- Open access
- Published: 06 October 2020

Quantitative detection and staging of presymptomatic cognitive decline in familial Alzheimer’s disease: a retrospective cohort analysis
- Antoinette O’Connor ORCID: orcid.org/0000-0003-4467-128X 1 , 2 ,
- Philip S. J. Weston 1 ,
- Ivanna M. Pavisic 1 , 2 ,
- Natalie S. Ryan 1 , 2 ,
- Jessica D. Collins 1 ,
- Kirsty Lu 1 ,
- Sebastian J. Crutch 1 ,
- Daniel C. Alexander 3 ,
- Nick C. Fox 1 , 2 na1 &
- Neil P. Oxtoby 3 na1
Alzheimer's Research & Therapy volume 12 , Article number: 126 ( 2020 ) Cite this article
3510 Accesses
10 Citations
18 Altmetric
Metrics details
Understanding the earliest manifestations of Alzheimer’s disease (AD) is key to realising disease-modifying treatments. Advances in neuroimaging and fluid biomarkers have improved our ability to identify AD pathology in vivo. The critical next step is improved detection and staging of early cognitive change. We studied an asymptomatic familial Alzheimer’s disease (FAD) cohort to characterise preclinical cognitive change.
Data included 35 asymptomatic participants at 50% risk of carrying a pathogenic FAD mutation. Participants completed a multi-domain neuropsychology battery. After accounting for sex, age and education, we used event-based modelling to estimate the sequence of cognitive decline in presymptomatic FAD, and uncertainty in the sequence. We assigned individuals to their most likely model stage of cumulative cognitive decline, given their data. Linear regression of estimated years to symptom onset against model stage was used to estimate the timing of preclinical cognitive decline.
Cognitive change in mutation carriers was first detected in measures of accelerated long-term forgetting, up to 10 years before estimated symptom onset. Measures of subjective cognitive decline also revealed early abnormalities. Our data-driven model demonstrated subtle cognitive impairment across multiple cognitive domains in clinically normal individuals on the AD continuum.
Conclusions
Data-driven modelling of neuropsychological test scores has potential to differentiate cognitive decline from cognitive stability and to estimate a fine-grained sequence of decline across cognitive domains and functions, in the preclinical phase of Alzheimer’s disease. This can improve the design of future presymptomatic trials by informing enrichment strategies and guiding the selection of outcome measures.
Alzheimer’s disease (AD) is clinically characterised by slowly progressive cognitive decline that leads to multi-domain impairment and loss of independence. It is estimated that currently 50 million people worldwide are living with symptomatic AD with prevalence expected to triple by the year 2050 [ 1 ]. There is an urgent economic and social need for a treatment that can slow disease progression.
To date, clinical trials in symptomatic AD have failed to identify a disease modifying treatment. Possible reasons include inadequate enrichment/screening (non-responders will dilute any treatment effect) and insensitive cognitive end-points. In addition, it is increasingly acknowledged that there is a need for earlier intervention [ 2 ]. The pathological process of AD begins over a decade prior to symptom onset [ 3 ]. This clinically silent period opens up a treatment window at a potentially more tractable stage of the disease.
As we move towards presymptomatic trials, a greater understanding of the earliest cognitive changes of AD is needed. In particular, there is a need for sensitive measures of cognitive decline as future prevention trials will be assessed on cognitive end-points [ 4 ]. Although biomarkers will be important to detect disease effects ultimately, it will be critical to show benefit on cognitive and functional measures.
Familial Alzheimer’s disease (FAD) provides a unique opportunity to characterise the early changes in AD as this is a rare autosomal dominant condition where mutations are virtually 100% penetrant. FAD is caused by mutations in the Amyloid Precursor Protein ( APP ), Presenilin 1 ( PSEN1 ), and Presenilin 2 ( PSEN2 ) genes [ 5 , 6 , 7 ]. Despite its rarity, FAD displays similarities with typical AD in both clinical presentation and pathophysiology [ 8 , 9 ].
It is now well recognised that presymptomatic cognitive changes occur in these kindreds. Deficits in verbal episodic memory have been most widely recognised, but impairments in processing speed, executive function and general intelligence have also been reported [ 10 , 11 , 12 ]. There is increasing interest in finding neuropsychological measures that can extend the interval over which presymptomatic cognitive decline is detectable; in particular, such measures would be very useful to complement biomarker changes. Measures of accelerated long-term forgetting (ALF), a process originally described in temporal lobe epilepsy, may be particularly sensitive to hippocampal dysfunction in AD [ 13 , 14 ]. ALF refers to a process whereby new material is learned and retained normally, but is then forgotten at a more rapid rate than in those with normal hippocampal integrity and function. ALF has been shown to be a feature of asymptomatic APOE 4 carriers and FAD mutation carriers at a time when performance on standard neuropsychological measures is unimpaired [ 14 , 15 ].
In this study, we aimed to characterise the sequence and timing of cognitive decline in presymptomatic AD by analysing neuropsychological measures in an asymptomatic FAD cohort using an established data-driven computational model.
Study design and participants
We recruited participants from a longitudinal study of FAD at the Dementia Research Centre at University College London from January 2015 to April 2016. Participants are eligible for inclusion in this ongoing study if they are over 18 years of age and have a parent with genetically confirmed FAD or a clinical diagnosis of FAD. For this sub-study, participants needed to be asymptomatic, scoring zero on the Clinical Dementia Rating (CDR) scale [ 16 ], with neither they nor their informant reporting progressive cognitive decline. Participants were excluded from this sub-study if they had substantial co-existing neurological or psychiatric disease. The study was approved by the local Research Ethics Committee, and all participants provided written informed consent.
Genetic testing using Sanger sequencing was conducted to determine mutation status. Genetic results were provided only to statisticians to ensure that participants and study staff remained blind to genetic status. All participants identified an informant who was interviewed separately to gain a collateral history. Estimated years to symptom onset (EYO) was calculated by subtracting participants’ age at assessment from the age at which their affected parent first developed progressive cognitive decline using a semi-structured interview of family members; a negative value meaning that the participant was younger than the estimate of their probable age at onset.
Participants underwent a battery of neuropsychological assessments that cover a broad spectrum of cognitive domains and functions:
General intelligence assessed using Performance IQ (PIQ) and Verbal IQ (VIQ) [ 17 ]. We also tested for a change in intellectual function using the dementia index, derived by subtracting the current IQ (measured by the Wechsler Abbreviated Scale of Intelligence) [ 17 ] from the predicted premorbid IQ (measured by the National Adult Reading Test) [ 18 ].
Global cognition assessed using the Mini-Mental State Examination (MMSE) [ 19 ].
Episodic memory:
Recognition Memory Test (RMT) words and faces [ 20 ].
Camden Paired Associate Learning (PAL) Test [ 21 ].
Accelerated long-term forgetting (ALF), using procedures reported previously [ 14 ]. The measures of interest were the proportion of material (list, story, figure) retained at 30 min that was later recalled at 7 days.
Subjective memory concerns were recorded using the Everyday Memory Questionnaire (EMQ) [ 22 ].
Working memory assessed using digit span forwards and backwards.
Timed executive function assessed using the Digit Symbol Substitution Test (DSST) [ 17 ].
Inhibition assessed using the Stroop test [ 23 ].
Arithmetic assessed using the Graded Difficulty Arithmetic (GDA) test [ 24 ].
Statistical analysis
From cross-sectional data, we estimated the probable sequence of cognitive decline in presymptomatic FAD using an event-based model [ 25 , 26 ]. The event-based model converts input data into severity scores (probability of abnormality) to estimate an order in which a set of measures become abnormal, and also estimate uncertainty in that ordering. The ordering and its uncertainty are inferred from the combinations of normal and abnormal measurements across individuals, using cross-sectional data, without defined cut-points, and without requiring any a priori staging variable (such as EYO). Due to the uniqueness of our cognitive battery, there exists no separate cohort for external validation, so the model was validated internally through cross-validation (described below).
We first removed healthy linear trends (if present, in noncarriers) of test score with years of formal education, age and sex. Event severity (probability of cognitive abnormality) in each test score is determined here using Kernel Density Estimation mixture modelling [ 25 ]. Using a mixture model replaces disease labels (mutation carrier/noncarrier) with pre-/post-event labels to allow for different cognitive profiles (and disease severity) across mutation carriers. This enables the sequence to be estimated without reference to an approximate staging variable (like EYO), which is not possible with standard groupwise comparisons. Data from a single visit per individual (the visit of their first ALF test) was used to fit the model.
The event-based model parameters are estimated using Markov Chain Monte Carlo (MCMC) sampling of the posterior distribution, which includes the maximum likelihood estimates and an explicit estimate of uncertainty [ 26 ].
The event-based model provides a fine-grained patient staging utility [ 27 ]. Based on their cognitive test score profile, participants were assigned to a numerical model stage reflecting their most probable position along the event sequence. This model stage reflects an individual’s current state of cumulative cognitive decline across the battery of tests.
Our model was cross-validated using repeated stratified 5-fold cross-validation (CV). This involves randomly splitting the cohort into 5 subset folds of approximately equal size and equal representation across groups (carriers/non-carriers). The event-based model is then fit (trained) on four folds and tested on the remaining fold, five times (using each fold as a test set once). This process was repeated for 50 different random 5-fold partitions, for a total of 250 trained models that are averaged to produce a robust and generalisable cross-validated model. We quantified similarity across CV folds using the Bhattacharyya coefficient which takes values between 0 and 1. We calculated accuracy of patient staging across folds using the final model to produce the ground truth model stage for all participants. We report details of cross-validation experiments in Additional file 1 .
Presymptomatic trajectories of group-level cognitive decline were estimated from the cumulative time between cognitive decline events, estimated via Bayesian regression of EYO against model stage.
We first describe the demographics of our study participants, then present our experimental results on the sequence and timing of cognitive decline in presymptomatic FAD.
Study participants
Participants’ demographic details are shown in Table 1 . Of the 35 participants recruited, 21 were APP or PSEN1 mutation carriers (Table e1, Additional file 1 for mutations) and 14 were non-carriers. The median EYO of mutation carriers was −6.5 years (interquartile range −9.2 to −5.0 years). Carriers and non-carriers were well-matched for age, sex EYO and years of education (see Table 1 ).
Sequence of presymptomatic cognitive decline
Figure 1 shows the data-driven sequence of cognitive decline events in presymptomatic FAD, and uncertainty in the sequence, represented as a positional density map. High confidence (left-to-right) in the ordering (top-to-bottom) appears as a narrow diagonal. Lower confidence appears as broader regions.
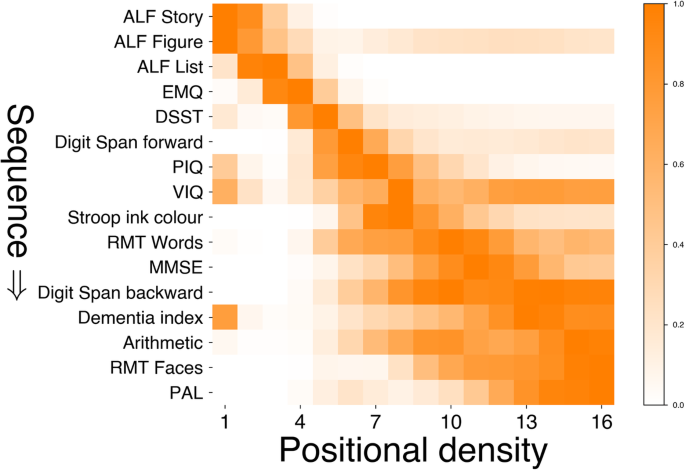
Estimated sequence of presymptomatic cognitive decline in FAD. Probabilistic heat map shows higher confidence (left-to-right) in the ordering (top-to-bottom) as hotter, narrow diagonal regions. Cooler, broader regions show lower confidence in the ordering of events. For abbreviations, see Table 1 caption
The model suggests the following sequence of cognitive decline in FAD: the first measure to become “abnormal” was ALF (story, figure and list), followed by subjective cognitive decline (EMQ), timed executive function (DSST), working memory (digit span: forwards), general intelligence (PIQ, subsequently VIQ) and inhibition (Stroop). Overlapping changes are then detected in other measures of episodic memory (RMT and later PAL), global cognition (MMSE) and arithmetic (GDA). This cross-validated model (see Statistical Methods and Additional file 1 ) reveals a robust and consistent sequence of presymptomatic cognitive decline: qualitatively, the positional density map is concentrated towards the diagonal, especially early in the sequence, and quantitatively, the Bhattacharyya coefficient for cross-validation similarity was 0.67.
Figure 2 demonstrates the staging potential of the model for this presymptomatic cohort. Each participant in the dataset was assigned a model stage that best reflects their measurements (see the “ Methods ” section and Young et al. [ 27 ]). Staging proportions for mutation carriers and noncarriers are shown in Fig. 2 a, where a clear separation is seen between mutation carriers (advanced stages) and noncarriers (earliest stages, except for one outlier with a discordant cognitive profile; see Fig. 2 caption). This separation can be exploited to classify future patients (mutation carriers) from non-carriers in this presymptomatic cohort with very high accuracy, as shown in Fig. 2 c—90% balanced accuracy is achieved by classifying participants at stage 2 (ALF Story + ALF Figure) or above as future patients. This suggests that our generative model may be useful for discriminative applications in preclinical Alzheimer’s disease. Figure 2 b shows that model stage correlates with EYO in mutation carriers, despite EYO being completely separate from the model.
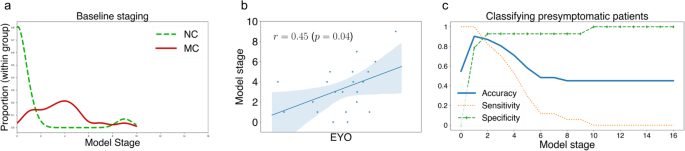
Model staging results. a Model stage distribution by mutation status. Smoothed histograms of individual model stage in mutation carriers (red) and noncarriers (broken green). Mutation carriers are at more-advanced stages, with all noncarriers except for one outlier being at stage one (ALF Story) or zero. This outlier demonstrated inconsistencies in cognitive performance with discordant scoring across tests of the same cognitive domain, e.g. low event probability for ALF list vs high event probability for ALF story. b Model stage correlates with EYO in mutation carriers. Model stage correlates with EYO ( x -axis labels omitted to avoid unblinding). Linear regression gives r 2 = 0.24 ( p < 0.05). c Model stage can be used to classify patients (mutation carrier) from controls (noncarriers) at a very early presymptomatic stage, with very high accuracy (> 90%). Abbreviations: NC, noncarrier; MC, mutation carrier
Timing of presymptomatic cognitive decline
Figure 3 shows our model-estimated curves for the timing of preclinical cognitive decline in this FAD cohort. Our model demonstrates data-driven cognitive abnormality as a function of EYO, estimated by combining our cross-validated model (Fig. 1 ) with EYO—see Statistical Methods and Additional file 1 for details. Our model first detects decline in measures of accelerated long-term forgetting; verbal measures (ALF Story and List) begin to change before visual measures (ALF Figure). In this asymptomatic cohort, our model estimates that changes in measures of ALF start approximately a decade prior to estimated symptom onset. Levels of self-reported subjective cognitive impairment also begin to increase around this time. Later changes are seen in measures of timed executive function (DSST) and general intelligence (PIQ). As estimated symptom onset approaches, subtle changes start to occur in measures of inhibition (Stroop), verbal intelligence (VIQ), global cognition (MMSE), memory (RMT, PAL, digit span), and arithmetic (GDA).
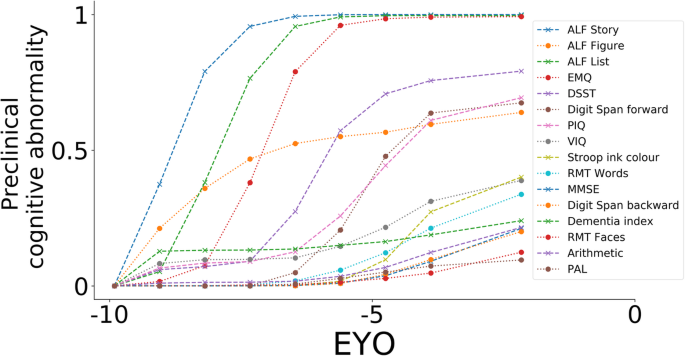
Data-driven preclinical cognitive decline in familial Alzheimer’s disease. Cumulative probability of preclinical abnormality as a function of years prior to estimated disease onset. A plateau means the data suggest that preclinical cognitive decline has stalled—it does not mean that the test score is at floor/ceiling. Progression sequence is that of the cross-validated event-based model in Fig. 1
Our work uses data-driven modelling to assess the sequence, timing and uncertainties of presymptomatic cognitive decline, advancing existing understanding of cognitive impairment in preclinical AD and providing a mechanism for detailed staging of presymptomatic individuals. The probabilistic cascade broadly agrees with current knowledge of cognitive decline in Alzheimer’s disease. Here we discuss our results in the context of previous work and highlight new avenues for further investigation.
Previous studies in sporadic AD and FAD had demonstrated impairments in long-term retention (i.e. accelerated forgetting) in clinically normal individuals and have shown that severity correlates with proximity to symptom onset [ 14 , 15 ]. Our data-driven modelling of a clinically normal FAD cohort provides an insight into the timing of presymptomatic cognitive decline. In this cohort, we found changes in measures of accelerated forgetting starting approximately a decade prior to estimated symptom onset. This timing overlaps with the onset of metabolic changes in fluorodeoxyglucose PET in previous studies of FAD [ 3 ]. Cerebral hypometabolism reflects synaptic dysfunction which would be expected to result in cognitive decline. However, cognitive changes this far from symptom onset have not been routinely detected by traditional neuropsychology measures.
Our results reveal a distinct ordering of cognitive decline in presymptomatic mutation carriers. Our model demonstrates early changes in tests of timed executive function, inhibition and performance IQ. This result is consistent with previous findings of early deficits in fronto-subcortical and general intellectual function in AD [ 10 , 11 , 28 ]. Preclinical change in measures of processing speed and executive function has also been reported in cognitively normal amyloid-positive individuals [ 29 ]. These results suggest that neuronal damage due to AD may be widespread even at an asymptomatic stage, highlighting the importance of conducting multi-domain cognitive testing in future presymptomatic studies.
Studies modelling cognition in preclinical late onset AD (LOAD) have also shown the value of multi-domain testing [ 30 ]. Cognitive composites, like the preclinical Alzheimer’s cognitive composite (PACC) which is a combined measure of episodic memory, executive function and global cognition, have demonstrated declining performance amongst asymptomatic amyloid-positive groups [ 31 , 32 ]. We also found change in these domains amongst cognitively normal individuals on the FAD continuum. Additionally, our staging model demonstrates differences in the ordering of change across these individual domains, declines in sensitive measures of memory (ALF) preceding declines in executive function (DSST) and global cognition (MMSE). Similarly, individual measures within the PACC do not change uniformly [ 33 ]. Consistent with our findings in FAD, sensitive memory tests detect early decline in preclinical LOAD, potentially even before the threshold of amyloid positivity is reached, while measures of global cognition begin to change closer to symptom onset [ 33 , 34 ].
Our results on the timing of presymptomatic cognitive decline reveal differences in verbal and visual measures. Abnormality in verbal measures of accelerated forgetting preceded changes in the visual measure; this needs to be replicated in a larger longitudinal study but implies verbal tests may be particularly adept at detecting early cognitive decline in FAD. This finding holds also with other verbal measures of episodic memory (RMT Words), which showed decline before visual measures (RMT Faces) in our study, as found previously [ 10 ]. Given that verbal episodic memory is critically dependent on the left hippocampus, whereas visual memory is more dependent on right hemispheric function, our results are consistent with a previous report of earlier left sided hippocampal atrophy in FAD [ 35 ]. However, this result needs to be interpreted with caution as there is no clear consensus on the hemispheric lateralisation of preclinical hippocampal atrophy in FAD [ 36 ].
There is growing interest in the clinical utility of subjective cognitive decline in AD. Memory concerns have demonstrated associations with pathological hallmarks of disease (cortical tau deposition as well as amyloid accumulation) [ 37 , 38 ]. Subjective cognitive decline may, at least in some individuals, predict subsequent cognitive decline and progression to dementia. However, in FAD, there have been conflicting reports on the utility of measures of subjective cognitive decline [ 39 , 40 ]. In our study, self-reported decline appeared early in the sequence of preclinical cognitive change. Our finding is consistent with a previous report of higher self-reported memory concerns in mutation carriers compared to non-carriers in an asymptomatic PSEN1 kindred [ 40 ]. Similar results for predicting symptomatic progression in sporadic AD [ 41 ] add credence to the idea that some level of self-insight into cognitive difficulties is present during the preclinical course of AD.
Our finding that tests of different cognitive measures are sensitive to detecting change over different time periods is analogous to hypothetical models of AD progression [ 42 ] where biomarkers are dynamic during finite, sequential intervals. Here we only investigated the preclinical phase, so while the plateaus in Fig. 3 may suggest that preclinical cognitive decline has slowed, longitudinal follow-up into the symptomatic phase is needed to clarify the full dynamic range of these measures. Such longitudinal work will also be important to fully characterise cognitive trajectories, as non-uniform patterns of change across neuropsychology measures have previously been demonstrated in prodromal AD [ 43 ]. Notwithstanding this, our results may be helpful to inform the selection of neuropsychology measures in clinical testing, as well as the choice of screening and outcome measures in future trials. For example, in AD prevention trials, accelerated forgetting (ALF Story and List) and subjective cognitive decline (EMQ) may be useful for early screening, while other measures (e.g. Performance IQ) may be more useful as outcome measures. This could be operationalised using our model staging utility: individuals at earlier model stages might be deemed suitable for screening into an early intervention clinical trial, and model stage could be used longitudinally as an outcome measure. Model stage could also inform clinical decision making and prognostication; individuals at later model stage being at higher risk of subsequent progression.
Our model demonstrates a high degree of certainty for the staging of early events; however, there is significant overlap for later cognitive measures. Phenotypic heterogeneity, which will result in inter-individual variability in cognitive profiles [ 44 ], may be contributing to this uncertainty. Alternatively, overlay in event ordering may be due to these neuropsychology measures declining simultaneously. However, one needs to be cautious when interpreting the significance of our model staging, particularly for late events, as mutation carriers in this study were on average over 7 years away from estimated symptom onset. Individuals this many years from symptom onset often show little to no change in many neuropsychological measures [ 3 , 11 , 12 ]. Future work in larger FAD cohorts with greater sampling of the late preclinical phase is needed to better clarify the timing of cognitive changes across the preclinical-clinical watershed, which could include data-driven subtyping [ 45 ].
Limitations
