert in Portugal helping our readers with all their legal and tax issues. Please, get in touch with Joana Torres Fernandes via her Expatra profile: https://expatra.com/author/joanatfernandes/ . Good luck with your relocation.
Thank you for an excellent article.
I have dual citizenship (DK and USA) and my wife is a USA citizen. I assume I will not have too many problems living/retiring in Portugal, but what about my wife? Do I have to apply for family reunification, and are the rules as stringent/creasy as in DK?
Thanks, Claus
Hi Claus, It shouldn’t be difficult for your wife to join you in Portugal as you are an EU citizen. Generally, non-EU members will have to apply for a residence permit as a family member of an EU national. This might clarify the situation a bit: https://europa.eu/youreurope/citizens/residence/family-residence-rights/non-eu-wife-husband-children/index_en.htm You might want to check it with a lawyer. Our Portugal expert Joana and her team can help you with this: https://expatra.com/author/joanatfernandes/
@Ola Degteva,
Thank you for your quick response.
When the time comes, I will certainly contact a lawyer.
Hi, just wanted to know if there is any advantages, paperwork facilities or agreements for mexicans?
Hello Jorge, The application to become a Portuguese resident is the same for all all non-EU citizens and there are several types of Residence Permits in Portugal, depending on your goals. For more information on the visa application process, you can check out https://www.lvpadvogados.com/ I hope this helps! All the best, Joana
Planning a vacation/holiday this year to experience Portugal. Are there any immigration tours? Someone who takes you around with information about the best way to handle the process for retirement?
Hello Nancy, I’m afraid I have never heard of Immigration tours here in Portugal. However, my team and I will gladly assist you with all matters regarding your relocation to Portugal, once you decide to take that step. For more information, please check https://www.lvpadvogados.com/ I hope I was able to help! All the best, Joana
My wife and I own a property in The Algarve for around 15 years and we visit regularly. So regular that we are in danger of breaching the 90 day Schengen allowance. We plan to retire in a couple of years from the UK to live permanently in Portugal but what is the best Visa for us to have so we don’t fall foul of the 90 days please
This is an excellent article, the best-written I have seen on the topic.
Where can I find out about moving to Madeira [in Portugal] and if the visa process, healthcare, shipping household goods, and other information ? I would also like to find a recommended real estate agent in Madeira who speaks English and works with ex pats -Thanks -Collette F
Hi Collete, you will find more information on moving to Portugal here: https://expatra.com/guides/portugal/living-in-portugal-the-expats-guide/ We can also put you in touch with a relocation specialist who helps expats in every step of the move: from finding a property to removals, to paperwork, visas, bank accounts, etc. If you are interested, please contact us here: https://expatra.com/contact/
Hi – a healthcare query – can you clarify if EU passport holders who don’t have a European Health Insurance Card can access basic NHS services straightaway they become residents in Portugal or do they need to wait 5 years?
EU citizens do not need to wait for 5 years, however, having a EHIC can be very useful in case you need healthcare access while you are registering with local health authorities.
I have a similar query to Emmet. I am a U.K citizen and resident. However I also hold dual nationality with an EU country but have never had residency in the EU. My husband (British) and I are looking to retire to Portugal in the near future and possibly buy property there. What is my position regarding this, especially re healthcare as I believe it is residency rather than nationality based ? Also any comments on my husband’s situation / our joint situation would be welcome. We both have GHIC cards.
I am not qualified to give any immigration advice, you need professional help in your situation. Let me see if I can get an immigration professional to comment.
Thanks for this.
Hi Fiona, sorry for the delay. I have just emailed you the contact details of a lawyer who could help you with your questions.
Leave a Reply Cancel reply
Your email address will not be published. Required fields are marked *
Save my name, email, and website in this browser for the next time I comment.
Latest Posts

Malaysia My Second Home (MM2H) Retirement Visa: A 2024 Update
The key information about the Malaysia My Second Home (MM2H) program and its major 2024 update.

Retiring In Malaysia: Your Complete Guide To A Rewarding Expat Life
Thinking of retiring to Malaysia? We uncover the essential facts and information that you need to succeed.

Living In Florianópolis, Brazil, As An Expat Retiree
All about Florianópolis as a retirement destination: the best neighborhoods and beaches, costs, things to do and culinary delights – see why Floripa might be your perfect retirement spot.
Don't be surprised if UEFA Euro 2024 isn't Cristiano Ronaldo's last hurrah with Portugal
Portugal might be one of the best sides at Euro 2024 , but as been the case for the past two decades, most of the talk around in the lead-up to the tournament has been about the status of superstar Cristiano Ronaldo .
Now 39 years old and the most prolific goal scorer in international history, Ronaldo will be playing in his record sixth European championship , having made his first appearance at Euro 2002 as an 18-year-old.
Ronaldo has been mostly out of the public eye for the past 18 months, playing for Al Nassr in Saudi Arabia on a contract rumored to earn him in the high nine figures annually. At his age and having spent more than a year against lesser competition, fans and pundits alike wonder what Ronaldo, still Portugal's captain, can offer the national team back on the world's biggest stage.
Ronaldo might not be as quick as he was in his prime, but he scored 50 goals for Al-Nassr last season and 10 times in Portugal's Euro 2024 qualifying campaign. His nose for goal clearly hasn't faded.
“For us he’s a goalscorer, someone who can make that final move, stretch defenders, open spaces," Portugal manager Roberto Martinez said. "He has changed his way of playing slightly, but he’s in on merit and the numbers are there to back that up.”
In all likelihood, Ronaldo will come off the bench in this tournament. That's not something totally new, considering he didn't start either of Portugal's two knockout round games at the 2022 World Cup — albeit when he was out of form and favor in the final weeks of his second Manchester United tenure.
"Cristiano is prepared to help the team and give everything he can give," Martinez said. "And there is no other player in the world of soccer who can bring what Cristiano can to the dressing room."
Ronaldo helped Portugal win its only European championship back in 2016, a stunning run capped by an extra-time win over host France in the final , a match that Ronaldo was forced out of by injury.
"He represents inspiration, he represents that everything is possible, he represents that you can dream and you can achieve it," Portugal defender Ruben Dias said.
"It's obviously a pleasure to have him with us in this moment of his career. He represents to us that he really wants to win again and he's our captain and obviously we follow him to the end."
Portugal kicks off Euro 2024 against the Czech Republic on Tuesday in Leipzig, but will this even be Ronaldo's final tournament?
Martinez said that Ronaldo , already the first player in history with 200 international appearances, has expressed interest in reaching 250.
And if he just keeps scoring goals, it won't be shocking to see him in Portugal's squad as a 41-year-old for the 2026 World Cup.
Contributing: Reuters

- My Favorites
You have successfully logged in but...
... your login credentials do not authorize you to access this content in the selected format. Access to this content in this format requires a current subscription or a prior purchase. Please select the WEB or READ option instead (if available). Or consider purchasing the publication.

- Disclaimers
Significant educational progress but challenges remain
An experienced and qualified teaching body but ageing, lacking the benefits of local management and with limited opportunities for collaboration, educational supply has considerably diversified but may be too fragmented at the upper secondary level, portugal’s higher education system has expanded but needs to contribute further to the upskilling and reskilling of the adult, covid-19: the second year of the pandemic, more information.
In Portugal, the share of 25-34 year-olds with tertiary attainment increased substantially over the last two decades (from 13% in 2000 to 47% in 2021). Although the tertiary education is the most common highest level of attainment among Portuguese young adults, there is still 17% of 25-34 year-olds without upper secondary attainment, 3 percentage points higher than the OECD average.
The spending per student across primary to tertiary education in Portugal is lower than the OECD average. In 2019, Portugal spent USD 10 535 per student (in equivalent USD converted using PPPs for GDP) compared to the OECD average of USD 11 990 per student.
Between 2015 and 2021, the statutory salaries of lower secondary teachers in Portugal increased 3%, less than the average across OECD countries (6%). Lower secondary (general programmes) teachers in Portugal earn 33% more than other tertiary-educated workers. Portugal is one of the few countries where teachers’ average actual salaries remain higher than earnings of tertiary-educated workers since, as a group, the teaching population is ageing and, consequently, a large proportion of teachers are close to the top of their teaching career.
Together with salaries, the working hours present some key measures of the working lives of the teachers. Following the OECD countries, the number of teaching hours per year required from teachers in Portugal tends to decrease as the level of education increases. The annual teaching hours are 965 hours per year at pre-primary level, 869 hours at primary level, and 667 hours at lower secondary level and at upper secondary level (general programmes).
For teachers, the proportion of their statutory working time spent teaching provides information on the amount of time available for non-teaching activities. In Portugal, teachers at the upper secondary level formally dedicated 51% of their working time to non-teaching activities. The average across OECD and partner countries is 56%.
The share of all 17-years-old Portuguese enrolled in the general upper secondary programmes (mainly scientific-humanistic upper secondary courses) is higher than the share enrolled in the upper secondary vocational education (57% against 35%) Still, both percentages are slightly above the OECD averages.
In Portugal, the average age of graduation from vocational upper secondary education is 20 years bellow the OECD average at 22 years old. All the graduates from vocational upper secondary have direct access to tertiary education.
In 2020, the average age of new entrants into long first degree, bachelor’s and master’s programmes were 19, 21 and 24 respectively (against OECD averages of 21, 22 and 27, respectively). The lower representation of the adult (18 years old or more) population among the new entrants in the tertiary education indicates Portugal needs to contribute further to the upskilling and reskilling of this population.
Trends in educational attainment in Portugal
Educational attainment has been increasing throughout the OECD, in particular at tertiary level. Between 2000 and 2021, the share of 25-34 year-olds with tertiary attainment increased on average by 21 percentage points. In Portugal, the share increased at an even faster pace, by 35 percentage points (from 13% in 2000 to 47% in 2021) (Figure 1). Portugal is one of the 24 OECD countries where tertiary education is the most common highest level of attainment among 25-34 year-olds.