Our study has limitations. First, the sample size is small ( n = 35). Therefore, replication of our findings in other, preferably large FAD cohorts, like the Dominantly Inherited Alzheimer’s Network, is desirable [ 46 ]. Ideally such a cohort would be followed into the symptomatic phase. Such longitudinal data could be used to test the longitudinal consistency of our model while also offering the potential to personalise the model using within-person cognitive change. Follow-up data also enables fitting of alternative disease progression models such as differential equation models which could also be used to inform cognitive trajectories and timings [ 47 , 48 ]. Secondly, the known inaccuracies in EYO [ 49 ] limits the accuracy of our estimates of the timing of presymptomatic cognitive decline. Ideally, we would repeat our analysis on this same data using actual age of onset in place of EYO, after following all individuals through to symptom onset. Third, neuropsychological tests of any given domain are not uniformly sensitive, and inclusion of more sensitive measures may lead to changes in the position of that domain within the sequence. Finally, we did not account for practice effects, which tend to confound assessment of cognitive decline. This is also a strength of our study in that we were able to estimate the sequence and timing of presymptomatic cognitive decline, despite not removing this potential confounder.
In summary, our study has exploited an established computational modelling approach to reveal novel insight into the precise nature of presymptomatic cognitive decline in AD, using only cross-sectional data from a unique battery of neuropsychological tests. We have argued in favour of the utility of our data-driven findings for enabling and empowering presymptomatic clinical trials in Alzheimer’s disease.
Availability of data and materials
The datasets used and/or analysed during the current study are available from the corresponding author on reasonable request.
Abbreviations
Alzheimer’s disease
Accelerated long-term forgetting
Amyloid Precursor Protein
Clinical Dementia Rating Scale
Cross-validation
Digit Symbol Substitution Test
Everyday Memory Questionnaire
Estimated years to symptom onset
- Familial Alzheimer’s disease
Graded Difficulty Arithmetic
late onset Alzheimer’s disease
Markov Chain Monte Carlo
Mini-Mental State Examination
Paired Associate Learning
Performance IQ
Presenilin 1
Presenilin 2
Recognition Memory Test
GBD 2016 Dementia Collaborators E, Szoeke CEI, Vollset SE, Abbasi N, Abd-Allah F, Abdela J, et al. Global, regional, and national burden of Alzheimer’s disease and other dementias, 1990–2016: a systematic analysis for the Global Burden of Disease Study 2016. Lancet Neurol. 2019;18(1):88–106.
Article Google Scholar
Sperling RA, Rentz DM, Johnson KA, Karlawish J, Donohue M, Salmon DP, et al. The A4 study: stopping AD before symptoms begin? Sci Transl Med. 2014;6(228):228fs13.
Article PubMed PubMed Central CAS Google Scholar
Bateman RJ, Xiong C, Benzinger TL, Fagan AM, Goate A, Fox NC, et al. Clinical and biomarker changes in dominantly inherited Alzheimer’s disease. N Engl J Med. 2012;3679367(30):795–804.
Article CAS Google Scholar
Fda, Cder, Mccrayk. Early Alzheimer’s disease: developing drugs for treatment guidance for industry 2018;.
Google Scholar
Levy-Lahad E, Wasco W, Poorkaj P, Romano D, Oshima J, Pettingell W, et al. Candidate gene for the chromosome 1 familial Alzheimer’s disease locus. Science (80- ). 1995;269(5226):973–7.
Sherrington R, Rogaev EI, Liang Y, Rogaeva EA, Levesque G, Ikeda M, et al. Cloning of a gene bearing missense mutations in early-onset familial Alzheimer’s disease. Nature. 1995;375(6534):754–60.
Article CAS PubMed Google Scholar
Goate A, Chartier-Harlin M-C, Mullan M, Brown J, Crawford F, Fidani L, et al. Segregation of a missense mutation in the amyloid precursor protein gene with familial Alzheimer’s disease. Nature. 1991;349(6311):704–6.
Cairns NJ, Perrin RJ, Franklin EE, Carter D, Vincent B, Xie M, et al. Neuropathologic assessment of participants in two multi-center longitudinal observational studies: the Alzheimer Disease Neuroimaging Initiative (ADNI) and the Dominantly Inherited Alzheimer Network (DIAN). Neuropathology. 2015;35(4):390–400.
Article PubMed PubMed Central Google Scholar
Bateman RJ, Aisen PS, De Strooper B, Fox NC, Lemere CA, Ringman JM, et al. Autosomal-dominant Alzheimer’s disease: a review and proposal for the prevention of Alzheimer’s disease. Alzheimers Res Ther. 2010;3(1):1.
Fox NC, Warrington EK, Seiffer AL, Agnew SK, Rossor MN, Rossor MN. Presymptomatic cognitive deficits in individuals at risk of familial Alzheimer’s disease. A longitudinal prospective study. Brain. 1998;121:1631–9.
Article PubMed Google Scholar
Storandt M, Balota DA, Aschenbrenner AJ, Morris JC. Clinical and psychological characteristics of the initial cohort of the dominantly inherited alzheimer network (DIAN). Neuropsychology. 2014;28(1):19–29.
Aguirre-Acevedo DC, Lopera F, Henao E, Tirado V, Muñoz C, Giraldo M, et al. Cognitive decline in a Colombian kindred with autosomal dominant Alzheimer disease: a retrospective cohort study. JAMA Neurol. 2016;73(4):431–8.
Butler CR, Graham KS, Hodges JR, Kapur N, Wardlaw JM, Zeman AZJ. The syndrome of transient epileptic amnesia. Ann Neurol. 2007;61(6):587–98.
Weston PSJ, Nicholas JM, Henley SMD, Liang Y, Macpherson K, Donnachie E, et al. Accelerated long-term forgetting in presymptomatic autosomal dominant Alzheimer’s disease: a cross-sectional study. Lancet Neurol. 2018;17(2):123–32.
Zimmermann JF, Butler CR. Accelerated long-term forgetting in asymptomatic APOE ε4 carriers. Lancet Neurol. 2018;17(5):394–5.
Morris JC. The Clinical Dementia Rating (CDR): current version and scoring rules. Neurology. 1993;43(11):2412–4.
Weschler D. Wechsler Adult Intelligence Scale -Revised. New York: Psychological Corporation; 1981.
The National Adult Reading Test (NART) H a z e l E. N e l s o n w i t h J o n a t h a n W i l l i s o n Hazel E Nelson Part I: The Original Study NART and WAIS (1982).
Folstein MF, Folstein SE, McHugh PR. “Mini-mental state”: a practical method for grading the cognitive state of patients for the clinician. J Psychiatr Res. 1975;12(3):189–98.
Warrington E. Recognition Memory Test: Rmt.(Words). Test Booklet 1; 1984.
Warrington EK. The Camden memory tests : manual: Psychology Press; 1996. p. 16.
Rami L, Mollica MA, García-Sanchez C, Saldaña J, Sanchez B, Sala I, et al. The Subjective Cognitive Decline Questionnaire (SCD-Q): a validation study. J Alzheimers Dis. 2014;41(2):453–66.
Stroop JR. Studies of interference in serial verbal reactions. J Exp Psychol. 1935;18(6):643–62.
Jackson M, Warrington EK. Arithmetic skills in patients with unilateral cerebral lesions. Cortex. 1986 Dec 1;22(4):611–20.
Firth NC, Primativo S, Brotherhood E, Young AL, Yong KXX, Crutch SJ, et al. Sequences of cognitive decline in typical Alzheimer’s disease and posterior cortical atrophy estimated using a novel event-based model of disease progression. Alzheimer’s Dement. 2020;alz.12083.
Fonteijn HM, Modat M, Clarkson MJ, Barnes J, Lehmann M, Hobbs NZ, et al. An event-based model for disease progression and its application in familial Alzheimer’s disease and Huntington’s disease. Neuroimage. 2012;60(3):1880–9.
Young AL, Oxtoby NP, Daga P, Cash DM, Fox NC, Ourselin S, et al. A data-driven model of biomarker changes in sporadic Alzheimer’s disease. Brain. 2014;137(Pt 9):2564–77.
Binetti G, Magni E, Padovani A, Cappa SF, Bianchetti A, Trabucchi M, et al. Executive dysfunction in early Alzheimer’s disease. J Neurol Neurosurg Psychiatry. 1996;60:91–3.
Article CAS PubMed PubMed Central Google Scholar
Ho JK. Neuropsychological profiles and trajectories in preclinical Alzheimer’s disease. J Int Neuropsychol Soc. 2018;24:693–702.
Baker JE, Lim YY, Pietrzak RH, Hassenstab J, Snyder PJ, Masters CL, et al. Cognitive impairment and decline in cognitively normal older adults with high amyloid-β: a meta-analysis. 2017;.
Book Google Scholar
Donohue MC, Sperling RA, Salmon DP, Rentz DM, Raman R, Thomas RG, et al. The preclinical Alzheimer cognitive composite: measuring amyloid-related decline. JAMA Neurol. 2014;71(8):961–70.
Donohue MC, Sperling RA, Petersen R, Sun C-K, Weiner MW, Aisen PS. Association between elevated brain amyloid and subsequent cognitive decline among cognitively normal persons. JAMA. 2017;317(22):2305.
Mormino EC, Papp KV, Rentz DM, Donohue MC, Amariglio R, Quiroz YT, et al. Early and late change on the preclinical Alzheimer’s cognitive composite in clinically normal older individuals with elevated amyloid β. Alzheimers Dement. 2017 Sep 1;13(9):1004–12.
Insel PS, Donohue MC, Sperling R, Hansson O, Mattsson-Carlgren N. The A4 study: β-amyloid and cognition in 4432 cognitively unimpaired adults. Ann Clin Transl Neurol. 2020;7(5):776–85.
Kinnunen KM, Cash DM, Poole T, Frost C, Benzinger TLS, Ahsan RL, et al. Presymptomatic atrophy in autosomal dominant Alzheimer’s disease: a serial magnetic resonance imaging study. Alzheimers Dement. 2018;14(1):43–53.
Fox NC, Warrington EK, Freeborough PA, Hartikainen P, Kennedy AM, Stevens JM, et al. Presymptomatic hippocampal atrophy in Alzheimer’s disease: a longitudinal MRI study. Brain 1996;119(6):2001–2007.
Buckley RF, Hanseeuw B, Schultz AP, Vannini P, Aghjayan SL, Properzi MJ, et al. Region-specific association of subjective cognitive decline with tauopathy independent of global β-amyloid burden. JAMA Neurol. 2017;74(12):1455.
La Joie R, Perrotin A, Egret S, Pasquier F, Tomadesso C, Mézenge F, et al. Qualitative and quantitative assessment of self-reported cognitive difficulties in nondemented elders: association with medical help seeking, cognitive deficits, and β-amyloid imaging. Alzheimer’s Dement Diagnosis Assess Dis Monit. 2016;5:23–34.
Laske C, Sohrabi HR, Jasielec MS, Müller S, Koehler NK, Gräber S, et al. Diagnostic value of subjective memory complaints assessed with a single item in dominantly inherited Alzheimer’s disease: results of the DIAN study. Biomed Res Int. 2015;2:2015.
Norton DJ, Amariglio R, Protas H, Chen K, Aguirre-Acevedo DC, Pulsifer B, et al. Subjective memory complaints in preclinical autosomal dominant Alzheimer disease. Neurology. 2017;89(14):1464–70.
Buckley RF, Maruff P, Ames D, Bourgeat P, Martins RN, Masters CL, et al. Subjective memory decline predicts greater rates of clinical progression in preclinical Alzheimer’s disease. Alzheimers Dement. 2016;12(7):796–804.
Jack CR, Knopman DS, Jagust WJ, Shaw LM, Aisen PS, Weiner MW, et al. Hypothetical model of dynamic biomarkers of the Alzheimer’s pathological cascade. Lancet Neurol. 2010;9(1):119–28.
Mura T, Proust-Lima C, Jacqmin-Gadda H, Akbaraly TN, Touchon J, Dubois B, et al. Measuring cognitive change in subjects with prodromal Alzheimer’s disease. J Neurol Neurosurg Psychiatry. 2014;85(4):363–70.
Scheltens NME, Galindo-Garre F, Pijnenburg YAL, van der Vlies AE, Smits LL, Koene T, et al. The identification of cognitive subtypes in Alzheimer’s disease dementia using latent class analysis. J Neurol Neurosurg Psychiatry. 2016;87(3):235–43.
Young AL, Marinescu RV, Oxtoby NP, Bocchetta M, Yong K, Firth NC, et al. Uncovering the heterogeneity and temporal complexity of neurodegenerative diseases with subtype and stage inference. Nat Commun. 2018;9(1):4273.
Moulder KL, Snider BJ, Mills SL, Buckles VD, Santacruz AM, Bateman RJ, et al. Dominantly inherited alzheimer network: facilitating research and clinical trials. Alzheimer’s Res Therapy. 2013;5:1–7.
Villemagne VL, Burnham S, Bourgeat P, Brown B, Ellis KA, Salvado O, et al. Amyloid β deposition, neurodegeneration, and cognitive decline in sporadic Alzheimer’s disease: a prospective cohort study. Lancet Neurol. 2013;12(4):357–67.
Oxtoby NP, Young AL, Cash DM, Benzinger TLS, Fagan AM, Morris JC, et al. Data-driven models of dominantly-inherited Alzheimer’s disease progression. Brain. 2018;141(5):1529–44.
Ryman DC, Acosta-Baena N, Aisen PS, Bird T, Danek A, Fox NC, et al. Symptom onset in autosomal dominant Alzheimer disease: a systematic review and meta-analysis. Neurology. 2014;83(3):253–60.
Download references
Acknowledgements
Not applicable.
AOC is an Alzheimer’s Society Clinical Research Fellow (AS-CTF-18-001). We acknowledge support from the EuroPOND project—this project has received funding from the European Union’s Horizon 2020 research and innovation programme under grant agreement number 666992. NPO and DCA acknowledge support from the UCL Centre for Medical Image Computing via the UK’s EPSRC grant number EP/M020533/1. This work was supported by the NIHR University College London Hospitals Biomedical Research Centre and UK Dementia Research Institute and Rosetrees Trust. NSR is supported by a University of London Chadburn Academic Clinical Lectureship in Medicine. SJC was supported by an EPSRC grant (EP/M006093/1). NPO is a UKRI Future Leaders Fellow (MRC MR/S03546X/1).
Author information
Nick C. Fox and Neil P. Oxtoby contributed equally to this work.
Authors and Affiliations
Dementia Research Centre, UCL Queen Square Institute Of Neurology, 8-11 Queen Square, London, WC1N 3AR, UK
Antoinette O’Connor, Philip S. J. Weston, Ivanna M. Pavisic, Natalie S. Ryan, Jessica D. Collins, Kirsty Lu, Sebastian J. Crutch & Nick C. Fox
UK Dementia Research Institute at UCL, UCL, London, UK
Antoinette O’Connor, Ivanna M. Pavisic, Natalie S. Ryan & Nick C. Fox
Department of Computer Science, UCL Centre for Medical Image Computing, 1st Floor, 90 High Holborn, London, WC1V 6LJ, UK
Daniel C. Alexander & Neil P. Oxtoby
You can also search for this author in PubMed Google Scholar
Contributions
AOC, NPO and NCF conceived the study. AOC, PSJW, AOC, KL and JDC contributed to participant recruitment and data collection. NPO and AOC were responsible for data management and data analyses. All authors were involved in the interpretation of results and writing the report. The authors read and approved the final manuscript.
Corresponding authors
Correspondence to Antoinette O’Connor or Neil P. Oxtoby .
Ethics declarations
Ethics approval and consent to participate.
The study was approved by the Queen Square Research Ethics Committee, and all participants provided written informed consent.
Consent for publication
Not applicable
Competing interests
Additional information, publisher’s note.
Springer Nature remains neutral with regard to jurisdictional claims in published maps and institutional affiliations.
Supplementary information
Additional file 1..
Details of cross-validation experiments
Rights and permissions
Open Access This article is licensed under a Creative Commons Attribution 4.0 International License, which permits use, sharing, adaptation, distribution and reproduction in any medium or format, as long as you give appropriate credit to the original author(s) and the source, provide a link to the Creative Commons licence, and indicate if changes were made. The images or other third party material in this article are included in the article's Creative Commons licence, unless indicated otherwise in a credit line to the material. If material is not included in the article's Creative Commons licence and your intended use is not permitted by statutory regulation or exceeds the permitted use, you will need to obtain permission directly from the copyright holder. To view a copy of this licence, visit http://creativecommons.org/licenses/by/4.0/ . The Creative Commons Public Domain Dedication waiver ( http://creativecommons.org/publicdomain/zero/1.0/ ) applies to the data made available in this article, unless otherwise stated in a credit line to the data.
Reprints and permissions
About this article
Cite this article.
O’Connor, A., Weston, P.S.J., Pavisic, I.M. et al. Quantitative detection and staging of presymptomatic cognitive decline in familial Alzheimer’s disease: a retrospective cohort analysis. Alz Res Therapy 12 , 126 (2020). https://doi.org/10.1186/s13195-020-00695-2
Download citation
Received : 09 July 2020
Accepted : 17 September 2020
Published : 06 October 2020
DOI : https://doi.org/10.1186/s13195-020-00695-2
Share this article
Anyone you share the following link with will be able to read this content:
Sorry, a shareable link is not currently available for this article.
Provided by the Springer Nature SharedIt content-sharing initiative
- Preclinical
- Neuropsychology
- Event-based modelling
Alzheimer's Research & Therapy
ISSN: 1758-9193
- General enquiries: [email protected]
- Reference Manager
- Simple TEXT file
People also looked at
Editorial article, editorial: quantitative imaging methods and analysis in alzheimer's disease assessment.
- 1 European University Cyprus, Engomi, Cyprus
- 2 Department of Radiology and Imaging Sciences, School of Medicine, Emory University, Atlanta, GA, United States
- 3 Senior Lecturer in Biomedical Image Analysis giCentre, Department of Computer Science, School of Science and Technology City, University of London, London, United Kingdom
Editorial on the Research Topic Quantitative imaging methods and analysis in Alzheimer's disease assessment
Alzheimer's disease (AD) is a complex condition gradually eroding memory and cognitive abilities. While cognitive tests aid diagnosis, their limitations include an inability to detect subtle early-stage changes, subjective interpretation influenced by various factors, and inconsistent predictive accuracy. Imaging techniques play a pivotal role in AD detection by revealing structural and functional brain changes associated with the disease. Quantitative MRI highlights brain atrophy, ruling out other causes, while PET scans visualize hallmark proteins like beta-amyloid and tau. Cerebrospinal fluid (CSF) analysis assesses biomarkers linked to AD, notably Aβ42 and tau proteins. The APOE gene variants, specifically ε4, influence AD susceptibility. EEG, measuring brain electrical activity, offers supplementary information but isn't a primary diagnostic tool.
The goal of this Research Topic was to investigate the impact of quantitative imaging in the assessment of AD by using structural MRI or molecular neuroimaging such as PET as results from cognitive tests are not sufficient to accurately and reliably make the diagnosis of AD. Also, it evaluated the performance of deep learning methods in the classification and prediction of AD. Early detection is crucial for effective intervention and treatment.
• The study “ Piecing it together: atrophy profiles of hippocampal subfields relate to cognitive impairment along the Alzheimer's disease spectrum ,” investigated the relationship between AD and hippocampal subfield-specific neurodegeneration, aiming to enhance prognostic procedures. The findings suggest that considering subfield-specific hippocampal degeneration, along with cognitive assessments, could offer a sensitive prognostic approach for tracking disease trajectories in individuals on the AD spectrum ( Christopher-Hayes et al. ).
• The study “ A cross-sectional study of explainable machine learning in Alzheimer's disease: diagnostic classification using MR radiomic features” focused on improving the assessment of AD by addressing the limitations of cognitive tests and late-stage brain atrophy detection through qualitative imaging. The researchers used Machine Learning (ML) methods, particularly XGBoost, to analyze radiomic features from the entorhinal cortex and hippocampus. The use of explainable ML approaches, such as SHAP (SHapley Additive exPlanations), demonstrated the potential for earlier AD diagnosis, better disease management, and the development of novel treatment strategies ( Leandrou et al. ).
• The study “Early-stage mapping of macromolecular content in APP NL-F mouse model of Alzheimer's disease using nuclear overhauser effect (NOE) MRI” explored the potential of Nuclear Overhauser enhancement (NOE) MRI as a non-invasive method for early detection of AD. NOE MRI provides contrast sensitive to lipid and protein content in the brain, which are known to be altered in AD pathology. Using template-based analyses and optimizing parameters for NOE specificity, the researchers detected changes in lipids and proteins in an AD mouse model in the hippocampus, hypothalamus, entorhinal cortex, and fimbria, indicating disruptions in the phospholipid bilayer of cell membranes in both gray and white matter regions. The findings suggest that NOE MRI could be a valuable tool for monitoring early-stage changes in lipid-mediated metabolism in AD and potentially other disorders, offering high spatial resolution ( Swain et al. ).
• The study “ Bayesian workflow for the investigation of hierarchical classification models from Tau-PET and structural MRI Data across the Alzheimer's disease spectrum” addressed the challenges of early-stage AD diagnosis and explores the role of tau neurofibrillary tangles in brain networks. Using a Bayesian workflow and data from a longitudinal cohort, the researchers developed hierarchical multinomial logistic regression models based on tau-PET and structural MRI data. The findings suggest that considering hierarchical models encompassing ROI and network-level heterogeneity improves predictive accuracy in assessing AD using tau-PET and structural MRI data ( Belasso et al. ).
• The study “ A holo-spectral EEG analysis provides early detection of cognitive decline and predicts progression to Alzheimer's disease,” aimed to differentiate individuals with MCI and AD from cognitively normal (CN) individuals, as well as predict the progression from MCI to AD using a newly developed technique called Holo-Hilbert Spectral Analysis (HHSA) applied to resting state EEG (rsEEG). The HHSA analysis revealed distinctive patterns in amplitude modulation (AM) power across frequency bands in MCI and various AD stages compared to CN controls. ML algorithms successfully discriminated between groups with good sensitivity and specificity in cross-sectional analysis. In a longitudinal follow-up of MCI patients, HHSA identified significant differences in AM power between stable and converted MCI groups. The study suggests that integrating HHSA into EEG signals, combined with ML, holds promise for differentiating cognitive states and predicting progression in individuals with MCI ( Chu et al. ).
• The study “ APOE ε 4 accelerates the longitudinal cerebral atrophy in OASIS-3 elders without dementia at enrolment” investigated the modulation of the APOE gene on the trajectory of cerebral atrophy during the conversion from CN to dementia. Using a voxel-wise whole-brain perspective and data from the OASIS-3 neuroimaging cohort, the researchers applied a linear mixed-effects model to detect cerebrum regions with non-linear atrophic trajectories influenced by AD conversion and to examine the impact of APOE variants on these trajectories. Results showed that participants transitioning from CN to dementia exhibited faster quadratically accelerated atrophy in bilateral hippocampi compared to persistent CN individuals. The study contributes to understanding how APOE ε4 influences hippocampal atrophy acceleration and the progression from normal cognition to dementia ( Huang et al. ).
• The study “ Brain atrophy in non-demented individuals in a long term longitudinal study from two independent cohorts ” investigated the longitudinal atrophy rates in individuals with AD, those with increased phosphorylated Tau at Thr181 (pTau181) without amyloidopathy (A–T+N±), and biomarker-negative non-demented subjects. Using data from two independent cohorts, the results showed that individuals with increased pTau181 levels without amyloidopathy (A–T + N±) did not exhibit a unique longitudinal atrophy pattern compared to AD or biomarker-negative subjects. In contrast, those with both increased pTau181 and amyloidopathy (A+T + N±) showed marked longitudinal atrophy in the temporal lobe. The study suggests that increased pTau181 levels without concomitant amyloidopathy may not indicate a neurodegenerative disorder, as there was no significant atrophy rate compared to controls in these individuals ( Haas et al. ).
Future work entails addressing resolution limitations in imaging, enhancing quantitative assessment for precise analysis, understanding the disease's heterogeneity, and integrating diverse data sources. Identifying reliable imaging biomarkers specific to AD is crucial, requiring standardization in protocols and analysis methods across studies and clinical settings. Additionally, interpreting imaging findings accurately amid various health conditions and age-related changes is pivotal. Overcoming these challenges demands collaborative efforts, technological advancements, standardized protocols, and a deeper understanding of AD's intricate mechanisms.
Author contributions
SL: Writing – original draft. CR-A: Contributed to manuscript preparation and revision. DQ: Read and approved the final submitted version. All authors discussed the results and commented on the manuscript.
The author(s) declare that no financial support was received for the research, authorship, and/or publication of this article.
Conflict of interest
The author declares that the research was conducted in the absence of any commercial or financial relationships that could be construed as a potential conflict of interest.
Publisher's note
All claims expressed in this article are solely those of the authors and do not necessarily represent those of their affiliated organizations, or those of the publisher, the editors and the reviewers. Any product that may be evaluated in this article, or claim that may be made by its manufacturer, is not guaranteed or endorsed by the publisher.
Keywords: Alzheimer's disease, quantitative imaging, machine learning, PET, CSF, gene
Citation: Leandrou S, Qiu D and Reyes-Aldasoro CC (2024) Editorial: Quantitative imaging methods and analysis in Alzheimer's disease assessment. Front. Aging Neurosci. 15:1340877. doi: 10.3389/fnagi.2023.1340877
Received: 19 November 2023; Accepted: 23 November 2023; Published: 09 January 2024.
Edited and reviewed by: Allison B. Reiss , New York University, United States