1. Data for tertiary education include upper secondary or post-secondary non-tertiary programmes (less than 5% of adults are in this group).
2. Year of reference differs from 2000: 2002 for Israel and 2003 for Iceland.
Countries are ranked in descending order of the difference in the share of tertiary-educated 25-34 year-olds between 2000 and 2021.
Source : OECD (2022), Education at a Glance Database, http://stats.oecd.org/ . See Source section for more information and Annex 3 for notes ( https://www.oecd.org/education/education-at-a-glance/EAG2022_X3-A.pdf ).
Upper secondary attainment is often seen as a minimum qualification for successful labour market participation. Although the general increase in educational attainment has seen a parallel decline in the share of 25-34 year-olds without upper secondary attainment, 14% of young adults across the OECD still left school without an upper secondary qualification. In Portugal, the share is 17%, which is higher than the OECD average.
In Portugal, there has been a considerable improvement in participation among diverse student groups due to the strong policy focus on inclusion in education, with the establishment of comprehensive frameworks to provide individual support to all students who need it. Nevertheless, students’ background and personal characteristics still have a significant impact on their educational outcomes (OECD, 2022[1]) .
Financial resources invested in education
All OECD countries devote a substantial share of national output to educational institutions. In 2019, OECD countries spent on average 4.9% of their gross domestic product (GDP) on primary to tertiary educational institutions. In Portugal, the corresponding share was 4.8%. Between 2008 and 2019, funding for educational institutions from all sources grew by 12% in Portugal. Over the same period, the increase in GDP was lower 5%. Consequently, expenditure on educational institutions as a share of GDP grew by 0.3 percentage points over the same time period (from 4.5% to 4.8%). Public spending on primary to tertiary education was 10% of total government expenditure in Portugal, also lower than the OECD average (10.6%) (Figure 2).
Spending on educational institutions as share of GDP or public budgets are important measures that countries place on education in their budgeting decisions. However, they do not show the total amount of funding per student because GDP levels, public budgets and student numbers vary from country to country. Across primary to tertiary education, OECD countries spend an average of USD 11 990 per student (in equivalent USD converted using PPPs for GDP) on educational institutions each year. In comparison, Portugal spent USD 10 535 per student in 2019. Its cumulative expenditure on educating a student from the age of 6 to 15 was USD 98 983, which was slightly below the OECD average of USD 105 502.
Across OECD countries, the provision of education at primary and secondary levels in terms of curricula, teaching styles and organisational management leads, on average, to similar patterns of expenditure per student from primary to post-secondary non-tertiary levels. OECD countries as a whole spend on average around USD 9 923 per student at primary and USD 11 400 per student at secondary level. In Portugal, the values are USD 8 992 at primary and USD 11 162 per student at secondary level.
In contrast to lower levels of education, spending on tertiary education varies widely across OECD countries. Expenditure per student at tertiary level in Portugal is higher than at other levels of education, as is the case in almost all other OECD countries. The average expenditure per student in Portugal is USD 11 858 per year, which is about USD 2 900 higher than that of the primary level and USD 700 higher than that of the secondary level. It is among the lowest across OECD countries. The average expenditure at tertiary level (USD 17 559) is driven up by high values in a few countries. At 25%, the share of research and development (R&D) expenditure makes up a smaller fraction of expenditure on tertiary education in Portugal than on average across OECD countries (29%).
Public funding dominates non-tertiary education (primary, secondary and post-secondary non-tertiary) in all OECD countries, even after transfers to the private sector. Private funding accounts for 10% of expenditure at primary, secondary and post-secondary non-tertiary levels on average across OECD, while this share was 11% in Portugal in 2019. At the tertiary level the share of private expenditure reached 31%, which was the same as the OECD average.

1. 1. Year of reference differs from 2019. Refer to the source table for more details.
2. 2. Primary education includes pre-primary programmes.
Countries are ranked in descending order of total public expenditure on education as a percentage of total government expenditure.
Source : OECD/UIS/Eurostat (2022), Table C4.1. See Source section for more information and Annex 3 for notes ( https://www.oecd.org/education/education-at-a-glance/EAG2022_X3-C.pdf ).
Labour market outcomes
Higher educational attainment is often associated with better employment prospects and Portugal is no exception. In 2021 the employment rate among 25-34 year-olds with tertiary education in Portugal was 14 percentage points higher than among those with below upper secondary attainment and 5 percentage points higher than among those with upper secondary or post-secondary non-tertiary attainment. On average across OECD countries, the employment rate among 25-34 year-olds with a tertiary qualification was 26 percentage points higher than among those with below upper secondary attainment and 8 percentage points higher than among those with upper secondary or post-secondary non-tertiary attainment. However, Portugal still has 70% of the 25-34 year-olds with below secondary attainment employed which is 12% higher than the OECD average.
While the positive link between educational attainment and employment rates holds for both men and for women across the OECD, it is particularly strong for women. In Portugal, 63% of women with below upper secondary attainment were employed in 2021, compared to 86% of those with tertiary attainment. In contrast, the figures were 74% and 80% for men.
On average, tertiary attainment generates a wide range of labour-market benefits, including high employment rates. Yet, there are significant differences depending on the field of study. In 2021, employment rates in Portugal were highest among tertiary-educated individuals who studied information and communication technologies with 96% and lowest among those who studied natural sciences, mathematics and statistics at 83%. However, these differences need to be put into perspective. Even among 25-64 year-olds with tertiary attainment in the field with the lowest employment rate, this was 0.3 percentage points higher than among those with upper secondary attainment (all fields combined).
Across the OECD, the labour market benefits of tertiary attainment have proved especially strong during economic crises. This was also the case during the COVID-19 pandemic in Portugal. Between 2019 and 2020, unemployment for 25-34 year-old workers with below upper secondary attainment increased by 2.3 percentage points, by 3.5 percentage points for workers with upper secondary attainment and by 1.2 percentage points for workers with tertiary attainment. In 2021, unemployment for workers with below upper secondary attainment remained constant, compared to 2020 while it rose by 0.8 percentage points for workers with upper secondary attainment and decreased by 0.4 percentage points for workers with tertiary attainment.
Educational attainment affects not just employment prospects, but also wage levels. On average across the OECD, 25-64 year-old workers with upper secondary or post-secondary non-tertiary attainment earn 29% more than workers with below upper secondary attainment, while those with tertiary attainment earn about twice as much. In Portugal, the earnings advantage of tertiary-educated workers was even greater than the OECD average. In 2020, workers with upper secondary or post-secondary non-tertiary attainment earned 25% more than those with below upper secondary attainment and those with tertiary attainment earned more than twice as much.
Wages also differ according to the field of study. In Portugal, tertiary attainment in information and communication technologies generates the highest earnings. Full-time full-year workers aged 25-64 with a tertiary degree in this field earn on average more than twice as much as workers with upper secondary attainment (all fields combined). In contrast, tertiary attainment in arts leads to the lowest wages. Workers with this educational background earn on average 26% more than the wage of workers with upper secondary attainment (all fields combined).
The share of young people neither employed nor in formal education or training (NEET) provides indications on the transition from education to the labour market. In Portugal, 14.3% of young people in the age group 18-24 are NEET, 1.8 percentage points lower than the OECD average. In the age group 25-29 this percentage is 16.3%, still below the OECD average (17.6%).
While teachers should meet minimum qualification requirements to enter the teaching profession and become fully qualified teachers, some teachers may have higher qualification levels. The most prevalent level of qualification of teachers aged 25-64 among the 25 OECD countries and other participants with available data is the bachelor’s degree. In Portugal, at all levels of school education, the proportion of teachers aged 25-64 with a master’s degree or higher, is about 88%. The high qualification levels of teachers in Portugal are explained by the requirement that teacher candidates undertake pedagogical training at master’s level in education, as part of pre-service preparation.
The teaching profession in Portugal is experienced and ageing. At all levels of the education system, 45% or more teachers are above 50 years of age, a proportion that is above the OECD average of about 40%. (OECD, 2021[2])
The salaries of teachers and school heads are an important determinant of the attractiveness of the teaching profession, but they also represent the single largest expenditure item in formal education. In most OECD countries, the statutory salaries of teachers (and school heads) in public educational institutions increase with the level of education they teach, and also with experience. Actual salaries also increase with the level of education. On average across OECD countries, actual salaries range from USD 41 941 at the pre-primary level to USD 53 682 at the upper secondary level. In Portugal actual salaries average USD 52 095 at pre-primary level and USD 50 209 at upper secondary level. It is worth noting that in Portugal, regardless of the teaching level, all teachers are paid on the same salary range, which consists of ten levels of four years each, except the fifth level which lasts two years. Therefore, the differences observed in actual salaries between levels of education results from differences in the structure of the teacher population by experience between levels of education.
Between 2015 and 2021, on average across OECD countries, the statutory salaries of teachers at lower secondary level (general programmes) with 15 years of experience and the most prevalent qualifications increased by 6% in real terms. In Portugal, salaries increased less than the OECD average, by 3%.
Teachers’ average actual salaries remain lower than earnings of tertiary-educated workers in almost all OECD countries, and at almost all levels of education. However, Portugal is one of the few exceptions to this rule, since, as a group, the teaching population is ageing and, consequently, a large proportion of teachers are close to the top salaries in their teaching career. Lower secondary (general programmes) teachers in Portugal earn 33.1% more than other tertiary-educated workers. Likewise, school heads’ actual salaries in Portugal are much higher than the earnings of other tertiary educated workers. This is similar to most OECD countries, where school heads tend to earn well above the average earnings of tertiary educated workers.
Together with the salaries, teaching hours and the extent of the non-teaching duties may also affect the decision to join the teaching profession. The average number of teaching hours per year required from a typical teacher in public educational institutions in OECD countries tends to decrease as the level of education increases. This is also the case in Portugal. Based on official regulations or agreements, annual teaching hours in Portugal are 965 hours per year at pre-primary level, 869 hours at primary level, 667 hours at lower secondary level (general programmes) and 667 hours at upper secondary level (general programmes) (Figure 3).
Regulations in Portugal specify teachers’ total annual statutory working time and the allocation of time spent at school. At the upper secondary level, 51% of teachers’ working time is formally dedicated to non-teaching activities in Portugal, compared to an average of 56% across OECD and partner countries. During their working hours, teachers also perform various non-teaching tasks such as lesson planning and preparation, marking students’ work and communicating or co-operating with parents or guardians.
Participation in professional development activities is considered an important responsibility of teachers at all levels of education, as it is mandatory for all teachers at all levels in 25 countries and other participants. Only four countries, including Portugal, allow teachers to participate in professional development activities at their own discretion at all levels. However, Portuguese teachers engage little in significant collective learning activities and many never participate in such activities as co-teaching or peer observation. Portuguese teachers rarely benefit from formal induction programmes and almost no classroom observations of teaching practice occur. Similarly, Portuguese school leaders have access to minimal ongoing professional development for the purposes of developing their instructional leadership capacities (Liebowitz et al., 2018[3]) .
While Portugal benefits from experienced and well-paid teaching staff, to better improve the learning environment and the organisation of schools there could be benefits from increasing relevant opportunities for the professional development of teachers, greater incentives to engage in collaborative work and giving more autonomy to schools to choose the teachers whose profiles better suit their needs (Liebowitz et al., 2018[3]) .

1. Actual teaching time (in Latvia except for pre-primary level).
2. Reference year differs from 2021. Refer to the source table for details.
3. Average planned teaching time in each school at the beginning of the school year.
Countries and other participants are ranked in descending order of the number of teaching hours per year in general upper secondary education.
Source : OECD (2022), Table D4.1. See Source section for more information and Annex 3 for notes ( https://www.oecd.org/education/education-at-a-glance/EAG2022_X3-D.pdf ).
Compulsory education begins at the age of 6 and ends at the age of 18 in Portugal. The range of ages for which at least 90% of the population are enrolled is longer than the period of compulsory education and goes from the age of 4 to the age of 17. This is similar to most other OECD countries, where more than 90% of the population are also enrolled for longer than the period of compulsory education. In Portugal, the upper secondary level is part of compulsory education and includes general and vocational programmes.
The enrolment rate of all 17-years-old Portuguese in the general upper secondary programmes is 57%, and 35% in the vocational upper secondary, slightly above the OECD averages (55% and 31% respectively). In both programs, the enrolment rate decreases with age, following the trend observed in the OECD average.
The average age of graduation from general upper secondary programmes varies from 17 to 21 years across OECD countries and is 21 years in Portugal. Differences in the average age of graduation from vocational upper secondary education are much larger and vary from 16 to 34 years across the OECD. These differences largely depend on whether vocational upper secondary students usually enrol in these programmes towards the end of their compulsory education or in mid-career. In Portugal, the average age of graduation from vocational upper secondary education is 20 years, which is below the OECD average at 22 years (Figure 4). Portugal is one of the 12 OECD countries where all vocational upper secondary graduates have direct access to tertiary education.

1. 1. Average age is based on all graduates instead of first-time graduates.
Countries are ranked in descending order of the average age of first-time upper secondary graduates in general programmes.
Source : OECD//Eurostat/UIS (2022), Tables B3.1 and B3.2. See Source section for more information and Annex 3 for notes ( https://www.oecd.org/education/education-at-a-glance/EAG2022_X3-B.pdf ).
In almost all OECD countries, women make up the majority of those graduating from general upper secondary education. In Portugal, the share is 55% (OECD average 55%). In contrast, men are overrepresented among graduates of vocational upper secondary programmes in most OECD countries, as is the case in Portugal where they make up 54% of all vocational upper secondary graduates, slightly below the OECD average (55%).
In Portugal, 61% of 18-24 year-olds are still in full- or part-time education or training at either upper secondary or tertiary level (above the OECD average of 54%). A subset of these students (5% of 18-24 year-olds) combine their education or training with some form of employment in Portugal, compared to 17% on average across the OECD.
In the last few years, Portuguese authorities have been promoting the vocational education and training (VET) pathways as a measure to reduce drop-out rates, increase secondary completion rates and provide professional training to facilitate entry into the job market.
In Portugal, the tertiary education level is provided by a binary education system comprising polytechnics and universities.
In 2020, the total number of students enrolled in tertiary education in Portugal was 380 235. As is the case in all OECD countries, a majority of students enrolled at the tertiary level in Portugal are bachelor’s students (57%). However, the next commonest enrolment level varies from country to country. In Portugal, master's students make up the second largest group of tertiary students at 33%. This is also the case in 25 other OECD countries, while in the remaining 14 countries with available data, short-cycle tertiary students form the second largest group. In Portugal, only 5% of students are enrolled in a short-cycle tertiary programme.
At 24%, business, administration and law was the most popular field of study among new entrants into tertiary education in Portugal, which is the case in most OECD countries. Despite the growing need for digital skills and the good employment prospects of students with degrees in information and communication technologies (ICT), only a small fraction of entrants into tertiary education choose this field. In Portugal, 96% of 25-64 year-olds with a tertiary ICT qualification are employed, but ICT students make up only 3% of new entrants into tertiary education. This is below the OECD average of 6%. Education is also a field of study with the lowest demand. Only 4% of the new tertiary entrants in Portugal sought to follow a career in education. This fact, along with the ageing teaching workforce, raises concerns about a shortage of teachers in the near future in Portugal.
Over the decades, independent private institutions have been established to meet the increased demand for tertiary education. On average across the OECD, 17% of students are enrolled in independent private institutions, but this figure masks large differences between countries. In Portugal, 19% of tertiary students are enrolled in such institutions. Independent private institutions charge higher annual tuition fees on average than public institutions for master’s programmes in all OECD countries and other participants with available data, except in Chile and Lithuania.
Enabling students to enrol on a part-time basis is an important way to facilitate access to tertiary education. Many part-time students would not be able to study full time, for example because they have child-care responsibilities or have to work to fund their studies. The share of part-time students at the tertiary level in Portugal is 5%, below the OECD average (22%). It remained at a similar level (with a difference of less than 1 percentage point between 2013 and 2020)
Despite the labour market advantages of a tertiary degree, many tertiary students do not graduate on time or do not graduate at all. In Portugal, 38% of bachelor’s students graduate within the theoretical programme duration. Across the OECD, the completion rate within the theoretical programme duration ranges from 12% to 69%. Completion rates three years after the theoretical programme duration are significantly higher in most countries and the differences between OECD countries are somewhat narrower. In Portugal, 72% of bachelor’s students have graduated within three years after the end of the theoretical programme duration, compared to 68% on average across the OECD.
In all OECD countries, tertiary completion rates are higher for women than for men. In Portugal, 79% of women graduated within three years after the end of the theoretical programme duration at the bachelor’s level, compared to 63% of men. On average across the OECD, there is little systematic difference between the completion rates of public and private institutions, but the figures differ from country to country. In Portugal, 73% of bachelor's students graduate from public institutions within three years after the end of the theoretical programme duration, while the share is 69% for private institutions.
Among 25-64 year-olds in Portugal, master's degrees are the most common tertiary attainment at 21% of the population followed by bachelor's degrees at 9% and short-cycle tertiary qualifications with less than 1%. This is different from the OECD average, where bachelor’s degrees are most common (19%), followed by master’s degrees (14%) and short cycle tertiary qualifications (7%). As in all OECD countries and other participants, only a small fraction of the population holds a doctoral degree: the share is 1% in Portugal.
National averages provide only an incomplete picture of the situation in any given country. In most OECD countries, there are large differences in educational attainment across subnational regions. This is also the case in Portugal. In 2021, the difference between the region with the highest share of 25-64 year-olds with tertiary attainment (Metropolitan area of Lisbon, at 41%) and that with the lowest share (Autonomous Region of the Azores, at 17%) was 24 percentage points. These subnational variations do not only reflect differences in education opportunities. To a large degree, they are due to economic conditions and internal migration patterns.
In most OECD countries including in Portugal, tertiary-educated adults have higher rates of participation in non-formal education and training than those with a lower level of educational attainment. In 2021, 21% of 25-64 year-olds with tertiary attainment in Portugal had participated in non-formal education and training in the four weeks prior to being surveyed, compared to 3% of their peers with below upper secondary attainment.
However, higher education in Portugal is largely oriented to the needs of the traditional age groups of students. In 2020, the average age of new entrants into long first degree, bachelor’s and master’s programmes was 19, 21 and 24 respectively (against OECD averages of 21, 22 and 27, respectively). The higher education institutions offer only moderately diversified and relatively inflexible study opportunities, which is a commonly mentioned barrier limiting the role of these tertiary institutions in upskilling and reskilling (OECD, 2022[4]) . Also, the population of Portugal is ageing at a faster pace than populations in most OECD countries which is likely to reduce demand for higher education among traditional student populations. This places additional pressure for higher education institutions to adjust their provision to encourage adult populations to engage in upskilling and reskilling in higher education (OECD, forthcoming[5])
Over time, the introduction of new admission routes to higher education has helped to widen and diversify access to higher education. Procedures for applicants aged over 23, initially introduced in 2006, were simplified in 2014, while more recent policies have aimed to increase participation in higher education among those graduating from the vocational tracks of upper secondary education Additionally, higher education institutions, namely the polytechnics, have adjusted their offerings to this new student population (OECD, forthcoming[5]) .
The COVID-19 pandemic disrupted traditional schooling in 2020 and the first half of 2021, leading to school closures across all OECD countries. While most shut down their premises entirely in the wake of the pandemic in 2020, by 2021 the situation had improved and returned to normal in most countries in 2022. In Portugal, primary and secondary schools were entirely closed for 47-62 days during the school year 2019/20, for 25-45 days in 2020/21 and stayed open in 2021/22 (Figure 5). There were no partial closures in 2019/20, 2020/21 and 2021/22.
Teacher absences also affected the regular operation of schools during the pandemic, whether due to COVID-19 infections or because of precautionary quarantine. However, only approximately half of countries collected information on teacher absenteeism. Portugal collected such data. In contrast to many other countries, teacher absenteeism increased slightly (by between 1% and 5%) between 2019/20 and 2021/22.
National examinations have also been affected by the pandemic. At general upper secondary level, 18 OECD countries postponed their national examinations during the school year 2019/20, while 10 countries even cancelled them entirely. In 2020/21, national examinations were postponed in 9 countries and cancelled in 6 countries. Portugal rescheduled its national examinations in 2019/20 and in 2020/21.
Most countries conducted assessments of the impact of school closures on learning outcomes at various levels of education and along several dimensions. Portugal has conducted studies to evaluate the effects of the pandemic and its impact on primary, lower secondary, upper secondary general and vocational education. The assessments covered mathematics, reading and science. Like many other countries, Portugal also evaluated dimensions such as the effectiveness of distance-learning strategies during school closures, non-cognitive skills the relations between parents and students during lockdowns as well as the mental health and well-being of students and teachers.
In school year 2022, national programmes to support students affected by the pandemic were implemented in Portugal at pre-primary, primary, lower secondary, upper secondary general and vocational and tertiary level. At primary to upper secondary education, measures to address the effects of the COVID-19 pandemic included, community mobilisation campaigns to bring students back to school, early warning systems to identify students at risk of dropping out, referral systems for students in need of specialised services, additional school nutrition services, psychosocial and mental health support to students, automatic re-enrolment of students in school, tutoring programmes or financial support for tutoring and additional water, sanitation and hygiene services. The government has already assessed the effectiveness of these programmes.
The increased digitalisation of education has been a major consequence of the COVID-19 pandemic in many OECD countries. At lower secondary level, Portugal has responded to the pandemic with an enhanced provision of digitalised assessments/exams, digital tools at school, distance learning, hybrid learning, in-service digital training to teachers and digital training to students.
The challenges related to the COVID-19 pandemic have created additional costs for education systems. Preliminary budget estimates for 2021 suggest that, compared to 2020, the education budget at pre-primary to upper secondary level in Portugal increased slightly (by between 1% and 5%, in nominal terms), while it increased strongly (by more than 5%) at the tertiary level.
The COVID-19 pandemic had a significant impact on adult learning in most OECD countries. In 2020, the share of adults who participated in a formal or non-formal education and training activity in the four weeks prior to being surveyed decreased by 2 percentage points on average across OECD countries compared with 2019. However, in 2021, participation in non-formal education and training returned to pre-pandemic levels in most countries. In Portugal, a similar pattern emerged. From 2019 to 2020, the share of adults participating in a formal or non-formal education and training activity remained unchanged. From 2020 to 2021, it increased by 3 percentage points and has thus increased above pre-pandemic levels.
Young adults who are not in employment, education or training (NEET) for prolonged periods are at risk of adverse economic and social outcomes in both the short and the long term. After increasing during the COVID-19 pandemic in 2020, the share of 18-24 year-olds who are NEET in Portugal declined in 2021. The share of NEET among young adults was 14% in 2021, above pre-COVID levels.