Copyright © 2024 Leandrou, Qiu and Reyes-Aldasoro. This is an open-access article distributed under the terms of the Creative Commons Attribution License (CC BY) . The use, distribution or reproduction in other forums is permitted, provided the original author(s) and the copyright owner(s) are credited and that the original publication in this journal is cited, in accordance with accepted academic practice. No use, distribution or reproduction is permitted which does not comply with these terms.
*Correspondence: Stephanos Leandrou, s.leandrou@euc.ac.cy
This article is part of the Research Topic
Quantitative Imaging Methods and Analysis in Alzheimer's Disease Assessment
Thank you for visiting nature.com. You are using a browser version with limited support for CSS. To obtain the best experience, we recommend you use a more up to date browser (or turn off compatibility mode in Internet Explorer). In the meantime, to ensure continued support, we are displaying the site without styles and JavaScript.
- View all journals
- My Account Login
- Explore content
- About the journal
- Publish with us
- Sign up for alerts
- Data Descriptor
- Open access
- Published: 14 April 2023
A peptide-centric quantitative proteomics dataset for the phenotypic assessment of Alzheimer’s disease
- Gennifer E. Merrihew ORCID: orcid.org/0000-0003-4903-0318 1 ,
- Jea Park 1 ,
- Deanna Plubell 1 ,
- Brian C. Searle 2 ,
- C. Dirk Keene ORCID: orcid.org/0000-0002-5291-1469 3 ,
- Eric B. Larson 4 ,
- Randall Bateman ORCID: orcid.org/0000-0002-7729-1702 5 ,
- Richard J. Perrin 6 ,
- Jasmeer P. Chhatwal 7 ,
- Martin R. Farlow 8 ,
- Catriona A. McLean 9 ,
- Bernardino Ghetti 10 ,
- Kathy L. Newell 10 ,
- Matthew P. Frosch 11 ,
- Thomas J. Montine 12 &
- Michael J. MacCoss ORCID: orcid.org/0000-0003-1853-0256 1
Scientific Data volume 10 , Article number: 206 ( 2023 ) Cite this article
3587 Accesses
5 Citations
14 Altmetric
Metrics details
- Alzheimer's disease
Alzheimer’s disease (AD) is a looming public health disaster with limited interventions. Alzheimer’s is a complex disease that can present with or without causative mutations and can be accompanied by a range of age-related comorbidities. This diverse presentation makes it difficult to study molecular changes specific to AD. To better understand the molecular signatures of disease we constructed a unique human brain sample cohort inclusive of autosomal dominant AD dementia (ADD), sporadic ADD, and those without dementia but with high AD histopathologic burden, and cognitively normal individuals with no/minimal AD histopathologic burden. All samples are clinically well characterized, and brain tissue was preserved postmortem by rapid autopsy. Samples from four brain regions were processed and analyzed by data-independent acquisition LC-MS/MS. Here we present a high-quality quantitative dataset at the peptide and protein level for each brain region. Multiple internal and external control strategies were included in this experiment to ensure data quality. All data are deposited in the ProteomeXchange repositories and available from each step of our processing.
Similar content being viewed by others
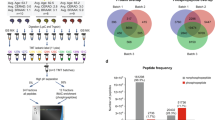
Global quantitative analysis of the human brain proteome and phosphoproteome in Alzheimer’s disease
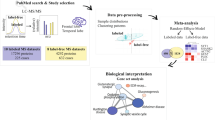
Insights into the changes in the proteome of Alzheimer disease elucidated by a meta-analysis
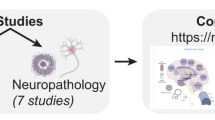
Compilation of reported protein changes in the brain in Alzheimer’s disease
Background & summary.
Alzheimer’s disease (AD) is a major global public health problem. In the US, AD is the seventh most common cause of death for all ages and sexes. In contrast to ischemic heart disease, stroke and several forms of cancer, AD is increasing as a cause of death, of years lived with disability, and of disability-adjusted life years 1 . Success in limiting acute illnesses in the developing world is shifting the burden to non-communicable diseases, with an expected dramatic rise in AD globally by 2025 2 . Existence of forms of AD with known genetic causes or risk, and forms without known genetic underpinnings, highlight the potential for multiple molecular drivers and perhaps multiple pathogenic pathways involved in disease onset and progression. Moreover, longitudinal population-based cohort studies have repeatedly observed that AD is commonly comorbid with pathologic changes of vascular brain injury (VBI), Lewy body disease (LBD), limbic-associated TDP-43 encephalopathy (LATE), and/or hippocampal sclerosis 3 , 4 . AD is a chronic illness whose ultimate clinical expression as dementia follows years, if not decades, of injury, response to injury, consumption of reserve, and exhaustion of compensation. Determining the molecular profile of its various forms independent of comorbidities will be fundamental to efforts to develop tailored therapies that specifically target the molecular mechanism(s) of AD.
Thus, a primary focus of this data resource was the selection of tissue specimens based on current guidelines for AD neuropathologic change (ADNC) 3 , 4 and specific exclusion for the presence of alternative potential causes of dementia, resulting in the carefully annotated examples of AD and controls free of medically significant comorbidities. Additionally, the specimens selected spanned the range of disease severity from the categories designated as high cognitive function (HCF) with no or low ADNC, HCF with intermediate or high ADNC, sporadic AD dementia (ADD, with intermediate or high ADNC), and autosomal dominant ADD (with causal mutations in PSEN1 , PSEN2 , or APP , and high ADNC). All research participants whose brains were used for this study underwent detailed, research quality, longitudinal cognitive assessments. For individuals without dementia, all had their last evaluation within 2 years of death and had neuropsychological test results in the upper quartile of the cohort to minimize interval conversion. All samples were obtained using a rapid autopsy protocol with postmortem interval less than 8 hours (except autosomal dominant AD due to practical limitations), flash frozen in liquid nitrogen, and kept frozen at −80 °C prior to analysis. All samples were matched for age and sex except for those from individuals with autosomal dominant AD who experienced earlier onset.
Due to this careful specimen selection, we now have a unique sample set that can be used to study the molecular underpinnings of autosomal dominant ADD, sporadic ADD, and high burden ADNC with HCF without the confounding comorbidities faced in similar molecular profiling experiments. While previous studies have investigated the molecular profile of AD without excluding comorbidities, the high prevalence of these diseases, each of which can cause dementia on its own when present at high level, likely underlines the specificity of these profiles for AD. Likewise, because autosomal dominant AD is rare, typical molecular profiling studies have focused only on individuals with sporadic ADD, thereby limiting perspective on this heterogeneous disease. These points emphasize the uniqueness of this proteomics dataset for a more comprehensive assessment of the different forms of AD.
To make the most of this unique sample cohort, we used mass spectrometry proteomics methods that give reproducible and highly quantitative data. Most of the large-scale proteomics experiments studying the human brain have been performed using a mass spectrometry approach known as data-dependent acquisition (DDA). This approach is extremely powerful for building lists of proteins present in brain tissue and has been useful when combined with tandem mass tags for modest numbers of samples that can be performed within a “plex”. However, irregular sampling by DDA makes it challenging to provide robust and quantitative measurements across more samples than can fit in a plex. When using DDA, the number of peptides sampled is limited by the MS/MS sampling speed despite the dynamic range and peak capacity of the mass analyzer. A single MS spectrum can contain over one hundred different molecular species, of which only a handful are analyzed by MS/MS prior to the next full scan 5 . This general approach has become extremely powerful for cataloging proteins and modifications, but its irregular sampling results in missing data, requires extensive fractionation to sample low abundance peptides, and results in variable peptide sampling between runs of the same sample. Although the missing values in multiplexing tandem mass tags can be reduced at a protein level, it remains a problem on the peptide level due to the variable peptide sampling between runs 6 . In AD it is known that a subset of residues or peptides may be altered in disease, such as the amyloid-β region of amyloid precursor protein APP, or the proline-rich domain of Tau (MAPT) 2 . Consistent sampling and quantification on the peptide level is therefore necessary to accommodate such cases 7 .
An alternative to DDA is an acquisition approach known as data independent acquisition (DIA) that acquires comprehensive MS/MS information in a single LC-MS/MS run using a repeated cycle of wide-window MS/MS scans. The computational analysis of DIA spectra can be performed in the same “targeted” manner as fully targeted data; i.e., fragment ion chromatograms for each peptide can be extracted and used for quantification. However, unlike fully targeted data acquisition, DIA analysis can be done for any peptide in the sampled range (e.g., between 400 and 1000 m/z ), rather than just for a subset of pre-specified peptides. Thus, the reproducible targeting and confident MS/MS-based quantification of parallel reaction monitoring (PRM) can be combined with DDA’s ability to detect and measure thousands of proteins. Like PRM methods, DIA requires reproducible chromatographic separation of peptides for reproducible quantification. This systematic sampling is important when scaling to data sets with samples prepared and run over many batches.
Typically, tens to hundreds of biological samples are processed and analyzed using LC-MS/MS in quantitative proteomics experiments. The regularity of DIA enables researchers to make peptide detections in one sample and use that information to inform the detection of the same peptides in other samples. DIA offers four key improvements over DDA. (1) Because peptides are sampled systematically, more peptides are detected in a DIA analysis than a DDA analysis in an equivalent length analysis 8 , 9 . (2) The same precursor m/z range is sampled, at the same RT, in all runs – eliminating the issues associated with stochastically sampled DDA data. (3) DIA analysis can make use of previously measured information to improve peptide measurement (e.g. known retention time, known fragmentation patterns, and which peptides provide stable and precise quantitative measurements) 10 , 11 , 12 , 13 , 14 . (4) Peptide detection can be assessed directly from DIA data, simplifying downstream analysis. These data provide an archive of all detectable molecular species within the measured mass range of the instrument. This methodology benefits from the reproducible and comprehensive sampling of the latest DIA methodology with an innovative approach used to improve peptide precursor selectivity 5 . The combination of the unique specimens with systematically collected mass spectrometry data creates a resource for the scientific community to test new hypotheses about the molecular features of different forms of AD dementia.
Human brain samples
Brain tissue samples were stratified into 4 groups based on clinical, pathological and genetic data and four brain regions (superior and middle temporal gyri or SMTG, hippocampus at the level of the lateral geniculate nucleus, inferior parietal lobule or IPL and caudate nucleus at the level of the anterior commissure). Cognitive status was determined as dementia or not dementia by DSM-IVR criteria. Individuals diagnosed as not dementia were from the Adult Changes in Thought (ACT) study and were included only if the last research evaluation was within 2 years of death and the last cognitive testing score using the cognitive abilities screening instrument (CASI) was in the upper quartile for the ACT cohort (>90); our definition of HCF. Brains from individuals with HCF who had no or low ADNC were designated “HCF/low ADNC” and those with intermediate or high ADNC were designated “HCF/high ADNC”. All individuals diagnosed with ADD had intermediate or high level ADNC and were further subclassified as sporadic (“Sporadic ADD”) or ADD caused by a mutation in PSEN1, PSEN2 , or APP (“Autosomal Dominant ADD”). Sporadic AD cases were from the ACT study and the University of Washington (UW) AD Research Center (ADRC), and Autosomal Dominant ADD cases were from the UW ADRC and the Dominantly Inherited Alzheimer Network (DIAN). Excluded was any case with LBD or LATE-NC other than involving amygdala, territorial infarcts, more than 2 cerebral microinfarcts, or hippocampal sclerosis. Time from death to cryopreservation of tissue, postmortem interval (PMI), was <8 hr in all cases except for those in the Autosomal Dominant ADD group. Details of sample stratification for the four brain regions (SMTG, Hippocampus, IPL and Caudate) are provided in Tables 1 – 4 .
Ethics oversight
All study cohort participants were collected and provided informed consent under protocols approved by the Institutional Review Board (IRB) at University of Washington, Kaiser Permanente Washington, and Stanford University. The UW and Stanford Human Subjects Division deems the use of pre-existing de-identified samples exempt from full IRB review and, thus, treated this project as non-human subjects research.
Sample metadata, batch design and references
Each human brain region was divided into batches of 14 individual samples and 2 pooled references for a total of 16. The first batch of each region was also used to create a region-specific reference pool to be used as a “common reference” and/or single point calibrant, which was homogenized, aliquoted, frozen, and used to compare between batches within a brain region. Human cerebellum and occipital lobe tissue was homogenized, pooled, aliquoted and frozen to be used as a “batch reference” for comparison between batches and other brain regions. Batch design was randomly balanced based on group ratios. For example, batches from the SMTG brain region contained 5 “Sporadic ADD”, 4 “Autosomal Dominant ADD”, 2 “HCF/low ADNC”, and 3 “HCF/high ADNC” samples. Metadata for the samples from the SMTG, Hippocampus, IPL and Caudate brain regions is provided in Supplementary Tables 1–4 available on Panorama Public in the “Supplementary Data” subfolder 15 , 16 . For each region the metadata includes; sample batch, age, sex, post-mortem interval, APOE genotype, cognitive status, study of origin, and consensus Braak stage and CERAD score.
Sample homogenization and protein digestion
Two 25 μm frozen sections of brain tissue were resuspended in 120 μl of lysis buffer of 5% SDS, 50 mM triethylammonium bicarbonate (TEAB), 2 mM MgCl2, 1X HALT phosphatase and protease inhibitors, vortexed and briefly sonicated at setting 3 for 10 s with a Fisher sonic dismembrator model 100. A microtube was loaded with 30 μl of lysate and capped with a micropestle for homogenization with a Barocycler 2320EXT (Pressure Biosciences Inc.) for a total of 20 minutes at 35 °C with 30 cycles of 20 seconds at 45,000 psi followed by 10 seconds at atmospheric pressure. Protein concentration was measured with a BCA assay. Homogenate of 50 μg was added to a process control of 800 ng of yeast enolase protein (Sigma) which was then reduced with 20 mM DTT and alkylated with 40 mM IAA. Lysates were then prepared for S-trap column (Protifi) binding by the addition of 1.2% phosphoric acid and 350 μl of binding buffer (90% methanol, 100 mM TEAB). The acidified lysate was bound to column incrementally, followed by 3 wash steps with binding buffer to remove SDS and 3 wash steps with 50:50 methanol:chloroform to remove lipids and a final wash step with binding buffer. Trypsin (1:10) in 50 mM TEAB was then added to the S-trap column for digestion at 47 °C for one hour. Hydrophilic peptides were then eluted with 50 mM TEAB and hydrophobic peptides were eluted with a solution of 50% acetonitrile in 0.2% formic acid. Elutions were pooled, speed vacuumed and resuspended in 0.1% formic acid.
Injection of samples are one ug of total protein (16 ng of enolase process control) and 150 fmol of a heavy labeled Peptide Retention Time Calibrant (PRTC) mixture (Pierce). The PRTC is used as a peptide process control. Library pools are an equivalent amount of every sample (including references) in the batch. For example, a batch library pool consists of the 14 samples from the batch and two references. System suitability (QC) injections are 150 fmol of PRTC and BSA.
Liquid chromatography and mass spectrometry
One µg of each sample with 150 femtomole of PRTC was loaded onto a 30 cm fused silica picofrit (New Objective) 75 µm column and 3.5 cm 150 µm fused silica Kasil1 (PQ Corporation) frit trap loaded with 3 µm Reprosil-Pur C18 (Dr. Maisch) reverse-phase resin analyzed with a Thermo Easy-nLC 1200. The PRTC mixture is used to assess system suitability before and during analysis. Four of these system suitability runs are analyzed prior to any sample analysis and then after every six sample runs another system suitability run is analyzed. Buffer A was 0.1% formic acid in water and buffer B was 0.1% formic acid in 80% acetonitrile. The 40-minute system suitability gradient consists of a 0 to 16% B in 5 minutes, 16 to 35% B in 20 minutes, 35 to 75% B in 1 minute, 75 to 100% B in 5 minutes, followed by a wash of 9 minutes and a 30-minute column equilibration. The 110-minute sample LC gradient consists of a 2 to 7% for 1 minutes, 7 to 14% B in 35 minutes, 14 to 40% B in 55 minutes, 40 to 60% B in 5 minutes, 60 to 98% B in 5 minutes, followed by a 9 minute wash and a 30-minute column equilibration. Peptides were eluted from the column with a 50 °C heated source (CorSolutions) and electrosprayed into a Thermo Orbitrap Fusion Lumos Mass Spectrometer with the application of a distal 3 kV spray voltage. For the system suitability analysis, a cycle of one 120,000 resolution full-scan mass spectrum (350–2000 m/z ) followed by a data-independent MS/MS spectra on the loop count of 76 data-independent MS/MS spectra using an inclusion list at 15,000 resolution, AGC target of 4e5, 20 millisecond (ms) maximum injection time, 33% normalized collision energy with a 8 m/z isolation window. For the sample digest, first a chromatogram library of 6 independent injections is analyzed from a pool of all samples within a batch. For each injection a cycle of one 120,000 resolution full-scan mass spectrum with a mass range of 100 m/z (400–500 m/z , 500–600 m/z , 600–700 m/z , 700–800 m/z , 800–900 m/z , 900–1000 m/z ) followed by a data-independent MS/MS spectra on the loop count of 26 at 30,000 resolution, AGC target of 4e5, 60 ms maximum injection time, 33% normalized collision energy with a 4 m/z overlapping isolation window. The chromatogram library data is used to quantify proteins from individual sample runs. These individual runs consist of a cycle of one 120,000 resolution full-scan mass spectrum with a mass range of 350–2000 m/z , AGC target of 4e5, 100 ms maximum injection time followed by a data-independent MS/MS spectra on the loop count of 76 at 15,000 resolution, AGC target of 4e5, 20 ms maximum injection time, 33% normalized collision energy with an overlapping 8 m/z isolation window. Application of the mass spectrometer and LC solvent gradients are controlled by the ThermoFisher Xcalibur (version 3.1.2412.24) data system. Mass spectrometry run order for all samples is provided in Supplementary Tables 5–8 available on Panorama Public.
Peptide detection and quantitative signal processing
Thermo RAW files were converted to mzML format using Proteowizard (version 3.0.20064) using vendor peak picking and demultiplexing with the settings of “overlap_only” and Mass Error = 10.0 ppm 5 . On column chromatogram libraries were created using the data from the six gas phase fractionated “narrow window” DIA runs of the pooled reference as described previously 17 . These narrow windows were analyzed using EncyclopeDIA (version 1.4.10) with the default settings (10 ppm tolerances, trypsin digestion, HCD b- and y-ions) of a Prosit predicted spectra library based the Uniprot human canonical FASTA (January 2021). The results from this analysis from each brain region were saved as a “Chromatogram Library” in EncyclopeDIA’s eLib format where the predicted intensities and iRT of the Prosit library were replaced with the empirically measured intensities and RT from the gas phase fractionated LC-MS/MS data. The “wide window” DIA runs were analyzed using EncyclopeDIA (version 1.4.10) requiring a minimum of 3 quantitative ions and filtering peptides with q-value ≤ 0.01 using Percolator 3.01. After analyzing each file individually, EncyclopeDIA was used to generate a “Quant Report” which stores all the detected peptides, integration boundaries, quantitative transitions, and statistical metrics from all runs in an eLib format. The Quant Report eLib library is imported into Skyline (daily version 22.2.1.278) with the human uniprot FASTA as the background proteome to map peptides to proteins, perform peak integration, manual evaluation, and report generation. A csv file of peptide level total area fragments (TAFs) for each replicate was exported from Skyline using the custom reporting capabilities of the document grid 18 .
Quantitative data post-processing, normalization, and batch correction
Despite precautions taken to ensure equivalent sample preparation, handling and acquisition, additional post-processing was performed to normalize, and batch adjust the quantitative data to remove residual technical noise. Modeling the proportional changes of peptide/protein group intensities, log 2 transformation is applied followed by a Median Deviation (MD) normalization to the peptide total area fragment values (level 2 data) across instrument runs within a brain region (Eq. 1 ) under the assumption that median total area fragment values should be equal sans batch effect from known and unknown sources of variability.
MD Normalization, by calculating the deviation from the median of the sample total area fragment, should neither remove scale information nor de-weigh outlier signals that may be of biological relevance 19 . Here, the MD normalized peptide F of each sample is given by the following. The peak areas ( A i ) for each peptide i are first log 2 transformed and then normalized by equalizing the median peak areas across all samples using the equation:
The effectiveness and validity of the normalization approach is then assessed by evaluating the comparability of the peptide abundance distribution across samples (Fig. 2b ), and by the reproducibility of those peptide abundances across replicate samples (Fig. 3a ). Peptide abundances are then adjusted for batch effect by fitting a linear model and “regressing” out the factors with known unwanted sources of variation to return a matrix of residuals. The detection of the presence of batch effect pre- and post-adjustment is assessed by exploring the data variance structure through Principal Variance Component Analysis (PVCA) ( https://bioconductor.org/packages/release/bioc/html/pvca.html ) (Supplementary Figure 4 available on Panorama Public) and Principal component Analysis (PCA) using projections onto the first three principal components. The normalization and batch adjusted peptide abundances are available as the level 3 A data file. Using DIA, all observable peptides in one sample will be sampled in all of the other biological replicates 12 , 13 , 20 . Due to the comprehensive sampling nature of DIA we can extract information for the same transitions across all samples in an experiment. The resulting zeros in our peptide abundance data therefore represent signals below our limit of detection and are not treated as missing data. After protein group inference, protein abundances are batch corrected using the same method as the peptide data.
Protein grouping and inference
The processing and ‘roll-up’ of DIA data borrows from the established strategies adopted in the DDA field in which the quantification of peptides and their corresponding protein groups is inferred through the modification of IDPicker algorithm 21 . In summary, to quantify the peptide/protein groups, a bipartite graph of peptide-protein interactions is constructed to generate groupings through the parsimony reduction of the graph as it is implemented in MSDaPl. Then, the peptide abundances at the nodes are summed to estimate the abundance of the peptide groups and proteins that match the same set of peptide groups are merged into a single node in the graph, forming an indistinguishable protein group 22 .
Data Records
The Skyline documents, raw files for quality control and DIA data are available at Panorama Public. ProteomeXchange ID: PXD034525. https://doi.org/10.6069/wefm-vv52 . Access URL: https://panoramaweb.org/ADBrainCleanDiagDIA.url 16 .