Note : The data underlying this report were produced through the Survey on Joint National Responses to COVID 19, a collaborative effort conducted by the United Nations Educational, Scientific and Cultural Organization (UNESCO), the United Nations Children’s Fund (UNICEF), the World Bank (WB), and the Organisation for Economic Co-operation and Development (OECD). Data for other levels of education are available at https://www.oecd.org/education/Results-4th-wave-COVID-Survey-OECD-database.xlsx
1. Data for 2021 and 2022 are missing.
Countries and other participants are ranked in descending order of the total number of days lower secondary schools were fully closed during the school years 2019/20 (2020), 2020/21 (2021) and 2021/22 (2022).
Source : OECD/UIS/UNESCO/UNICEF/WB (2022).
[3] Liebowitz, D. et al. (2018), OECD Reviews of School Resources: Portugal 2018 , OECD Publishing, Paris, https://doi.org/10.1787/9789264308411-en .
[7] OECD (2022), Eduction at a Glance 2022: OECD Indicators , OECD Publishing, Paris, https://doi.org/10.1787/3197152b-en .
[4] OECD (2022), Enhancing labour market relevance and outcomes of higher education: Country note Portugal , OECD Publishing, Paris, https://doi.org/10.1787/bcf4a8e3-en .
[8] OECD (2022), Pathways to Professions: Understanding Higher Vocational and Professional Tertiary Education Systems , OECD Reviews of Vocational Education and Training, OECD Publishing, Paris, https://doi.org/10.1787/a81152f4-en .
[6] OECD (2022), “Regional education” , OECD Regional Statistics (database) , https://doi.org/10.1787/213e806c-en .
[1] OECD (2022), Review of Inclusive Education in Portugal , OECD Publishing, Paris, https://doi.org/10.1787/a9c95902-en .
[2] OECD (2021), Education at a Glance 2021: OECD Indicators , OECD Publishing, Paris, https://doi.org/10.1787/b35a14e5-en .
[5] OECD (forthcoming), Resourcing Higher Education in Portugal , OECD Publishing, Paris.
For more information on Education at a Glance 2022 and to access the full set of Indicators, see: https://doi.org/10.1787/3197152b-en
For more information on the methodology used during the data collection for each indicator, the references to the sources and the specific notes for each country, see Annex 3 ( https://www.oecd.org/education/education-at-a-glance/EAG2022_Annex3.pdf ).
For general information on the methodology, please refer to the OECD Handbook for Internationally Comparative Education Statistics: Concepts, Standards, Definitions and Classifications ( https://doi.org/10.1787/9789264304444-en ).
Updated data can be found on line at https://doi.org/10.1787/eag-data-en and by following the StatLinks 2 under the tables and charts in the publication.
Data on subnational regions for selected indicators are available in the OECD Regional Statistics (database) (OECD, 2022). When interpreting the results on subnational entities, readers should take into account that the population size of subnational entities can vary widely within countries. For example, regional variation in enrolment may be influenced by students attending school in a different region from their area of residence, particularly at higher levels of education. Also, regional disparities tend to be higher when more subnational entities are used in the analysis.
Explore, compare and visualise more data and analysis using the Education GPS:
https://gpseducation.oecd.org/
The data on educational responses during COVID-19 were collected and processed by the OECD based on the Joint Survey on National Responses to COVID-19 School Closures, a collaborative effort conducted by the United Nations Educational, Scientific and Cultural Organization (UNESCO); the UNESCO Institute for Statistics (UIS); the United Nations Children's Fund (UNICEF); the World Bank; and the OECD.
Questions can be directed to:
Directorate for Education and Skills
[email protected]
This document, as well as any data and map included herein, are without prejudice to the status of or sovereignty over any territory, to the delimitation of international frontiers and boundaries and to the name of any territory, city or area. Extracts from publications may be subject to additional disclaimers, which are set out in the complete version of the publication, available at the link provided.
https://doi.org/10.1787/3197152b-en
© OECD 2022
The use of this work, whether digital or print, is governed by the Terms and Conditions to be found at https://www.oecd.org/termsandconditions .
Social Media Should Come With a Warning, Says U.S. Surgeon General

- Share article
The U.S. surgeon general is calling for a warning label on social media alerting users that “social media is associated with significant mental health harms in adolescents.”
In a New York Times opinion piece, Surgeon General Dr. Vivek Murthy said there is enough evidence mounting that shows a connection between social media and adolescents’ deteriorating mental health that a surgeon general’s warning label—similar to what appears on cigarette packages—is warranted.
“One of the most important lessons I learned in medical school was that in an emergency, you don’t have the luxury to wait for perfect information,” Murthy wrote in the New York Times. “You assess the available facts, you use your best judgment, and you act quickly.”
Murthy had already signaled his concerns with the potentially harmful effects of social media on kids and teens when he issued an advisory last year warning about the risks social media poses to youth mental health.
A surgeon general’s warning label, though, would have to be approved by Congress before it could be applied to social media platforms.
Many educators say that social media—and students’ near-constant access to it via cellphones—has become a major challenge for schools. More than 200 school districts have sued the major social media companies , claiming that they have created highly addictive products that are damaging students’ mental health, leaving schools to deal with the fallout.
Four years ago, nearly 100 school districts used a similar argument in lawsuits against e-cigarette manufacturers, claiming the problems the hard-to-detect tobacco products caused amounted to a public nuisance. A class action lawsuit against JUUL was settled in 2022.
State and federal efforts to curb social media use among youth
Some state and federal lawmakers are also trying to restrict young kids’ access to social media , with the aim of improving their mental health, adding to the mounting pressure on social media companies.
For their part, social media companies say they are taking steps to protect their youngest users’ safety and well-being on their platforms through parental controls, age-verification features, and time-management tools.
There is evidence that warning labels like those on tobacco products can change people’s behavior, Murthy wrote in the New York Times. And he pointed to a recent survey of Latino parents showing that three-quarters said they would limit or monitor their children’s social media use if they saw a surgeon general’s warning on the platforms used by their families.

Murthy did concede in the opinion essay that a warning label would not completely negate the harms and challenges presented by social media, a sentiment echoed by educators.
Michael Lubelfeld is the superintendent of North Shore School District 112 in a suburb of Chicago, and his district is among those suing the social media companies over the youth mental health crisis. He’s unsure the warning label will make a difference, although he said he applauds the surgeon general’s effort.
“Because to some degree the cat’s already out of the bag, there’s tremendous usage among children who are having a very difficult time dealing with it,” he said. “I don’t know that parents are always aware of what their kids are doing on their smartphones.”
A warning label would help raise awareness, said Beth Houf, the principal of Capital City High School in Jefferson, Mo.
“It’s a step in helping the situation, but there is more that needs to be done,” she said.
Houf, who has also led elementary and middle schools in her career, said she’s seen the negative effects social media has on students’ mental health worsen over the 17 years she’s been a principal. Despite the cyberbullying and the unhealthy comparisons kids make of each other on social media, they can’t seem to turn away from these platforms, she said.
“When you hear something or have a vibration in your pocket, the fear that you’re missing out on something you feel like you have to engage with—that makes it harder to pay attention in the classroom,” she said.
Anjali Verma, a rising senior at Pennsylvania Leadership Charter School, a statewide charter school, also thinks a warning label alone won’t make a big difference.
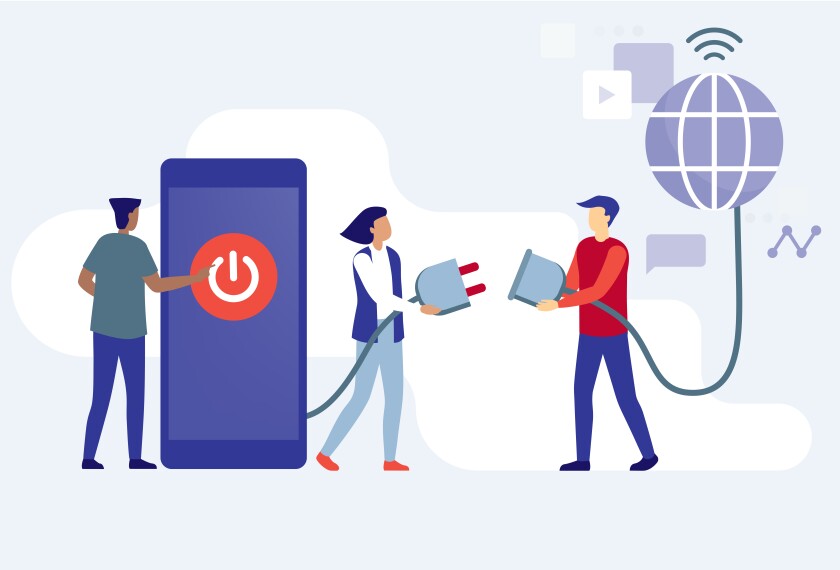
“I think it’s a good place to start, but it’s a small step,” she said. “But I think it’s important still to have that moment of reflection.”
Anjali uses a mindfulness app on her phone that prompts her to practice a breathing exercise before opening a social media app. She said that pause makes her stop and think about why she’s getting on the app and what she’ll get out of it, which she has found helpful. A warning label may have the same effect, she said.
Seeing the good and bad of social media
Anjali pointed out that there is an assumption from adults that social media is nothing but bad for adolescents. She, and many of her peers, see the impact of social media on their lives as much more nuanced—both good and bad.
“Social media is more than TikTok dances,” she said. “I think in terms of advocacy and creating social change, social media has been instrumental.”
Murthy said social media platforms should also not be allowed to collect sensitive data from children nor use manipulative features like algorithms, push notifications, and the infinite scroll, which all “contribute to excessive use.” Murthy also renewed his calls for social media companies to share their data on the health effects of their products with independent scientists and the public.
But schools and parents also have roles to play, Murthy said.
“Schools should ensure that classroom learning and social time are phone-free experiences,” he said. “Parents, too, should create phone-free zones around bedtime, meals, and social gatherings to safeguard their kids’ sleep and real-life connections—both of which have direct effects on mental health.”
Sign Up for EdWeek Update
Edweek top school jobs.

Sign Up & Sign In

China has become a scientific superpower
From plant biology to superconductor physics the country is at the cutting edge.

I n the atrium of a research building at the Chinese Academy of Sciences ( CAS ) in Beijing is a wall of patents. Around five metres wide and two storeys high, the wall displays 192 certificates, positioned in neat rows and tastefully lit from behind. At ground level, behind a velvet rope, an array of glass jars contain the innovations that the patents protect: seeds.
Your browser does not support the <audio> element.
CAS —the world’s largest research organisation—and institutions around China produce a huge amount of research into the biology of food crops. In the past few years Chinese scientists have discovered a gene that, when removed, boosts the length and weight of wheat grains, another that improves the ability of crops like sorghum and millet to grow in salty soils and one that can increase the yield of maize by around 10%. In autumn last year, farmers in Guizhou completed the second harvest of genetically modified giant rice that was developed by scientists at CAS .
The Chinese Communist Party ( CCP ) has made agricultural research—which it sees as key to ensuring the country’s food security —a priority for scientists. Over the past decade the quality and the quantity of crop research that China produces has grown immensely, and now the country is widely regarded as a leader in the field. According to an editor of a prestigious European plant-sciences journal, there are some months when half of the submissions can come from China.
A journey of a thousand miles
The rise of plant-science research is not unique in China. In 2019 The Economist surveyed the research landscape in the country and asked whether China could one day become a scientific superpower. Today, that question has been unequivocally answered: “yes”. Chinese scientists recently gained the edge in two closely watched measures of high-quality science, and the country’s growth in top-notch research shows no sign of slowing. The old science world order, dominated by America, Europe and Japan, is coming to an end.

One way to measure the quality of a country’s scientific research is to tally the number of high-impact papers produced each year—that is, publications that are cited most often by other scientists in their own, later work. In 2003 America produced 20 times more of these high-impact papers than China, according to data from Clarivate, a science analytics company (see chart 1). By 2013 America produced about four times the number of top papers and, in the most recent release of data, which examines papers from 2022, China had surpassed both America and the entire European Union ( EU ).
Metrics based on citations can be gamed, of course. Scientists can, and do, find ways to boost the number of times their paper is mentioned in other studies, and a recent working paper, by Qui Shumin, Claudia Steinwender and Pierre Azoulay, three economists, argues that Chinese researchers cite their compatriots far more than Western researchers do theirs. But China now leads the world on other benchmarks that are less prone to being gamed. It tops the Nature Index, created by the publisher of the same name, which counts the contributions to articles that appear in a set of prestigious journals. To be selected for publication, papers must be approved by a panel of peer reviewers who assess the study’s quality, novelty and potential for impact. When the index was first launched, in 2014, China came second, but its contribution to eligible papers was less than a third of America’s. By 2023 China had reached the top spot.
According to the Leiden Ranking of the volume of scientific research output, there are now six Chinese universities or institutions in the world top ten, and seven according to the Nature Index. They may not be household names in the West yet, but get used to hearing about Shanghai Jiao Tong, Zhejiang and Peking (Beida) Universities in the same breath as Cambridge, Harvard and ETH Zurich. “Tsinghua is now the number one science and technology university in the world,” says Simon Marginson, a professor of higher education at Oxford University. “That’s amazing. They’ve done that in a generation.”

Today China leads the world in the physical sciences, chemistry and Earth and environmental sciences, according to both the Nature Index and citation measures (see chart 2). But America and Europe still have substantial leads in both general biology and medical sciences. “Engineering is the ultimate Chinese discipline in the modern period,” says Professor Marginson, “I think that’s partly about military technology and partly because that’s what you need to develop a nation.”
Applied research is a Chinese strength. The country dominates publications on perovskite solar panels, for example, which offer the possibility of being far more efficient than conventional silicon cells at converting sunlight into electricity. Chinese chemists have developed a new way to extract hydrogen from seawater using a specialised membrane to separate out pure water, which can then be split by electrolysis. In May 2023 it was announced that the scientists, in collaboration with a state-owned Chinese energy company, had developed a pilot floating hydrogen farm off the country’s south-eastern coast.
China also now produces more patents than any other country, although many are for incremental tweaks to designs, as opposed to truly original inventions. New developments tend to spread and be adopted more slowly in China than in the West. But its strong industrial base, combined with cheap energy, means that it can quickly spin up large-scale production of physical innovations like materials. “That’s where China really has an advantage on Western countries,” says Jonathan Bean, CEO of Materials Nexus, a British firm that uses AI to discover new materials.
The country is also signalling its scientific prowess in more conspicuous ways. Earlier this month, China’s Chang’e-6 robotic spacecraft touched down in a gigantic crater on the far side of the Moon, scooped up some samples of rock, planted a Chinese flag and set off back towards Earth. If it successfully returns to Earth at the end of the month, it will be the first mission to bring back samples from this hard-to-reach side of the Moon.
First, sharpen your tools
The reshaping of Chinese science has been achieved by focusing on three areas: money, equipment and people. In real terms, China’s spending on research and development ( R & D ) has grown 16-fold since 2000. According to the most recent data from the OECD , from 2021, China still lagged behind America on overall R & D spending, dishing out $668bn, compared with $806bn for America at purchasing-power parity. But in terms of spending by universities and government institutions only, China has nudged ahead. In these places America still spends around 50% more on basic research, accounting for costs, but China is splashing the cash on applied research and experimental development (see chart 3).