DIA data is available in 5 different categories based on the level of post-processing (Fig. 1e ) for each brain region. Level 0 represents the raw data in two different formats - the raw format is directly from the Thermo mass spectrometer and the mzML format is the demultiplexed version of the raw data (Proteowizard version 3.0.20064). Level 1 describes the zipped Skyline document grouped by batch. Level 2 is a csv file grouped by batch of the Skyline output with the integrated peak area for each peptide (row) in each replicate (column). Level 3 A is a csv file of the normalized peptide abundance across all batches. Level 3B is a csv file of the normalized protein abundance across all batches.
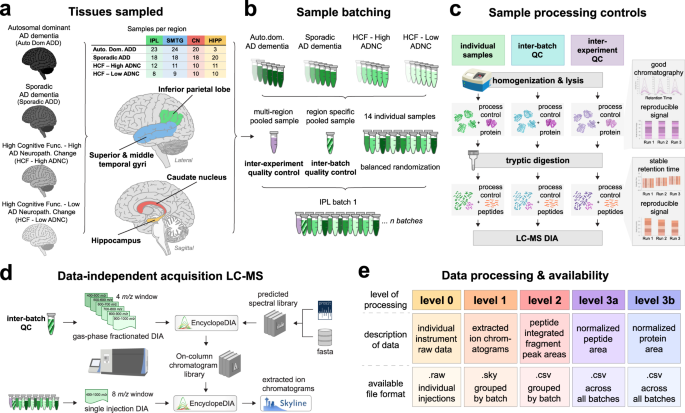
Experimental scheme for the collection of the proteomics data using data independent acquisition-mass spectrometry. ( a ) Brain tissue sections from 4 regions were analyzed for all groups. ( b ) Samples were prepared and analyzed in batches of 16, with 14 individual samples per batch selected by balanced randomization. Each batch contained an inter-experiment quality control sample generated from pooling portions of several individual samples from across all 4 brain regions sampled. Each batch also contained an inter-batch quality control sample generated from pooling portions of individual samples within that brain region. ( c ) In addition to quality control samples, both protein and peptide sample processing controls were included in all samples to track system suitability. ( d ) For each batch an on-column data independent acquisition chromatogram library is generated from overlapping, narrow window gas-phase fractionation of an inter-batch QC. Individual samples are acquired by a single injection wider window data independent acquisition method. Peptide detection and scoring is performed using EncyclopeDIA and extracted ion chromatograms integrated with Skyline. ( e ) The proteomics data is publicly available on the Panorama web server in 5 different states, each corresponding to the level of post-processing.
Quality control Skyline documents, peptide QC plots and instrument raw files for system suitability runs are provided by brain region. The Skyline documents and peptide QC plots for enolase and PRTC process controls are provided by brain region. The instrument raw files for process controls are the same as DIA sample raw files by brain region. An interactive dashboard is available for the SMTG and Hippocampus data.
Technical Validation
Balanced and controlled experiment design.
We have designed our experiment to perform quantitative, peptide-centric proteomics using brain tissue from four different brain regions selected specifically because they represent distinct anatomical regions with varying pathological involvement by AD (Fig. 1 ). The experimental design was intended to compare individual samples from the four different categorical disease groups within each brain region. Samples were prepared in batches of 16 samples which consisted of 14 brain tissue samples and two external control samples. The batch size was determined by the number of samples, 16, that could be prepared within a Barocycler (Pressure Biosciences, Inc.). For each batch, the samples were randomized in a balanced block design (Supplementary Table 5 available on Panorama Public).
Within each batch we included both internal and external controls. Internal controls were added to each sample to provide a QC check of the sample preparation and LC-MS data collection process. These “Process Controls” consisted of the addition of yeast enolase protein after lysis and prior to digestion and the Pierce Retention Time Calibration (PRTC; 15 synthetic stable isotope labeled peptides) peptide mixture following digestion. The “Protein Internal Control” was used to assess the protein digest and peptide recovery and the “Peptide Internal Control” was used to distinguish between sample preparation and measurement issues post-digestion.
The two external controls were different brain lysates that were prepared, measured, and analyzed with the rest of the samples in the batch. One of the controls was a brain region specific pool used to assess between batch quality control. This inter-batch quality control is composed of a randomized balanced pooled sample set for each respective brain region. For example, the inter-batch quality control “TRPR” is composed of 3 HCF/high ADNC samples, 3 HCF/low ADNC samples, 3 AutoDom ADD samples and 5 Sporadic ADD samples from the SMTG. The same inter-batch quality control is run in every batch of the experiment from the SMTG and was used to assess data quality post-normalization. The second external control was an inter-brain region quality control (“HAD” samples) and composed of a homogenate of a mix of cerebellum and occipital lobe tissue which we had ample material available to use throughout all our brain tissue experiments. The cerebellum and occipital lobe external control is distinct from the rest of the brain tissue regions in the experiment, but this should not hinder the interpretation of the experimental results, as this control is only monitoring the reproducibility of our entire system. The same pool of inter-brain external control was prepared and run in every batch across all brain regions for the entire experiment.
We can determine when our sample preparation and system is not functioning as expected with a combination of system suitability checks, inter-batch quality controls, inter-experiment quality controls and process controls (Fig. 1b ). Our system suitability consists of a mixture of a BSA tryptic digest and PRTC prior to sample analysis and throughout sample collection at a frequency of once every six to eight samples.
Run level and experiment level peptide and protein detections
For each sample in each brain region tryptic peptides can be detected at a 1% FDR cut-off. IPL samples ranged from 37840–73168, SMTG ranged from 51582–69590, hippocampus from 32995–59853, and caudate nucleus ranged from 31426–58105 (Table 5 ). To integrate data across all individual samples within each brain region we control with an experiment level error rate. This leads to the same peptides quantified in all samples within a brain region; 48271 in IPL, 40346 in SMTG, 31863 in hippocampus, and 26135 in caudate nucleus. These peptides map to 6497 quantified proteins in IPL, 5851 in SMTG, 5117 in hippocampus, and 4636 in caudate nucleus (Table 5 ). The distribution of peptide abundances is aligned with median normalization, as demonstrated with the SMTG data (Fig. 2b ) as well as all brain regions (Supplementary Figures 1 and 2 available on Panorama Public).
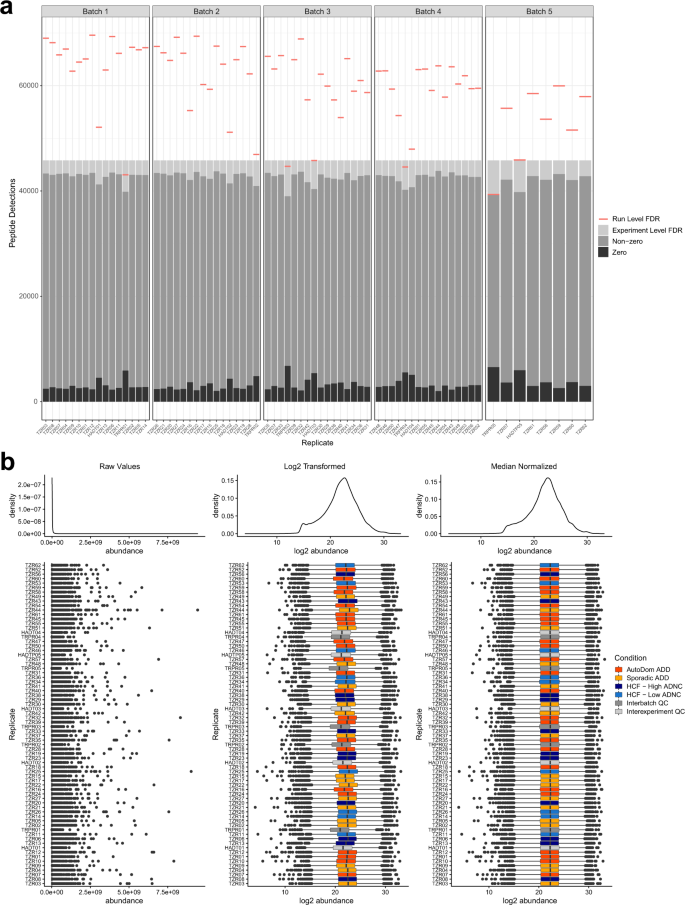
Peptides detected in individual runs and across the entire dataset for SMTG. ( a ) The number of peptides detected for each sample in the SMTG brain region experiment, with those detected in the sample at a 1% FDR indicated by the red line, and those detected across all samples at an 1% experiment level FDR indicated in gray. Although zeros exist in this remaining data, these represent a lack of signal above background. ( b ) Kernel density plots (above) and box plots (below) for each individual sample show the distribution of peptide abundances before and after log2 transformation and then median normalization. Sample groups are highlighted with different colors in the log2 transformation and median normalization box plots. Figure is generated with level 2 data.
Inter-batch precision and reproducibility
The inclusion of inter-batch quality control samples allows us to assess the impact of normalization and batch correction on peptide and protein quantitative reproducibility. For example, the SMTG experiment was split into 5 batches for processing and acquisition. Using the inter-batch control replicate samples, the coefficient of variation can be calculated for all peptides quantified in the SMTG. The distribution of peptide coefficient of variation improves with normalization and batch correction, with the mean decreasing about 8.2%. Likewise, the protein coefficient of variation also improves following batch correction, with the mean decreasing by about 1.25% (Fig. 3 ). Peptide and protein quantities are highly correlated across inter-batch replicates. Inter-batch control replicate samples in SMTG have peptide Pearson correlation coefficients ranging from 0.867 to 0.950, and protein correlations ranging from 0.894 to 0.960 (Fig. 4 ).
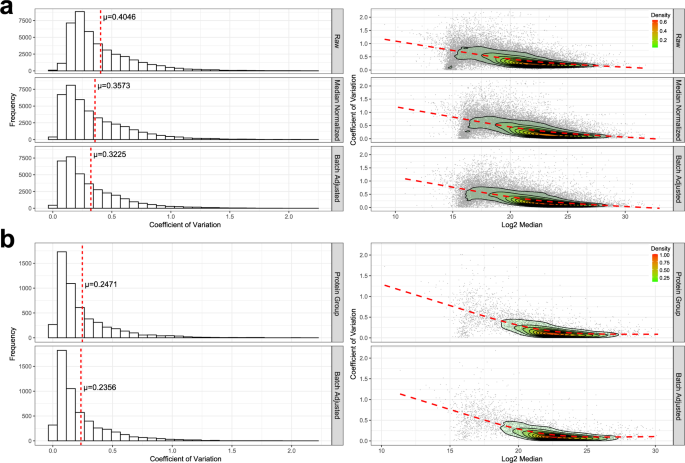
Effect of normalization and batch correction on the inter-batch variance for SMTG. ( a ) The effect of median normalization and batch correction on the inter-batch peptide coefficient of variance. Mean coefficient of variation (μ) is indicated by the red line. Figure 3a is generated from level 2 to 3a data. ( b ) Effect of batch correction on the inter-batch protein coefficient of variance. The relationship between coefficient of variation and the log2 median abundance is visualized with a loess fit of a contoured density plot (red line). Figure 3b is generated from level 2 to 3b data.
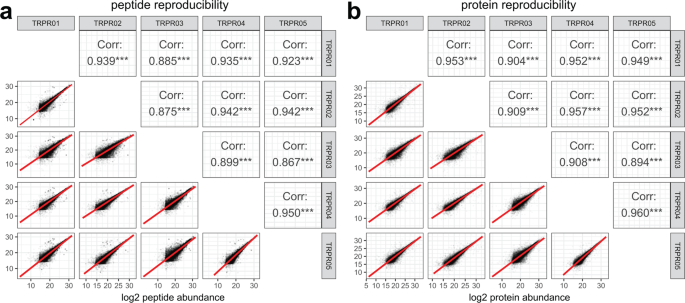
Correlation of the quantitative results from five different sample preparation and analysis batches following normalization and batch correction for SMTG. ( a ) The log2 peptide abundances measured in the inter-batch quality control samples across each of the 5 SMTG experimental batches, with the Pearson correlation coefficient. Figure 4a is generated from level 3a data. ( b ) The log2 protein group abundances from the inter-batch quality control samples in the 5 SMTG batches samples, and their Pearson correlation coefficient. Significance is indicated with *** being p < 0.001. Figure 4b is generated from level 3b data.
Expected biological differences
Of the peptides quantified, we detect several proteins known to be involved in AD. In the SMTG data we quantify peptides mapping to 250 proteins found in AD-related pathways (Supplemental Fig. 5 available on Panorama Public). Preliminary assessment of peptide and protein data show we can distinguish between sample groups (Supplemental Fig. 3 available on Panorama Public). Differential abundance analysis between HCF/low ADNC and autosomal dominant ADD in SMTG captures known biology (Fig. 5 ). Protein groups found to be significantly different between the groups include previously documented increases in Amyloid precursor protein (APP/A4), Apolipoprotein E (APOE), SPARC-related modular calcium-binding protein 1 (SMOC1), midkine (MK/MDK) and netrin-1 (NET1/NTN1) 23 . We detect and quantify two peptides mapping to the n- and c-terminal sides of the alpha-secretase cleavage site in amyloid-β sequence (Fig. 6 ). Both peptides have an expected difference in abundance across the sample groups in all four brain regions, with autosomal dominant ADD having the highest distribution, followed by sporadic ADD, HCF/high ADNC, and HCF/low ADNC. Peptides mapping to microtubule-associated protein tau (MAPT) also have some expected differences between the experimental groups. In the SMTG brain region the seven quantified peptides spanning the microtubule binding region of MAPT (residues 243–368) are increased in autosomal dominant ADD, followed by sporadic AD, compared to both HCF groups. This trend has been observed previously and leads to the aggregated protein abundance to be differential in the same manner as the microtubule binding region peptides 24 .
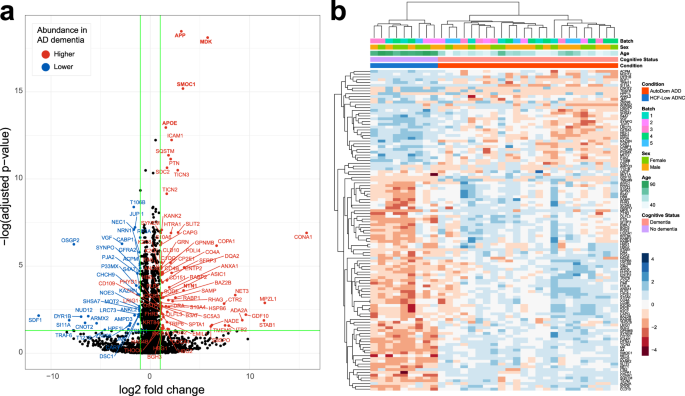
Summary data of quantitative changes observed for SMTG. ( a ) Volcano plot showing proteins that are statistically different between the autosomal dominant Alzheimer’s disease (AD) dementia (ADD) and the high cognitive function (HCF)/low AD neuropathologic change (ADNC) SMTG using cut-off of log2 fold-change > ± 0.5 and FDR adjusted p-value < 0.05. ( b ) Heatmap showing complete linkage hierarchical clustering of 33 SMTG samples using the 115 proteins with significant differences between ADD and the HCF-low ADNC samples. Figure 5 is generated from level 3b data.
Usage Notes
We provide quantitative data both for tryptic peptides directly and for protein groups derived from peptide quantities. Based on extensive existing knowledge of AD, we know that modified forms of the APP or amyloid-β and Tau proteins are important in disease progression. Historically mass spectrometry proteomics data has aggregated multiple tryptic peptides per protein coding sequence to arrive at a singular protein level value. This would result in a loss of important information regarding the status of the individual peptides 7 . By collecting these samples by DIA we can reproducibly quantify individual tryptic peptides across our entire sample set. With this peptide data we observe differentially abundant peptides within a protein coding gene. If aggregated to a singular value this important signal would be lost.
Use Case 1. Sporadic and autosomal dominant Alzheimer disease dementia differential peptide and protein abundance
This mass spectrometry data has typically been reported as relative protein group abundance measures, enabling differential abundance testing of protein groups between disease states (Fig. 6 ). In SMTG there are proteins with statistically different abundance between healthy controls and autosomal dominant ADD. This type of analysis can be extended to all brain regions. A large portion of research investigating the molecular and pathological basis of AD has been generated using model systems informed by genetic causes. Studying individuals with sporadic ADD can be challenging due to the presence of comorbidities. Here we present data generated from both autosomal dominant ADD with minimal comorbidities and sporadic ADD with minimal comorbidities. These data can be used to better understand biological differences or similarities in these two types of ADD.
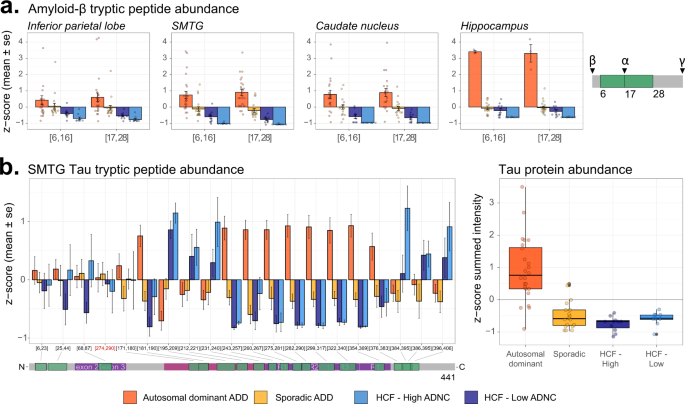
Amyloid-β and tau peptide abundances recapitulate known molecular changes for the SMTG. ( a ) Two tryptic peptides mapping to amyloid-β (amino acid residues 6–16: HDSGYEVHHQK, 17–28: LVFFAEDVGSNK) are z-scored across all individuals and plotted as the mean and standard error for each sample group. ( b ) Abundances for tryptic peptides mapping to tau are z-scored across all individuals and plotted as the mean and standard error for each sample group. While there are some common signatures within protein domains, these signatures are lost if aggregated to a protein level. Tryptic peptides are labeled based on their first and last amino acid residues. Figure 6 is generated from level 3a data.
Use Case 2. Differential peptide and protein abundance with neuropathologic markers and dementia
The HCF/high ADNC samples have classic histopathologic features of AD - amyloid-β plaques and neurofibrillary tangles - at levels overlapping with individuals who have Sporadic ADD. This enables analyses to measure the differences in protein pathology between individuals who have developed dementia and those who have not developed dementia despite similar levels of high amyloid-β plaques and Tau tangles.
Use Case 3. Mass spectrometry data reuse and reanalysis
Beyond the analyses presented here, the storage of this data is done in a way that facilitates use of this data for informing subsequent mass spectrometry assay development. All data from this project is available on the Panorama server-based data repository application for targeted mass spectrometry assays 15 , 16 . Thus, all extracted ion chromatogram information from every sample across all brain regions is available in an interactive Skyline document format, and readily available for download and reuse. Information about fragment ions and chromatography is important for the development of targeted assays 25 , 26 , making this data valuable to the wider community.
Using DIA mass spectrometry methods allows for the data to be easily reanalyzed. This could be used to search for post-translational modifications or sequence variants not searched for in our current analysis. Additionally, this data can be reanalyzed to look at other unique features, such as isomerized peptides. The feasibility of this was recently shown by Hubbard et al . through reanalysis of a subset of this dataset 27 . Peptide-centric reanalysis is possible due to the comprehensive sampling by data-independent acquisition of a preset range across all samples.
Code availability
The MSConvert installer and documentation is available from https://proteowizard.sourceforge.io/ .
EncyclopeDIA is available at https://bitbucket.org/searleb/encyclopedia/ . The Skyline-daily installer and documentation are available from https://skyline.ms/skyline.url . The source code for both the MSConvert and Skyline projects are available as part of the Proteowizard project https://github.com/ProteoWizard/pwiz .
All code used for the analysis of the data matrix including data QC, normalization, pre-processing, visualization, and figure generation is available online at https://github.com/uw-maccosslab/ADBrainCleanDiagDIA .
Vos, T. et al . Global, regional, and national incidence, prevalence, and years lived with disability for 301 acute and chronic diseases and injuries in 188 countries, 1990–2013: a systematic analysis for the Global Burden of Disease Study 2013. The Lancet 386 , 743–800 (2015).
Article Google Scholar
Scheltens, P. et al . Alzheimer’s disease. The Lancet 397 , 1577–1590 (2021).
Article CAS Google Scholar
Hyman, B. T. et al . National Institute on Aging-Alzheimer’s Association guidelines for the neuropathologic assessment of Alzheimer’s disease. Alzheimers Dement 8 , 1–13 (2012).
Article PubMed PubMed Central Google Scholar
Montine, T. J. et al . Recommendations of the Alzheimer’s disease-related dementias conference. Neurology 83 , 851–860 (2014).
Amodei, D. et al . Improving Precursor Selectivity in Data-Independent Acquisition Using Overlapping Windows. J Am Soc Mass Spectrom 30 , 669–684 (2019).
Article CAS PubMed PubMed Central Google Scholar
Brenes, A., Hukelmann, J., Bensaddek, D. & Lamond, A. I. Multibatch TMT Reveals False Positives, Batch Effects and Missing Values. Mol Cell Proteomics 18 , 1967–1980 (2019).
Plubell, D. L. et al . Putting Humpty Dumpty Back Together Again: What Does Protein Quantification Mean in Bottom-Up Proteomics? J Proteome Res 21 , 891–898 (2022).
Fernández-Costa, C. et al . Impact of the Identification Strategy on the Reproducibility of the DDA and DIA Results. J Proteome Res 19 , 3153–3161 (2020).
Pino, L. K. et al . The Skyline ecosystem: Informatics for quantitative mass spectrometry proteomics. Mass Spectrom Rev 39 , 229–244 (2020).
Article ADS CAS PubMed Google Scholar
Rosenberger, G. et al . Statistical control of peptide and protein error rates in large-scale targeted data-independent acquisition analyses. Nat Methods 14 , 921–927 (2017).
Venable, J. D., Dong, M. Q., Wohlschlegel, J., Dillin, A. & Yates, J. R. Automated approach for quantitative analysis of complex peptide mixtures from tandem mass spectra. Nat Methods 1 , 39–45 (2004).
Article CAS PubMed Google Scholar
Gillet, L. C. et al . Targeted data extraction of the MS/MS spectra generated by data-independent acquisition: a new concept for consistent and accurate proteome analysis. Mol Cell Proteomics 11 , O111.016717 (2012).
Ting, Y. S. et al . PECAN: library-free peptide detection for data-independent acquisition tandem mass spectrometry data. Nat Methods 14 , 903–908 (2017).
Searle, B. C. et al . Chromatogram libraries improve peptide detection and quantification by data independent acquisition mass spectrometry. Nat Commun 9 , 10.1038 (2018).
Google Scholar
Sharma, V. et al . Panorama: a targeted proteomics knowledge base. J Proteome Res 13 , 4205–4210 (2014).
Merrihew, G. E. et al . A Peptide-Centric Quantitative Proteomics Dataset for the Phenotypic Assessment of Alzheimer’s Disease. Panorama Public https://doi.org/10.6069/wefm-vv52 (2022).
Pino, L. K., Just, S. C., MacCoss, M. J. & Searle, B. C. Acquiring and Analyzing Data Independent Acquisition Proteomics Experiments without Spectrum Libraries. Mol Cell Proteomics 19 , 1088–1103 (2020).
MacLean, B. et al . Skyline: an open source document editor for creating and analyzing targeted proteomics experiments. Bioinformatics 26 , 966–968 (2010).
Ma, Z.-Q. et al . IDPicker 2.0: Improved protein assembly with high discrimination peptide identification filtering. J Proteome Res 8 , 3872–3881 (2009).
Egertson, J. D. et al . Multiplexed MS/MS for improved data-independent acquisition. Nat Methods 10 , 744–746 (2013).
Sharma, V., Eng, J. K., Maccoss, M. J. & Riffle, M. A mass spectrometry proteomics data management platform. Mol Cell Proteomics 11 , 824–831 (2012).
Webb-Robertson, B.-J. M., Matzke, M. M., Jacobs, J. M., Pounds, J. G. & Waters, K. M. A statistical selection strategy for normalization procedures in LC-MS proteomics experiments through dataset-dependent ranking of normalization scaling factors. Proteomics 11 , 4736–4741 (2011).
Johnson, E. C. B. et al . Large-scale deep multi-layer analysis of Alzheimer’s disease brain reveals strong proteomic disease-related changes not observed at the RNA level. Nature Neuroscience 25 , 213–225 (2022).
Wesseling, H. et al . Tau PTM Profiles Identify Patient Heterogeneity and Stages of Alzheimer’s Disease. Cell 183 , 1699–1713.e13 (2020).
Bereman, M. S., MacLean, B., Tomazela, D. M., Liebler, D. C. & MacCoss, M. J. The development of selected reaction monitoring methods for targeted proteomics via empirical refinement. Proteomics 12 , 1134–1141 (2012).
Searle, B. C., Egertson, J. D., Bollinger, J. G., Stergachis, A. B. & MacCoss, M. J. Using Data Independent Acquisition (DIA) to Model High-responding Peptides for Targeted Proteomics Experiments. Mol Cell Proteomics 14 , 2331–2340 (2015).
Hubbard, E. E. et al . Does Data-Independent Acquisition Data Contain Hidden Gems? A Case Study Related to Alzheimer’s Disease. J Proteome Res 21 , 118–131 (2022).
Download references
Acknowledgements
Support for this research was provided by the National Institute of Aging (RF1 AG053959, U19 AG065156, F31 AG069420, R01 AG075802). The collection of tissue samples were supported by the UW Alzheimer’s Disease Research Center (P30 AG066509), the Adult Changes in Thought study (U19 AG066567), the Nancy and Buster Alvord Endowment (C.D.K.), the Massachusetts Alzheimer Disease Research Center (P30 AG062421), The Dominantly Inherited Alzheimer Network (U19 AG032438, UF1AG032438), the German Center for Neurodegenerative Diseases (DZNE), Raul Carrea Institute for Neurological Research (FLENI), the Research and Development Grants for Dementia from Japan Agency for Medical Research and Development, AMED, and the Korea Health Technology R&D Project through the Korea Health Industry Development Institute (KHIDI). This manuscript has been reviewed by DIAN Study investigators for scientific content and consistency of data interpretation with previous DIAN Study publications. We acknowledge the altruism of the participants and their families and contributions of the research and support staff at each of the participating sites for their contributions to this study.
Author information
Authors and affiliations.
Department of Genome Sciences, University of Washington, Seattle, Washington, 98195, USA
Gennifer E. Merrihew, Jea Park, Deanna Plubell & Michael J. MacCoss
Department of Biomedical Informatics, The Ohio State University, Columbus, Ohio, 43210, USA
Brian C. Searle
Department of Laboratory Medicine and Pathology, University of Washington, Seattle, Washington, 98195, USA
C. Dirk Keene
Department of Medicine, University of Washington, Seattle, Washington, 98195, USA
Eric B. Larson
Department of Neurology, Washington University School of Medicine, 660 South Euclid Avenue, Box 8111, St. Louis, Missouri, 63110, USA
Randall Bateman
Department of Pathology, Washington University School of Medicine, 660 South Euclid Avenue, Box 8111, St. Louis, Missouri, 63110, USA
Richard J. Perrin
Massachusetts General Hospital, Department of Neurology, Harvard Medical School, 15 Parkman St, Suite 835, Boston, Massachusetts, 02114, USA
Jasmeer P. Chhatwal
Department of Neurology, Indiana University School of Medicine, Indianapolis, Indiana, 46202, USA
Martin R. Farlow
Department of Anatomical Pathology, Alfred Health, Melbourne, VIC, 3004, Australia
Catriona A. McLean
Department of Pathology and Laboratory Medicine, Indiana University School of Medicine, Indianapolis, Indiana, 46202, USA
Bernardino Ghetti & Kathy L. Newell
C.S. Kubik Laboratory for Neuropathology, and Massachusetts Alzheimer Disease Research Center, Massachusetts General Hospital, Boston, Massachusetts, 02114, USA
Matthew P. Frosch
Department of Pathology, Stanford University, Stanford, CA, 94305, USA
- Thomas J. Montine
You can also search for this author in PubMed Google Scholar
Contributions
G.E.M., J. P. and D.L.P. share equal first authorship. G.E.M., M.J.M. and T.J.M. designed the experiment. G.E.M. prepared the brain tissue, ran the mass spectrometer, performed the bioinformatic signal processing, and organized the data repository. J.P. performed data quality control, normalization, batch correction and statistics. J.P. and D.P. produced the figures and interpreted the results. B.C.S. designed mass spectrometry methods and software. C.D.K., E.B.L., R.B., R.J.P., J.P.C., M.R.F., C.A.M., B.G., K.L.N. and M.P.F. provided specimens. G.E.M., J.P., D.L.P., T.J.M. and M.J.M. wrote the paper. All authors reviewed the manuscript.
Corresponding authors
Correspondence to Thomas J. Montine or Michael J. MacCoss .