Money is meticulously directed into strategic areas. In 2006 the CCP published its vision for how science should develop over the next 15 years. Blueprints for science have since been included in the CCP ’s five-year development plans. The current plan, published in 2021, aims to boost research in quantum technologies, AI , semiconductors, neuroscience, genetics and biotechnology, regenerative medicine, and exploration of “frontier areas” like deep space, deep oceans and Earth’s poles.
Creating world-class universities and government institutions has also been a part of China’s scientific development plan. Initiatives like “Project 211”, the “985 programme” and the “China Nine League” gave money to selected labs to develop their research capabilities. Universities paid staff bonuses—estimated at an average of $44,000 each, and up to a whopping $165,000—if they published in high-impact international journals.
Building the workforce has been a priority. Between 2000 and 2019, more than 6m Chinese students left the country to study abroad, according to China’s education ministry. In recent years they have flooded back, bringing their newly acquired skills and knowledge with them. Data from the OECD suggest that, since the late 2000s, more scientists have been returning to the country than leaving. China now employs more researchers than both America and the entire EU .
Many of China’s returning scientists, often referred to as “sea turtles” (a play on the Chinese homonym haigui , meaning “to return from abroad”) have been drawn home by incentives. One such programme launched in 2010, the “Youth Thousand Talents”, offered researchers under 40 one-off bonuses of up to 500,000 yuan (equivalent to roughly $150,000 at purchasing-power parity) and grants of up to 3m yuan to get labs up and running back home. And it worked. A study published in Science last year found that the scheme brought back high-calibre young researchers—they were, on average, in the most productive 15% of their peers (although the real superstar class tended to turn down offers). Within a few years, thanks to access to more resources and academic manpower, these returnees were lead scientists on 2.5 times more papers than equivalent researchers who had remained in America.
As well as pull, there has been a degree of push. Chinese scientists working abroad have been subject to increased suspicion in recent years. In 2018 America launched the China Initiative, a largely unsuccessful attempt to root out Chinese spies from industry and academia. There have also been reports of students being deported because of their association with China’s “military-civilian fusion strategy”. A recent survey of current and former Chinese students studying in America found that the share who had experienced racial abuse or discrimination was rising.
The availability of scientists in China means that, for example in quantum computing, some of the country’s academic labs are more like commercial labs in the West, in terms of scale. “They have research teams of 20, 30, even 40 people working on the same experiments, and they make really good progress,” says Christian Andersen, a quantum researcher at Delft University. In 2023 researchers working in China broke the record for the number of quantum bits, or qubits, entangled inside a quantum computer.
China has also splurged on scientific kit. In 2019, when The Economist last surveyed the state of the country’s scientific research, it already had an enviable inventory of flashy hardware including supercomputers, the world’s largest filled-aperture radio telescope and an underground dark-matter detector. The list has only grown since then. The country is now home to the world’s most sensitive ultra-high-energy cosmic-ray detector (which has recently been used to test aspects of Albert Einstein’s special theory of relativity), the world’s strongest steady-state magnetic field (which can probe the properties of materials) and soon will have one of the world’s most sensitive neutrino detectors (which will be used to work out which type of these fundamental subatomic particles has the highest mass). Europe and America have plenty of cool kit of their own, but China is rapidly adding hardware.
Individual labs in China’s top institutions are also well equipped. Niko McCarty, a journalist and former researcher at the Massachusetts Institute of Technology who was recently given a tour of synthetic biology labs in China, was struck by how, in academic institutions, “the machines are just more impressive and more expansive” than in America. At the Advanced Biofoundry at the Shenzhen Institute of Advanced Technology, which the country hopes will be the centre of China’s answer to Silicon Valley, Mr McCarty described an “amazing building with four floors of robots”. As Chinese universities fill with state-of-the-art equipment and elite researchers, and salaries become increasingly competitive, Western institutions look less appealing to young and ambitious Chinese scientists. “Students in China don’t think about America as some “scientific Mecca” in the same way their advisers might have done,” said Mr McCarty.

Take AI , for example. In 2019 just 34% of Chinese students working in the field stayed in the country for graduate school or work. By 2022 that number was 58%, according to data from the AI talent tracker by MacroPolo, an American think-tank (in America the figure for 2022 was around 98%). China now contributes to around 40% of the world’s research papers on AI , compared with around 10% for America and 15% for the EU and Britain combined. One of the most highly cited research papers of all time, demonstrating how deep neural networks could be trained on image recognition, was written by AI researchers working in China, albeit for Microsoft, an American company. “China’s AI research is world-class,” said Zachary Arnold, an AI analyst at the Georgetown Centre for Security and Emerging Technology. “In areas like computer vision and robotics, they have a significant lead in research publications.”
Growth in the quality and quantity of Chinese science looks unlikely to stop anytime soon. Spending on science and technology research is still increasing—the government has announced a 10% increase in funding in 2024. And the country is training an enormous number of young scientists. In 2020 Chinese universities awarded 1.4m engineering degrees, seven times more than America did. China has now educated, at undergraduate level, 2.5 times more of the top-tier AI researchers than America has. And by 2025, Chinese universities are expected to produce nearly twice as many P h D graduates in science and technology as America.
To see further, ascend another floor
Although China is producing more top-tier work, it still produces a vast amount of lower-quality science too. On average, papers from China tend to have lower impact, as measured by citations, than those from America, Britain or the EU . And while the chosen few universities have advanced, mid-level universities have been left behind. China’s second-tier institutions still produce work that is of relatively poor quality compared with their equivalents in Europe or America. “While China has fantastic quality at the top level, it’s on a weak base,” explains Caroline Wagner, professor of science policy at Ohio State University.
When it comes to basic, curiosity-driven research (rather than applied) China is still playing catch-up—the country publishes far fewer papers than America in the two most prestigious science journals, Nature and Science . This may partly explain why China seems to punch below its weight in the discovery of completely new technologies. Basic research is particularly scant within Chinese companies, creating a gap between the scientists making discoveries and the industries that could end up using them. “For more original innovation, that might be a minus,” says Xu Xixiang, chief scientist at LONG i Green Energy Technology, a Chinese solar company.
Incentives to publish papers have created a market for fake scientific publications. A study published earlier this year in the journal Research Ethics , featured anonymous interviews from Chinese academics, one of whom said he had “no choice but to commit [research] misconduct”, to keep up with pressures to publish and retain his job. “Citation cartels” have emerged, where groups of researchers band together to write low-quality papers that cite each other’s work in an effort to drive up their metrics. In 2020 China’s science agencies announced that such cash-for-publication schemes should end and, in 2021, the country announced a nationwide review of research misconduct. That has led to improvements—the rate at which Chinese researchers cite themselves, for example, is falling, according to research published in 2023. And China’s middle-ranking universities are slowly catching up with their Western equivalents, too.
The areas where America and Europe still hold the lead are, therefore, unlikely to be safe for long. Biological and health sciences rely more heavily on deep subject-specific knowledge and have historically been harder for China to “bring back and accelerate”, says Tim Dafforn, a professor of biotechnology at University of Birmingham and former adviser to Britain’s department for business. But China’s profile is growing in these fields. Although America currently produces roughly four times more highly influential papers in clinical medicine, in many areas China is producing the most papers that cite this core research, a sign of developing interest that presages future expansion. “On the biology side, China is growing remarkably quickly,” says Jonathan Adams, chief scientist at the Institute for Scientific Information at Clarivate. “Its ability to switch focus into a new area is quite remarkable.”
The rise of Chinese science is a double-edged sword for Western governments. China’s science system is inextricably linked with its state and armed forces—many Chinese universities have labs explicitly working on defence and several have been accused of engaging in espionage or cyber-attacks. China has also been accused of intellectual-property theft and increasingly stringent regulations have made it more difficult for international collaborators to take data out of the country; notoriously, in 2019, the country cut off access to American-funded work on coronaviruses at the Wuhan Institute of Virology. There are also cases of Chinese researchers failing to adhere to the ethical standards expected by Western scientists.
Despite the concerns, Chinese collaborations are common for Western researchers. Roughly a third of papers on telecommunications by American authors involve Chinese collaborators. In imaging science, remote sensing, applied chemistry and geological engineering, the figures are between 25% and 30%. In Europe the numbers are lower, around 10%, but still significant. These partnerships are beneficial for both countries. China tends to collaborate more in areas where it is already strong like materials and physics. A preprint study, released last year, found that for AI research, having a co-author from America or China was equally beneficial to authors from the other country, conferring on average 75% more citations.
Several notable successes have come from working together, too. During the covid-19 pandemic a joint venture between Oxford University’s Engineering Department and the Oxford Suzhou Centre for Advanced Research developed a rapid covid test that was used across British airports. In 2015 researchers at University of Cardiff and South China Agricultural University identified a gene that made bacteria resistant to the antibiotic colistin. Following this, China, the biggest consumer of the drug, banned its use in animal feed, and levels of colistin resistance in both animals and humans declined.
In America and Europe, political pressure is limiting collaborations with China. In March, America’s Science and Technology Agreement with China, which states that scientists from both countries can collaborate on topics of mutual benefit, was quietly renewed for a further six months. Although Beijing appears keen to renew the 45-year-old agreement, many Republicans fear that collaboration with China is helping the country achieve its national-security goals. In Europe, with the exception of environmental and climate projects, Chinese universities have been effectively barred from accessing funding through the Horizon programme, a huge European research initiative.
There are also concerns among scientists that China is turning inwards. The country has explicit aims to become self-reliant in many areas of science and technology and also shift away from international publications as a way of measuring research output. Many researchers cannot talk to the press—finding sources in China for this story was challenging. One Chinese plant scientist, who asked to remain anonymous, said that she had to seek permission a year in advance to attend overseas conferences. “It’s contradictory—on the one hand, they set restrictions so that scientists don’t have freedoms like being able to go abroad to communicate with their colleagues. But on the other hand, they don’t want China to fall behind.”
Live until old, learn until old
The overwhelming opinion of scientists in China and the West is that collaboration must continue or, better, increase. And there is room to do more. Though China’s science output has grown dramatically, the share that is conducted with international collaborators has remained stable at around 20%—Western scientists tend to have far more international collaborations. Western researchers could pay more attention to the newest science from China, too. Data from a study published last year in Nature Human Behaviour showed that, for work of equivalent quality, Chinese scientists cite Western papers far more than vice versa. Western scientists rarely visit, work or study in China, depriving them of opportunities to learn from Chinese colleagues in the way Chinese scientists have done so well in the West.
Closing the door to Chinese students and researchers wishing to come to Western labs would also be disastrous for Western science. Chinese researchers form the backbone of many departments in top American and European universities. In 2022 more of the top-tier AI researchers working in America hailed from China than from America. The West’s model of science currently depends on a huge number of students, often from overseas, to carry out most day-to-day research.
There is little to suggest that the Chinese scientific behemoth will not continue growing stronger. China’s ailing economy may eventually force the CCP to slow spending on research, and if the country were to become completely cut off from the Western science community its research would suffer. But neither of these looks imminent. In 2019 we also asked if research could flourish in an authoritarian system. Perhaps over time its limits will become clear. But for now, and at least for the hard sciences, the answer is that it can thrive. “I think it’d be very unwise to call limits on the Chinese miracle,” says Prof Marginson. “Because it has had no limits up until now.” ■
Curious about the world? To enjoy our mind-expanding science coverage, sign up to Simply Science , our weekly subscriber-only newsletter.
This article appeared in the Science & technology section of the print edition under the headline “Soaring dragons”

From the June 15th 2024 edition
Discover stories from this section and more in the list of contents
More from Science & technology

The dominant model of the universe is creaking
Dark energy could break it apart

Only 5% of therapies tested on animals are approved for human use
More rigorous experiments could improve those odds

The secret to taking better penalties
Practise with an augmented-reality headset
Like people, elephants call each other by name
And anthropoexceptionalism takes another tumble
Elon Musk’s Starship makes a test flight without exploding
Crucially, the upper stage of the giant rocket survived atmospheric re-entry
Zany ideas to slow polar melting are gathering momentum
Giant curtains to keep warm water away from glaciers strike some as too risky
- Share full article
Advertisement
Supported by
California Today
California May Ban Legacy Admissions at Universities
The State Assembly passed a bill banning colleges from considering family ties to donors or alumni in admissions decisions.

By Soumya Karlamangla

California could become the fourth state to ban legacy admissions preferences at universities under a bill making its way through the State Legislature.
Many selective colleges have historically given to the children or grandchildren of alumni — who are much more likely to be white and wealthy than other applicants — an advantage in the admissions process. But the practice, never particularly popular with the public, has come under scrutiny since the U.S. Supreme Court ruled last year against affirmative action policies at colleges and universities.
After the court’s decision, some schools — including Occidental College, Carnegie Mellon and Wesleyan University — decided to stop giving preference to legacy applicants.
Now, California lawmakers are considering AB 1780 , a bill that would prohibit universities in the state from giving preferential treatment to applicants because of their family ties to donors or alumni.
With affirmative action banned in higher education, “it makes complete sense to now ensure that we don’t look at someone’s wealth or lineage with the university to decide whether to admit them,” Phil Ting, a Bay Area Democrat who is the bill’s author, told me. The bill “doesn’t ban admitting donors’ or alumni children,” he added. “It just ensures that there’s no preferential treatment.”
Colorado and Virginia recently passed laws banning legacy admissions at public institutions of higher education. Maryland has done so at both public and private institutions. California’s public colleges and universities already give no preference to legacy candidates; the new bill would ban the practice at private institutions.
We are having trouble retrieving the article content.
Please enable JavaScript in your browser settings.
Thank you for your patience while we verify access. If you are in Reader mode please exit and log into your Times account, or subscribe for all of The Times.
Thank you for your patience while we verify access.
Already a subscriber? Log in .
Want all of The Times? Subscribe .
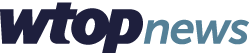
The Ten Commandments must be displayed in Louisiana classrooms under requirement signed into law
The Associated Press
June 19, 2024, 1:59 PM
- Share This:
- share on facebook
- share on threads
- share on linkedin
- share on email
BATON ROUGE, La. (AP) — Louisiana has become the first state to require that the Ten Commandments be displayed in every public school classroom under a bill signed into law by Republican Gov. Jeff Landry on Wednesday.
The GOP-drafted legislation mandates that a poster-sized display of the Ten Commandments in “large, easily readable font” be required in all public classrooms, from kindergarten to state-funded universities. Although the bill did not receive final approval from Landry, the time for gubernatorial action — to sign or veto the bill — has lapsed.
Opponents question the law’s constitutionality, warning that lawsuits are likely to follow. Proponents say the purpose of the measure is not solely religious, but that it has historical significance. In the law’s language, the Ten Commandments are described as “foundational documents of our state and national government.”
The displays, which will be paired with a four-paragraph “context statement” describing how the Ten Commandments “were a prominent part of American public education for almost three centuries,” must be in place in classrooms by the start of 2025.
The posters would be paid for through donations. State funds will not be used to implement the mandate, based on language in the legislation.
The law also “authorizes” — but does not require — the display of the Mayflower Compact, the Declaration of Independence and the Northwest Ordinance in K-12 public schools.
Similar bills requiring the Ten Commandments be displayed in classrooms have been proposed in other states including Texas , Oklahoma and Utah. However, with threats of legal battles over the constitutionality of such measures, no state besides Louisiana has had success in making the bills law.
Legal battles over the display of the Ten Commandments in classrooms are not new.
In 1980, the U.S. Supreme Court ruled that a similar Kentucky law was unconstitutional and violated the establishment clause of the U.S. Constitution, which says Congress can “make no law respecting an establishment of religion.” The high court found that the law had no secular purpose but rather served a plainly religious purpose.
Louisiana’s controversial law, in a state ensconced in the Bible Belt, comes during a new era of conservative leadership in the state under Landry, who replaced two-term Democratic Gov. John Bel Edwards in January.
The GOP also has a two-thirds supermajority in the Legislature, and Republicans hold every statewide elected position, paving the way for lawmakers to push through a conservative agenda during the legislative session that concluded earlier this month.
Copyright © 2024 The Associated Press. All rights reserved. This material may not be published, broadcast, written or redistributed.
Related News
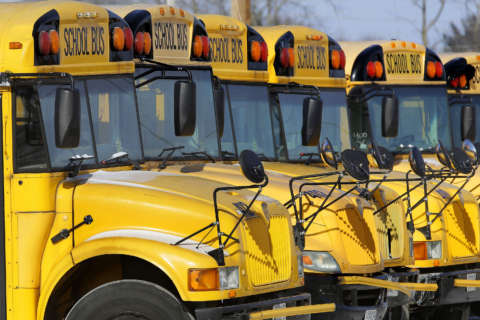
Louisiana becomes the first state to require that the Ten Commandments be displayed in public school classrooms
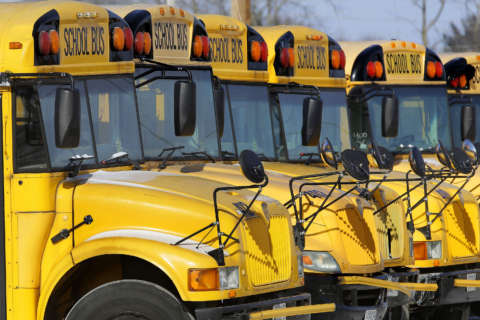
Virginia Senate fails to act on changes to military education benefits program; Youngkin stunned
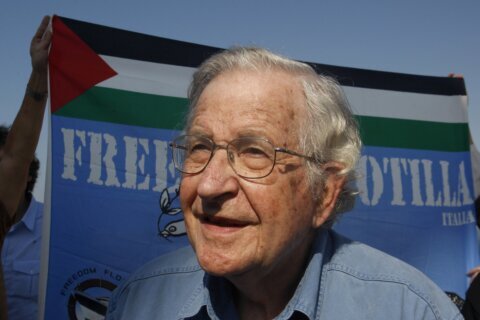
Noam Chomsky’s wife says reports of famed linguist’s death are false
Recommended.
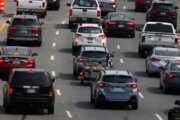
Truck crash on Route 50 leads to 14-mile, 9-hour backup leading to Bay Bridge
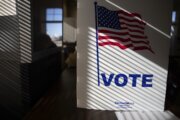
Virginia primary: Here are the Democratic and Republican winners in the closely watched races that could tip balance of power
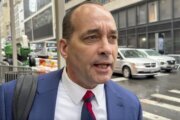
Cliffhanger: Virginia's Bob Good locked in tight contest against Trump-endorsed John McGuire
Related categories:.
StarTribune
Jay ronald ruckdaschel.
Ruckdaschel, Jay Ronald (April 23, 1933 - June 9, 2024) A loving father, tender and kind, what beautiful memories you left behind. --Anonymous Jay Ronald Ruckdaschel, age 91, died peacefully at home in Hopkins, MN on June 9, 2024, surrounded by his three loving daughters. Jay - beloved husband, father, grandfather, great grandfather, brother, uncle, cousin, and friend - was a kind, loving man with a strong faith in God. Always one to smile, he loved to sing, play the harmonica and piano, and spread joy to all around him. An avid Twins fan, we remember fondly his text-by-text replay keeping family and friends informed. Jay was born on April 23, 1933, in Sisseton, SD, the first of ten to Ralph Ruckdaschel and Violet (Johnston) Ruckdaschel. He grew up on a farm near Claire City, SD, and was active in basketball, football, and track in high school. In 1951, Jay attended Huron College while working to pay for his room, board, and tuition. During his freshmen year, he played on the basketball team which advanced to the NAIA national tournament in Kansas City. In his sophomore year, he met the love of his life, Margaret Alice Klicker (better known as "Tootie"). Their plans to marry were postponed when Jay was drafted into the Army and served during the Korean War from August 18, 1953 through May 17, 1955. After Jay's return from Korea, he and Tootie married on June 5, 1955. Together they had three daughters: Karla, Kaye, and Kim. Jay received his Bachelor's Degree from Huron College in 1957, a Master of Education Degree from South Dakota State University in 1960, and an Educational Specialist Degree from Mankato State University in 1977. Jay and Tootie, both dedicated educators, lived in several towns in South Dakota and Minnesota throughout the years. After graduating from Huron College in 1957, Jay's first job was as coach and teacher in Bancroft, SD. In 1960, Jay and Tootie moved to Volga, SD where Jay was guidance counselor and principal, and from 1964 through 1974 was superintendent. From 1974 to 1980, Jay was superintendent in Tyler, MN, and from 1980 to 1985 was superintendent in Winner, SD. Jay finished his career in Pierre, SD with the Associated School Board Association from 1985 to 1997, serving as Assistant Executive Director and Director of the SD Teacher Placement Center. Jay was honored to receive the South Dakota Bell Award for his outstanding service and dedication as an educator. After retirement, Jay and Tootie moved to Huron, SD in 1999 and then Hopkins, MN in 2008, remaining active in the community and their church. They volunteered for many heartfelt causes including the South Dakota Retired School Personnel (Jay was past president), AARP Drivers Safety Program, Rotary Club, Reading Council, Lions Club, and Special Olympics. Jay and Tootie also spent much of their retirement years traveling and visiting family, including multiple bus tours and trips overseas to Germany, Mexico, England, Portugal, and France. And Jay was a proud lifelong member of the VFW and American Legion. Ever devoted to his "sweetheart" Tootie during their 63 years of marriage, Jay became an avid texter with family and friends after Tootie's death on February 13, 2019. He continued Tootie's tradition of sending birthday and holiday cards as well as giving "flying 20s" to his grandchildren and great grandchildren. Jay appreciated the simple things: food was always good, people were good, and God was his rock. He also cherished friendships, from his childhood friends to those he made in recent years. He treasured time with his Chapel View friends with whom he did fitness walking, played bingo and Wi-Fi bowling, and shared memories. Jay is survived by his children Karla Hauk, Kaye (Todd) Eckstaine, and Kim (Kevin) Ruckdaschel-Haley; grandchildren Jeremy Hauk, Jesse Hauk, Kirsten Eckstaine, Jacob Eckstaine, Zachary Haley, Sophia Haley, and Harry Momoh; great grandchildren Maggie Hauk, Molly Hauk, Dominic Hernandez, Sauntee Hauk, Lainie Hauk and Sam Hauk; siblings Marlene Isensee, Luella (Louis) Bettermann, Donna Sherman, Earl Ruckdaschel, Betty (John) Maxe, Alvin (Susan) Ruckdaschel, and Alice (Dan) Conklin, and many nieces, nephews, cousins and friends. Preceding Jay in death are his parents; wife, Margaret; infant daughter, Lonna; beloved grandson Joshua Hauk; and sisters Barbara Bollig and Lyla Harris. Jay taught us how to live each day, enjoy life's simple pleasures, love each other, be humble, enjoy music, and be positive and kind. Until we meet again, Jay, we love you and you remain in our hearts. A celebration of Jay's life will be held Saturday, August 3, at Gethsemane Lutheran Church in Hopkins, MN, at 2 p.m., with a pre-service gathering starting at 1 p.m. In lieu of flowers, memorials can be made to Gethsemane Lutheran Church, the church Jay loved. All are welcome to wear Twins attire, if desired, in celebration of Jay's favorite team. Washburn-McReavy.com Strobeck Johnson 952-938-9020
Published on June 19, 2024
Star Tribune reviews all guest book entries to ensure appropriate content.
Our staff does not correct grammar or spelling. FAQ
2:00 PM, Saturday August 3rd, 2024
Pre-service gathering at church starting at 1 pm.
Gethsemane Lutheran Church
Dad had a strong faith and loved his church.
Preceded By
© 2024 StarTribune. All rights reserved.
Print Obituary
Do you want to include the guest book.