Ethics declarations
Competing interests.
G.E.M., J.P., D.L.P., and M.J.M. declare the following competing financial interest(s): The MacCoss Lab at the University of Washington has a sponsored research agreement with Thermo Fisher Scientific, the manufacturer of the instrumentation used in this research. M.J.M. is a paid consultant for Thermo Fisher Scientific. The remaining authors declare no competing interests.
Additional information
Publisher’s note Springer Nature remains neutral with regard to jurisdictional claims in published maps and institutional affiliations.
Rights and permissions
Open Access This article is licensed under a Creative Commons Attribution 4.0 International License, which permits use, sharing, adaptation, distribution and reproduction in any medium or format, as long as you give appropriate credit to the original author(s) and the source, provide a link to the Creative Commons license, and indicate if changes were made. The images or other third party material in this article are included in the article’s Creative Commons license, unless indicated otherwise in a credit line to the material. If material is not included in the article’s Creative Commons license and your intended use is not permitted by statutory regulation or exceeds the permitted use, you will need to obtain permission directly from the copyright holder. To view a copy of this license, visit http://creativecommons.org/licenses/by/4.0/ .
Reprints and permissions
About this article
Cite this article.
Merrihew, G.E., Park, J., Plubell, D. et al. A peptide-centric quantitative proteomics dataset for the phenotypic assessment of Alzheimer’s disease. Sci Data 10 , 206 (2023). https://doi.org/10.1038/s41597-023-02057-7
Download citation
Received : 31 October 2022
Accepted : 08 March 2023
Published : 14 April 2023
DOI : https://doi.org/10.1038/s41597-023-02057-7
Share this article
Anyone you share the following link with will be able to read this content:
Sorry, a shareable link is not currently available for this article.
Provided by the Springer Nature SharedIt content-sharing initiative
This article is cited by
Brain proteomic analysis implicates actin filament processes and injury response in resilience to alzheimer’s disease.
- Gennifer E. Merrihew
Nature Communications (2023)
Whole genome deconvolution unveils Alzheimer’s resilient epigenetic signature
- Eloise Berson
- Anjali Sreenivas
Quick links
- Explore articles by subject
- Guide to authors
- Editorial policies
Sign up for the Nature Briefing: Translational Research newsletter — top stories in biotechnology, drug discovery and pharma.

Click through the PLOS taxonomy to find articles in your field.
For more information about PLOS Subject Areas, click here .
Loading metrics
Open Access
Peer-reviewed
Research Article
Evaluation of knowledge and attitudes regarding Alzheimer’s disease and related dementia among medical students in Palestine: A cross-sectional study
Roles Conceptualization, Data curation, Formal analysis, Investigation, Methodology, Project administration, Software, Supervision, Validation, Visualization, Writing – original draft, Writing – review & editing
* E-mail: [email protected]
Affiliation Faculty of Medicine and Health Sciences, Department of Biomedical Sciences, An-Najah National University, Nablus, Palestine

Affiliation Faculty of Medicine and Health Sciences, Department of Medicine, An-Najah National University, Nablus, Palestine
Affiliation Intern Medical Doctor, Rafeedia Surgical Hospital, Nablus, Palestine
Affiliation Faculty of Medicine, Research and Teaching Assistant, Arab American University, Jenin, Palestine
Affiliation Faculty of Medicine and Health Sciences, Department of Allied and Applied Medical Sciences, An-Najah National University, Nablus, Palestine
- Mohammad Abuawad,
- Ahmad Rjoub,
- Yazan Dumaidi,
- Motaz Daraghma,
- Mustafa Ghanim,
- Maha Rabayaa,
- Johnny Amer
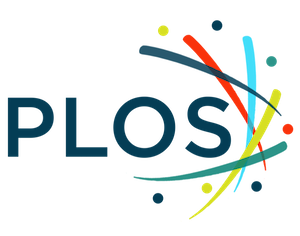
- Published: May 17, 2024
- https://doi.org/10.1371/journal.pone.0304012
- Peer Review
- Reader Comments
Introduction
Dementia, a major global health concern, is an acquired disorder that causes a progressive decline in cognitive abilities, affecting learning and memory, language, executive function, complex attention, perceptual-motor skills, and social cognition. Our study aims to evaluate the knowledge and attitudes regarding dementia and Alzheimer’s disease among medical students.
This cross-sectional study was conducted among 393 medical students in Palestine from August 2023 to November 2023. The assessment of knowledge and attitude toward dementia was measured using the Alzheimer’s Disease Knowledge Scale (ADKS) and Dementia Attitude Scale (DAS). The data were analyzed using SPSS version 26, and the Mann-Whitney U-test and the Kruskal-Wallis test were used to compare the mean between the groups with a 5% significance level.
The overall mean score of the student’s knowledge of dementia measured by the ADKS was 18.91 (±3.32 SD) out of 30. The mean score of the student’s attitude toward dementia measured by the DAS was 91.68 (±3.32 SD). Clinical students had higher ADKS scores than pre-clinical students (p-value < 0.001). No significant differences in the knowledge and attitudes toward dementia were found between males and females. The medical students’ knowledge and attitude scores were positively correlated (ρ = 0.227, p-value <0.001).
Palestinian medical student’s knowledge about Alzheimer’s disease and dementia is insufficient, with students in the clinical phase showing better understanding than pre-clinical students. The findings highlight a necessity for enhancing the dementia curriculum and conducting further studies to evaluate training’s impact on students’ knowledge and attitudes.
Citation: Abuawad M, Rjoub A, Dumaidi Y, Daraghma M, Ghanim M, Rabayaa M, et al. (2024) Evaluation of knowledge and attitudes regarding Alzheimer’s disease and related dementia among medical students in Palestine: A cross-sectional study. PLoS ONE 19(5): e0304012. https://doi.org/10.1371/journal.pone.0304012
Editor: Shahabedin Rahmatizadeh, Shahid Beheshti University of Medical Sciences, ISLAMIC REPUBLIC OF IRAN
Received: January 30, 2024; Accepted: May 3, 2024; Published: May 17, 2024
Copyright: © 2024 Abuawad et al. This is an open access article distributed under the terms of the Creative Commons Attribution License , which permits unrestricted use, distribution, and reproduction in any medium, provided the original author and source are credited.
Data Availability: All relevant data are within the manuscript and its Supporting Information files.
Funding: The author(s) received no specific funding for this work.
Competing interests: The authors have declared that no competing interests exist.
1. Introduction
Dementia is an acquired disorder that causes cognitive abilities to decline over time, affecting learning and memory, language, executive function, complex attention, perceptual-motor skills, and social cognition [ 1 , 2 ]. Dementia, a major global health concern, currently affects over 55 million people worldwide [ 3 ], with low- and middle-income countries accounting for more than 60% of cases. Each year, nearly 10 million new cases are documented [ 4 ]. The varied causes of dementia stem from various diseases and injuries affecting the brain, with Alzheimer’s disease being the most common, accounting for 60–70% of cases [ 5 ]. According to the World Health Organization, dementia is currently the seventh leading cause of death and is a significant contributor to disability and dependency in the elderly on a global scale [ 6 ]. The global economic burden of dementia reached a staggering 1.3 trillion US dollars in 2019 [ 7 ].
Dementia is clinically defined as the loss of cognitive functioning (thinking, remembering, learning, and reasoning) and behavioral abilities are lost to the point where they interfere with a person’s quality of life and activities. Although memory loss is common, it is not the only sign of dementia [ 8 ]. The concept of dementia is still perceived as a normal aging process by patients, caregivers, and even medical practitioners. As per a study in Australia, conducted on adults aged 40–65 years old, it has been noted that 50% of the participants are not able to have a meaningful conversation with patients with dementia [ 9 ]. Another study conducted in Qatar demonstrated an average score of 5.3 out of 7 (approximately 75%) when filling the seven measures of knowledge regarding AD and dementia by healthcare professionals showed moderate knowledge of Alzheimer’s disease (AD) and dementia. Physicians had the most knowledge, followed by students, educators, researchers, nurses, and others [ 10 ].
In the State of Palestine, the prevalence of dementia among individuals aged 50 and older is 2.08%, rising to 4.01% in those aged 60 and older [ 11 ]. This highlights the significance of dementia as an essential clinical entity that healthcare practitioners face in their practice. It emphasizes the importance of evidence-based education, which is supported by data on medical education outcomes. According to our review of the literature, such data is lacking, emphasizing the importance of conducting this study. In this study, we aim to evaluate the awareness and attitude of medical students in the State of Palestine regarding Alzheimer’s disease and dementia.
2.1. Setting and study design
The study took place among the Palestinian Medical Students enrolled in the “Medical Doctor” program in the seven medical schools in five provinces in Palestine: An-Najah National University, Al-Azhar University-Gaza, Palestine Polytechnic University, Arab American University, Islamic University of Gaza, Al-Quds University, and Hebron University to gauge the efficacy of medical education systems in the Palestinian medical faculties in incorporating the Alzheimer’s disease (AD) and dementia concepts within the existing medical curricula, a comprehensive assessment is required. The neuroscientific and neuroanatomical courses during the foundational years counting three hours of credit cover AD and dementia in their intended learning objectives. Furthermore, these subjects are addressed during the clinical years within the three-week neurology rotation. The study was done as a cross-sectional online survey undertaken to gauge these parameters of dementia and Alzheimer’s disease among medical students in Palestine. The study participants were recruited between 22-08-2023 and 07-11-2023. The study design allowed us to collect data from the different geographical areas of the Palestinian medical faculties in the West Bank and Gaza through an online accessible form that was shared on all the students’ platforms. All undergraduate medical students from the medical schools in Palestine were included in the study except the First-year students. The reason behind excluding the first-year students is that this study was carried out during their first semester of Medical School which includes no courses that tackle Alzheimer’s disease or dementia. Medical students who did not consent to participate in this study were excluded. A convenient sampling technique was used, and a total of 393 medical students participated in this study. The participants were recruited by sharing the questionnaire using Google form on their study groups, courses, and university emails. The sample size was calculated using the Raosoft Ⓡ Sample Size Calculator. The formula for determining the sample size (n) and margin of error (E) is expressed as follows: n = N* x / ((N-1) E 2 + x), where N represents the total population size. Given a margin of error (E) of 5% and a confidence level of 95%, with a population size (N) of 15000 and a response distribution of 50%, the formula utilizes a z score of 1.96. The minimum recommended sample size was 375. Having an adequate sample size helped reduce the random error.
2.2. Ethical and consent approval
Ethical approval was taken from the institutional review board at An-Najah National University (Ref: Med. August. 2023/34). A statement of the study’s objectives and purposes was included in the opening section of the questionnaire. The statement included that the collected data would be evaluated and analyzed anonymously and no personal identifiers were collected. The participants were informed of the voluntary nature of their participation in this study and they could withdraw from the study at any time. They were informed that by submitting their response to the questionnaire on Google Forms, they are considered to have agreed to willingly participate in this study. In addition, no personal information was obtained from the participants.
2.3. Data collection instrument
The data collection was collected through a survey using Google Forms and evacuated into Google Spreadsheets. The survey was divided into three sections: the first section included sociodemographic information: age, gender, year of study, university, marital status, residence type, experience with dementia, and source of information. The second section included the 30 items of the Alzheimer’s Disease Knowledge Scale (ADKS), a validated and reliable measurement tool [ 12 ], while the third section included the Dementia Attitudes Scale (DAS), a scale with established validity and reliability [ 13 ]. The ADKS consists of 30 True/False items divided into 7 domains, tackling the symptoms (4 items), risk factors (6 items), assessment and diagnosis (4 items), course of the disease (4 items), life impact (3 items), caregiving (5 items), and treatment (4 items). The incorrect answer was given 0 points while the correct answer was given 1 point, and the sum of the correct answers was used to calculate the total ADKS. We used the ADKS as it is suitable to assess the knowledge of Alzheimer’s disease among medical students [ 14 ]. The DAS is a scale consisting of 20 items, with a 7-point Likert scale. The responses to the Likert scale were “Strongly Disagree”, “Disagree”, “Slightly Disagree”, “Neutral”, Slightly Agree”, “Agree”, and “Strongly Agree”. The total scores of the responses to the 20 items of the DAS range from 20 to 140. It is divided into two main domains, the Dementia Knowledge domain (items 3,7,10,11,12,14,15,18,19,20), and the Social Comfort domain (items 1,2,4,5,6,8,9,13,16,17). Each of the two domains has scores ranging from 10 to 70, and the sum of the two domains gives the total DAS score. The negatively worded items of the DAS (2, 6, 8, 9, 16, and 17) were reversely coded. The DAS was used to assess the medical students’ attitudes toward Alzheimer’s disease and related dementia [ 13 ]. The DAS was developed to measure attitudes towards dementia for both college students and direct care workers [ 13 ]. DAS internal consistency was originally reported using Cronbach’s alpha value of 0.83–0.85. The ADKS test-retest reliability coefficient was 0.81 and Internal Consistency Cronbach’s alpha was 0.71 [ 12 ]. Furthermore, the questionnaire reliability was reevaluated by conducting a pilot study on 30 students and the total Cronbach alpha was 0.77. As a result, the questionnaire’s validity and reliability were assured before conducting the study. The questionnaire was in English, as it is the official teaching language in the medical schools in Palestine.
2.4. Data analysis
The statistical package of Social Science (SPSS) version 26.0 and Google Spreadsheets were used for analyzing the data in this study. The sociodemographic characteristics, ADKS and its domains, and the DAS and its domains were described using descriptive statistics. Qualitative data were described as frequencies and percentages, while quantitative data were described as means and standard deviations. The Kolmogorov-Smirnov test was used to assess the normality of the continuous data, and it revealed that the ADKS (p-value < 0.001), the scores of all the ADKS domains (p-value < 0.001), the DAS (p-value < 0.001), the scores of the dementia knowledge domain (p-value < 0.001), and the score of the Social Comfort domain (p-value < 0.001) were not normally distributed. Therefore, the Mann-Whitney U-test and the Kruskal-Wallis test were used to compare the means between the groups [ 15 ]. The chi-square test was the statistical approach that was used in order to determine the differences between the groups. The Spearman correlation by ranks was used to evaluate the correlation between the ADKA and DAS. Significant differences between groups have been assessed and associations found are highlighted later on in the discussion. The significance level was set at 5%.
3.1. Sociodemographic characteristics of medical students
A total of 393 medical students from all of the medical faculties in Palestine responded to the questionnaire. Notably, 83% of the students were aged 20 years or older, with the remaining 17% of them falling below the age of 20. The mean age for all of the participants was 20.91±1.60 years. Gender distribution revealed 53.4% females and 46.6% males. The students from the pre-clinical study phase who participated in our study were about 54.7% of the total participants, while 45.3% were from the clinical study phase. The majority of the students were single (98.5%), and 60.1% of them lived in urban areas. Exploring their familiarity with dementia, only 32.6% of students reported prior experience with dementia, and their major source of information about dementia was the Internet (47.3%), followed by the curriculum (45.6%). The sociodemographic characteristics of the participants are presented in Table 1 .
- PPT PowerPoint slide
- PNG larger image
- TIFF original image
https://doi.org/10.1371/journal.pone.0304012.t001
3.2. Knowledge of medical students toward Alzheimer’s disease and dementia
The mean score on the Alzheimer’s Disease Knowledge Scale (ADKS) was 18.91 (±3.32 SD) out of 30, representing 63% of the maximum score, with the lowest and highest scores of 10 and 30 respectively. Scores across ADKS domains varied from 45.7% to 78%. Notably, the items of the risk factors domain had the lowest percent of correct scores, while the items of the assessment and diagnosis domain had the highest percent of correct scores. Clinical students had higher ADKS scores than pre-clinical students (U = 14828, Z = 3.861, and p-value < 0.001). There were no significant differences in the scores of the ADKS domains between the pre-clinical and clinical students except for the assessment and diagnosis domain, in which the clinical students had higher scores in the assessment and diagnosis domain than pre-clinical students, with U = 14213, Z = 4.669, and p-value < 0.001. The mean scores of the ADKS domains and the comparison in scores between the pre-clinical and clinical students are presented in Table 2 .
https://doi.org/10.1371/journal.pone.0304012.t002
Table 3 presents the 30 statements of the ADKS divided into the 7 domains, along with the corresponding correct answers. The most correctly answered item from the ADKS was “Alzheimer’s disease is one type of dementia.” from the assessment and diagnosis category, in which about 88% of the students answered the correct answer. The least correctly answered item from the ADKS was “When people with Alzheimer’s disease begin to have difficulty taking care of themselves, caregivers should take over right away.” from the caregiving category in which only 18% of the students answered the correct answer.
https://doi.org/10.1371/journal.pone.0304012.t003
3.3. Attitudes of medical students toward dementia
The mean Dementia Attitude Scale (DAS) was 91.68 (±3.32 SD) out of 140, representing 65.5% of the maximum score, with the lowest and highest scores of 54 and 126, respectively. The mean social comfort score was 44.27 (±7.61 SD) out of 70, with a 63.2% mean correct rate. The mean dementia knowledge score was 47.41 (±13.04 SD) out of 70, with a 67.7% mean correct rate. No statistically significant differences were observed in the DAS scores, Social comfort scores, or dementia knowledge Scores between the pre-clinical and clinical students. Detailed mean scores of the DAS domains and the comparative analysis between the pre-clinical and clinical students are presented in Table 4 .
https://doi.org/10.1371/journal.pone.0304012.t004
The results obtained for DAS items are presented in Table 5 . About 81.4% of the students disagree with the statement that they feel afraid of people with ADRD, and 70% of them disagree with the statement that they feel uncomfortable being around people with ADRD. Moreover, 55.7% of the students disagree that they cannot imagine taking care of someone with ADRD. In the knowledge domain, about 71.5% of the students agree that every person with ADRD has different needs, while 71.8% of them agree that it is important to know the past history of people with ADRD. Meanwhile, 67.4% of the students believe that a lot now can be done to improve the lives of people with ADRD.
https://doi.org/10.1371/journal.pone.0304012.t005
3.4. Relationship between Alzheimer’s disease and dementia knowledge and attitude of medical students toward dementia
As shown in Table 6 , the ADKS and DAS have a positive association (ρ = 0.227, p-value <0.001). A similar positive association between the overall ADKS and the scores of the dementia knowledge domain of the DAS was observed (ρ = 0.286, p-value <0.001). The scores of the assessment and diagnosis domain of the ADKS were positively associated with the overall DAS (ρ = 0.198, p-value <0.001), and the scores of the dementia knowledge domain of the DAS (ρ = 0.228, p-value <0.001). The scores of the caregiving domain of the ADKS were positively associated with the overall DAS (ρ = 0.204, p-value <0.001), the scores of the Social comfort domain of the DAS (ρ = 0.101, p-value = 0.046), and the scores of the dementia knowledge domain of the DAS (ρ = 0.229, p-value <0.001), respectively. On the other hand, the scores of the life impact domain of the DAS were negatively associated with the scores of the social comfort domain of the DAS (ρ = -0.113, p-value = 0.025). The scores of the course and treatment domains of the ADKS were also found to have a good association with the overall DAS and the scores of the dementia knowledge domain of the DAS.
https://doi.org/10.1371/journal.pone.0304012.t006
3.5. Factors affecting the medical students’ knowledge and attitude toward dementia
Table 7 demonstrates the factors affecting the knowledge and attitude scores of the medical students in Palestine. Medical students in the clinical phase had higher ADKS scores than students in the pre-clinical phase (U = 14828, Z = 3.861, and p-value < 0.001). Medical students living in cities had higher DAS scores than students living in villages and refugee camps (p-value = 0.044). On the other hand, there was no significant association between the age groups, gender, marital status, experience with dementia, source of information, and the Alzheimer’s disease Knowledge Score and the Dementia Attitude Scale scores.
https://doi.org/10.1371/journal.pone.0304012.t007
4. Discussion
This study aimed to assess the knowledge of Alzheimer’s disease and related dementia among medical students across Palestine, as well as to analyze their attitudes toward Dementia. Furthermore, we sought to compare the results between the pre-clinical and clinical students. Generally, our study’s findings demonstrate limited knowledge of Alzheimer’s disease and related Dementia among the medical students in Palestine, with a mean ADKS of 18.91 representing 63% of the correct answers. This finding was similar to the findings reported in previous studies in Jordan [ 16 ], Western India [ 17 ], and China [ 18 , 19 ]. Another study conducted in Hong Kong using a different tool, the Alzheimer’s Disease Knowledge (ADK) test, demonstrated poor knowledge of Alzheimer’s disease and related dementia among medical students [ 20 ]. Conversely, the level of Alzheimer’s disease knowledge of Palestinian medical students was lower than the level of knowledge of Australian medical students [ 21 ], Norwegian medical students [ 22 ], and medical students in the United Kingdom [ 23 ].
Our study revealed a significant difference in the ADKS scores between the pre-clinical and clinical students in Palestine, with the clinical students scoring higher ADKS scores than the Pre-Clinical students. This may be explained by the higher frequency of clinical students’ exposure to dementia-related courses than the pre-clinical students. The study conducted by Wang et al demonstrated that Chinese medical students’ knowledge of dementia was significantly associated with clinical practice and dementia education or training [ 18 ]. No significant differences in ADKS scores between males and females were found in our study, suggesting that gender does not significantly affect medical students’ knowledge of dementia. This finding was similar to the findings reported in the study conducted by Gong et al among the undergraduate health professional students in China [ 19 ]. On the other hand, Wang et al reported that gender was significantly associated with Chinese medical students’ knowledge of dementia [ 18 ]. The students’ reported experience with Dementia turned out to be not a significant factor that affected their knowledge of dementia. Similar results were reported among Chinese Undergraduate Health Professional students [ 19 ]. We also found that marital status, type of residency, and source of information were not significantly associated with the medical students’ knowledge of dementia.
Regarding the domains of the ADKS, the study’s findings showed that the medical students in Palestine have a defect in the knowledge concerning the risk factors of developing Alzheimer’s disease, with a mean correct rate of 45.7%, and in the knowledge concerning the caregiving of patients with dementia, with a mean correct rate of 52.6%. This finding was similar to those reported among medical students in Western India [ 17 ]. On the other hand, the students’ knowledge of the risk factors of dementia (2.74 (45.7%) ±1.28) and caregiving of patients with dementia (2.63 (52.6%) ±1.05) were lower than the reported level of knowledge concerning these domains in Norway (4.19 (69.8%) ±1.21, and 3.96 (79.2%) ± 1.01, respectively) and China (4.09 (68%) ± 1.06, and 3.08 (62%) ± 1.08, respectively) [ 19 , 22 ]. This may be attributed to the fact that about 67.4% of the Palestinian medical students who took part in our study had no experience with dementia. Our participants correctly answered 78% of the questions in the assessment and diagnosis domain, 70.7% of the questions in the treatment and management domain, and 70.5% of the questions in the course of the disease domain. This indicates that the medical students in Palestine have better knowledge of the diagnosis and management of Alzheimer’s disease and this finding was supported by the findings reported from the study of the knowledge of Alzheimer’s Disease among undergraduate healthcare students in Norwegian and Hong Kong [ 20 , 22 ]. However, the observed disparities in knowledge among medical students in Palestine, specifically their high level in diagnosis and management as opposed to their relative deficit in understanding risk factors, may be attributed to several factors. It could be due to the fact that the medical curriculum in Palestine places a greater emphasis on clinical aspects, diagnostic procedures, and therapeutic intervention, potentially enhancing students’ competencies in these areas. Conversely, the emphasis on risk factors might be not integrated as extensively into the educational curriculum, resulting in a relative knowledge gap in this particular domain. Additionally, variations in available educational resources, faculty expertise, and the overall structure of medical education programs may contribute to divergent levels of knowledge acquisition across different facets of Alzheimer’s disease. Clinical students tend to have higher scores in the diagnosis and management domain than pre-clinical students. Clinical students were more likely to correctly answer some of the questions in the other domains than the pre-clinical students; yet, they tend to have similar scores in the rest of the symptoms, risk factors, course, life impact, caregiving, and treatment domains of the ADKS.
Medical students in Palestine generally displayed a low level of positive attitude toward dementia with a mean DAS of 91.68 (±3.32 SD) out of 140 (65.5%). This finding was in line with the findings reported in previous studies of the attitude of the pre-clinical medical students at Columbia University (pre-interventional) [ 24 ], Chinese medical students [ 19 ], Australian medical students [ 21 ], and medical students in the United Kingdom (pre-interventional) [ 23 ], while medical students in Western India demonstrated relatively negative attitudes toward dementia [ 17 ]. Furthermore, the mean scores of Palestinian medical students in the Social Comfort domain of the DAS were slightly higher than the baseline mean of the Social Comfort domain for the pre-clinical students at Columbia University [ 24 ] and lower than the baseline mean for the medical students in the United Kingdom [ 23 ]. The mean scores of the dementia knowledge domain of the DAS for the Palestinian medical students were lower than the baseline mean for the pre-clinical students at Columbia University and the medical students in the United Kingdom [ 23 , 24 ]. Surprisingly, we found no significant differences in the scores of the DAS, the social comfort domain, and the dementia knowledge domain between the pre-clinical and clinical students in Palestine. Moreover, gender, marital status, experience with dementia, and source of information were not associated with the attitudes of the Palestinian medical students toward dementia. It was worth mentioning that the Palestinian medical students living in urban areas had higher DAS scores than those living in rural areas and refugee camps.
A positive correlation between the ADKS and DAS was found in our study (ρ = 0.227, p-value <0.001). A similar positive correlation between the ADKS and DAS was found among undergraduate healthcare students in China [ 19 ]. While our study found a positive association between the ADKS and the scores of the dementia knowledge domain of the DAS (ρ = 0.286, p-value <0.001), no significant associations between the ADKS and the subdomains of the DAS were found in China [ 19 ]. This indicates that the higher level of dementia knowledge possibly affects the positive attitude of Palestinian medical students toward dementia. Notably, the scores of the Palestinian medical students in the “Assessment and Diagnosis”, “Course”, “Caregiving”, and “Treatment” domains of the ADKS were positively correlated with the DAS and the dementia knowledge domain. In general, we highly recommend the evaluation of the current dementia curriculum in the medical faculties in Palestine and the incorporation of further training modules to build the capacities of the medical students in Palestine to ensure their empowerment to identify and properly manage cases of dementia.
4.1 Strengths and limitations
This is the first study that evaluates the Palestinian medical students’ knowledge of dementia and their attitudes toward dementia. The participants in this study were diverse in terms of sociodemographic variables, such as gender, phase of medical training, and type of residency, making them more likely to represent the study population. The exclusion of first-year medical students from the study enabled us to assess the current level of knowledge and attitudes of the medical students based on the current medical curriculum. Our study has some limitations. First, our study was a cross-sectional observational study; we did not introduce any intervention in the form of an educational course or training program to improve the medical students’ knowledge and attitudes toward dementia. Therefore, further prospective studies that include measures to assess the effectiveness of the medical education program on the improvement of the students’ knowledge and correct their attitudes are needed. We recommend using the findings of this study as a reference to develop further interventional studies or training modules. Secondly, we collected the data using an online Google Form as a self-administered questionnaire; no interviews with the participants were done. This might have led to a social desirability bias, in which the participants answered the attitude-related questions not based on their true beliefs, but rather according to what they considered socially acceptable. Thirdly, we used validated tools to assess the knowledge of Alzheimer’s disease and related dementia among medical students, but the ADKS contained 30 true or false questions, rather than case scenarios or multiple choice questions, which made it difficult to assess the real students’ knowledge of dementia. Fourth, the questionnaire contained over 60 items across the three sections, which probably contributed to a lower response rate to the questionnaire.
5. Conclusion
Medical students in Palestine have limited knowledge of Alzheimer’s disease and related Dementia and a relatively low level of positive attitude toward dementia. The higher the level of students’ knowledge of Alzheimer’s disease and related Dementia the more likely they tend to have a positive attitude toward dementia. There is a need for evaluation of the current dementia curriculum in the medical schools in Palestine and a need for the inclusion of more dementia training modules, especially in the pre-clinical phase. Further interventional studies to assess the impact of dementia Training/Education on the knowledge and attitudes of medical students in Palestine are needed.
Supporting information
https://doi.org/10.1371/journal.pone.0304012.s001
Acknowledgments
The authors would like to thank An-Najah National University ( www.najah.edu ) for the technical support provided to publish the present manuscript.
- View Article
- Google Scholar
- PubMed/NCBI

Advancing Alzheimer’s research and taking knowledge home
The Center for Innovation in Brain Science is developing a diverse workforce of future scientists to fill critical gaps in Alzheimer’s disease research.

(From left) Angel-Grace Charity Leslie is researching neuroinflammation as a measurement of Alzheimer’s disease progression under the guidance of Kathleen Rodgers, PhD.
Photo by Kris Hanning, UArizona Health Sciences Office of Communications
Growing up on the Navajo Nation, Angel-Grace Charity Leslie ’s life revolved around family. She took after her grandmother when it came to a love of nature, and she shared a special bond with her oldest brother.

Kathleen Rodgers, PhD, and Angel-Grace Charity Leslie’s mentoring relationship started during the URBRAIN program and continues through AZ-TRADD.
Sadly, her brother developed epilepsy while she was in elementary school.
“I was with him the first time he had a seizure. That’s when we started going to doctor’s appointments and neurology exams,” she said. “He was very sick while I was growing up and suddenly passed away in 2020. I wanted to help him and people like him. That’s what got me here.”
“Here” is the University of Arizona Health Sciences , where Leslie, a medical pharmacology doctoral candidate at the College of Medicine – Tucson is part of a unique training program at the Center for Innovation in Brain Science . Her drive to understand the brain’s connection to epilepsy led her to mentor Kathleen Rodgers, PhD , the center’s associate director of translational neuroscience and a professor of pharmacology at the College of Medicine – Tucson.
Leslie first visited UArizona Health Sciences in 2019, when she was an undergraduate student studying biology at Diné College, the nation’s first tribal college. She was one of several students who participated in the Undergraduate Readying for Burgeoning Research for American Indian Neuroscientists program, a collaborative partnership with Diné College funded by a $1.3 million National Institutes of Health grant.
URBRAIN was designed to encourage interest in neuroscience among Native American students and create a pipeline of Navajo students to advance from Diné College to neuroscience programs at top-tier research universities, including the University of Arizona.
“URBRAIN is really where I got my start with data science. This program and my mentors really molded me and made my work now as a graduate student possible,” said Leslie, who is the first URBRAIN participant to enroll at the University of Arizona.
Her current research is funded in part by another NIH training grant, Translational Research in Alzheimer’s Disease and AD-Related Dementias, or AZ-TRADD. The program is co-led by Rodgers and Roberta Diaz Brinton, PhD , director of the Center for Innovation in Brain Science and a professor of pharmacology.
Laying the groundwork to discover treatments
Alzheimer’s disease is the seventh leading cause of death in the United States and is the most common cause of dementia among older adults, according to the National Institute on Aging. Native Americans are more likely to develop Alzheimer’s disease or other forms of dementia than white or Asian Americans, according to the Alzheimer’s Association.