IMAGES
VIDEO
COMMENTS
Regardless of whether you choose a state school or a private one, the education system in Portugal is the same. It consists of four stages and comprises the following educational levels: Preschool education (Educação Pré-escolar): Ages 3 to 6. Primary education (Ensino Básico): Ages 6 to 15.
Education in Portugal is free and compulsory until the age of 18, when students usually complete their year 12. However, only one of those requirements is necessary. The education is regulated by the State through the Ministry of Education.There is a system of public education and also many private schools at all levels of education. The first Portuguese medieval universities, such as the ...
Overview of the education system (EAG 2023) In Portugal, 40% of 15-19 year-olds are enrolled in general upper secondary education and 24% in vocational upper secondary education. A further 10% are enrolled in lower secondary programmes and 18% in tertiary programmes. This compares to an OECD average of 37% enrolled in general upper secondary ...
Public primary schools in Portugal. Because public education is free in Portugal, most locals choose this option. Indeed, caregivers only need to cover a few costs such as books, school meals, and the occasional field trip. Overall, the quality of public education in Portugal is good.
Portugal. This country note provides an overview of the key characteristics of the education system in Portugal. It draws on data from Education at a Glance 2023. In line with the thematic focus of this year's Education at a Glance, it emphasises vocational education and training (VET), while also covering other parts of the education system.
Gender inequalities in education and outcomes. In Portugal, 5.6% of students in lower secondary and 7.2% in upper secondary initial education repeated a grade in 2019, compared to 1.9% and 3% respectively on average across OECD countries. Boys are more likely to repeat a grade at lower secondary initial education than girls.
institutions. On 12 March, Portugal announced the closure of all education institutions from 16 March. Portugal began reopening higher education from 4 May and upper secondary from 18 May; basic education would continue at a distance to the end of the academic year. In light of the work of the Education Policy Outlook in 2020 in the context of this
This new system began in 2006 and was fully in place in Portugal by the 2009/2010 school year. The education system in Portugal is overseen by the Ministry of Education (Direção-Geral da Educação - DGE), covering pre-school through secondary school. Private schools in Portugal require approval from the Ministry of Higher Education.
Sharing good practices & tools for solving the biggest challenges in education. SDG 4 Knowledge Hub. ... Search. Pexels. Story. Transforming Education in Portugal: Empowering a student movement and beefing up digital learning. An inspiring movement is sweeping through Portugal's schools, empowering students and making sure they are engaged in ...
The basic education school system in Portugal caters to children aged 6 to 15, over the course of three cycles: First cycle (primeiro ciclo or 1º ciclo): Grades 1 to 4. Second cycle (segundo ciclo or 2º ciclo): Grades 5 to 6. Third cycle (terceiro ciclo or 3º ciclo): Grades 7 to 9. Public school is free, and therefore the preferred option ...
In Portugal, the primary school day usually runs from 09:00 to 15:30. Attendance at primary school is compulsory for children aged between 6 and 15. Your child's time at primary school will be split into three cycles. The first cycle covers Grades 1 to 4, the second cycle covers Grades 5 to 6, and the third cycle covers Grades 7 to 9.
The Education System in Portugal. Portugal's education system is mixed, with both public and private institutions providing education from kindergarten through to higher education. Education Facts about Portugal. Portugal has a mixed public-private school system. School is mandatory from six to 18 years of age.
Despite high enrolment rates, tertiary attainment in Portugal suffers from low completion rates. In Portugal, around 41% of 19-20 year-olds - the age at which tertiary education begins in most OECD countries - are enrolled in tertiary education, above the OECD average of 37%. Completion of tertiary education, however, remains a challenge.
Data, policy advice and research on Portugal including economy, education, employment, environment, health, tax, trade, GDP, unemployment rate, inflation and PISA., This country policy profile on education in Portugal is part of the Education Policy Outlook series. Building on the first policy profile for Portugal (2014), it offers a concise analysis of where the education system stands today ...
The education system in Portugal is regulated by the State through the Ministry of Education and the Ministry of Science, Technology and Higher Education. As we said, the public education system is the most used and best implemented, but there are excellent private schools at all levels of education. The school year configuration in Portugal ...
Students of public schools located in Lisbon, central and northern Portugal, have better grades than the rest. This isn't to say that other public schools aren't worth attending. Here is a list of the top 10 Portuguese public schools: Primary and Secondary School José Relvas, Santarém. Quinta das Palmeiras Secondary School, Covilhã.
The Portuguese education system. Schooling in Portugal is compulsory from ages six to 18, and the entire education system is governed by two departments. The Ministry of Education (Ministério da Educação) handles primary and secondary education, while the Ministry of Science, Technology and Higher Education (Ministério da Ciência ...
Portugal. This country note provides an overview of the key characteristics of the education system in Portugal. It draws on data from Education at a Glance 2023.In line with the thematic focus of this year's Education at a Glance, it emphasises vocational education and training (VET), while also covering other parts of the education system. Data in this note are provided for the latest ...
Portugal. In Portugal, the share of 25-34 year-olds with tertiary attainment increased substantially over the last two decades (from 13% in 2000 to 47% in 2021). Although the tertiary education is the most common highest level of attainment among Portuguese young adults, there is still 17% of 25-34 year-olds without upper secondary attainment ...
Looking for the best reasons to study abroad in Portugal? Beautiful beaches, high quality education, and a unique experience await while studying abroad. ... Beautiful beaches, high quality education, and a unique experience await while studying abroad. By using our site you agree to our use of cookies. Read our Cookie Policy for ...
In recent years, Portugal has made efforts to modernize its educational infrastructure, incorporating innovative teaching methods and technology to enhance the learning experience. The education system in Portugal is divided into the following educational levels: Preschool education (Educação Pré-escolar): Ages 3 to 6
State education is quite good in Portugal, but there are a few problems, including funding, insufficient teachers, and class sizes. Private schools . Many are faith-based, have smaller class sizes, and have more options for various clubs and activities. The costs are between €400 and €550 a month, depending on location.
Portugal might be one of the best sides at Euro 2024, but as been the case for the past two decades, most of the talk around in the lead-up to the tournament has been about the status of superstar ...
Despite the growing need for digital skills and the good employment prospects of students with degrees in information and communication technologies (ICT), only a small fraction of entrants into tertiary education choose this field. In Portugal, 96% of 25-64 year-olds with a tertiary ICT qualification are employed, but ICT students make up only ...
The U.S. surgeon general is calling for a warning label on social media alerting users that "social media is associated with significant mental health harms in adolescents." In a New York ...
This article appeared in the Science & technology section of the print edition under the headline "Soaring dragons"
Colorado and Virginia recently passed laws banning legacy admissions at public institutions of higher education. Maryland has done so at both public and private institutions.
BATON ROUGE, La. (AP) — Louisiana has become the first state to require that the Ten Commandments be displayed in every public school classroom under a bill…
Catherine, Princess of Wales joined other British royals on the balcony of Buckingham Palace for the King's official birthday, capping her first public appearance since being diagnosed with cancer.
Ruckdaschel, Jay Ronald (April 23, 1933 - June 9, 2024) A loving father, tender and kind, what beautiful memories you left behind. --Anonymous Jay Ronald Ruckdaschel, age 91, died peacefully at ...