(From left) At the end of the URBRAIN program, Leslie presented her research to faculty and peers.
Photo by Noelle Haro-Gomez, UArizona Health Sciences Office of Communications
In a healthy brain, billions of nerve cells, or neurons, process and transmit information through electrical and chemical signals. These neurons work together to send messages to different parts of the brain and to organs and muscles throughout the body. When neurons are unable to work together, the result is a lo ss of brain function as seen in Alzheimer’s disease.
Amyloid plaques are a hallmark of Alzheimer’s disease. The immune system clears these sticky bits of protein without incident in a healthy brain. In people with Alzheimer’s, though, the plaques clump between neurons in the brain and disrupt normal cell-to-cell communication, resulting in memory loss and cognitive decline that eventually makes life-sustaining tasks like swallowing food increasingly difficult.
“We normally think of our immune system fighting off invaders like viruses and bacteria, but in this case, the immune system is treating the plaques as the invaders,” Rodgers said. “Normally, immune cells work to wall off and clear these plaques, but in Alzheimer’s disease they can’t keep up. This causes chronic inflammation, which can damage and even cause death to neurons. The immune cells themselves can become inflamed and unable to do the job they are supposed to do.”
Leslie is working with mouse models of Alzheimer’s disease to observe the actions of immune cells and the resulting inflammatory response in hopes that the information can be used as a measurement of disease progression.
“By creating a model for inflammation over time, researchers might be able use it as a baseline to determine at what stage of Alzheimer’s disease their therapeutic is most effective,” Leslie said.
Passing down and sharing knowledge
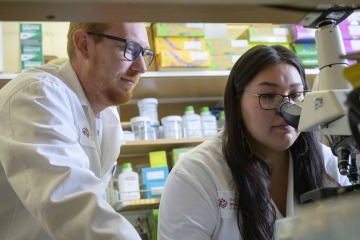
Graduate research assistant David Bradford mentored Leslie when she participated in URBRAIN and helped make her transition to the University of Arizona a smooth one.
“Angel is doing great work. The immune system is one of the most challenging systems to work with. It can almost make the bioenergetic systems of the brain look simple,” Brinton said, adding that Leslie is a great representative for URBRAIN and AZ-TRADD.
“The AZ-TRADD program was our center’s first training grant, and it has led the way for our subsequent training grants for undergraduates and junior faculty.”
As part of AZ-TRADD, students take courses ranging from bioinformatics to coding to the ethics of leadership. The program also involves a mentoring opportunity with URBAIN participants, which provides Leslie the opportunity to pass down some of what she learned to a current Diné College student.
“This university is a big place, and it could have been really daunting for me coming in as an undergraduate,” she said. “I was really lucky to have a smooth transition. David Bradford, who was a graduate student I worked with while in URBRAIN, was a big part of that. He was and continues to be a great mentor. If I can be that for someone else, I think that is really special.”
For Leslie, the training programs not only serve as steppingstones to a future career, but also are part of a journey toward helping a community she loves.
“Back home we have a saying: ‘Go into the world, learn as much as you can and bring it back’,” Leslie said. ‘Knowing that I might be able to bring this critical knowledge back to the Navajo Nation gives me a lot of pride.”
Kathleen Rodgers, PhD Associate Director, Translational Neuroscience, Center for Innovation in Brain Science, UArizona Health Sciences Professor, Department of Pharmacology, College of Medicine – Tucson Professor, Clinical Translational Sciences, College of Health Sciences Member, BIO5 Institute
Roberta Diaz Brinton, PhD Director, Center for Innovation in Brain Science, UArizona Health Sciences Professor, Department of Pharmacology, College of Medicine – Tucson Professor, Department of Neurology, College of Medicine – Tucson Professor, Evelyn F McKnight Brain Institute Professor, Department of Psychology, College of Science Professor, Department of Pharmacology and Toxicology, R. Ken Coit College of Pharmacy Professor, Clinical Translational Sciences, College of Health Sciences Member, BIO5 Institute
Related Stories
Meet a doctoral student researching treatments for brain disorders
Program empowers native students in neuroscience discovery
Brian Brennan UArizona Health Sciences Office of Communications 520-621-3510, [email protected]

An official website of the United States government
The .gov means it’s official. Federal government websites often end in .gov or .mil. Before sharing sensitive information, make sure you’re on a federal government site.
The site is secure. The https:// ensures that you are connecting to the official website and that any information you provide is encrypted and transmitted securely.
- Publications
- Account settings
Preview improvements coming to the PMC website in October 2024. Learn More or Try it out now .
- Advanced Search
- Journal List
- Health Psychol Res
- v.10(3); 2022

Impact of Alzheimer’s Disease on Caregivers in the United States
1 Trinity Preparatory School, Winter Park, FL, USA
Rohan Mangal
2 University of Miami, Miller School of Medicine, Miami, FL, USA
3 The Warren Alpert Medical School of Brown University, Providence, RI, USA
Carlos Lopez-Ortiz
4 HCA Florida Ocala Hospital, Ocala, FL, USA
Latha Ganti
5 Emergency Medicine, University of Central Florida College of Medicine, Orlando, FL, USA; Envision Physician Services, Nashville, TN, USA
Background/Objective
First discovered by Alois Alzheimer in 1906, Alzheimer’s disease (AD) has become a growing global health concern with numerous implications for individuals and modern society. As patients’ symptoms of dementia continue to worsen, they gradually grow more limited in their independence. As a result, family members and close acquaintances often become caregivers for the patient and become more involved in maintaining the patient’s lifestyle. The authors of this research sought to study what motivates caregivers to look after AD patients and the impact of AD on their lifestyles and physical, mental, and social health.
The authors crafted a survey of fourteen questions and collected data from 200 caregivers regarding AD’s impact on their physical, emotional, and social health along with their key demographic and background information (e.g. whether or not they are a caregiver for a patient with AD).
The results indicate that most AD caregivers are family members (78%). AD often strains the caregiver’s relationship with the patient (47.5%), and 58% of participating caregivers report extreme stress levels while 65% realize it is incredibly difficult to take care of a patient. Because of the increased levels of stress, 47% of participating caregivers sleep less; 43% feel more isolated from their family; and, 35.5% did not try to get help from others. Love and a strong sense of duty seem to be the primary motivation for caregivers to take care of a patient. Worry for the patient (50%), financial worries (12.5%), lack of medical experience (10.5%), and their own health (10.5%) seem to be the primary stressors for caregivers. Caregivers rely on numerous support systems and coping mechanisms, with physical activity (54.5%) and increased alcohol and marijuana usage (35.5%) the most frequent.
Conclusions
Although caregivers have support systems and found coping methods that would alleviate their burden of caring for a patient with AD, the clear negative impact AD has on caregivers affects their physical, mental, and social health. As there is currently no cure for AD, compassion and greater support for caregivers are necessary.
Introduction
First discovered in a 50-year-old woman by Alois Alzheimer in 1906, Alzheimer’s disease (AD) is the progression of cognitive and functional decline caused by the death of neurons. AD primarily targets the brain during the initial stages of the disease including the inability to encode and store new memories. Changes in the patient’s cognition and behavior signify that the disease progressed into the later stages. Alterations in amyloid precursor protein (APP) cleavage and production of APP fragment beta-amyloid along with hyperphosphorylated tau protein production, and risk genes (e.g. ApoE) cause a reduction in synaptic strength, synaptic loss, and overall neurodegeneration. 1,2 Alzheimer’s Disease International began an evidence-based Delphi consensus on the prevalence of dementia globally in 2005. The Delphi study estimated that about 42.3 million patients are diagnosed with AD with 4.6 million new cases of dementia every year. They also concluded that the countries that have the most cases of dementia are China, the western Pacific, western Europe, and the USA. 3 Niu et al. conducted a meta-analysis of the prevalence of AD in Europe and concluded that the incidence of AD was approximately 7.02 per 1000 people per year for men and 13.25 per 1000 people annually for women with the rates increasing with age. 4 Among patients with AD, the Alzheimer’s Association concluded in 2016 that about 81% are ≥75 years old, 15% are 65-74 years old, and 4% are ≤65 years old. 5 As it is the most common form of dementia, AD has grown into a global health concern. Effective treatment for AD has become necessary to control the prevalence of AD among both US and global communities.
Currently, there are only two categories of pharmacologic therapy available for patients with AD. Patients with mild, moderate, and severe AD are recommended cholinesterase inhibitors donepezil, rivastigmine, and galantamine. Alternative therapies include following the Mediterranean diet (e.g. fresh produce, whole grains, olive oil, seafood), regular aerobic exercise, and nutraceutical huperzine A, all of which have the capacity to improve both the patient’s memory functions and common activities in their daily life. 6 While these therapies can curb the effects of AD, they can only delay the progression of AD; there is yet a solid and effective treatment for AD.
Due to the great prevalence of AD globally every year, afflicted patients gradually lose the ability to store new memories and conduct their daily activities. As a result, family members and close friends must become the patient’s caregivers and maintain the patient’s mental stability and lifestyle. The sudden change in the caregivers’ daily lifestyle, the amount of time they must dedicate to the patient, the excessive costs, and other circumstances caused caregivers to face unique circumstances, which can negatively impact their own mental health and stress levels. According to a study conducted by Barbara Grabher, caregivers are afflicted with work-related stress in addition to taking care of a patient with AD. For example, 57% of caregivers must go to work late, leave early, or take time off, 16% must take a leave of absence, and 18% had to change their job from full-time to part-time. Along with work-related stress, caregivers are afflicted with stress for finding time to take care of the patient and a decline in their own physical and mental health. 7
A study of 309 caregivers from China discovered that depression, anxiety, and sleeping problems are their most common challenges. In addition, caregivers dedicate most of their time to taking care of the patient with AD, not leaving any time for themselves. The authors of this study found out that the lower the functional status of the patient, the higher the burden for the caregiver. 8 A study conducted by Truglio-Londrigan et al. used the Kreuger and Casey systematic focus group interview method. Data was collected through transcriptions of 90-minute recorded sessions among participants. The study discovered that caregivers offer themselves up for taking care of the patient and realized that they all needed some sort of support to endure all the challenges. 9
Several other variables can contribute to the increase of stress among caregivers, including their relationship with the patient, their motivations, and financial factors. The authors of this study sought to investigate the emotional, physical, and social impact AD has on caregivers.
Recruitment and Data Collection
Two hundred (N = 200) caregivers of patients with AD within the US were surveyed through a survey research platform that utilizes organic sampling built on Random Device Engagement (RDE). Using artificial intelligence (AI) to track unique respondent identification, RDE contacts users in their natural environments. Participants can answer the survey through any device while also performing their daily activities. The survey platform has numerous partnerships with approximately 120,000 other applications and more than 700 million global users. As a result, the survey platform stimulates the randomization of participants and their eligibility for the survey through incentives specific to the user’s daily activities on their device. The advanced AI algorithm and technology prevent fraud from single users from several accounts and suspicious or illogical responses to survey questions. The survey platform uses weighting to give certain respondents more weight so that the overall survey demographics match correctly for age and gender. All results are reported with this weighting. 10
One screening question was used to determine participant eligibility for the study. The question asked whether or not the participants are currently a caregiver for a patient with AD. The subsequent thirteen questions focus on the motivations behind taking care of the patient with AD, how their duty as caregivers impacts their physical, mental, and social health, and other factors that may contribute to their stress. For some of the questions, multiple selections among the multiple choices were allowed. The final question was open-ended and designed to capture the participants’ feelings not discussed in the survey.
Statistical Analysis
Data were analyzed using JMP 16 for Mac. Participants with a household income of less than $25,000 annually were considered “low-income”. For comparing 2 x 2 contingency tables, Fisher’s two-tailed exact test was used. Ninety-five percent confidence intervals (CI) for odd ratios are Wald-based. All results were weighted to match the univariate distributions of age and gender.
Ethical considerations
HCA Centralized Algorithms for Research Rules on IRB Exemptions (CARRIE) issued study exemption #2022-475.
Due to the screening question, all participants were caregivers of a patient with AD. There were 200 participants total with 104 (52%) females and 96 (48%) males. The median age of participating caregivers was 37 years, with an interquartile range of 32-47, a maximum of 75 years, and a minimum of 16 years. 10.5% of participants were divorced, 36% married, 33.5% single, 8.5% live with their partners, and the remaining 11.5% are separated or widowed. White, black, and Hispanic caregivers make up approximately 91% of the participants. 43% are considered low-income participants (less than $50,000 annually); 34% are considered middle-income participants; and, the remaining 23% are high-income participants. Participants also reported other demographic variables such as their education, number of children, employment status, and their occupations. Each question is explored in detail in the following.
As a caregiver, what is your relationship to the patient with Alzheimer’s?
30.5% of participating caregivers reported that they are the parents of patients with AD. 5.5% of participants are the spouses of patients with AD. 16.5% are the children of patients with AD. 11.5% reported that they are close friends of the patient. 25.5% reported that they are relatives of the patient. 10.5% reported others ( Fig. 1 ).

How has Alzheimer’s disease impacted your relationship with the patient?
47.5% of participants noted a more strained relationship with the patient. 19.5% of the participants said that they have improved their relationship with the patient. 33% noted that AD has no impact on their relationship with the patient.
On a scale of 1-5, please rate your stress from being a caregiver (1 = least stress, 5 = extremely stressed)
1.5% of the participants rated their stress as 1, implying that they aren’t as stressed compared to others while being a caregiver. 8% of the participants rated their stress as a 2. And 32% of the participants gave a rating of 3. And 36% of the participants gave a rating of 4. And 22% of the participants gave a rating of 5, implying that they are the most stressed compared to other participants.
How difficult would you say is it to take care of an Alzheimer’s patient?
3.5% of participants reported that taking care of a patient with AD is very easy. 2.5% of participating caregivers said that taking care of a patient with AD is easy. 28.5% of participants noted that taking care of a patient with AD is medium (moderately difficult). 38% of participants reported that taking care of a patient is hard, and 27% thought that taking care of a patient is extremely hard. These results demonstrate that more than half of the participating caregivers thought that taking care of a patient with AD is difficult.
How has being a caregiver impacted your sleep?
47% of participants report that they became sleep deprived after becoming caregivers. 3% of participants note that they sleep more after becoming caregivers. 50% of participants report that they sleep approximately the same amount, implying that becoming a caregiver doesn’t affect their sleep as much.
What causes the most stress for you as a caregiver?
56.5% of participants are stressed because they are worried about the patient with AD. 11.5% of participants are worried about their own health and well-being. 10.5% of participants are afflicted with financial worries, which contributes to most of their stress as a caregiver. Approximately 15% of participating caregivers said that most of their stress comes from their lack of medical experience and Alzheimer’s. 3% of participants note that most of their stress comes from their current occupation. 3% commented that they aren’t stressed as a caregiver ( Fig. 2 ).

As a caregiver, approximately how many hours per week do you take care of the patient?
27.5% of participating caregivers dedicate about 10-20 hours per week to taking care of a patient. 22.5% of participants dedicate 24/7 (all their time) hours per week to taking care of a patient. 18% of participants dedicate 6-10 hours per week to taking care of a patient. This data demonstrates that more than half of the sample size dedicates at least six hours per week to taking care of a patient with AD. This would result in the caretaker having less time for themselves, which would potentially increase their stress. 20.5% of participants dedicate 4-6 hours per week to taking care of a patient. 11.5% of participants dedicate less than four hours per week to taking care of a patient.
What motivates you to care for the patient?
A majority of 75.5% of participants noted that their main motivation for taking care of a patient with AD is love. 10.5% of participating caregivers felt a sense of duty that propels them to take care of a patient. 6% of participants note that they must take care of a patient because of the lack of financial resources to hire someone else. 4.5% of participants said that there’s a financial benefit to them for taking care of a patient. 2.5% of participants are pressured by social factors to take care of the patient. 1.5% of participants said that guilt was their primary motivation ( Fig. 3 ).

After becoming a caregiver, would you say you became more socially isolated from your friends and family?
As a majority of participating caregivers dedicate most of their time to taking care of a patient, they would have less time to dedicate to themselves, family, and friends. Interestingly, a majority of 57% of participants stated that they aren’t socially isolated from their friends and family after becoming a caregiver. Only 43% of participants said that they feel more socially isolated from their friends and family. Using Fisher’s exact test, the authors didn’t find any significant differences in the prevalence of social isolation among participating caregivers across gender, the number of children, race or income. A majority of social isolation seems to occur in the middle-aged groups, such as 25-34 and 35-44 years old ( Fig. 4 ), and this was statistically significant when compared to the younger age group of 18-24 (p = 0.0274). On univariate analysis, education was also statistically significant for the responses (p = 0.0008).

Have you done anything to alleviate your stress?
For this question, multiple responses were allowed. Increased participation in mindfulness activities (e.g. yoga), physical activity and exercise, professional help, drug use (e.g. alcohol and marijuana), getting a new hobby, and getting into unhealthy habits were found in 22.5%, 54.5%, 13.5%, 35.5%, 26.5%, and 10% and of the weighted population, respectively. About 20% of participants had not done anything to relieve their stress.
Did you ever try to get help from others?
About 64.5% of participating caregivers attempted to get help from others. On the other hand, 35.5% of participants didn’t receive help from others. A contingency table analysis was used. Having a postgraduate graduate was statistically significant (p = 0.0072, 95% CI = 1.564-17.37) for getting outside help. Postgraduate compared to university education yielded an odds ratio of 3.326, p = 0.0204, 95% CI 1.231-11.89. Participants were 233% more likely to ask for help if they have a postgraduate versus university degree and 421% higher if they have a postgraduate degree compared to a high school degree.
Are there any other factors/circumstances you would like to share about the stress as a caregiver has on you? (e.g. specific examples of how your daily life changed after becoming a caregiver, how you initially felt when you heard the patient was first diagnosed with Alzheimer’s, etc.)
For the final question, the authors created an open-ended question designed to capture any remaining feelings from the participants. As such, there wasn’t information for numerical patterns to emerge. Below are a few verbatim responses that capture the range of sentiments experienced by participating caregivers.
- “Finding time for myself.”
- “I wish our medical and elderly support system were much better than it is. We need better government support in this department.”
- “My mom doesn’t understand what is wrong with her. She will say she doesn’t feel like herself and that’s hard to relate to.”
- “Trying to keep them safe is hard.”
- “I have a soft spot for patients with Alzheimer’s, due to more and more being left alone with no family involved.”
- “I vowed to always be here for her and will not turn my back.”
- “Afraid to take care of them.”
- “It’s extremely hard to watch my loved one change and forget his family.”
AD has brought severe physical, mental, and social repercussions among caregivers, such as becoming more isolated from their friends and family as well as losing sleep, and resorting to drug usage. As the number of AD cases rises every year, more patients lose the ability to store new memories and perform daily functions. Caregivers must dedicate more time to taking care of the patient, leaving no time for themselves. As reported by our survey, AD adversely impacts wellness and increases the stress of US caregivers, which is measured by self-reported increased social isolation, and sleep deprivation. Consistent with these results, Yu et. al indicates that the decreasing level of cognitive function in patients, high hours, and lack of support for caregiving are directly related to a caregiver’s burden. 11 Because of these challenges, caregivers are likely to experience increased anxiety and depression and a decreased quality of life. 12 This accounts for the increasing levels of stress among participating caregivers who are within the class of 25 to 44 year-olds compared to younger caregivers. This is likely because middle-aged caregivers have time-consuming occupations, as well as take care of other circumstances (e.g. raising a family, financial woes).
Based on our survey’s results, the majority of caregivers are the parents, relatives, or children of their patients. This demonstrates that a majority of caregivers are family to the patient with AD. Of the relatives, it seems the caregivers who are the most stressed are 25-44 years old. When the survey asked participating caregivers to rate their stress on a scale of one to five (1 = least stress, 5 = extremely stressed), more than 58% of participants noted that they are incredibly stressed. In addition, when asked how difficult it is to take care of a patient with AD, more than half of the cohort noted that it is indeed difficult to take care of a patient. Caregivers must spend a fair amount of time taking care of the patient, such as making the patient eat and sleep at regular intervals and spending a lot of financial resources to maintain the patient’s daily lifestyle. For example, the survey asked participants approximately how many hours per week they take care of a patient. Approximately more than half of the participants dedicate at least six hours per week to take care of a patient with AD. This means that the caregivers barely have any time for themselves or for their family, adversely adding on to their stress. In addition, being close to the patient makes it more difficult for their caregivers because it’s emotionally wounding to see the patients slowly forget them. In addition to taking care of the patient with AD, middle-aged caregivers must balance other challenges, such as limited time, energy, financial resources, while also taking care of their own children. In addition, according to Liu et al, older females and spouses play an important role in providing family care for patients with AD. 8 Due to these unique challenges, participating middle-aged caregivers may experience increased stress, anxiety, and even depression.
AD also has repercussions on the social health of participating US caregivers ( Fig. 5 ).

For example, it has the capacity to impact the relationship between caregivers and the patient. When the survey asked participants how AD impacts their relationship with the patient, nearly half of the participants (47.5%) noted a more strained relationship with the patient. As AD gradually degrades the connections between neuron cells and eventually the neuron cells themselves, the patient would gradually lose their memories, especially the memories regarding their identities and relationships with their close friends and family. Because of this, caregivers’ relationships with patients (especially if they’re relatives of the patient) can become more strained. Surprisingly, about one-third of the sample size said that AD doesn’t impact their relationship with the patient. Perhaps these specific participants are hospital caregivers who are assigned to the patient, so they wouldn’t have any emotional attachments to the patient. Other possibilities could be that the caregivers are motivated by monetary factors so that they won’t feel any attachments toward the patient. Relations with the patient seemed to improve for 19.5% of the participating caregivers. This could result from the increasing involvement of the caretaker with the patient’s lifestyle. In addition, they could feel closer to the patient when they comfort the patient from anxiety and depression. In addition, when the survey asked whether participants felt more socially isolated from their friends and family. Interestingly, a majority of participating caregivers said that they don’t feel isolated from their friends and family. This is probably because they realize the numerous challenges that come with taking care of a patient with AD. Therefore, they likely rely on a variety of support systems (e.g. friends and family) to help them endure the challenges. As a result, they would feel more connected with their family instead of becoming more isolated. On the other hand, 43% of the participants become more socially isolated from their friends and family. These participants probably wouldn’t have as many support systems. Because taking care of a patient takes a tremendous amount of time, the caregivers would have less time for themselves and their families. As a result, they would feel more isolated from their friends and family. In addition, when the survey inquired whether caregivers received more help from others, a majority (64.5%) tried to find help. Because of the numerous challenges, the stress levels of caregivers would increase. Therefore, they would rely on the people around them, their friends and family, to help them get through the process. while 35.5% didn’t attempt to find help. A minority probably didn’t find help probably because they didn’t have the financial resources to get help. These results are aligned with other existing reports. The greater cognitive, psychological, behavior, and motor impairment of patients with AD, the higher the levels of burden and distress in their caregivers, who, as a result, adopt coping strategies and seek familial and social support. 13
AD also has the potential to impact the physical health of US caregivers. Interestingly, the survey reports that half of the participants sleep a regular amount while 47 lose more sleep after becoming caregivers. If caregivers are sleeping the normal amount, it implies that they have a great support system that allows them to receive more hours to sleep. On the other hand, caregivers are dedicated a lot of time to patients and maintaining their lifestyle (e.g. making the patient sleep and eat), along with their own family, occupations, and other circumstances that would increase their stress levels. In addition to sleeping issues, the eating habits of caregivers are also negatively impacted by AD. For example, Rivière et. al discovered that there’s a direct relationship between the decline of cognition and motor functions in patients with AD and the decline of healthy eating habits in caregivers. 14
Caregivers have a variety of motivations to take care of patients along with factors that contribute the most to their stress and methods to alleviate their own stress. A majority of participants (75.5%) noted that their main motivation for taking care of patients with AD is their love for the patient (possibly as their close friend or family). A fair amount of participants mentioned a sense of duty as being their main drive to take care of the patient. This demonstrates the variety of motives that drive caregivers to take care of patients with AD. In addition, several factors contribute to the high-stress level of caregivers. In line with the results of Grabher et. al, worries for their loved ones and financial strains seem to be the top stressors for US caregivers. As a result of the numerous difficulties they faced, several caregivers came up with coping strategies. According to our survey, a majority of people seemed to use healthy coping strategies (e.g. yoga, physical exercise, or getting professional help). On the other hand, because low-income participants seemed to be the major demographic in this sample size, a noted increase in drug usage (e.g. alcohol and marijuana) seemed to be the less-expensive method of relieving their stress. In addition, another study discovered that emotion-focused coping, associated with religion and spirituality, was used most commonly among caregivers and can reduce the symptoms of depression and anxiety. 15
In summary, several motivations and incentives drive caregivers to take care of patients with AD, with love and financial woes being the primary ones. Because of the vast amount of resources it takes to care for a patient, caregivers are expected to become more involved in the patient’s daily lives, thus leaving them with less time for themselves, their family, and their occupations. As a result, the stress can increase, which will adversely impact their physical, mental, and social health. Caretakers must rely on a support system (e.g. their friends and family) and coping strategies in order to mediate their increasing level of stress. Coping strategies can include healthy ones, such as physical exercise or seeking professional help, but it can also include cheaper, unhealthy ones, such as resorting to drinking and smoking. Because AD doesn’t yet have a cure, caregivers are the primary workforce to take care of patients and are expected to be increasingly involved in maintaining a patient’s life.
Limitations
These results, while representative of the US geographic population, are limited to caregivers who are registered to complete surveys with the survey platform. Possibly because of monetary and other incentives given by the research survey platform, the majority of participants seem to come from low-income participants. In this survey, approximately 43% of participants are of low-income status while the national average is 11.4%. 16 Possibly due to personal reasons, a handful of participants preferred not to disclose their income or occupation. Our slightly disproportionate sample indicates that our findings may not fully represent all US caregivers. In addition, the survey questions may not have been completely eliminated in biases despite the authors’ best efforts. Participants may be biased to give optimistic or conversely, exaggerated answers to the questions, so there’s a chance that the results aren’t completely accurate. For example, 30.5% of caregivers reported being the parents of patients with AD. This result seems unlikely since AD commonly occurs in the elderly population. Finally, this study’s small sample size (N = 200) makes the results more restricted in representing the overall population of US caregivers in comparison to having a larger sample size.
Future investigative measures should include looking into the specific experiences of caregivers as well as other factors, such as whether caregivers are officially diagnosed with mental disorders (e.g. clinical depression, anxiety), the stress of caregivers who care for patients with other neurodegenerative diseases such as dementia and Parkinson disease, and areas of improvement for specific support systems and healthier coping strategies for caregivers.
AD degrades the cognitive, behavioral, psychological, and motor functions of thousands of patients in the US every year. The gradual progression of the disease causes an increase in stress and grief in caregivers, negatively impacting their physical, mental, and social health (e.g. becoming more isolated from their friends, and losing sleep). Because of this, caregivers have been leaning on recent support systems and coping mechanisms to deal with stress. This study demonstrates that compassion, comfort, and greater support for caregivers is needed to alleviate their burden.
This research was supported (in whole or in part) by HCA Healthcare and/or an HCA Healthcare affiliated entity. The views expressed in this publication represent those of the author(s) and do not necessarily represent the official views of HCA Healthcare or any of its affiliated entities.
- 1. Soria Lopez JA, González HM, Léger GC. Alzheimer’s disease. Handbook of clinical neurology . 2019;167:231-255. doi:10.1016/b978-0-12-804766-8.00013-3 [ PubMed ] [ Google Scholar ]
- 2. Ballard C, Gauthier S, Corbett A, Brayne C, Aarsland D, Jones E. Alzheimer’s disease. Lancet (London, England) . 2011;377(9770):1019-1031. doi:10.1016/s0140-6736(10)61349-9 [ PubMed ] [ Google Scholar ]
- 3. Ferri CP, Prince M, Brayne C, et al. Global prevalence of dementia: a Delphi consensus study. Lancet (London, England) . 2005;366(9503):2112-2117. doi:10.1016/s0140-6736(05)67889-0 [ PMC free article ] [ PubMed ] [ Google Scholar ]
- 4. Niu H, Álvarez-Álvarez I, Guillén-Grima F, Aguinaga-Ontoso I. Prevalence and incidence of Alzheimer’s disease in Europe: A meta-analysis. Prevalencia e incidencia de la enfermedad de Alzheimer en Europa: metaanálisis. Neurologia (Barcelona, Spain) . 2017;32(8):523-532. doi:10.1016/j.nrl.2016.02.016 [ PubMed ] [ Google Scholar ]
- 5. Alzheimer’s Association. 2016 Alzheimer’s disease facts and figures. Alzheimer’s dementia : the journal of the Alzheimer’s Association . 2016;12(4):459-509. doi:10.1016/j.jalz.2016.03.001 [ PubMed ] [ Google Scholar ]
- 6. Weller J, Budson A. Current understanding of Alzheimer’s disease diagnosis and treatment. F1000Research . 2018;7:F1000 Faculty Rev-1161. doi:10.12688/f1000research.14506.1 [ PMC free article ] [ PubMed ] [ Google Scholar ]
- 7. Grabher BJ. Effects of Alzheimer Disease on Patients and Their Family. Journal of nuclear medicine technology . 2018;46(4):335-340. doi:10.2967/jnmt.118.218057 [ PubMed ] [ Google Scholar ]
- 8. Liu S, Li C, Shi Z, et al. Caregiver burden and prevalence of depression, anxiety and sleep disturbances in Alzheimer’s disease caregivers in China. Journal of clinical nursing . 2017;26(9-10):1291-1300. doi:10.1111/jocn.13601 [ PubMed ] [ Google Scholar ]
- 9. Truglio-Londrigan M, Slyer JT. Caregiver decisions along the Alzheimer’s disease trajectory. Geriatric nursing (New York, NY) . 2019;40(3):257-263. doi:10.1016/j.gerinurse.2018.10.015 [ PubMed ] [ Google Scholar ]
- 10. Rothschild D, Konitzer T. Random Device Engagement (RDE) with Organic Samples . Pollfish® Inc; 2020. Accessed June 30, 2022. https://resources.pollfish.com/market-research/random-device-engagement-and-organic-sampling [ Google Scholar ]
- 11. Yu H, Wang X, He R, Liang R, Zhou L. Measuring the Caregiver Burden of Caring for Community-Residing People with Alzheimer’s Disease. PloS one . 2015;10(7):e0132168. doi:10.1371/journal.pone.0132168 [ PMC free article ] [ PubMed ] [ Google Scholar ]
- 12. Alzheimer’s Association. 2015 Alzheimer’s disease facts and figures. Alzheimer’s dementia: the journal of the Alzheimer’s Association . 2015;11(3):332-384. doi:10.1016/j.jalz.2015.02.003 [ PubMed ] [ Google Scholar ]
- 13. Raggi A, Tasca D, Panerai S, Neri W, Ferri R. The burden of distress and related coping processes in family caregivers of patients with Alzheimer’s disease living in the community. Journal of the neurological sciences . 2015;358(1-2):77-81. doi:10.1016/j.jns.2015.08.024 [ PubMed ] [ Google Scholar ]
- 14. Rivière S, Gillette-Guyonnet S, Andrieu S, et al. Cognitive function and caregiver burden: predictive factors for eating behaviour disorders in Alzheimer’s disease. International journal of geriatric psychiatry . 2002;17(10):950-955. doi:10.1002/gps.724 [ PubMed ] [ Google Scholar ]
- 15. Monteiro AMF, Santos RL, Kimura N, Baptista MAT, Dourado MCN. Coping strategies among caregivers of people with Alzheimer disease: a systematic review. Trends in psychiatry and psychotherapy . 2018;40(3):258-268. doi:10.1590/2237-6089-2017-0065 [ PubMed ] [ Google Scholar ]
- 16. Beech BM, Ford C, Thorpe RJJr, Bruce MA, Norris KC. Poverty, Racism, and the Public Health Crisis in America. Frontiers in public health . 2021;9:699049. doi:10.3389/fpubh.2021.699049 [ PMC free article ] [ PubMed ] [ Google Scholar ]
Trends and hotspots in tea and Alzheimer's disease research from 2014 to 2023: A bibliometric and visual analysis
- Meng, Xuefang
- Liang, Qian
- Wei, Yingxiu
The positive effects of tea on Alzheimer's disease (AD) have increasingly captured researchers' attention. Nevertheless, the quantitative comprehensive analysis in the relevant literatur is lack. This paper aims to thoroughly examine the current research status and hotspots from 2014 to 2023, providing a valuable reference for subsequent research. Documents spanning from 2014 to 2023 were searched from the Web of Science, and the R software, VOSviewer, and Citespace software were used for analysis and visualization. A total of 374 documents were contained in the study. The rate of article publications exhibited a consistent increase each year from 2014 to 2023. Notably, China emerged as the leading country in terms of published articles, followed by the United States and India. Simultaneously, China is also in a leading position in cooperation with other countries. Molecules emerged as the most frequently published journal, while the Journal of Alzheimer's Disease secured the top spot in terms of citations. The identified main keywords included oxidative stress, amyloid, epigallocatechin gallate, and green tea polyphenol, among others. These focal areas delved into the antioxidative and anti-amyloid aggregation actions of tea's polyphenolic components. Furthermore, the particularly way in which epigallocatechin gallate delivers neuroprotective outcomes by influencing molecules related to AD represents a focal point of research. The increasing attention from researchers on the role of tea in ameliorating AD positions it as a hot spot in the development of anti-AD drugs in the development of future. Through our generalized analysis of the current landscape and hotspots regarding tea's application in AD, this study provides an estimable reference for future research endeavors.
- Bibliometric;
- Alzheimer's disease;
- Polyphenol;
- Epigallocatechin gallate
Alzheimer's disease and coffee: a quantitative review
Affiliation.
- 1 Department of Preventive Medicine and Public Health, Faculty of Medicine, University of Cordoba, Cordoba, Spain.
- PMID: 17427282
- DOI: 10.1179/174313206X152546
Purpose: To estimate the pooled risk of coffee consumption for Alzheimer's disease (AD).
Material and methods: We have reviewed all observational studies that evaluated the association between AD risk and coffee consumption. Four studies were identified: two case-control studies and two cohorts. These studies were carried out between 1990 and 2002.
Results: There was an obvious protective effect of coffee consumption in the pooled estimate [risk estimate: 0.73 (95% confidence interval: 0.58-0.92)]. However, the homogeneity test was highly significant (p<0.01), indicating heterogeneity across the pooled studies. Pooled analysis applying the random effect model was 0.79 with 95% confidence interval overlapping unity (95% confidence interval: 0.46-1.36). Three studies assessed coffee consumption by interview questionnaire. The risk of AD in coffee consumers versus non-consumers in studies that used interview questionnaire had a pooled risk estimate of 0.70 with 95% confidence interval 0.55-0.90.
Conclusion: Although our pooled estimates show that coffee consumption is inversely associated with the risk of AD, the four studies had heterogeneous methodologies and results. Further prospective studies evaluating the association between coffee consumption and AD are strongly needed.
Publication types
- Meta-Analysis
- Alzheimer Disease / drug therapy
- Alzheimer Disease / etiology*
- Alzheimer Disease / prevention & control*
- Case-Control Studies
- Cohort Studies
- Feeding Behavior / physiology*
- Risk Factors
- Surveys and Questionnaires

IMAGES
VIDEO
COMMENTS
Background Understanding the earliest manifestations of Alzheimer's disease (AD) is key to realising disease-modifying treatments. Advances in neuroimaging and fluid biomarkers have improved our ability to identify AD pathology in vivo. The critical next step is improved detection and staging of early cognitive change. We studied an asymptomatic familial Alzheimer's disease (FAD) cohort to ...
Longitudinal data collection and sharing initiatives represent a step change for research into Alzheimer's disease (AD) progression. ... which combines various disease biomarkers into a quantitative signature of disease progression. 1 Data‐driven methods have been used to identify subtypes or clusters in progression trajectories, 10 or the ...
1. Introduction. Alzheimer's disease (AD) (named after the German psychiatric Alois Alzheimer) is the most common type of dementia and can be defined as a slowly progressive neurodegenerative disease characterized by neuritic plaques and neurofibrillary tangles (Figure 1) as a result of amyloid-beta peptide's (Aβ) accumulation in the most affected area of the brain, the medial temporal ...
The grantee organization is the Northern California Institute for Research and Education, and the study is coordinated by the Alzheimer Disease Cooperative Study at the University of California, San Diego, Calif. ADNI data are disseminated by the Laboratory of Neuro Imaging at the University of California, Los Angeles, Calif.
Introduction: In this phase of the ongoing What Matters Most study series, designed to evaluate concepts that are meaningful to people affected by Alzheimer's disease (AD), we quantified the importance of symptoms, impacts, and outcomes of AD to people at risk for or with AD and care partners of people with AD. Methods: We administered a web-based survey to individuals at risk for or with AD ...
Introduction. Alzheimer disease (AD) is a neurodegenerative disease in the brain. It is one of the most common types of dementia, accounting for about 60-80% of the total number of dementia patients (Lee et al., 2019; Spasov et al., 2019).AD usually has a chronic or progressive nature, and a variety of high-order cortical dysfunctions appear, manifested by symptoms such as cognitive decline ...
Alzheimer's disease (AD) pathology is often evident among individuals without overt signs or symptoms of dementia, suggesting that AD is best conceptualized as a continuum across both preclinical and clinical stages [1, 2].Current evidence suggests that the most commonly used clinical outcome assessments may not capture important aspects of living with AD, regardless of stage, such as ...
Findings from this quantitative assessment are fully supportive of qualitative WMM phase 1 study results INTRODUCTION Alzheimer's disease (AD) pathology is often evident among individuals without overt signs or symptoms of dementia, suggesting that AD is best conceptualized as a continuum across both preclinical and clinical stages [1, 2 ...
Purpose of review: The role of white matter damage in the progression of Alzheimer's disease and the associated cognitive symptoms is becoming increasingly clearer. This is partly because of the advent of diffusion tensor imaging, which, in combination with other quantitative MRI techniques, offers unique insights into the patholophysiology of Alzheimer's disease in vivo.
Quantitative imaging methods and analysis in Alzheimer's disease assessment. Alzheimer's disease (AD) is a complex condition gradually eroding memory and cognitive abilities. While cognitive tests aid diagnosis, their limitations include an inability to detect subtle early-stage changes, subjective interpretation influenced by various factors ...
The collection of tissue samples were supported by the UW Alzheimer's Disease Research Center (P30 AG066509), the Adult Changes in Thought study (U19 AG066567), the Nancy and Buster Alvord ...
Abstract. Several neurodegenerative diseases including Alzheimer's Disease (AD) are characterized by ubiquitin-positive pathological protein aggregates. Here, an immunoaffinity approach is utilized to enrich ubiquitylated isopeptides after trypsin digestion from five AD and five age-matched control postmortem brain tissues. Label-free MS-based ...
Abstract. We propose a single, quantitative metric called the disease evaluation factor (DEF) and assess its efficiency at. estimating disease burden in normal, control subjects ( CTRL) and ...
1 BACKGROUND. Prevalence of Alzheimer's disease and related dementias (ADRD) is high, affecting more than 10% of those aged 65 years or more, 1 with risk doubling every 5 years. 2 The Lancet Commission has identified 12 modifiable "life-course" risk factors responsible for an estimated 40% of the population attributable risk for dementia, 3 with age, genetics, and other factors accounting ...
Abstract. This paper provides some ideas for improving the quality of Alzheimer disease (AD) research projects by using the appropriate quantitative methods. Statistical tests for answering research questions are presented according to the types of design used. Recently published research studies illustrate some pitfalls to avoid and issues to ...
Introduction Dementia, a major global health concern, is an acquired disorder that causes a progressive decline in cognitive abilities, affecting learning and memory, language, executive function, complex attention, perceptual-motor skills, and social cognition. Our study aims to evaluate the knowledge and attitudes regarding dementia and Alzheimer's disease among medical students. Methods ...
Background: Alzheimer's disease affects profoundly the quality of human behavior and cognition. The very broad distribution of its severity across various human subjects requires the quantitative diagnose of Alzheimer's disease beyond the conventional tripartite classification of cohorts such as cognitively normal (CN), mild cognitive impairment (MCI), Alzheimer's disease (AD).
Given the increase in methodological pluralism in research on brain health, cognitive aging, and neurodegenerative diseases, this scoping review aims to provide a descriptive overview and qualitative content analysis of studies stating the use of participatory research approaches within Alzheimer's disease and related dementias (ADRD ...
1. Introduction. In 2010, it was estimated that 35.6 million individuals worldwide were living with Alzheimer's disease (AD) or a related dementia, prompting the World Health Organization (WHO) to call for action and to make investing in health and social systems that will improve care and services for people with dementia and their caregivers a priority (World Health Organization, 2012).
Introduction. In 2010, it was estimated that 35.6 million individuals worldwide were living with Alzheimer's disease (AD) or a related dementia, prompting the World Health Organization (WHO) to call for action and to make investing in health and social systems that will improve care and services for people with dementia and their caregivers a priority (World Health Organization, 2012).
Her current research is funded in part by another NIH training grant, Translational Research in Alzheimer's Disease and AD-Related Dementias, or AZ-TRADD. The program is co-led by Rodgers and Roberta Diaz Brinton, PhD, director of the Center for Innovation in Brain Science and a professor of pharmacology.
Briefly, both quantitative and qualitative approaches ... "Someone defended a PhD thesis about a blood test to detect Alzheimer's disease (AD) even before the first symptoms, but it is not routinely used. ... MIND-AD is an EU Joint Programme Neurodegenerative Disease Research (JPND) project, ...
The positive effects of tea on Alzheimer's disease (AD) have increasingly captured researchers' attention. Nevertheless, the quantitative comprehensive analysis in the relevant literatur is lack. This paper aims to thoroughly examine the current research status and hotspots from 2014 to 2023, providing a valuable reference for subsequent research.
Background: The What Matters Most (WMM) study was initiated to evaluate symptoms, AD-related impacts, treatment-related needs, preferences, and priorities among individuals with or at risk for Alzheimer's disease (AD) and their care partners. The objective of this qualitative study phase was to identify a comprehensive set of concepts of interest that are meaningful to individuals across the ...
Introduction: During the last decade, physical activity (PA) (or "exercise") has been identified as one of the main modifiable factors that influence the development of Alzheimer's disease (AD) pathophysiology. We performed an umbrella review to summarize the evidence on the association between PA/exercise and the risk of developing AD risk, and the effect of exercise interventions on the ...
The authors of this research sought to study what motivates caregivers to look after AD patients and the impact of AD on their lifestyles and physical, mental, and social health. ... Alzheimer's Association. 2015 Alzheimer's disease facts and figures. Alzheimer's dementia: the journal of the Alzheimer's Association. 2015;11(3) ...
The positive effects of tea on Alzheimer's disease (AD) have increasingly captured researchers' attention. Nevertheless, the quantitative comprehensive analysis in the relevant literatur is lack. This paper aims to thoroughly examine the current research status and hotspots from 2014 to 2023, providing a valuable reference for subsequent research. Documents spanning from 2014 to 2023 were ...
Purpose: To estimate the pooled risk of coffee consumption for Alzheimer's disease (AD). Material and methods: We have reviewed all observational studies that evaluated the association between AD risk and coffee consumption. Four studies were identified: two case-control studies and two cohorts. These studies were carried out between 1990 and 2002.